- 1Geriatria, Accettazione Geriatrica e Centro di ricerca per l’invecchiamento, IRCCS INRCA, Ancona, Italy
- 2Department of Clinical and Molecular Sciences, Università Politecnica Delle Marche, Ancona, Italy
- 3Clinic of Laboratory and Precision Medicine, IRCCS INRCA, Ancona, Italy
- 4Scientific Direction, IRCCS INRCA, Ancona, Italy
- 5Department of Medical Sciences, University of Ferrara, Ferrara, Italy
- 6Department of Experimental and Clinical Medicine, Geriatric Intensive Care Unit, University of Florence, Florence, Italy
- 7Intermediate Care Unit, Nursing Home Misericordia, Pisa, Italy
- 8Unit of Geriatrics, Department of Medicine, Campus Bio-Medico University and Teaching Hospital, Rome, Italy
Background: Once the pandemic ended, SARS-CoV-2 became endemic, with flare-up phases. COVID-19 disease can still have a significant clinical impact, especially in older patients with multimorbidity and frailty.
Objective: This study aims at evaluating the main characteristics associated to in-hospital mortality among data routinely collected upon admission to identify older patients at higher risk of death.
Methods: The present study used data from Gerocovid-acute wards, an observational multicenter retrospective-prospective study conducted in geriatric and internal medicine wards in subjects ≥60 years old during the COVID-19 pandemic. Seventy-one routinely collected variables, including demographic data, living arrangements, smoking habits, pre-COVID-19 mobility, chronic diseases, and clinical and laboratory parameters were integrated into a web-based machine learning platform (Just Add Data Bio) to identify factors with the highest prognostic relevance. The use of artificial intelligence allowed us to avoid variable selection bias, to test a large number of models and to perform an internal validation.
Results: The dataset was split into training and test sets, based on a 70:30 ratio and matching on age, sex, and proportion of events; 3,520 models were set out to train. The three predictive algorithms (optimized for performance, interpretability, or aggressive feature selection) converged on the same model, including 12 variables: pre-COVID-19 mobility, World Health Organization disease severity, age, heart rate, arterial blood gases bicarbonate and oxygen saturation, serum potassium, systolic blood pressure, blood glucose, aspartate aminotransferase, PaO2/FiO2 ratio and derived neutrophil-to-lymphocyte ratio.
Conclusion: Beyond variables reflecting the severity of COVID-19 disease failure, pre-morbid mobility level was the strongest factor associated with in-hospital mortality reflecting the importance of functional status as a synthetic measure of health in older adults, while the association between derived neutrophil-to-lymphocyte ratio and mortality, confirms the fundamental role played by neutrophils in SARS-CoV-2 disease.
Introduction
On 5 May 2023, the World Health Organization officially declared the end of the SARS-CoV-2 health emergency that started 3 years earlier, on 11 March 2020. In these 3 years, according to World Health Organization (WHO) estimates, the SARS-CoV-2 virus caused about 20 million deaths and numerous other health and social problems. However, although the pandemic emergency is over, COVID-19 has not disappeared. Indeed, during the 2023-2024 winter, COVID-19 cases and hospitalization rates increased across the European Union (EU) (European Centre for Disease Prevention). Although many cases are asymptomatic, COVID-19 can still substantially increase in-hospital mortality, especially in older adults (Rizza et al., 2024). It is therefore crucial for clinicians to identify as early as possible those subjects who are at the highest risk of developing severe COVID-19 during hospitalization to optimize the use of health resources and to decrease the chance of fatal outcomes. Several studies on hospitalized patients affected by COVID-19 have been conducted with the aim of investigating demographics, clinical conditions and laboratory markers associated with severe disease outcomes (Wynants et al., 2020; Maestre-Muniz et al., 2022).
In 2020 Mendes et al. (2020) conducted a retrospective cohort study on 235 older Caucasian patients of mean age 86 ± 6.5 years, considering demographics, clinical, imaging and few routine laboratory parameters to determine predictors of in-hospital mortality related to COVID-19 in older patients. Using logistic regression and Cox proportional hazard models to predict mortality they found that male sex, crackles, a higher fraction of inspired oxygen, and functionality were independent risk factors for in-hospital mortality. In a post-hoc analysis on 1,520 patients aged ≥65 years from the HOPE COVID-19 registry, the authors found that age ≥75 years, dementia, low peripheral oxygen saturation, severe lymphopenia and qSOFA scale >1 were independent predictors of in-hospital mortality (Becerra-Muñoz et al., 2021). In a single-centre prospective study on 239 older patients (median age 85 years), Covino et al. (2021) found that regardless of disease severity, increasing age, dementia, and impairment in activities of daily living (ADL) were strong risk factors for in-hospital mortality. Finally, Ramos-Rincon et al. in a multicenter, retrospective, observational study on hospitalized COVID-19 older adults confirmed the possible relevance of preadmission clinical status on in-hospital mortality beyond parameters related to disease severity (Ramos-Rincon et al., 2021). Since the beginning of the pandemic, numerous evidence has also accumulated on the possible role of neutrophils in the severity of COVID-19 (Wu et al., 2020).
More specifically, a higher neutrophil-to-lymphocyte ratio (NLR) has been shown to predict mortality in hospitalized older adults (Di Rosa et al., 2023) and in COVID-19 patients (Alkhatip et al., 2021). Neutrophil-to-lymphocyte ratio was related to in-hospital mortality in a Spanish cohort of 177 hospitalized COVID-19 older patients with a World Health Organization ordinal scale 4 (oxygen by masque or nasal prongs) or 5 (non-invasive ventilation or high-flow oxygen) (Lozano et al., 2022). In a previous work we also found that, in geriatric patients admitted to hospital for COVID-19, beyond age, laboratory markers at admission, such as high neutrophil percentage and NLR, were among the best and independent predictors of in-hospital mortality (Olivieri et al., 2022). The predictive models vary in their results on the basis of different variables considered and could be biased by a pre-selection of variable to be included in multivariate models. Machine learning and artificial intelligence algorithms can overcome these issues (Wendland et al., 2023) and potentially enhance the predictive capabilities of models developed with traditional statistics (Riela, 2023). Casas-Rojo et al. (2023), using machine learning techniques to develop predictive models of mortality in patients with COVID-19 from the SEMI-COVID-19 registry at hospital admission, found that the model developed with machine learning technique has a better predictive capacity than a previous model developed on the same population using traditional statistical methods. In this study, using a web-based auto-machine-learning platform and an artificial intelligence decision support system, we aim to identify the main factors associated with in-hospital mortality among several clinical, anamnestic, and laboratory data routinely collected at admission in a cohort of hospitalized older adults in different Italian hospitals.
Materials and methods
Study design and participants
The present study used data of 819 patients from the Gerocovid-acute wards substudy, an observational multicenter retrospective-prospective initiative, enrolling individuals aged ≥60 years either retrospectively or prospectively, conducted in geriatric and internal medicine wards in older subjects who had been confirmed to be infected with SARS-CoV-2 by real-time reverse transcriptase-polymerase chain reaction assay regardless of the clinical symptoms. The enrollment started on 1 March 2020 and ended on 31 December 2020, with a follow-up until 30 June 2021. A complete description of the study methodology has been previously published (Trevisan et al., 2021; Okoye et al., 2022; Coin et al., 2023). The study was conducted following the STROBE guidelines. Data registration was performed using a dedicated electronic register designed by Bluecompanion (UK, France) to collect all clinical data from every investigational site across Italy. The primary outcome was in-hospital mortality, defined as death during the hospitalization.
Ethics statement
The study protocol has been approved by the Ethics Committee of the Campus Bio-Medico University (reference number 22/20 OSS) and registered under the ClinicalTrials.gov database (reference number NCT04379440). Each study site’s ethics committee approved the protocol. All statistical analyses were performed on anonymized data. All research was performed in accordance with relevant guidelines and regulations.
Data collection and preprocessing
At hospital admission, GeroCovid researchers collected data concerning the demographic data (sex, age, ethnicity), living arrangements, smoking habits, and pre-COVID-19 mobility (categorized as moving independently, using walking aid/moving with a wheelchair, moving with assistance in a wheelchair/bedridden). The presence of chronic diseases was retrieved from medical charts, in particular arterial hypertension, cardiovascular diseases (including cardiomyopathies, ischemic heart disease, heart failure, atrial fibrillation), chronic obstructive pulmonary disease (COPD), diabetes mellitus, obesity, chronic renal failure, depression, and cognitive impairment.
