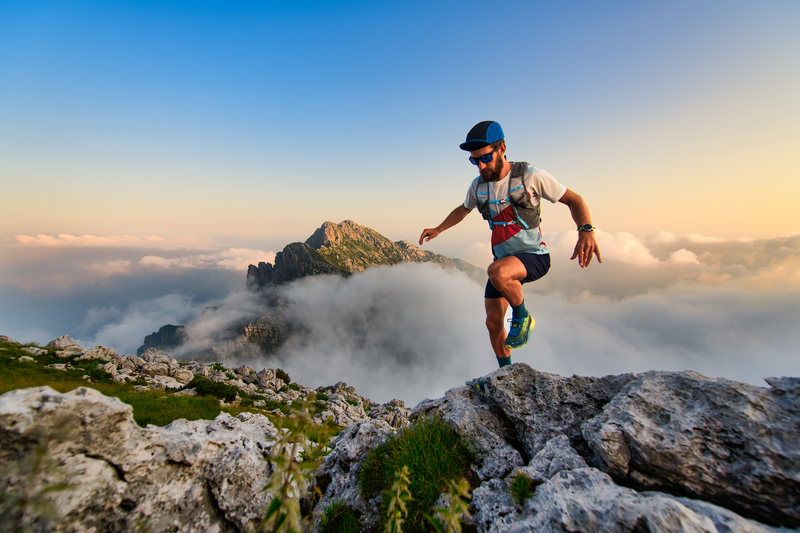
94% of researchers rate our articles as excellent or good
Learn more about the work of our research integrity team to safeguard the quality of each article we publish.
Find out more
ORIGINAL RESEARCH article
Front. Artif. Intell. , 06 October 2022
Sec. AI for Human Learning and Behavior Change
Volume 5 - 2022 | https://doi.org/10.3389/frai.2022.1006173
This article is part of the Research Topic Ethical Design of Artificial Intelligence-based Systems for Decision Making View all 9 articles
Medical artificial intelligence (AI) is important for future health care systems. Research on medical AI has examined people's reluctance to use medical AI from the knowledge, attitude, and behavioral levels in isolation using a variable-centered approach while overlooking the possibility that there are subpopulations of people who may differ in their combined level of knowledge, attitude and behavior. To address this gap in the literature, we adopt a person-centered approach employing latent profile analysis to consider people's medical AI objective knowledge, subjective knowledge, negative attitudes and behavioral intentions. Across two studies, we identified three distinct medical AI profiles that systemically varied according to people's trust in and perceived risk imposed by medical AI. Our results revealed new insights into the nature of people's reluctance to use medical AI and how individuals with different profiles may characteristically have distinct knowledge, attitudes and behaviors regarding medical AI.
Medical artificial intelligence (AI) is critical to the future of medical diagnosis and can provide expert-level medical decisions. For example, in telemedicine, it is crucial to apply medical AI for diagnoses such as COVID-19 and skin cancer (Esteva et al., 2017; Hao, 2020; Hollander and Carr, 2020; Wosik et al., 2020). This advantage is particularly critical for improving the level of medical care in poor areas of developing countries (Topol, 2019). Despite this importance, there are many barriers to applying medical AI in health-care systems (Dwivedi et al., 2021). A multitude of studies have documented these barriers, including the public not having enough AI knowledge and people expressing negative attitudes toward medical AI in social media (Promberger and Baron, 2006; Eastwood et al., 2012; Price, 2018; Cadario et al., 2021). At the behavioral level, health-care system providers are reluctant to use medical AI, and patients hold doubts about using medical AI (Longoni et al., 2019). In light of previous studies on medical AI, it is critical for scholars to develop a better holistic understanding of how knowledge, negative attitudes and behavior factors are combined to influence the acceptance of medical AI by the population.
