- 1New Industry Creation Hatchery Center, Tohoku University, Sendai, Japan
- 2Department of Advanced Biomedical Research, Faculty of Medicine, University of Yamanashi, Yamanashi, Japan
- 3Department of Otorhinolaryngology, Head and Neck Surgery, Faculty of Medicine, University of Yamanashi, Yamanashi, Japan
- 4Laboratory of Food and Nutritional Sciences, Department of Local Produce and Food Sciences, Faculty of Life and Environmental Sciences, University of Yamanashi, Yamanashi, Japan
- 5Department of Orthopaedic Surgery, Faculty of Medicine, University of Yamanashi, Yamanashi, Japan
- 6Laboratory of Embryology and Genomics, Faculty of Life and Environmental Sciences, University of Yamanashi, Yamanashi, Japan
- 7Faculty of Engineering, University of Yamanashi, Yamanashi, Japan
- 8Department of Respiratory Medicine, Faculty of Medicine, University of Yamanashi, Yamanashi, Japan
Obesity and ageing are the most important risk factors for sleep apnoea syndrome (SAS); however, the role of body mass index (BMI) on sleep status in healthy older adults is unclear. To explore sleep parameters according to BMI among active older adults, we cross-sectionally examined the relationship between sleep-related parameters and BMI in 32 Japanese adults aged from 83 to 95 years without long-term care who were unaware of having SAS. Correlation and linear regression analyses were performed. Moderate or severe SAS prevalence was high in both those with low (68.8%) and high (68.8%) BMI. A higher increase in apnoea-hypopnoea index (AHI) was negatively correlated with sleep depth in the high-BMI group. In the low-BMI group, the number of awakenings and age were positively correlated with AHI. Older adults may have SAS regardless of their BMI, and the sleep status of patients with SAS may vary by BMI.
1 Introduction
Sleep apnoea syndrome (SAS), defined as moderate or severe SAS (Apnonea-hypopnea index [AHI] ≥15/h) affects approximately 2%–50% of the middle-aged adult population in the U.S. and Australia (Young et al., 1993; Baldwin et al., 2001; Banno and Kryger, 2007; Peppard et al., 2013; Heinzer et al., 2016; McArdle et al., 2022), and the prevalence among patients with stroke exceeds 60% (Mohsenin and Valor, 1995; Bassetti and Aldrich, 1999; Parra et al., 2000). Well-documented risk factors for SAS include ageing (Young et al., 2002; Jordan et al., 2014; Matsumoto et al., 2020), obesity (Phillips et al., 1999; Newman et al., 2005; Yaggi et al., 2005; Hudgel et al., 2012; Lam et al., 2012; Fan et al., 2019), snoring (Martínez-García et al., 2012; Heinzer et al., 2015), high cholesterol levels (Nadeem et al., 2014), high red blood cell counts (Feliciano et al., 2017), smoking (Yaggi et al., 2005; Martínez-García et al., 2012; Heinzer et al., 2015), and male sex (Martínez-García et al., 2012). Of these, old age and obesity are major factors with increasing global prevalence, making SAS an important future concern. A weight gain of ≥10 kg is associated with a 5-fold risk of moderate or severe SAS in men and a 2.5-fold risk of moderate or severe SAS in women (Newman et al., 2005). We recently examined the prevalence of SAS in 32 relatively healthy older adults (average age, 87 years) in Yamanashi Prefecture who were not certified for long-term care, wherein 68.8% were found to have moderate or severe SAS (AHI ≥15/h), all of whom were unaware that they had SAS (Tanaka et al., 2022), suggesting a lack of awareness of this condition among older adults.
Although many studies have addressed SAS, particularly in populations aged <60 years, it remains unclear how the combination of ageing and obesity affects the prevalence and severity of SAS in older age groups. An analysis of 30 participants in a longitudinal study in San Francisco (baseline age, 57.7 years; mean age at last follow-up, 82.0 years) reported that sleep apnoea worsens with age, regardless of weight gain or loss (Bliwise et al., 2010). Additionally, a study conducted in Nagahama, Japan (Matsumoto et al., 2020) showed that the incidence of moderate or severe SAS exceeded 10% in adults aged >70 years with low body mass index (BMI). In our small multifaceted exploratory study of Japanese adults aged >80 years, muscle weakness and poor exercise habits were the main factors associated with SAS severity, with BMI not identified as a significant SAS-related factor (Tanaka et al., 2022). These findings suggest that some older adults develop SAS, although they have a low BMI. SAS-related factors in older adults with low BMI have not yet been investigated, and it is unclear whether differences in characteristics and pathophysiology exist between older adults with high and low BMI. To the best of our knowledge, no studies have investigated how the combination of ageing and BMI affects sleep quality.