All laboratory biomarkers, including arterial blood gas analysis, complete blood count, albumin, glucose, potassium, sodium, chloride, blood urea nitrogen (BUN), creatinine, aspartate aminotransferase (AST), alanine aminotransferase (ALT), gamma-GT, total bilirubin, lactate dehydrogenase (LDH), high-sensitivity CRP (hs-CRP), d-dimer, procalcitonin, INR, aPTT, and fibrinogen, were measured by standard procedures.
Variables with <40% of missing values were included in the dataset as predictors. Multiple imputation was performed on missing values using the package mice (van Buuren and Groothuis-Oudshoorn, 2011), assuming they were missing at random. No missing data on the primary outcome of in-hospital mortality were present. Data balancing was not performed in this study to preserve the real-world distribution of outcomes, which is inherently imbalanced in COVID-19 mortality datasets.
Statistical analysis
Variables were summarized using descriptive statistics. Median and interquartile ranges were used for continuous variables, and frequencies and proportions for categorical measures. Mann–Whitney U and Chi-squared tests were used to evaluate differences between groups.
Just Add Data Bio (JADBIO), a web-based auto-machine-learning platform for analyzing potential biomarkers (Tsamardinos et al., 2022), was used. The platform employs a multivariate analysis approach to (i) identify the minimal set of features necessary for predicting a specific outcome, (ii) develop the optimal predictive model using those selected features, and (iii) evaluate the model’s performance. To ensure unbiased performance estimation, it uses Bootstrap Bias Corrected Cross-Validation (BBC-CV), which accounts for the testing of multiple machine learning pipelines. The classification methods include linear, ridge, and Lasso regression, decision trees, random forests (RF), and support vector machines (SVMs) with both Gaussian and polynomial kernels. To create a simple and interpretable model, the platform uses Statistically Equivalent Signatures (SES) for feature selection (Tsagris and Tsamardinos, 2018). Machine learning techniques such as penalized Cox regression, survival decision trees, and survival random forests are employed to build the predictive models. Each stage of the analysis is cross-validated to ensure fair performance evaluation of the models, with bootstrapping added to eliminate any optimism bias from overfitting.
Here, we tested configurations optimized for different criteria, namely, performance, interpretability, and aggressive feature selection. The Performance-optimized model reports the configuration with the highest expected predictive performance. The interpretability-optimized model produces the best-performing configuration among those whose predictive algorithm generates models that are humanly interpretable. The Aggressive Feature Selection model enforces the identification of minimal size feature subsets at the expense of reduced performance, on average.
JADBIO 1.4.93 with extensive tuning effort and 6 CPU was used to model the dataset on the overall 82 variables by splitting data into a training set and a test set in a 70:30 ratio. The training set was used for model training and, the test set was used for model evaluation. The outcome was in-hospital mortality. Model performance was assessed through the Harrell’s concordance index (c-index). The c-index computes the percentage of patient-pairs correctly ordered by the predictive algorithm according to their time-to-event. Censored cases are dealt with by removing the corresponding pair whenever a meaningful comparison in terms of time-to-event is not possible. A c-index of 1 indicates perfect ranking of their patients according to their relative risk while 0.5 indicates random risk assessment, and a value <0.5 corresponds to a model performing worse than random guessing.
The resulting model can be obtained upon request to the Corresponding Author and run with Java executor for the classification of COVID-19 samples based on the variables presented in the results for further explorations.
Statistical analysis was performed using R version 4.1, and Jamovi version 2.3. A two-sided p < 0.05 was regarded as statistically significant for all tests.
Results
Baseline subject characteristics
A total of 819 geriatric patients (mean age 78.5 ± 9.5) who were hospitalized at 19 investigational sites due to COVID-19 were included in the analysis. The 26% (n = 213) of the enrolled patients died during the in-hospital stay. The mean number of days from hospital admission to discharge for the recovered patients was 22.1 ± 17.8, and that for the deceased patients was 14.2 ± 13.1. The minimum number of days for which patients in the recovered group remained hospitalized was 1 day, while the maximum number was 97 days for survived patients and 76 days for deceased patients.
The clinical and laboratory characteristics of the study cohort at admission are reported in Table 1. Deceased patients were significantly older than survivors, whereas no sex-related difference was highlighted in terms of mortality. The prevalence of impaired mobility, including individuals capable of walking with a device and those confined in bed, and poor nutritional status were significantly higher among deceased patients. Overall, deceased patients had worse general conditions at admission and were characterized by a higher prevalence of cardiac (cardiomyopathy, atrial fibrillation, heart failure), central nervous system (CNS), renal, and autoimmune comorbidities. Regarding the laboratory assessments, deceased patients had significantly lower levels of hemoglobin and platelets, higher neutrophil counts, and consistently lower counts of the other leukocyte populations.
Artificial intelligence-based in-hospital mortality prediction
The dataset was split into training and test sets based on a 70:30 ratio and matching on age, sex, and proportion of events. The JADBIO’s AI system estimated the out-of-sample performance of the models produced by each configuration using Repeated 10-fold CV without dropping (max. repeats = 20). Overall, 176 configurations × 20 repeats × 10 folds = 3,520 models were set out to train. Three predictive algorithms optimized for performance, interpretability, or aggressive feature selection, were computed (details in Methods). Of notice, the three algorithms converged on the same model, computed using Ridge Cox Regression with penalty hyper-parameter lambda = 1.0. The model achieved a good predictive ability, with a concordance index of 0.774 (95% CI 0.726–0.821) (Figure 1A). The complete analysis report is available at JADBio website (JADBio, 2024).
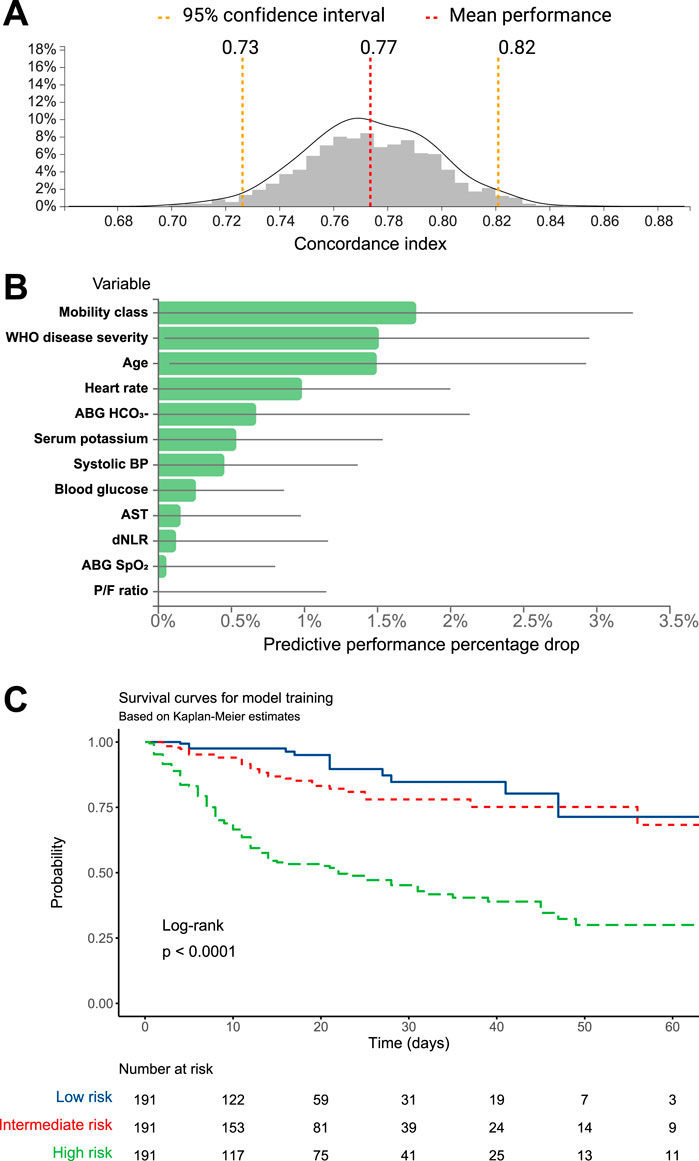
Figure 1. (A) C-statistic distributions and (B) predictive performance drop for the mortality prediction model computed on the training dataset. (C) Kaplan-Meier survival estimates for the training dataset, stratified according to categories of mortality risk.
The prediction algorithm selected 12 variables out of the 71 available variables. Variables included in the model, along with the model coefficients and derived odds ratios (OR) are reported, in order of decreasing importance, in Table 2.