Thus far, the most common method to explore the obstacles in the application of medical AI is to ask people to self-report variables regarding their knowledge, attitude, and behavior toward AI and then to explore the relationships among these variables by using regression-based statistical analyses (Xu and Yu, 2019; Abdullah and Fakieh, 2020; Cadario et al., 2021). This approach represents a variable-centered method in which the unique relationships of each factor with other variables are explored (Marsh et al., 2009). However, such an approach does not reveal the ways in which individuals may have knowledge, negative attitudes and behavior factors that combine to shape their profile (Ekehammar and Akrami, 2003). For example, some individuals may have high knowledge while still having high negative attitudes toward medical AI. These ideas suggest that distinct profiles of medical AI likely exist. To investigate such a possibility, a person-centered approach is needed to explore the presence of distinct subpopulations of medical AI that differentially combine knowledge, negative attitudes and behavior (Zyphur, 2009; Wang and Hanges, 2011). Unfortunately, this approach to medical AI has mostly been overlooked. A person-centered approach allows researchers to understand how knowledge of and negative attitudes and behaviors toward medical AI conjointly shape profiles by capturing unobserved heterogeneity in the way people report their knowledge, negative attitudes and behaviors toward medical AI. These profiles can be leveraged to understand the barriers and further aid the application of medical AI. For example, the profile of low knowledge of but high negative attitude toward medical AI might be used to identify public policy to reduce the negative attitude toward medical AI by increasing the science knowledge of medical AI. Overall, there is value in examining whether there exist different profiles of barriers to medical AI.
To address these questions, we adopt the knowledge, attitudes and behavior (KAB) model (Kemm and Close, 1995; Yi and Hohashi, 2018) to understand the barriers to medical AI. The KAB model is particularly helpful and relevant for understanding and explaining the barriers to adopting medical AI. The core tenet of this model is that knowledge, attitudes, and behaviors are three related factors that are used to promote technology diffusion (Hohashi and Honda, 2015). Importantly, this model recognizes that these three factors are useful at identifying barriers to technology. Moreover, scholars have identified that the distinction between subjective knowledge and objective knowledge is important to understanding the barriers to medical AI. For instance, one recent study found that subjective knowledge of medical AI drives healthcare provider utilization (Cadario et al., 2021). Moreover, they found that greater subjective knowledge of medical decisions made by humans than medical AI providers contributes to medical AI aversion. Their findings imply how reluctance to utilize medical AI is driven both by the difficulty of subjectively understanding how medical AI makes decisions and by their objective understanding of human decision making. Drawing upon the KAB model, we investigate the profiles of heterogeneity in medical AI's objective knowledge, subjective knowledge, negative attitudes, and behavior.
Therefore, the objective of this research was to identify and describe the diversity in people's reluctance to use medical AI and its associated antecedents by employing latent profile analysis (LPA) (Woo et al., 2018). Specifically, we first tried to establish KAB profiles of medical AI in Study 1. Then, we sought to replicate and theoretically develop KAB profiles of medical AI in Study 2. Moreover, we tried to theoretically develop the KAB profiles by addressing the antecedents.
In Study 1, we use an inductive approach to establish profiles of medical AI (Woo and Allen, 2014). A person-centered approach can establish quantitatively distinct profiles that differ in the levels of objectivity and knowledge of and negative attitudes and behaviors toward medical AI; it can also create qualitatively distinct profiles varying in the relative degree of objective knowledge and subjective knowledge of negative attitudes and behaviors toward medical AI. For instance, one profile may include people with high objective and subjective knowledge of as well as negative attitudes and behavior toward medical AI, while another includes low levels of objective and subjective knowledge of as well as negative attitudes and behavior toward medical AI. Given the various combinations that may occur, we pose the following question:
Research question: Are there distinct profiles of objective and subjective knowledge of and negative attitudes toward and behavior toward medical AI?
We recruited 328 participants online using convenience sampling. No participants were excluded. The participants provided informed consent and completed the survey. Table 1 provides demographic information on our sample.