This study aimed to determine how BMI affects sleep in older adults. Specifically, we aimed to explore sleep parameters (sleep depth, body position, number of awakenings during sleep, and AHI) according to BMI status among a cohort of adults aged >80 years (Tanaka et al., 2022) who were relatively healthy and did not require long-term care. These will contribute to the inference of risk factors and mechanisms of SAS in older adults and will serve as a catalyst for more detailed validation studies and biomarker searches to communicate risk to older adults.
2 Materials and methods
2.1 Study design, setting, and participants
In 2019, we invited 104 people from the Yamanashi Healthy-Active Life Expectancy study cohort (Kondo et al., 2008) to participate in the Yamanashi Healthy active long-living older people Biobank for healthy ageing biosciences and an intermediate questionnaire survey (Tanaka et al., 2022). We included participants who 1) did not require long-term care, 2) provided informed consent for the survey and SAS measurements, and 3) did not give the researchers the impression that they had difficulty participating in the study (e.g., a participant with poor understanding of the sleep measurement kit despite being told how to use it or who could not wear the sensor). All measurements, including the sleep apnoea test, were performed between January and December 2020. We excluded those for whom the SAS test could not be adequately performed (e.g., where measurement errors occurred).
2.2 Ethical considerations
This study was approved by the Ethics Committee of University of Yamanashi School of Medicine (approval number: 2096; approval date: December 2019). The study complied with the Declaration of Helsinki, the Japanese Ethical Guidelines for Medical Research Involving Human Subjects, Act on the Protection of Personal Information, and Ethical Guidelines for Human Genome and Genetic Analysis Research. We obtained written informed consent from the participants after explaining the study verbally and in writing.
2.3 Measurements
2.3.1 Physical measurements
Participants’ weight multi-frequency segmental body composition analyzer (Tanita MC-780A-N; Tanita Corp., Tokyo, Japan) (Yamada et al., 2017). Weight was measured by subtracting the weight of clothing (1 kg in January and February to account for heavier winter clothing and 0.5 kg from March to December). Height was measured using a stadiometer (Height Measurement HM 200P; Charder Electronic Co., Ltd., Taichung City, Taiwan). Systolic blood pressure, diastolic blood pressure, and pulse rate were measured using a sphygmomanometer (Terumo ES-W300ZZ; Terumo Corp., Tokyo, Japan).
2.3.2 Lifestyle and medical history questionnaire
Each participant completed a questionnaire on lifestyle habits and medical history, which included questions about alcohol consumption, smoking, and history of SAS.
2.3.3 Sleep apnoea test
The AHI was measured using a portable monitoring device (WatchPAT 200; Itamar Medical, Caesarea, Israel) as recommended in the American Academy of Sleep Medicine guidelines for obstructive SAS (Kapur et al., 2017). The gold-standard polysomnography (PSG) is a high-burden test for participants unaware of SAS. Because of the older age of the participants, we also avoided using a device that required the wearing of a unit to measure airflow through the nose and decided to use the WatchPAT 200, which is certified as a medical device as a simple testing device. Peripheral arterial tone signal measures the arterial pulsatile volume changes of the finger that are regulated by α-adrenergic innervation of the smooth muscles of the vasculature of the finger; thus, it reflects sympathetic nervous system activity. This augmentation in sympathetic activity accompanies increased heart rate and desaturation at the termination of respiratory events. The WatchPAT recorder is based predominantly on recordings of peripheral arterial tone signals and pulse rate (two important outputs of the autonomic nervous system). The WatchPAT system allows us to determine pulse rate, oxygen saturation, actigraphy data, and the 4% oxygen desaturation index and the respiratory event index, rapid eye movement (REM), light sleep, and deep sleep stages in the form of AHI, REM%, light sleep%, and deep sleep%, respectively (Nittman et al., 2004; Kapur et al., 2017). Furthermore, the WatchPAT system also automatically calculates percentage of time spent in the prone, supine, left side-lying, and right side-lying sleeping positions; the AHI for each sleep depth (i.e., the REM-AHI and NREM-AHI) as well as for each body position (prone, supine, left side-lying, and right side-lying); and number of awakenings during sleep.