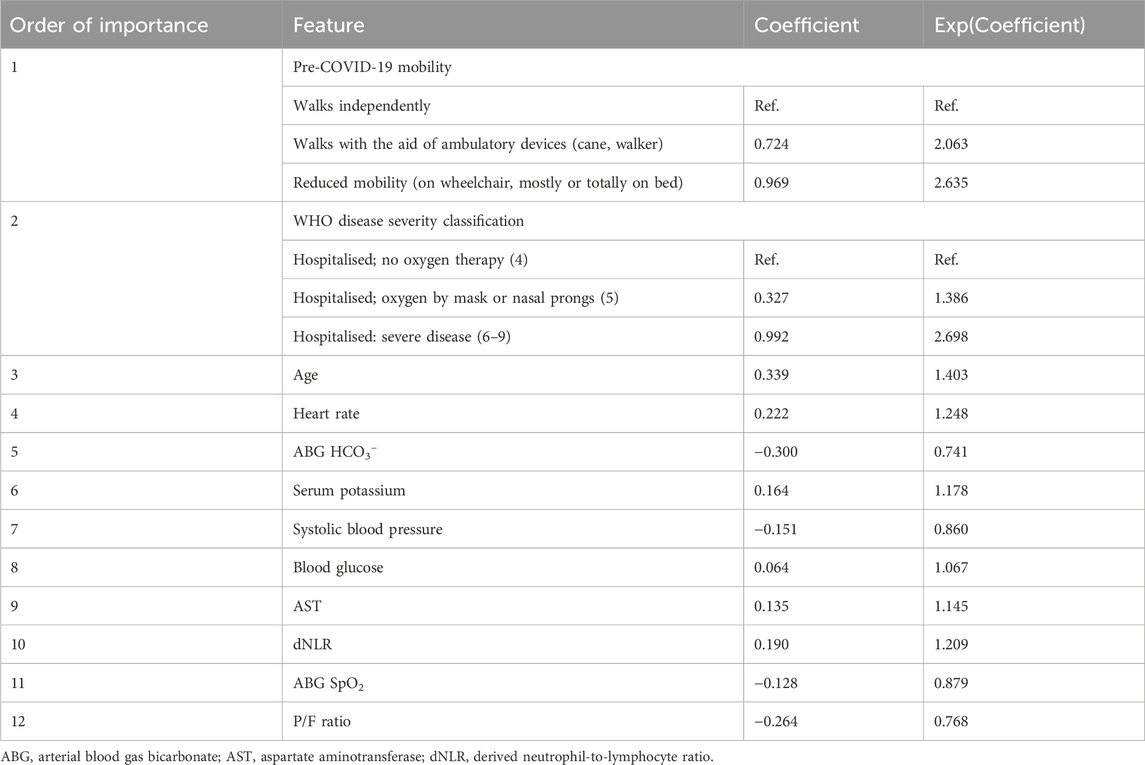
Table 2. Model coefficient for each predictor. A positive coefficient indicates that as the value of the independent variable increases, the mortality risk also increases.
The training model included age, pre-COVID-19 mobility, WHO disease severity, and variables that are routinely assessed upon hospital admission in COVID-19 patients, such as the ABG-derived parameters SpO2, HCO3−, and the P/F ratio, the vital signs systolic BP and heart rate, levels of blood glucose, aspartate aminotransferase (AST), and the derived neutrophil-to-lymphocyte ratio (dNLR, calculated as neutrophil count divided by the result of white blood cells (WBC) count minus neutrophil count).
The plots in Figure 1B show the predictive performance percentage drop that will result from removing specific variables from the model.
The model was used by the algorithm to estimate the mortality probability for each patient in the training group. Patients were then grouped based on tertiles of predicted mortality into high-, intermediate-, and low-risk. Kaplan-Meier survival functions, performed to illustrate differences in mortality according to the models’ predictions, showed that the training model achieved a significant stratification of patients according to the mortality risk (log-rank p < 0.001, Figure 1C). The Cox regression model, computed using the probability tertile as a predictor, confirmed the increasing trend of hazard ratios among groups, which were significantly different from each other (p < 0.001, Table 3).

Table 3. Survival statistics and Cox regression for in-hospital mortality prediction in the training dataset.
Significant differences among groups were highlighted in the distribution of all the variables included in the model (Figure 2). In particular, WHO disease severity, age, heart rate, and dNLR significantly increased from low-to intermediate-to high-risk patients, whereas systolic BP and the P/F ratio followed an opposite trend. Serum potassium, blood glucose, and AST were higher, whereas HCO3- and SpO2 were lower in high-risk compared to intermediate- and low-risk patients. Moreover, a progressive increase in the predicted mortality risk was observed with deteriorating pre-COVID-19 mobility.
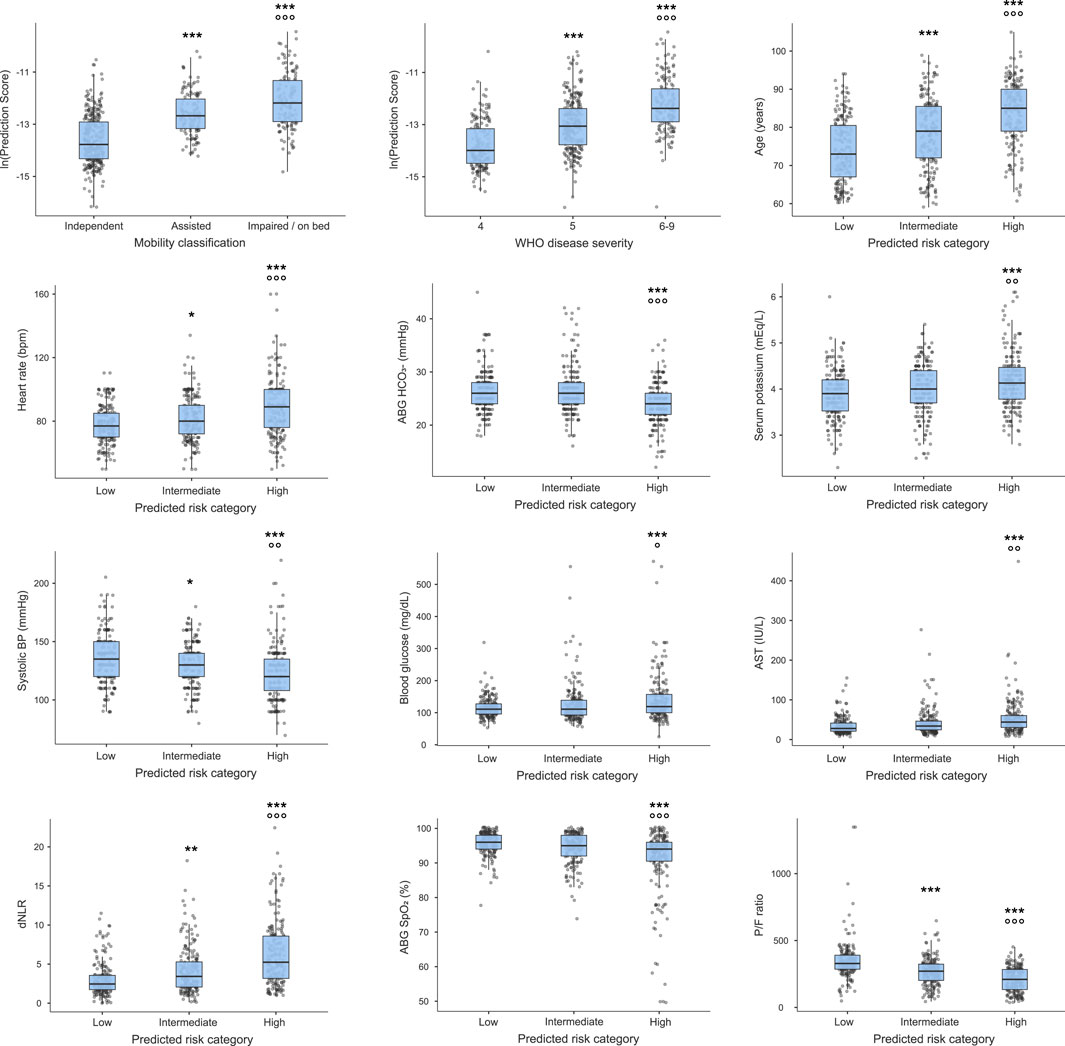
Figure 2. Risk prediction scores for the 12 predictors included in the training model. Figure legend: *p < 0.05, **p < 0.01, ***p < 0.001 vs. low-risk, walks independently, or WHO disease severity class 4; *p < 0.05, **p < 0.01, ***p < 0.001 vs. intermediate-risk, assisted mobility or WHO disease severity class 5 for Dunn’s post-hoc analysis.