We used the Cadario et al. (2021) three-item multiple choice test to measure the participants' objective understanding of medical AI. Each item had one correct answer for medical AI. We scored objective knowledge of medical AI by summing the correct answers. Thus, the objective knowledge of medical AI ranged from 0 to 3 (m = 1.12, SD = 0.83). Before the formal measurement, we interviewed doctors to ensure the accuracy of objective knowledge and expert validity.
We used the Cadario et al. (2021) three-item scale to measure the participants' subjective knowledge of medical AI. The participants were asked to indicate the extent to which they agreed with the included statements (1 = “don't quite understand,” 5 = “quite understand”). One sample item is “To what extent do you feel that you understand what a medical AI algorithm considers when making the medical decision” (α = 0.74).
We measured negative attitudes toward medical AI using an 8-item scale (Schepman and Rodway, 2020). The participants were asked to indicate their level of agreement with a list of statements (1 = “Strongly disagree,” 5 = “Strongly agree”). A sample item is as follows: “I find medical Artificial Intelligence sinister” (α = 0.84).
We measured the behavioral intention of medical AI use using a 5-item scale (Esmaeilzadeh, 2020). The participants were asked to indicate their level of agreement with a list of statements (1 = “Strongly disagree,” 5 = “Strongly agree”). A sample item is as follows: “I would like to use medical AI-based devices to manage my healthcare” (α = 0.84).
We first transformed raw measures of objective knowledge of medical AI, subjective knowledge of medical AI, negative attitudes toward medical AI, and behavioral intention toward medical AI use into z scores. Then, LPA was used to establish profiles of medical AI (Woo and Allen, 2014) using Mplus 8.3. We first established two profiles and then gradually increased the profiles until the model fitting index no longer improved (Nylund et al., 2007). For the model fitting index, referring to previous studies (Lo, 2001; Gabriel et al., 2015), we used the following: the log likelihood (LL), the free parameter (FP), the Akaike information criterion (AIC), the Bayesian information criterion (BIC), the sample-size-adjusted BIC (SSA–BIC), entropy, the bootstrap likelihood ratio test (BLRT), and the Lo-Mendell-Rubin likelihood ratio test (LMR). We consider the theoretical significance of the model and model indicators to identify the best-fitting model (Foti et al., 2012). The number of retained profiles should consider both the theoretical meaning of medical AI subpopulations and model indicators [lower LL, AIC, BIC, and SSA–BIC; higher entropy; and significant LMR (p < 0.05)].
Table 2 provides descriptive information for the study variables. As shown in Table 3, the 3-profile solution had low LL, AIC, and SSA-BIC. In addition, the elbow plot of BIC (Figure 1) shows that the slope of the BIC curve flattens around three profiles. Moreover, the 3-profile had significant LMR, unlike other solutions that had lower LL, AIC, and SSA-BIC. More importantly, the 3-profile had theoretical meaning for medical AI. Theoretically, as the number of profiles increased, these solutions contained redundant profiles of medical AI that modeled variants of the three main profiles. Thus, the 3-profile model can ensure theoretical parsimony while also meeting the statistical criterion. Together, these theoretical, visual and statistical considerations suggest that the 3-profile model is the best model with our data.
Figure 1. Goodness of fit of the BIC. The y-axis represents BIC (Bayesian information criterion); the x-axis represents the number of profiles (starting from 2).
Table 4 shows descriptive information of the retained profiles. As shown in Figure 2, among the 328 people who completed the questionnaires, 92 (28%) participants were classified into subtype 1, which had the lowest objective and subjective knowledge of medical AI, yet they also had a middle level of negative attitudes toward medical AI and the lowest level of behavioral intention regarding medical AI use.
Figure 2. Latent profiles KAB profiles of medical AI. The y-axis refers to the mean Z score of the participants' objective knowledge, subjective knowledge, negative attitude, and behavioral intentions.
A total of 191 (58%) participants were classified as subtype 2. The participants in this subtype showed a moderate level of objective and subjective knowledge of medical AI, yet they had the lowest negative attitudes toward medical AI and the highest behavioral intention toward medical AI use.