2.4 Statistics and reproducibility
First, the basic statistics regarding all parameters analysed in this study were performed including all participants and high- and low-BMI groups divided by the median BMI of all participants. A pooled t-test was performed to compare age and all sleep-related parameters obtained by the WatchPAT system in two groups: high-BMI and low-BMI; P-values were calculated. Second, a linear regression model was created to evaluate whether BMI could be used to predict each of all AHI parameters as continuous variables. Using the linear regression analysis, non-standardized estimation values β), P-values, and R2 were calculated to estimate the effect of each explanatory variable. Third, in each of the high/low-BMI groups and high/low-BMI groups with AHI ≥15/h, we performed multivariate correlation analysis of age and all sleep-related parameters considered in this study; we calculated the Spearman correlation coefficient ρ) and P-value. Finally, the parameters that showed interesting relationships using the multivariate correlation analysis were extracted and reevaluated by linear regression analysis. P-values < 0.05 were considered statistically significant. These statistical analyses were performed using JMP® Pro 17.0 (SAS Institute Inc., Cary, NC) software.
3 Results
3.1 Participants
In total, 32 (30.8%) of 104 participants met the YHAB study criteria, none of whom had been diagnosed with SAS or received continuous positive airway pressure treatment prior to the YHAB study. Of the 32 participants, 56% were male. Table 1 shows the participant characteristics, including the sleep and AHI data used in the analysis. The mean values for age and BMI were 86.7 years and 23.5 kg/m2, respectively. The mean percentage of rapid eye movement (REM%), light sleep (light sleep%), and deep sleep (deep sleep%) were 21.2%, 64.8%, and 14.0%, respectively. The AHI during REM sleep (REM-AHI; 28.9/h) value was higher than that during non-REM sleep (NREM-AHI; 22.1/h), and the mean AHI value in the supine position (29.6/h) was highest among all four positions.
3.2 Estimation of AHI characteristics using a linear regression model with BMI as the dependent variable
Table 2 shows the AHI characteristics in terms of sleep stage and body position estimated using a linear regression model with BMI as the dependent variable. All estimated values β) were positive. The P values of the REM-AHI (0.017) and AHI in right side lying (0.046) were significant at <0.05 and N > 10.
3.3 Basic comparison between high- and low-BMI groups
We calculated and compared the basic statistics for each parameter between the high- and low-BMI groups divided by the median BMI (23.75 kg/m2) (Figure 1A). The male ratio of both groups was the same (0.563). Eleven (68.8%) participants each in the high- and low-BMI groups had moderate or severe sleep apnoea (Figures 1B, C). Figure 2 shows the sleep stages of three representative participants: one without SAS (Figure 2A), one with a high BMI (Figure 2A), and one with a low BMI (Figure 2C). Focusing on the differences in sleep status (percentages of sleep stages, number of awakenings, and AHI) between the high- and low-BMI groups shown in Figure 2, the statistical differences in sleep parameters between groups were examined in detail. Among all the parameters investigated, only the REM-AHI showed a significant difference between the high- and low-BMI groups, both among all participants (35.1/h vs. 21.9/h, respectively, p = 0.005; Table 3) and among those with an AHI ≥15/h (40.8/h vs. 27.7/h, respectively, p = 0.018; Table 4). The male ratios of the compared parameters in the low- and high-BMI groups were 0.38–0.5 and 0.58–0.79, respectively, for all participants (Table 3), and 0.33–0.44 and 0.56–0.80, respectively, for participants with an AHI ≥15/h (Table 4).