Finally, the training model was validated against the test set, composed of 30% cases of the original dataset. The model maintained the performance achieved during training, with a validation concordance index of 0.774 (Figure 3A). Therefore, the survival function was computed for patients grouped according to tertiles of predicted mortality. The Kaplan-Meier survival function was statistically significant (log-rank p < 0.0001, Figure 3B), and the Cox regression computed using tertiles of predicted probability as predictors confirmed that the high-risk group had a higher mortality risk compared to the low- and intermediate-risk groups (Table 4).

Table 4. Survival statistics and Cox regression for in-hospital mortality prediction in the test set.
Discussion
In this study, from 71 available variables, including sociodemographic data, smoking habits, mobility, chronic diseases, and clinical and laboratory parameters, we identified 12 factors associated with in-hospital mortality in older inpatients with SARS-CoV-2 infection. These selected were able to detect with moderate accuracy patients at increased risk of in-hospital mortality.
Using a statistical analysis method based on machine learning and artificial intelligence allowed us to test all the variables collected during the study, avoiding variable selection bias. AI models have the advantage of capturing more complex, and not always linear, relationships between variables, offering the possibility to test several models (Ngiam and Khor, 2019). Here, routinely collected laboratory and clinical hospital admission data were integrated into a web-based machine learning platform to identify, among factors with established or putative association with unfavorable COVID-19 outcomes, those with the highest prognostic relevance in older patients.
In the final model, factors associated with a high risk of in-hospital mortality were pre-COVID-19 mobility, WHO disease severity, age, heart rate, ABG HCO3-, serum potassium, systolic blood pressure, blood glucose, AST, dNLR, ABG SpO2, P/F ratio.
As expected, factors identifying respiratory disease severity (WHO disease severity and ABG parameters) were associated with a worse prognosis (Santus et al., 2020). Other factors such as serum potassium concentration, low systolic blood pressure, higher blood glucose and AST values, and heart rate probably reflect acute organ/system failure, while age is a well-established risk factor for adverse outcomes in COVID-19 patients (Henkens et al., 2022).
One of the most relevant findings of our analysis is that mobility level before hospitalization was the only pre-morbid and the most important factor associated with in-hospital mortality. To the best of our knowledge, no other studies have investigated this association in SARS-CoV-2 older patients.
Mobility before hospitalization might be considered as a proxy of preadmission functional status and disability. Indeed, impaired activities of daily living have been shown to be associated with COVID-19 negative outcomes (Ramos-Rincon et al., 2021; Wang et al., 2022; Rodriguez-Sanchez et al., 2021; Laosa et al., 2020; Cangiano et al., 2020; Bruno et al., 2022). However, previous studies did not always address a population of very old adults, had a smaller sample size, or did not include a large set of variables. Moreover, although tools to assess functional status, such as the Barthel Index, do not require formal training, examiners need to be familiar with the functional item being assessed and the scoring system used. Instead, pre-morbid mobility is a piece of information easy to collect by any health personnel involved in the patient’s care.
A second finding of our study is that, in line with previous reports, elevated neutrophil and reduced lymphocyte counts, as reflected by the dNLR index, are important predictors of in-hospital mortality in older inpatients with SARS-CoV-2. While less impactful than other factors, the dNLR index still significantly contributed to a model encompassing variables related acute respiratory and organ dysfunction (Olivieri et al., 2022). Although neutrophils play a role in viral clearance, i.e., with the production of Interferon, they may favor the pathogenesis of SARS-CoV-2 and exacerbate its complications such as acute respiratory distress syndrome (ARDS), thrombosis, and multisystem inflammatory disease (McKenna et al., 2022) and strong evidence has been accumulated on the key role of neutrophils in severe COVID-19 pathogenesis (Zeng et al., 2021; Wang et al., 2020; Wan et al., 2020; Vafadar Moradi et al., 2021; Seyit et al., 2021; Masso-Silva et al., 2022; Ma et al., 2021; Liu et al., 2020; Li et al., 2020; Huang et al., 2020). Elevated neutrophils were found in the nasal epithelium, the lower respiratory tract, and the bloodstream in patients with SARS-CoV-2 infection (Reusch et al., 2021). Moreover, several researches showed that, in severe COVID-19 patients, neutrophils are not only abundant but also have an altered phenotype and functionality (McKenna et al., 2022; Reusch et al., 2021). In particular, increased production of neutrophil extracellular traps (NETs) has been found, with possible direct damage to the pulmonary endothelium and facilitation of the thrombosis pathway, as well as a greater presence of the neutrophil subtype responsible for the suppression of the adaptive immune response, usually typical of a chronic condition such as cancer (McKenna et al., 2022; Reusch et al., 2021).
Study limitations and strengths
Some limitations of this study should be acknowledged. Data were collected during the first and second COVID-19 waves. This implies that patients were unvaccinated, and variants of SARS-CoV-2 involved in the patients’ infection were different from those currently circulating in terms of the degree of infectivity, the ability to evade the immune response, and the severity of the disease caused.
The present analysis considered only in-hospital mortality, with no information on long-term mortality (or other long-term outcomes), although the occurrence of long-term sequelae of SARS-CoV-2 has also been widely documented.
We did not compare findings deriving from the application of machine learning algorithms with predictions based on conventional statistical methods. However, machine learning models are generally characterized by an overall better, or at least non-inferior, predictive capacity, also concerning COVID-19 outcomes (Casas-Rojo et al., 2023), and most of the predictors included in the model were previously extensively characterized for their prognostic role.
On the other hand, it should be recognized that although many studies have focused on specific aspects associated with SARS-CoV-2 disease-related mortality, only a few have comprehensively assessed a large number of factors in older inpatients, such as our study. Moreover, we considered only variables routinely collected in a hospital setting. This is particularly important since identifying clinical and laboratory parameters among those routinely collected can guide the physician in the early patient risk stratification, facilitating the assessment of the most appropriate care setting and an optimal allocation of health resources.
Conclusion
Three main conclusions can be drawn from our study. First, in a multivariable analysis encompassing all sociodemographic, acute clinical and laboratory findings, and comorbidities, mobility emerged as the strongest predictor and the only pre-morbid condition that could substantially influence in-hospital mortality in SARS-CoV-2 older adults. Second, the significant feature importance of dNLR that we observed in our model strongly confirms that in SARS-CoV-2 infection, unlike other viral infections, neutrophils play a fundamental role in the pathogenesis and worsening of COVID-19. In this context, dNLR could represent a feasible and inexpensive biomarker of COVID-19 severity in hospitalized older adults. Third, the endpoint of in-hospital mortality can be predicted with good accuracy at the time of admission using functional status indicators and commonly available laboratory results.
These findings support the application of machine learning to develop predictive algorithms based on existing clinical and laboratory variables and highlight the importance of functional status above all other chronic diseases and conditions as a synthetic health measure in older adults, which may be a strong predictor of adverse outcomes.
Data availability statement
The raw data supporting the conclusions of this article will be made available by the corresponding author on reasonable request. Requests to access the datasets should be directed to AC, a.cherubini@inrca.it.
Ethics statement
The study protocol has been approved by the Ethics Committee of the Campus Bio-Medico University (reference number 22/20 OSS) and registered under the ClinicalTrials.gov database (reference number NCT04379440). The studies were conducted in accordance with the local legislation and institutional requirements. Written informed consent for participation was not required from the participants or the participants’ legal guardians/next of kin in accordance with the national legislation and institutional requirements.
Author contributions
MF: Writing–original draft, Writing–review and editing. JS: Formal Analysis, Writing–original draft, Writing–review and editing. GD: Writing–review and editing. FS: Writing–review and editing. AB: Writing–review and editing. SV: Conceptualization, Writing–review and editing. CT: Writing–review and editing. SF: Writing–review and editing. FM: Conceptualization, Writing–review and editing. RA: Conceptualization, Writing–review and editing. FO: Writing–review and editing. AC: Conceptualization, Writing–original draft, Writing–review and editing.