Forty-five (14%) participants were classified as subtype 3. The participants in this subtype showed a high level of objective and subjective knowledge of medical AI, yet they had the highest level of negative attitudes toward medical AI and a middle level of behavioral intention toward medical AI use.
We first intended to replicate the main results of Study 1; thus, we expected to find the same 3 profiles of medical AI. Accordingly, we seek to explore the following question:
Research question 1: Will three distinct KAB profiles of medical AI emerge?
We also expected to extend Study 1 by examining the antecedents of KAB profiles of medical AI in Study 2. When exploring the KAB profiles, it is critical to identify factors that can predict KAB profile membership. Previous research argues that individuals' trust and risk perception of medical AI predict their reluctance to use medical AI (Esmaeilzadeh, 2020). Thus, we pose the following question:
Research question 2: Do trust perception of medical AI and risk perception of medical AI predict KAB profile membership?
We recruited 388 participants. No participants were excluded. The participants provided informed consent and completed the survey. Table 5 provides demographic information on our sample.
We used the same three-item multiple choice test to measure the participants' objective understanding of medical AI as in Study 1.
We used the same three items to measure subjective knowledge of medical AI as in Study 1 (α = 0.75).
We used the same 8 items to measure negative attitudes toward medical AI as in Study 1 (α = 0.85).
We used the same 5 items to measure behavioral intention regarding medical AI use as in Study 1 (α = 0.79).
We measured the behavioral intention toward medical AI use using a 5-item scale (Esmaeilzadeh, 2020). The participants were asked to indicate their level of agreement with the statements (1 = “Strongly disagree,” 5 = “Strongly agree”). A sample item is as follows: “I trust the medical AI algorithms used in healthcare” (α = 0.77).
We measured the behavioral intention toward medical AI use using a 5-item scale (Esmaeilzadeh, 2020). The participants were asked to indicate their level of agreement with the statements (1 = “Strongly disagree,” 5 = “Strongly agree”). A sample item is as follows: “The risk of using medical AI-based tools for medical purposes is high” (α = 0.85).
Table 6 reports descriptive information for our variables. Table 3 reports fit information for profile solutions. Table 4 illustrates descriptive information for the retained three-profile solution. The three-solution was chosen because it had lower AIC, BIC, and SSA-BIC. It also had the highest entropy. Moreover, the elbow plot of BIC (Figure 3) shows that the slope of the curve flattens around three profiles. Theoretically, when the number of profiles of medical AI increased, these profile solutions contained redundant profiles that included variants of the three main medical AI profiles. Thus, to ensure theoretical parsimony, we identified the three-profile solution as the best-fitting model for our data.
Figure 3. Goodness of fit of the BIC. The y-axis represents BIC (Bayesian information criterion); the x-axis represents the number of profiles (starting from 2).
For research question 1, we replicated the three profiles as in Study 1. As shown in Figure 4, among the 388 people who completed the questionnaires, 93 (24%) participants were classified into subtype 1, which had the lowest objective and subjective knowledge of medical AI, yet they also had a middle level of negative attitudes toward medical AI and the lowest level of behavioral intention toward medical AI use.
Figure 4. Latent profiles KAB profiles of medical AI. The y-axis refers to the mean Z score of the participants' objective knowledge, subjective knowledge, negative attitudes, and behavioral intentions.
A total of 264 (68%) participants were classified into subtype 2. The participants in this subtype showed a moderate level of objective and subjective knowledge of medical AI, yet they had the lowest negative attitudes toward medical AI and the highest behavioral intention toward medical AI use.
Thirty-one (8%) participants were classified as subtype 3. The participants in this subtype showed a high level of objective and subjective knowledge of medical AI, yet they had the highest level of negative attitudes toward medical AI and a middle level of behavioral intention toward medical AI use.