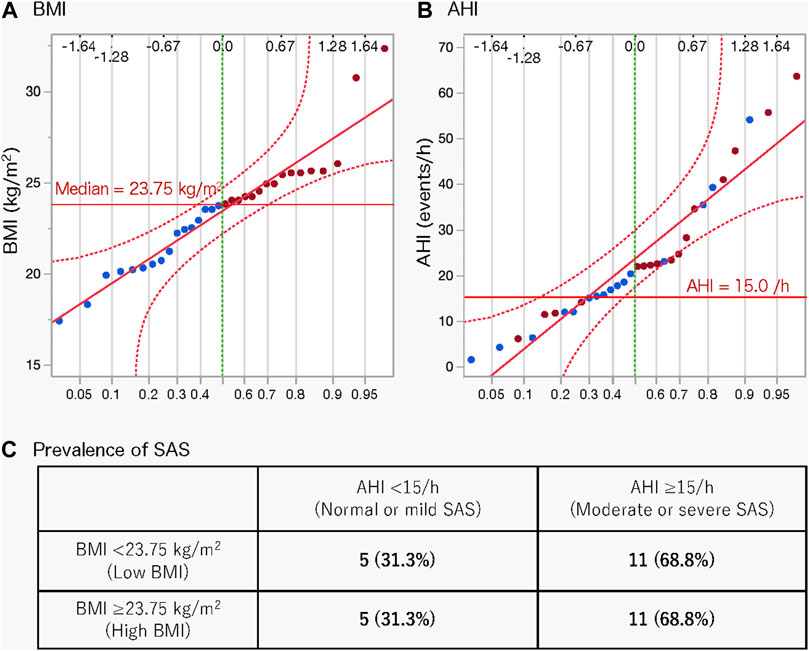
Figure 1. Normal quantile plots of BMI (A) and AHI (B) and prevalence of SAS according to BMI (C). Red circles indicate a BMI greater than the median value; blue circles indicate a BMI less than the median value. AHI, apnoea-hypopnea index; BMI, body mass index; SAS, sleep apnoea syndrome.
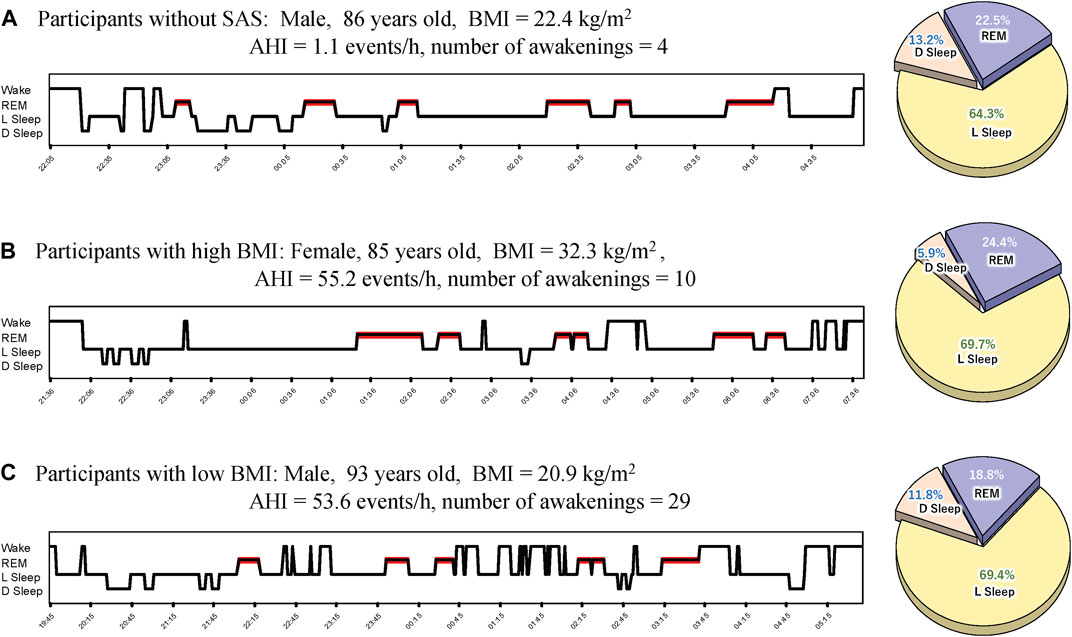
Figure 2. Representative sleep profiles. (A) Profile of a participant without SAS (with the lowest AHI), (B) a participant with SAS in the high-BMI group, and (C) a participant with SAS in the low-BMI group. AHI, apnoea-hypopnea index; BMI, body mass index; D Sleep, deep sleep; L Sleep, light sleep; REM, rapid eye movement; SAS, sleep apnoea syndrome.