GeroCovid Observational Working Group
Angela Marie Abbatecola [ASL Frosinone; RSA INI Città Bianca, Veroli (FR)], Domenico Andrieri [RSA Villa Santo Stefano, S. Stefano di Rogliano (CS)], Sara Antenucci [Ambulatorio Psicogeriatrico, Ortona (CH)], Rachele Antognoli (Azienda Ospedaliero Universitaria Pisana; RSA Villa Isabella, Pisa), Rafaele Antonelli RI (Università Campus Bio-Medico, Roma), Maria Paola Antonietti (Ospedale Regionale di Aosta), Viviana Bagalà (Azienda OspedalieroUniversitaria di Ferrara), Giulia Bandini (USL Toscana Centro, Ospedale San Jacopo, Pistoia), Salvatore Bazzano [ULSS 3 Serenissima, Presidio di Dolo (VE)], Giuseppe Bellelli (Ospedale San Gerardo, Monza), Andrea Bellio (Azienda Ospedale Università di Padova), Federico Bellotti (Azienda Ospedaliero-Universitaria di Ferrara), Enrico Benvenuti [USL Toscana Centro, Ospedale Santa Maria Annunziata, Bagno a Ripoli (FI)], Marina Bergamin (Azienda Ospedaliero-Universitaria di Parma), Marco Bertolotti (Azienda Ospedaliero-Universitaria di Modena), Carlo Adriano Biagini (USL Toscana Centro, Ospedale San Jacopo, Pistoia), Angelo Bianchetti (Istituto Clinico Sant’Anna, Brescia), Alessandra Bianchi [Spedali Civili, Montichiari (BS)], Mariangela Bianchi (Policlinico Sant’Orsola-Malpighi, Bologna), Paola Bianchi (Associazione Nazionale Strutture Territoriali e per la Terza Età, Roma), Francesca Biasin (Azienda Ospedale Università di Padova), Silvia Bignamini (Casa di Cura San Francesco, Bergamo), Damiano Blandini (Policlinico Sant’Orsola-Malpighi, Bologna), Stefano Bofelli (Fondazione Poliambulanza, Brescia), Cristiano Bontempi (Azienda Ospedale Università di Padova), Alessandra Bordignon (Azienda Ospedale Università di Padova), Luigi Maria Bracchitta (ATS Milano), Maura Bugada (Casa di Cura San Francesco, Bergamo), Carmine Cafariello [RSA Villa Sacra Famiglia, IHG, Roma; I RSA Geriatria, IHG, Guidonia (RM); III RSA Geriatria, IHG, Guidonia (RM); RSA Estensiva, IHG, Guidonia (RM); RSA Intensiva, IHG, Guidonia (RM)], Veronica Caleri (USL Toscana Centro, Ospedale San Jacopo, Pistoia), Valeria Calsolaro (Azienda Ospedaliero Universitaria Pisana; RSA Villa Isabella, Pisa), Donatella Calvani (USL Toscana Centro, Presidio Misericordia e Dolce, Prato; USL Toscana Centro, Ospedale Santo Stefano, Prato), Francesco Antonio Campagna [Centro di Riabilitazione San Domenico, Lamezia Terme (CZ)], Andrea Capasso (ASL Napoli 2 Nord), Sebastiano Capurso [RSA Bellosguardo, Civitavecchia (RM)], Silvia Carino [RSA San Domenico, Lamezia Terme (CZ); Centro di Riabilitazione San Domenico, Lamezia Terme (CZ); RSA Villa Elisabetta, Cortale (CZ); Casa Protetta Madonna del Rosario, Lamezia Terme (CZ)], Elisiana Carpagnano (Ospedale Giovanni XXIII Policlinico di Bari), Barbara Carrieri (IRCCS INRCA, Ancona), Viviana Castaldo (Presidio Ospedaliero Universitario Santa Maria della Misericordia, Udine), Manuela Castelli [Istituto Geriatrico Camillo Golgi, Abbiategrasso (MI)], Manuela Castellino [Fatebenefratelli, Presidio Ospedaliero Riabilitativo “Beata Vergine Consolata”, San Maurizio Canavese (TO)], Alessandro Cavarape (Presidio Ospedaliero Universitario Santa Maria della Misericordia, Udine), Ilaria Cazzulani (Ospedale San Gerardo, Monza), Carilia Celesti (Policlinico Universitario Campus Bio-Medico, Roma), Chiara Ceolin (Azienda Ospedale Università di Padova), Maria Giorgia Ceresini (Azienda Ospedaliero-Universitaria di Ferrara), Arcangelo Ceretti [Istituto Geriatrico Camillo Golgi, Abbiategrasso (MI)], AC (IRCCS INRCA, Ancona), Anita Chizzoli (Istituto Clinico Sant’Anna, Brescia), Erika Ciarrocchi (IRCCS INRCA, Ancona), Paola Cicciomessere (Azienda Ospedaliero Universitaria di Foggia), Alessandra Coin (Azienda Ospedale Università di Padova), Mauro Colombo [Istituto Geriatrico Camillo Golgi, Abbiategrasso (MI)], Annalisa Corsi (USL Toscana Centro, Ospedale San Jacopo, Pistoia), Antonella Crispino [RSA Villa Santo Stefano, S. Stefano di Rogliano (CS); RSA Villa Silvia, Altilia Grimaldi (CS)], Roberta Cucunato [RSA Villa Santo Stefano, S. Stefano di Rogliano (CS); RSA Villa Silvia, Altilia Grimaldi (CS)], Carlo Custodero (Ospedale Giovanni XXIII Policlinico di Bari), Federica D’Agostino [Casa Sollievo della Sofferenza, S. Giovanni Rotondo (FG)], Maria Maddalena D’Errico [Casa Sollievo della Soferenza, S. Giovanni Rotondo (FG)], Ferdinando D’Amico [RSA San Giovanni di Dio, Patti (ME); RSA Sant’Angelo di Brolo (ME)], Aurelio De Iorio (Azienda OspedalieroUniversitaria di Parma), Alessandro De Marchi [Policlinico Sant’Orsola-Malpighi, Bologna), Annalaura Dell’Armi (III RSA Geriatria, IHG, Guidonia (RM)], Marta Delmonte (Azienda OspedalieroUniversitaria di Ferrara), Giovambattista Desideri [Ospedale di Avezzano (AQ)], Maria Devita (Azienda Ospedale Università di Padova), Evelyn Di Matteo (Policlinico Universitario Campus Bio-Medico, Roma), Emma Espinosa [Azienda Ospedali Riuniti Marche Nord, Fano (PU)], Luigi Esposito [Casa Sollievo della Soferenza, S. Giovanni Rotondo (FG)], Chiara Fazio (Azienda Ospedaliero-Universitaria di Parma), Christian Ferro [RSA Sant’Angelo di Brolo (ME)], Chiara Filippini [Spedali Civili, Montichiari (BS)], Filippo Fini (Azienda Ospedale Università di Padova), Lucia Fiore [Casa Sollievo della Sofferenza, S. Giovanni Rotondo (FG)], Serafna Fiorillo [ASP Vibo Valentia; RSA Madonna delle Grazie, Filadelfa (VV); Casa di Riposo Mons. Francesco Luzzi, Acquaro (VV); Casa di Riposo Villa Betania, Mileto (VV); Casa di Riposo Pietro Rosano, Dasà (VV); Casa di Riposo Serena Diocesi, Mileto (VV); Alloggio per Anziani Villa Amedeo, Francavilla Angitola (VV); Casa Albergo Villa Fabiola, Monterosso Calabro (VV); Casa di Riposo Villa Sara, San Nicola da Crissa (VV); Casa di Riposo Don Mottola, Tropea (VV); Casa di Riposo San Francesco, Soriano Calabro (VV); RSA Anziani, Soriano Calabro (VV); Casa di Riposo Suore Missionarie del Catechismo, Pizzo (VV)], Caterina Fontana (Azienda Ospedaliero-Universitaria di Modena), Lina Forte [Ospedale di Avezzano (AQ)], Riccardo Franci Montorzi (Azienda Ospedaliero Universitaria Careggi, Firenze), Carlo SFu (Azienda Ospedaliero Universitaria Careggi, Firenze), SFu (Azienda Ospedaliero Universitaria Careggi, Firenze), Pietro Gareri (ASP Catanzaro), Pier Paolo Gasbarri (Associazione Nazionale Strutture Territoriali e per la Terza Età, Roma), Antonella Giordano (Azienda Ospedaliero Universitaria Careggi, Firenze), Evelina Giuliani [USL Toscana Centro, Ospedale Santa Maria Annunziata, Bagno a Ripoli (FI)], Roberta Granata (RSA Villa Sacra Famiglia, IHG, Roma), Antonio Greco [Casa Sollievo della Soferenza, S. Giovanni Rotondo (FG)], Nadia Grillo [RSA San Domenico, Lamezia Terme (CZ); Casa di Riposo San Domenico, Lamezia Terme (CZ); RSA Villa Elisabetta, Cortale (CZ)], Antonio Guaita [Istituto Geriatrico Camillo Golgi, Abbiategrasso (MI)], Liana Gucciardino (ASP Agrigento), Andrea Herbst (Azienda Ospedaliero Universitaria Careggi, Firenze), Marilena Iarrera [RSA Sant’Angelo di Brolo (ME)], Giuseppe Ielo (Azienda Ospedaliero-Universitaria di Parma), Valerio Alex Ippolito [Casa Protetta Villa Azzurra, Roseto Capo Spulico (CS)], Antonella La Marca [Casa Sollievo della Soferenza, S. Giovanni Rotondo (FG)], Umberto La Porta (Azienda Ospedaliero-Universitaria di Parma), Ilaria Lazzari (Policlinico Sant’Orsola-Malpighi, Bologna), Diana Lelli (Policlinico Universitario Campus Bio-Medico, Roma), Yari Longobucco (Azienda Ospedaliero-Universitaria di Parma), Francesca Lubian (Ospedale di Bolzano), Giulia Lucarelli (Azienda Ospedaliero Universitaria Careggi, Firenze; USL Toscana Centro, Ospedale San Jacopo, Pistoia), Flaminia Lucchini (Azienda Ospedaliero Universitaria Careggi, Firenze), Daniela Lucente [Spedali Civili, Montichiari (BS)], Lorenzo Maestri (Policlinico Sant’Orsola-Malpighi, Bologna), Marcello Maggio (Azienda Ospedaliero-Universitaria di Parma), Paola Mainquà [Azienda Ospedali Riuniti Marche Nord, Fano (PU)], Mariangela Maiotti [Ospedale San Giovanni Battista, Foligno (PG)], Alba Malara [RSA San Domenico, Lamezia Terme (CZ); Casa di Riposo Villa Marinella, Amantea (CS); Casa Protetta Madonna del Rosario, Lamezia Terme (CZ); Casa Protetta Villa Azzurra, Roseto Capo Spulico (CS); Centro di Riabilitazione San Domenico, Lamezia Terme (CZ); RSA Casa Amica, Fossato Serralta (CZ); RSA La Quiete, Castiglione Cosentino (CS); Casa di Riposo San Domenico, Lamezia Terme (CZ); RSA Villa Elisabetta, Cortale (CZ); RSA Villa Santo Stefano, S. Stefano di Rogliano (CS); RSA Villa Silvia, Altilia Grimaldi (CS)], Carlotta Mancini (Azienda Ospedaliero Universitaria Careggi, Firenze), Irene Mancuso [RSA San Giovanni di Dio, Patti (ME)], Eleonora Marelli [Istituto Geriatrico Camillo Golgi, Abbiategrasso (MI)], Alessandra Marengoni [Spedali Civili, Montichiari (BS)], Eleonora Marescalco (Azienda Ospedale Università di Padova), Benedetta Martin [Ospedale di Avezzano (AQ)], Valentina Massa [Casa Sollievo della Soferenza, S. Giovanni Rotondo (FG)], Giulia Matteucci (Azienda Ospedaliero-Universitaria di Ferrara), Irene Mattioli (Azienda Ospedaliero-Universitaria di Ferrara), Liliana Mazza (Policlinico Sant’Orsola-Malpighi, Bologna), Carmela Mazzoccoli (Ospedale Giovanni XXIII Policlinico di Bari), Fiammetta Monacelli (IRCCS Policlinico San Martino, Genova), Paolo Moneti (RSA Villa Gisella, Firenze), FM (Azienda Ospedaliero Universitaria Pisana; RSA Villa Isabella, Pisa), Federica Morellini (Azienda Ospedaliero-Universitaria di Modena), Maria Teresa Mormile (ASL Napoli 2 Nord), Enrico Mossello (Azienda Ospedaliero Universitaria Careggi, Firenze), Chiara Mussi (Azienda OspedalieroUniversitaria di Modena), Francesca Maria Nigro (USL Toscana Centro, Ospedale Santo Stefano, Prato), Marianna Noale (RSA AltaVita, Istituzioni Riunite di Assistenza, Padova), Chukwuma Okoye (Azienda Ospedaliero Universitaria Pisana), Giuseppe Orio (Policlinico Sant’Orsola-Malpighi, Bologna), Sara Osso [RSA La Quiete, Castiglione Cosentino (CS)], Chiara Padovan (AziendaOspedale Università di Padova), Annalisa Paglia (Azienda Ospedaliero Universitaria di Foggia), Giulia Pelagalli (Azienda Ospedaliero Universitaria Careggi, Firenze), Laura Pelizzoni (Policlinico Sant’Orsola-Malpighi, Bologna), Agostino Perri [RSA La Quiete, Castiglione Cosentino (CS)], Maria Perticone [Casa di Riposo Villa Marinella, Amantea (CS)], Giacomo Piccardo (IRCCS Policlinico San Martino, Genova), Alessandro Picci (Presidio Ospedaliero Universitario Santa Maria della Misericordia, Udine), Margherita Pippi [Ospedale San Giovanni Battista, Foligno (PG)], Giuseppe Provenzano (ASP Agrigento), Matteo Pruzzo (IRCCS Policlinico San Martino, Genova), Francesco Rafaele Addamo [RSA San Giovanni di Dio, Patti (ME)], Cecilia Rafaelli (Azienda Ospedale Università di Padova), Francesca Remelli (Azienda Ospedaliero-Universitaria di Ferrara), Onofrio Resta (Ospedale Giovanni XXIII Policlinico di Bari), Antonella Riccardi (Policlinico Sant’Orsola-Malpighi, Bologna), Daniela Rinaldi (Ospedale di Comunità (Camposampiero), Distretto Alta Padovana, ULSS 6 Euganea, Padova), Renzo Rozzini (Fondazione Poliambulanza, Brescia), Matteo Rubino (IRCCS Policlinico San Martino, Genova), Carlo Sabbà (Ospedale Giovanni XXIII Policlinico di Bari), Leonardo Sacco [Casa Sollievo della Soferenza, S. Giovanni Rotondo (FG)], Mariateresa Santoliquido [Casa Sollievo della Soferenza, S. Giovanni Rotondo (FG)], Mariella Savino [Casa Sollievo della Soferenza, S. Giovanni Rotondo (FG)], Francesco Scarso (Azienda Ospedaliero-Universitaria Sant’Andrea, Roma), Giuseppe Sergi (Azienda Ospedale Università di Padova), Gaetano Serviddio (Azienda Ospedaliero Universitaria di Foggia), Claudia Sgarito (ASP Agrigento), Giovanni Sgrò [RSA Istituto Santa Maria del Soccorso, Serrastretta (CZ); RSA San Vito Hospital, San Vito sullo Jonio (CZ); Casa Protetta Villa Mariolina, Montauro (CZ); Casa Protetta Villa Sant’Elia, Marcellinara (CZ)], Chiara Sidoli (Ospedale San Gerardo, Monza), Federica Sirianni [Casa di Riposo Villa Marinella, Amantea (CS)], Vincenzo Solfrizzi (Ospedale Giovanni XXIII Policlinico di Bari), Benedetta Soli (Azienda Ospedaliero-Universitaria di Modena), Debora Spaccaferro [RSA Estensiva, IHG, Guidonia (RM); RSA Intensiva, IHG, Guidonia (RM)], Fausto Spadea [RSA Casa Amica, Fossato Serralta (CZ)], Laura Spadoni [Ospedale San Giovanni Battista, Foligno (PG)], Laura Tafaro (Azienda Ospedaliero-Universitaria Sant’Andrea, Roma), Luca Tagliafco (IRCCS Policlinico San Martino, Genova), Andrea Tedde (Azienda Ospedaliero-Universitaria di Modena), Camilla Terziotti (Azienda Ospedale Università di Padova), Giuseppe Dario Testa (USL Toscana Centro, Ospedale San Jacopo, Pistoia), Maria Giulia Tinti [Casa Sollievo della Soferenza, S. Giovanni Rotondo (FG)], Francesco Tonarelli (USL Toscana Centro, Presidio Misericordia e Dolce, Prato), Elisabetta Tonon (USL Toscana Centro, Ospedale San Jacopo, Pistoia), CT (Ospedale di Comunità (Camposampiero), Distretto Alta Padovana, ULSS 6 Euganea, Padova; Azienda Ospedale Università di Padova), Rita Ursino [I RSA Geriatria, IHG, Guidonia (RM)], Filomena Vella (Azienda Sanitaria Universitaria Giuliano Isontina, Trieste), Maria Villanova (Azienda Ospedale Università di Padova), Aurora Vitali (Azienda Ospedaliero-Universitaria di Ferrara), SV (Azienda Ospedaliero-Universitaria di Ferrara), Francesca Zoccarato (Azienda Ospedale Università di Padova), Sonia Zotti (Policlinico Universitario Campus Bio-Medico, Roma), Amedeo Zurlo (Azienda OspedalieroUniversitaria di Ferrara).