Regarding antecedents, following previous studies (Vermunt, 2010; Asparouhov and Muthén, 2014), we used the RESTEP to test which variables are related to the profiles of medical AI. As shown in Table 7, we found that trust perception of medical AI, risk perception of medical AI, whether AI would replace my job, AI's benefit in medicine, and whether AI cooperates with humans are significant antecedents of the KAB profile membership of medical AI. Specifically, those perceiving a higher trust perception of medical AI were more likely to be in profile 2 [odds ratios (OR) = 14.91, p = 0.027] than in profile 1. Those perceiving a higher risk perception of medical AI were more likely to be in profiles 1 [odds ratios (OR) = 3.22, p = 0.026] and 3 (OR = 4.70, p = 0.048) than in profile 2. Those perceiving a higher perception of medical AI replacing my job were less likely to be in profile 3 [odds ratios (OR) = 0.17, p = 0.000] than in profile 2. Those perceiving a higher perception of medical AI's benefit in medicine were less likely to be in profiles 1 [odds ratios (OR) = 0.41, p = 0.000] and 3 (OR = 0.27, p = 0.000) than in profile 2. Those perceiving a higher trust perception of medical AI were more likely to be in profiles 2 [odds ratios (OR) = 14.91, p = 0.027] and 3 (OR = 2.84, p = 0.048) than in profile 1. Those perceiving a higher cooperation between medical AI and humans were less likely to be in profile 1 [odds ratios (OR) = 0.44, p = 0.00] than in profile 3. Those perceiving a higher cooperation between medical AI and humans were less likely to be in profile 1 [odds ratios (OR) = 0.54, p = 0.00] than in profile 2. We found no other significant results.
The results of this study showed that there is heterogeneity in people's medical AI use. We identified 3 profiles based on objective knowledge and subjective knowledge of and negative attitudes and behavioral intentions toward medical AI. First, the participants in profile 1 had the lowest objective and subjective knowledge of medical AI, yet they also had a middle level of negative attitudes toward medical AI and the lowest level of behavioral intention regarding medical AI use. Second, the participants in profile 2 showed a moderate level of objective and subjective knowledge of medical AI, yet they had the lowest negative attitudes toward medical AI and the highest behavioral intention toward medical AI use. Third, the participants in profile 3 showed a high level of objective and subjective knowledge of medical AI, yet they had the highest level of negative attitudes toward medical AI and a middle level of behavioral intention toward medical AI use.
Our research makes a variety of theoretical contributions. First, by taking a person-centered approach that categorized people into different profiles based upon their objective and subjective knowledge of and negative attitudes and behavioral intentions toward medical AI, our results depict a more holistic picture of people who are reluctant to use medical AI (Wang and Hanges, 2011). Most of our sampled individuals fell into profile 2, supporting the KAB model's hypothesis that knowledge, attitudes and behavior are related (Yi and Hohashi, 2018). That is, individuals with high knowledge have a low negative attitude and high behavioral intentions toward objects. The existence of profile 3 departs from the argument of the KAB model and the predominant variable-centered method that suggests links among knowledge, attitude and behavior instead highlighting the idea that these attributes and actions separately shape individuals' holistic picture of medical AI.
Second, while the KAB model (Chaffee and Roser, 1986; Abera, 2003) provides a useful lens through which to view the diversity of people's medical AI use, our study also gives back to this theory by revealing the shortcomings of this model. Notably, across the two samples, we did not observe a profile characterized by a middle level of objective and subjective knowledge of and middle levels of negative attitudes and behavioral intention toward medical AI, which could be a reasonable prediction derived from the KAB model. One potential explanation for this pertains to the complexity and heterogeneity of medical AI use (Cadario et al., 2021). That is, the barriers to medical AI use are not a simple phenomenon that can be completely explained by the KAB model. Instead, there is considerable heterogeneity in individuals reluctant to use medical AI. Thus, when considering complex phenomena such as medical AI use, we cannot simply use KAB to apply to this context and come to a simple conclusion.