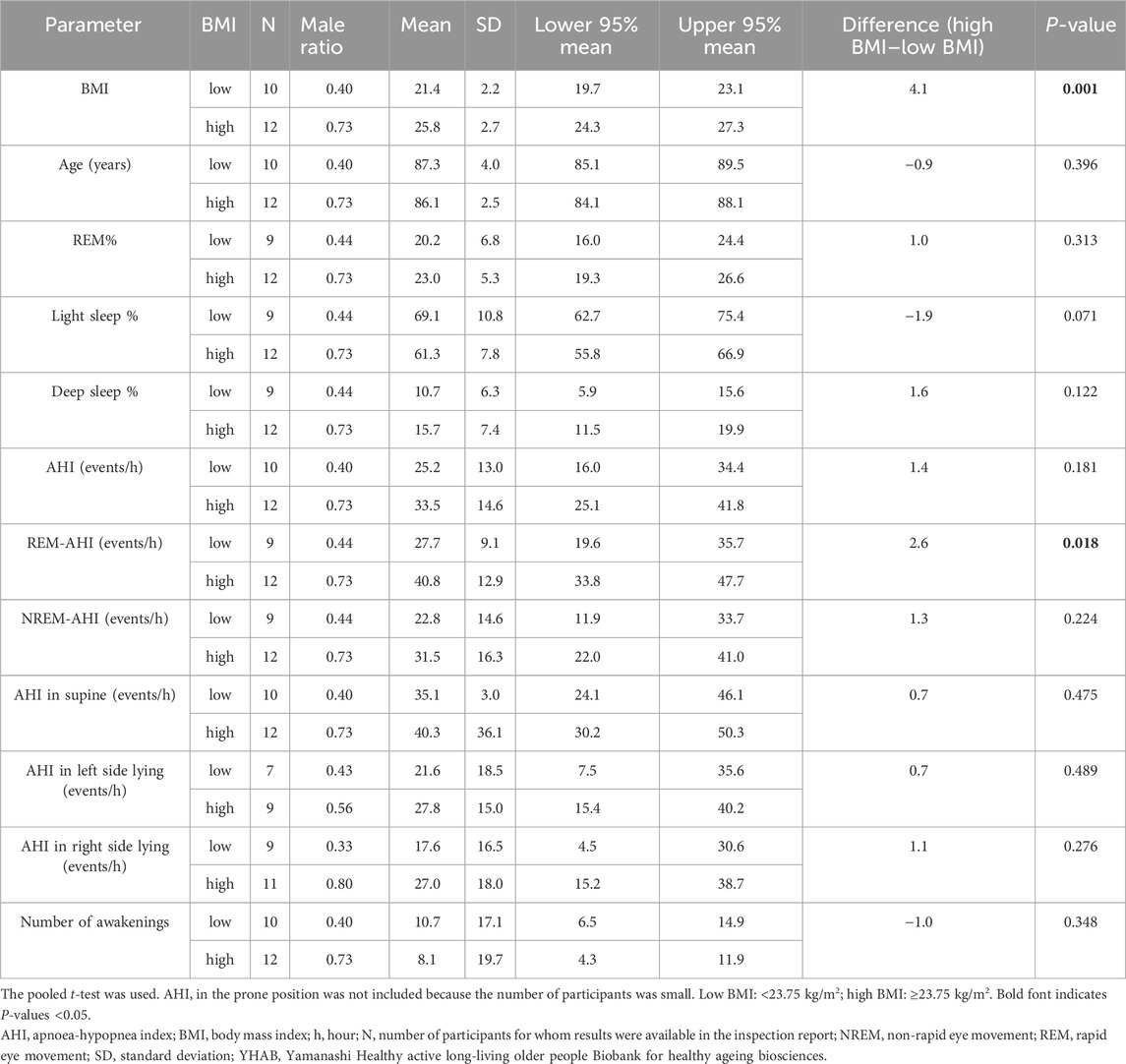
Table 4. Comparisons between the participants in the high- and low-BMI groups with an AHI ≥15/h in the YHAB dataset.