Funding
The author(s) declare that financial support was received for the research, authorship, and/or publication of this article. This work was supported by the Italian Ministry of Health (Ricerca Corrente to IRCCS INRCA).
Acknowledgments
We thank Gilda Borselli for her precious support for the organization of the GeroCovid initiative.
Conflict of interest
The authors declare that the research was conducted in the absence of any commercial or financial relationships that could be construed as a potential conflict of interest.
Publisher’s note
All claims expressed in this article are solely those of the authors and do not necessarily represent those of their affiliated organizations, or those of the publisher, the editors and the reviewers. Any product that may be evaluated in this article, or claim that may be made by its manufacturer, is not guaranteed or endorsed by the publisher.
Abbreviations
WHO, World Health Organization; EU, European Union; NLR, neutrophil-to-lymphocyte ratio; COPD, chronic obstructive pulmonary disease; JADBIO, Just Add Data Bio; BBC-CV, Bootstrap Bias Corrected Cross Validation; CNS, central nervous system; ABG, arterial blood gas bicarbonate; AST, aspartate aminotransferase; dNLR, derived neutrophil-to-lymphocyte ratio; WBC, white blood cells; n.a, not applicable; ARDS, acute respiratory distress syndrome; NETs, neutrophil extracellular traps.
References
Alkhatip, A., Kamel, M. G., Hamza, M. K., Farag, E. M., Yassin, H. M., Elayashy, M., et al. (2021). The diagnostic and prognostic role of neutrophil-to-lymphocyte ratio in COVID-19: a systematic review and meta-analysis. Expert Rev. Mol. diagnostics 21 (5), 505–514. doi:10.1080/14737159.2021.1915773
Becerra-Muñoz, V. M., Núñez-Gil, I. J., Eid, C. M., García Aguado, M., Romero, R., Huang, J., et al. (2021). Clinical profile and predictors of in-hospital mortality among older patients hospitalised for COVID-19. Age Ageing 50 (2), 326–334. doi:10.1093/ageing/afaa258
Bruno, R. R., Wernly, B., Flaatten, H., Fjolner, J., Artigas, A., Baldia, P. H., et al. (2022). The association of the Activities of Daily Living and the outcome of old intensive care patients suffering from COVID-19. Ann. intensive care 12 (1), 26. doi:10.1186/s13613-022-00996-9
Cangiano, B., Fatti, L. M., Danesi, L., Gazzano, G., Croci, M., Vitale, G., et al. (2020). Mortality in an Italian nursing home during COVID-19 pandemic: correlation with gender, age, ADL, vitamin D supplementation, and limitations of the diagnostic tests. Aging 12 (24), 24522–24534. doi:10.18632/aging.202307
Casas-Rojo, J. M., Ventura, P. S., Anton Santos, J. M., de Latierro, A. O., Arevalo-Lorido, J. C., Mauri, M., et al. (2023). Improving prediction of COVID-19 mortality using machine learning in the Spanish SEMI-COVID-19 registry. Intern. Emerg. Med. 18 (6), 1711–1722. doi:10.1007/s11739-023-03338-0
Coin, A., Noale, M., Gareri, P., Trevisan, C., Bellio, A., Fini, F., et al. (2023). Clinical profile of trazodone users in a multisetting older population: data from the Italian GeroCovid Observational study. Eur. Geriatr. Med. 14 (3), 465–476. doi:10.1007/s41999-023-00790-1
Covino, M., De Matteis, G., Polla, D. A. D., Santoro, M., Burzo, M. L., Torelli, E., et al. (2021). Predictors of in-hospital mortality AND death RISK STRATIFICATION among COVID-19 PATIENTS aged ≥ 80 YEARs OLD. Arch. Gerontol. Geriatr. 95, 104383. doi:10.1016/j.archger.2021.104383
Di Rosa, M., Sabbatinelli, J., Soraci, L., Corsonello, A., Bonfigli, A. R., Cherubini, A., et al. (2023). Neutrophil-to-lymphocyte ratio (NLR) predicts mortality in hospitalized geriatric patients independent of the admission diagnosis: a multicenter prospective cohort study. J. Transl. Med. 21 (1), 835. doi:10.1186/s12967-023-04717-z
European centre for disease prevention and control. Available at: https://www.ecdc.europa.eu/en/publications-data/european-respiratory-virus-surveillance-summary-erviss. Accessed 12 February 2024.
Henkens, M., Raafs, A. G., Verdonschot, J. A. J., Linschoten, M., van Smeden, M., Wang, P., et al. (2022). Age is the main determinant of COVID-19 related in-hospital mortality with minimal impact of pre-existing comorbidities, a retrospective cohort study. BMC Geriatr. 22 (1), 184. doi:10.1186/s12877-021-02673-1
Huang, C., Wang, Y., Li, X., Ren, L., Zhao, J., Hu, Y., et al. (2020). Clinical features of patients infected with 2019 novel coronavirus in Wuhan, China. Lancet 395 (10223), 497–506. doi:10.1016/S0140-6736(20)30183-5
JADBio, Gerocovid_Training_mortality. (2024). Available at: https://app.jadbio.com/share/e401b262-755f-41fc-bb8e-35cc995d0163
Laosa, O., Pedraza, L., Alvarez-Bustos, A., Carnicero, J. A., Rodriguez-Artalejo, F., and Rodriguez-Manas, L. (2020). Rapid assessment at hospital admission of mortality risk from COVID-19: the role of functional status. J. Am. Med. Dir. Assoc. 21 (12), 1798–1802. doi:10.1016/j.jamda.2020.10.002
Li, L., Li, J., Gao, M., Fan, H., Wang, Y., Xu, X., et al. (2020). Interleukin-8 as a biomarker for disease prognosis of coronavirus disease-2019 patients. Front. Immunol. 11, 602395. doi:10.3389/fimmu.2020.602395
Liu, J., Liu, Y., Xiang, P., Pu, L., Xiong, H., Li, C., et al. (2020). Neutrophil-to-lymphocyte ratio predicts critical illness patients with 2019 coronavirus disease in the early stage. J. Transl. Med. 18 (1), 206. doi:10.1186/s12967-020-02374-0
Lozano, M., Iftimi, A., Briz-Redon, A., Peiró, J., Manyes, L., Otero, M., et al. (2022). Clinical characteristics of COVID-19 hospitalized patients associated with mortality: a cohort study in Spain. Infect. Med. (Beijing) 1 (2), 81–87. doi:10.1016/j.imj.2022.04.002
Ma, A., Zhang, L., Ye, X., Chen, J., Yu, J., Zhuang, L., et al. (2021). High levels of circulating IL-8 and soluble IL-2R are associated with prolonged illness in patients with severe COVID-19. Front. Immunol. 12, 626235. doi:10.3389/fimmu.2021.626235
Maestre-Muniz, M. M., Arias, A., and Lucendo, A. J. (2022). Predicting in-hospital mortality in severe COVID-19: a systematic review and external validation of clinical prediction rules. Biomedicines 10 (10), 2414. doi:10.3390/biomedicines10102414
Masso-Silva, J. A., Moshensky, A., Lam, M. T. Y., Odish, M. F., Patel, A., Xu, L., et al. (2022). Increased peripheral blood neutrophil activation phenotypes and neutrophil extracellular trap formation in critically ill coronavirus disease 2019 (COVID-19) patients: a case series and review of the literature. Clin. Infect. Dis. official Publ. Infect. Dis. Soc. Am. 74 (3), 479–489. doi:10.1093/cid/ciab437
McKenna, E., Wubben, R., Isaza-Correa, J. M., Melo, A. M., Mhaonaigh, A. U., Conlon, N., et al. (2022). Neutrophils in COVID-19: not innocent bystanders. Front. Immunol. 13, 864387. doi:10.3389/fimmu.2022.864387
Mendes, A., Serratrice, C., Herrmann, F. R., Genton, L., Périvier, S., Scheffler, M., et al. (2020). Predictors of in-hospital mortality in older patients with COVID-19: the COVIDAge study. J. Am. Med. Dir. Assoc. 21 (11), 1546–1554. doi:10.1016/j.jamda.2020.09.014