Third, our work supports and extends the KAB model on the role of trust perception and risk perception in shaping individuals' medical AI use by developing and operationalizing a coherent framework of antecedents of medical AI use profiles. Consistent with the AI literature (Esmaeilzadeh, 2020; Dwivedi et al., 2021), trust and risk perception, AI replacing the jobs of humans, AI's benefit in medicine and AI's cooperation with humans were differentially related to medical AI use profile.
Our study results provide many practical insights indicating the importance of helping individuals, media communicators, medical doctors and enterprise managers make sense of the complexity and heterogeneity of individuals' reluctance to use medical AI. For example, medical doctors could realize that some people exhibit consistent knowledge of and attitudes and behaviors toward medical AI, but others exhibit more variability in these domains, so there is no way to reach a simple and general conclusion about this subject. Importantly, our results highlight the importance of recognizing that there may be disassociation between someone's knowledge of and negative attitudes toward medical AI. Decision makers should be cautious when giving advice to individuals even if the individuals appear to have high knowledge of medical AI. Last, decision makers and policy makers may be able to create personalized intervention and dissemination programs to improve people's knowledge of AI, especially their subjective knowledge, and to help individuals in need actively adopt AI in seeking medical care in the future.
Our research has several limitations, which may offer fruitful directions for future research. First, future research may build upon our findings to explore whether the three identified profiles of medical AI exist and new profile(s) emerge in different cultural contexts with different samples to address the representativeness of the sample. Second, as people's knowledge of and negative attitudes and behavioral intentions toward medical AI might change over time, it is possible to employ latent transition analysis (Collins and Lanza, 2009) to address the shift in the KAB profile of medical AI. Third, in our study, objective knowledge and subjective knowledge were consistent, and there was no significant difference in shaping the profile of medical AI. This may be because our sample is the general public, and there is no significant difference between their objective and subjective knowledge of medical AI. However, for professionals, such as doctors, it is still worth exploring the effects of age in shaping people's reluctance to use medical AI in depth.
The burgeoning AI literature has been limited in its understanding of the diversity in people's reluctance to use medical AI. We used LPA to better understand the heterogeneity of people's reluctance to use medical AI regarding their knowledge, negative attitudes and behavioral intentions. Our results demonstrated that different medical AI profiles consistently exist, and it is helpful to use a person-centered approach to better understand the complexity of obstacles in people's reluctance to use medical AI.
The raw data supporting the conclusions of this article will be made available by the authors, without undue reservation.
The studies involving human participants were reviewed and approved by the Ethical Committee of Jinan University. The patients/participants provided their written informed consent to participate in this study.
HW, KL, and LH conceived and designed the research. HW and LH performed the research. HW, KL, QS, and LG analyzed the data and wrote the manuscript. All authors contributed to the article and approved the submitted version.
This work was supported by the Program of National Natural Science Foundation of China (Grant Numbers 72174075 and 71801109) and Humanity and Social Science Youth Foundation of Ministry of Education of China (Grant Numbers 19YJCZH073).
The authors declare that the research was conducted in the absence of any commercial or financial relationships that could be construed as a potential conflict of interest.
All claims expressed in this article are solely those of the authors and do not necessarily represent those of their affiliated organizations, or those of the publisher, the editors and the reviewers. Any product that may be evaluated in this article, or claim that may be made by its manufacturer, is not guaranteed or endorsed by the publisher.
The Supplementary Material for this article can be found online at: https://www.frontiersin.org/articles/10.3389/frai.2022.1006173/full#supplementary-material
Abdullah, R., and Fakieh, B. (2020). Health care employees' perceptions of the use of artificial intelligence applications: survey study. J. Med. Intern. Res. 22, e17620. doi: 10.2196/17620
Abera, Z. (2003). Knowledge, attitude and behavior (KAB) on HIV/AIDS/STDs among workers in the informal sector in Addis Ababa. Ethiop. J. Health Dev. 17, 53–61. doi: 10.4314/ejhd.v17i1.9781
Asparouhov, T., and Muthén, B. (2014). Auxiliary variables in mixture modeling: three-step approaches using M plus. Struct. Eq. Model. Multidiscip. J. 21, 329–341. doi: 10.1080/10705511.2014.915181
Cadario, R., Longoni, C., and Morewedge, C. K. (2021). Understanding, explaining, and utilizing medical artificial intelligence. Nat. Hum. Behav. 5, 1636–1642. doi: 10.1038/s41562-021-01146-0
Chaffee, S. H., and Roser, C. (1986). Involvement and the consistency of knowledge, attitudes, and behaviors. Commun. Res. 13, 373–399. doi: 10.1177/009365086013003006
Collins, L. M., and Lanza, S. T. (2009). Latent Class and Latent Transition Analysis: With Applications in the Social, Behavioral, and Health Sciences. Hoboken, NJ: John Wiley & Sons.