3.4 Effects of age and sleep parameters at different sleep stages in the high- and low-BMI groups
Multivariate correlation analysis (Figure 3; Table 5) findings differed between all the participants in the high-BMI group (Figure 3A), all the participants in the low-BMI group (Figure 3B), participants with an AHI ≥15/h with a high BMI (Figure 3C), and participants with an AHI ≥15/h with a low BMI (Figure 3D) as follows. All participants and participants with an AHI ≥15/h in the high-BMI group showed a significant negative correlation between deep sleep% and AHI (ρ = 0.503 and p = 0.047 for all participants and ρ = −0.748 and p = 0.005 for patients with an AHI ≥15/h); however, this was not observed in participants in the low-BMI group (ρ = −0.218 and p = 0.435 for all participants and ρ = −0.250 and p = 0.517 for patients with an AHI ≥15/h). All participants in the low-BMI group demonstrated a significant positive correlation between the number of awakenings and age (ρ = 0.651 and p = 0.006); however, this was not observed in participants in the low-BMI group with an AHI ≥15/h (ρ = 0.616 and p = 0.058) or participants in the high-BMI group (ρ = −0.420 and p = 0.105 for all participants and ρ = −0.332 and p = 0.292 for patients with an AHI ≥15/h). Participants in the low-BMI group with an AHI ≥15/h showed a significant positive correlation between the number of awakenings and AHI (ρ = 0.675 and p = 0.032); however, this was not observed in all participants in the low-BMI group (ρ = 0.374 and p = 0.155) or those in the high-BMI group (ρ = 0.188 and p = 0.485 for all participants and ρ = 0.288 and p = 0.364 for patients with an AHI ≥15/h). Although all participants in the low-BMI group presented a significant negative correlation between the number of awakenings and REM% (ρ = −0.628 and p = 0.012), this was not observed in participants in the low-BMI group with an AHI ≥15/h (ρ = −0.385 and p = 0.306) or participants in the high-BMI group (ρ = 0.164 and p = 0.543 for all participants and ρ = 0.205 and p = 0.744 for patients with an AHI ≥15/h). All participants in the low-BMI group demonstrated a significant negative correlation between light sleep% and REM% (ρ = −0.796 and p < 0.001 for all participants and ρ = −0.783 and p = 0.013 for those with an AHI ≥15/h); however, patients in the