Ngiam, K. Y., and Khor, I. W. (2019). Big data and machine learning algorithms for health-care delivery. Lancet Oncol. 20 (5), e262–e273. doi:10.1016/S1470-2045(19)30149-4
Okoye, C., Calsolaro, V., Calabrese, A. M., Zotti, S., Fedecostante, M., Volpato, S., et al. (2022). Determinants of cause-specific mortality and loss of independence in older patients following hospitalization for COVID-19: the GeroCovid outcomes study. J. Clin. Med. 11 (19), 5578. doi:10.3390/jcm11195578
Olivieri, F., Sabbatinelli, J., Bonfigli, A. R., Sarzani, R., Giordano, P., Cherubini, A., et al. (2022). Routine laboratory parameters, including complete blood count, predict COVID-19 in-hospital mortality in geriatric patients. Mech. ageing Dev. 204, 111674. doi:10.1016/j.mad.2022.111674
Ramos-Rincon, J. M., Buonaiuto, V., Ricci, M., Martín-Carmona, J., Paredes-Ruíz, D., Calderón-Moreno, M., et al. (2021). Clinical characteristics and risk factors for mortality in very old patients hospitalized with COVID-19 in Spain. J. Gerontol. A Biol. Sci. Med. Sci. 76 (3), e28–e37. doi:10.1093/gerona/glaa243
Reusch, N., De Domenico, E., Bonaguro, L., Schulte-Schrepping, J., Bassler, K., Schultze, J. L., et al. (2021). Neutrophils in COVID-19. Front. Immunol. 12, 652470. doi:10.3389/fimmu.2021.652470
Riela, P. M. (2023). Artificial intelligence for COVID-19 mortality prediction: improvement of risk stratification and clinical decision-making. Intern. Emerg. Med. 18 (6), 1617–1618. doi:10.1007/s11739-023-03358-w
Rizza, S., Bellia, A., Perencin, A., Longo, S., Postorino, M., Ferrazza, G., et al. (2024). In-hospital and long-term all-cause mortality in 75 years and older hospitalized patients with and without COVID-19. J. Gerontology Geriatrics 72, 83–88. doi:10.36150/2499-6564-N715
Rodriguez-Sanchez, I., Redondo-Martin, M., Furones-Fernandez, L., Mendez-Hinojosa, M., Chen-Chim, A., Saavedra-Palacios, R., et al. (2021). Functional, clinical, and sociodemographic variables associated with risk of in-hospital mortality by COVID-19 in people over 80 Years old. J. Nutr. health and aging 25 (8), 964–970. doi:10.1007/s12603-021-1664-9
Santus, P., Radovanovic, D., Saderi, L., Marino, P., Cogliati, C., De Filippis, G., et al. (2020). Severity of respiratory failure at admission and in-hospital mortality in patients with COVID-19: a prospective observational multicentre study. BMJ open 10 (10), e043651. doi:10.1136/bmjopen-2020-043651
Seyit, M., Avci, E., Nar, R., Senol, H., Yilmaz, A., Ozen, M., et al. (2021). Neutrophil to lymphocyte ratio, lymphocyte to monocyte ratio and platelet to lymphocyte ratio to predict the severity of COVID-19. Am. J. Emerg. Med. 40, 110–114. doi:10.1016/j.ajem.2020.11.058
Trevisan, C., Del Signore, S., Fumagalli, S., Gareri, P., Malara, A., Mossello, E., et al. (2021). Assessing the impact of COVID-19 on the health of geriatric patients: the European GeroCovid Observational Study. Eur. J. Intern Med. 87, 29–35. doi:10.1016/j.ejim.2021.01.017
Tsagris, M., and Tsamardinos, I. (2018). Feature selection with the R package MXM. F1000Res 7, 1505. doi:10.12688/f1000research.16216.2
Tsamardinos, I., Charonyktakis, P., Papoutsoglou, G., Borboudakis, G., Lakiotaki, K., Zenklusen, J. C., et al. (2022). Just Add Data: automated predictive modeling for knowledge discovery and feature selection. NPJ Precis. Oncol. 6 (1), 38. doi:10.1038/s41698-022-00274-8
Vafadar Moradi, E., Teimouri, A., Rezaee, R., Morovatdar, N., Foroughian, M., Layegh, P., et al. (2021). Increased age, neutrophil-to-lymphocyte ratio (NLR) and white blood cells count are associated with higher COVID-19 mortality. Am. J. Emerg. Med. 40, 11–14. doi:10.1016/j.ajem.2020.12.003
van Buuren, S., and Groothuis-Oudshoorn, K. (2011). Mice: multivariate imputation by chained equations in R. J. Stat. Softw. 45 (3), 1–67. doi:10.18637/jss.v045.i03
Wan, S., Xiang, Y., Fang, W., Zheng, Y., Li, B., Hu, Y., et al. (2020). Clinical features and treatment of COVID-19 patients in northeast Chongqing. J. Med. virology 92 (7), 797–806. doi:10.1002/jmv.25783
Wang, D., Hu, B., Hu, C., Zhu, F., Liu, X., Zhang, J., et al. (2020). Clinical characteristics of 138 hospitalized patients with 2019 novel coronavirus-infected pneumonia in wuhan, China. Jama 323 (11), 1061–1069. doi:10.1001/jama.2020.1585
Wang, E., Liu, A., Wang, Z., Shang, X., Zhang, L., Jin, Y., et al. (2022). The prognostic value of the Barthel Index for mortality in patients with COVID-19: a cross-sectional study. Front. public health 10, 978237. doi:10.3389/fpubh.2022.978237
Wendland, P., Schmitt, V., Zimmermann, J., Häger, L., Göpel, S., Schenkel-Häger, C., et al. (2023). Machine learning models for predicting severe COVID-19 outcomes in hospitals. Inf. Med. Unlocked 37, 101188. doi:10.1016/j.imu.2023.101188
Wu, C., Chen, X., Cai, Y., Xia, J., Zhou, X., Xu, S., et al. (2020). Risk factors associated with acute respiratory distress syndrome and death in patients with coronavirus disease 2019 pneumonia in wuhan, China. JAMA Intern. Med. 180 (7), 934–943. doi:10.1001/jamainternmed.2020.0994
Wynants, L., Van Calster, B., Collins, G. S., Riley, R. D., Heinze, G., Schuit, E., et al. (2020). Prediction models for diagnosis and prognosis of covid-19 infection: systematic review and critical appraisal. Bmj 369, m1328. doi:10.1136/bmj.m1328
Keywords: COVID-19, mobility, neutrophil-to-limphocyte ratio, in-hospital mortality, artificial intelligence
Citation: Fedecostante M, Sabbatinelli J, Dell’Aquila G, Salvi F, Bonfigli AR, Volpato S, Trevisan C, Fumagalli S, Monzani F, Antonelli Incalzi R, Olivieri F and Cherubini A (2024) Prediction of COVID-19 in-hospital mortality in older patients using artificial intelligence: a multicenter study. Front. Aging 5:1473632. doi: 10.3389/fragi.2024.1473632
Received: 31 July 2024; Accepted: 02 October 2024;
Published: 17 October 2024.
Edited by:
M. Victoria Delpino, CONICET Instituto de Investigaciones Biomédicas en Retrovirus y SIDA (INBIRS), ArgentinaReviewed by:
Maik Kschischo, University of Koblenz, GermanyDanay Saavedra, Center of Molecular Immunology, Cuba
Melsew Setegn Alie, Mizan-Tepi University, Ethiopia
Copyright © 2024 Fedecostante, Sabbatinelli, Dell’Aquila, Salvi, Bonfigli, Volpato, Trevisan, Fumagalli, Monzani, Antonelli Incalzi, Olivieri and Cherubini. This is an open-access article distributed under the terms of the Creative Commons Attribution License (CC BY). The use, distribution or reproduction in other forums is permitted, provided the original author(s) and the copyright owner(s) are credited and that the original publication in this journal is cited, in accordance with accepted academic practice. No use, distribution or reproduction is permitted which does not comply with these terms.
*Correspondence: Antonio Cherubini, a.cherubini@inrca.it
†These authors have contributed equally to this work and share first authorship