Dwivedi, Y. K., Hughes, L., Ismagilova, E., Aarts, G., Coombs, C., Crick, T., et al. (2021). Artificial intelligence (AI): Multidisciplinary perspectives on emerging challenges, opportunities, and agenda for research, practice and policy. Int. J. Inform. Manag. 57, 101994. doi: 10.1016/j.ijinfomgt.2019.08.002
Eastwood, J., Snook, B., and Luther, K. (2012). What people want from their professionals: attitudes toward decision-making strategies. J. Behav. Decis. Mak. 25, 458–468. doi: 10.1002/bdm.741
Ekehammar, B., and Akrami, N. (2003). The relation between personality and prejudice: a variable- and a person-centred approach. Eur. J. Pers. 17, 449–464. doi: 10.1002/per.494
Esmaeilzadeh, P. (2020). Use of AI-based tools for healthcare purposes: a survey study from consumers' perspectives. BMC Med. Inform. Decis. Mak. 20, 170. doi: 10.1186/s12911-020-01191-1
Esteva, A., Kuprel, B., Novoa, R. A., Ko, J., Swetter, S. M., Blau, H. M., et al. (2017). Dermatologist-level classification of skin cancer with deep neural networks. Nature 542, 115–118. doi: 10.1038/nature21056
Foti, R. J., Bray, B. C., Thompson, N. J., and Allgood, S. F. (2012). Know thy self, know thy leader: contributions of a pattern-oriented approach to examining leader perceptions. Leadership Quart. 23, 702–717. doi: 10.1016/j.leaqua.2012.03.007
Gabriel, A. S., Daniels, M. A., Diefendorff, J. M., and Greguras, G. J. (2015). Emotional labor actors: a latent profile analysis of emotional labor strategies. J. Appl. Psychol. 100, 863–879. doi: 10.1037/a0037408
Hao, K. (2020). Doctors are Using AI to Triage COVID-19 Patients. The Tools may be Here to Stay. Boston, MA: MIT Technology Review.
Hohashi, N., and Honda, J. (2015). Concept development and implementation of family care/caring theory in concentric sphere family environment theory. Open J. Nurs. 5, 749–757. doi: 10.4236/ojn.2015.59078
Hollander, J. E., and Carr, B. G. (2020). Virtually perfect? Telemedicine for covid-19. N. Engl. J. Med. 382, 1679–1681. doi: 10.1056/NEJMp2003539
Kemm, J. R., and Close, A. (1995). Health Promotion: Theory and Practice. London: Macmillan International Higher Education.
Lo, Y. (2001). Testing the number of components in a normal mixture. Biometrika 88, 767–778. doi: 10.1093/biomet/88.3.767
Longoni, C., Bonezzi, A., and Morewedge, C. K. (2019). Resistance to medical artificial intelligence. J. Cons. Res. 46, 629–650. doi: 10.1093/jcr/ucz013
Marsh, H. W., Lüdtke, O., Trautwein, U., and Morin, A. J. S. (2009). Classical latent profile analysis of academic self-concept dimensions: synergy of person- and variable-centered approaches to theoretical models of self-concept. Struct. Eq. Model. Multidiscip. J. 16, 191–225. doi: 10.1080/10705510902751010
Nylund, K. L., Asparouhov, T., and Muthén, B. O. (2007). Deciding on the number of classes in latent class analysis and growth mixture modeling: a Monte Carlo simulation study. Struct. Eq. Model. Multidiscip. J. 14, 535–569. doi: 10.1080/10705510701575396
Price, W. N. (2018). Big data and black-box medical algorithms. Sci. Transl. Med. 10, eaao5333. doi: 10.1126/scitranslmed.aao5333
Promberger, M., and Baron, J. (2006). Do patients trust computers? J. Behav. Decis. Mak. 19, 455–468. doi: 10.1002/bdm.542
Schepman, A., and Rodway, P. (2020). Initial validation of the general attitudes towards artificial intelligence scale. Comput. Hum. Behav. Rep. 1, 100014. doi: 10.1016/j.chbr.2020.100014
Topol, E. J. (2019). High-performance medicine: the convergence of human and artificial intelligence. Nat. Med. 25, 44–56. doi: 10.1038/s41591-018-0300-7
Vermunt, J. K. (2010). Latent class modeling with covariates: Two improved three-step approaches. Polit. Anal. 18, 450–469. doi: 10.1093/pan/mpq025
Wang, M., and Hanges, P. J. (2011). Latent class procedures: applications to organizational research. Org. Res. Methods 14, 24–31. doi: 10.1177/1094428110383988
Woo, S. E., and Allen, D. G. (2014). Toward an inductive theory of stayers and seekers in the organization. J. Bus. Psychol. 29, 683–703. doi: 10.1007/s10869-013-9303-z
Woo, S. E., Jebb, A. T., Tay, L., and Parrigon, S. (2018). Putting the “person” in the center. Org. Res. Methods 21, 814–845. doi: 10.1177/1094428117752467
Wosik, J., Fudim, M., Cameron, B., Gellad, Z. F., Cho, A., Phinney, D., et al. (2020). Telehealth transformation: COVID-19 and the rise of virtual care. J. Am. Med. Inform. Assoc. 27, 957–962. doi: 10.1093/jamia/ocaa067
Xu, L., and Yu, F. (2019). Factors that influence robot acceptance. Chin. Sci. Bull. 65, 496–510. doi: 10.1360/TB-2019-0136
Yi, Q., and Hohashi, N. (2018). Comparison of perceptions of domestic elder abuse among healthcare workers based on the knowledge-attitude-behavior (KAB) model. PLoS ONE 13, e0206640. doi: 10.1371/journal.pone.0206640
Keywords: medical AI, objective knowledge, subjective knowledge, negative attitude, behavioral intention
Citation: Wang H, Sun Q, Gu L, Lai K and He L (2022) Diversity in people's reluctance to use medical artificial intelligence: Identifying subgroups through latent profile analysis. Front. Artif. Intell. 5:1006173. doi: 10.3389/frai.2022.1006173
Received: 04 August 2022; Accepted: 20 September 2022;
Published: 06 October 2022.
Edited by:
Rashid Mehmood, King Abdulaziz University, Saudi ArabiaReviewed by:
Ben Chester Cheong, Singapore University of Social Sciences, SingaporeCopyright © 2022 Wang, Sun, Gu, Lai and He. This is an open-access article distributed under the terms of the Creative Commons Attribution License (CC BY). The use, distribution or reproduction in other forums is permitted, provided the original author(s) and the copyright owner(s) are credited and that the original publication in this journal is cited, in accordance with accepted academic practice. No use, distribution or reproduction is permitted which does not comply with these terms.
*Correspondence: Lingnan He, aGVsbjNAbWFpbC5zeXN1LmVkdS5jbg==
Disclaimer: All claims expressed in this article are solely those of the authors and do not necessarily represent those of their affiliated organizations, or those of the publisher, the editors and the reviewers. Any product that may be evaluated in this article or claim that may be made by its manufacturer is not guaranteed or endorsed by the publisher.
Research integrity at Frontiers
Learn more about the work of our research integrity team to safeguard the quality of each article we publish.