high-BMI group showed a smaller negative correlation between light sleep% and REM% than that shown by those in the low-BMI group, with statistical significance for all participants (ρ = −0.535 and p = 0.033), and without statistical significance for those with an AHI ≥15/h (ρ = −0.322 and p = 0.308). A property common to all groups was the predominant negative correlation between deep sleep% and light sleep%.
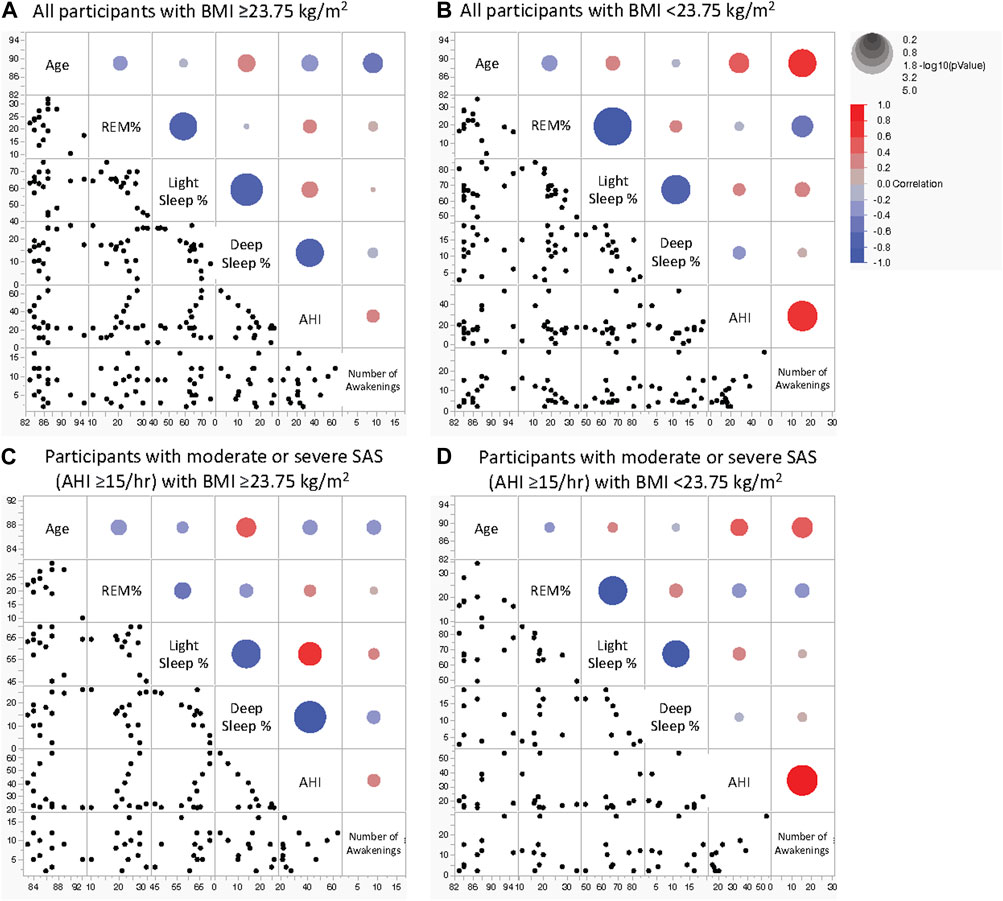
Figure 3. Scatterplot matrix for indicators of age and sleep parameters in older adults. Sleep stage percentage, AHI, and the number of awakenings in all participants with BMI ≥23.75 kg/m2 (A), all participants with BMI <23.75 kg/m2 (B), participants with moderate or severe SAS with BMI ≥23.75 kg/m2 (C), and participants with moderate or severe SAS with BMI <23.75 kg/m2 (D). The colour of the circles in the scatterplots indicates the correlation coefficient, and their size indicates the non-parametric density. Blue and red represent negative and positive correlation coefficients, respectively. The larger the circle, the smaller the P-value. AHI, apnoea-hypopnea index; BMI, body mass index; REM, rapid eye movement; NREM, non-rapid eye movement; SAS, sleep apnoea syndrome.
3.5 Estimation of age, deep sleep%, and number of awakenings using BMI
The high- and low-BMI groups were reanalysed using a linear regression model with respect to age (Table 6), deep sleep% (Table 7), and number of awakenings (Table 8), as the multivariate correlation analysis (Figure 3; Table 5) suggested that the relationships of these variables with AHI may differ between groups. As shown in the age estimation results (Table 6), β was >0 in the REM-AHI and AHI in the right side-lying position in the low-BMI group and showed statistical significance (p < 0.05; Table 6). Regarding deep sleep% analysis (Table 7), β was <0 in the AHI, REM-AHI, NREM-AHI, and AHI in the supine and right side-lying positions, with statistical significance (p < 0.05) in the high-BMI group. In the analysis of the number of awakenings (Table 8), β was >0 in all variables in the low-BMI group, with statistical significance (p < 0.05).
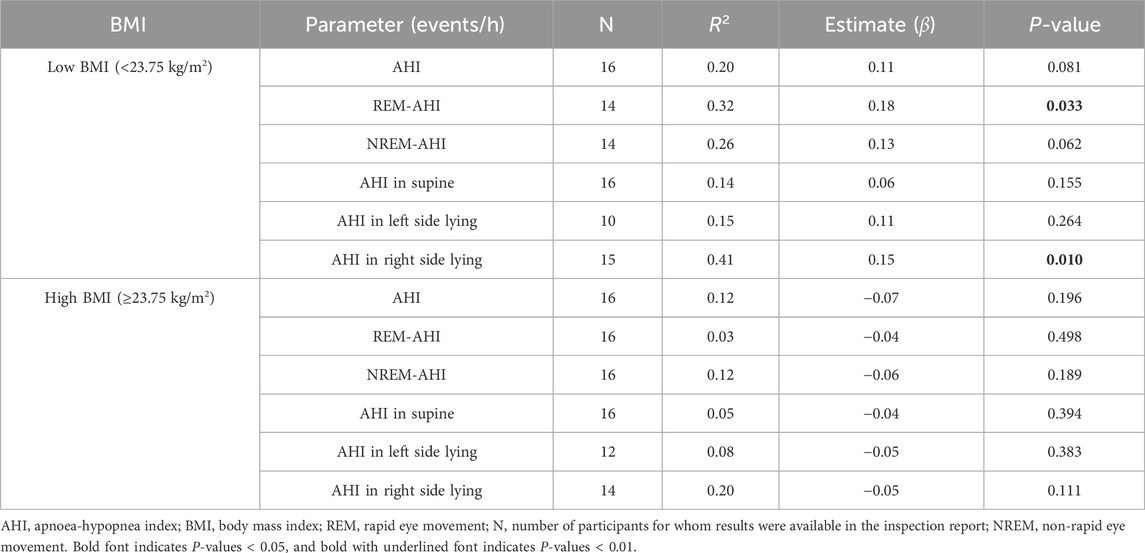
Table 6. Estimated effect of age on AHI characteristics in the high- and low-BMI groups using the linear regression model.
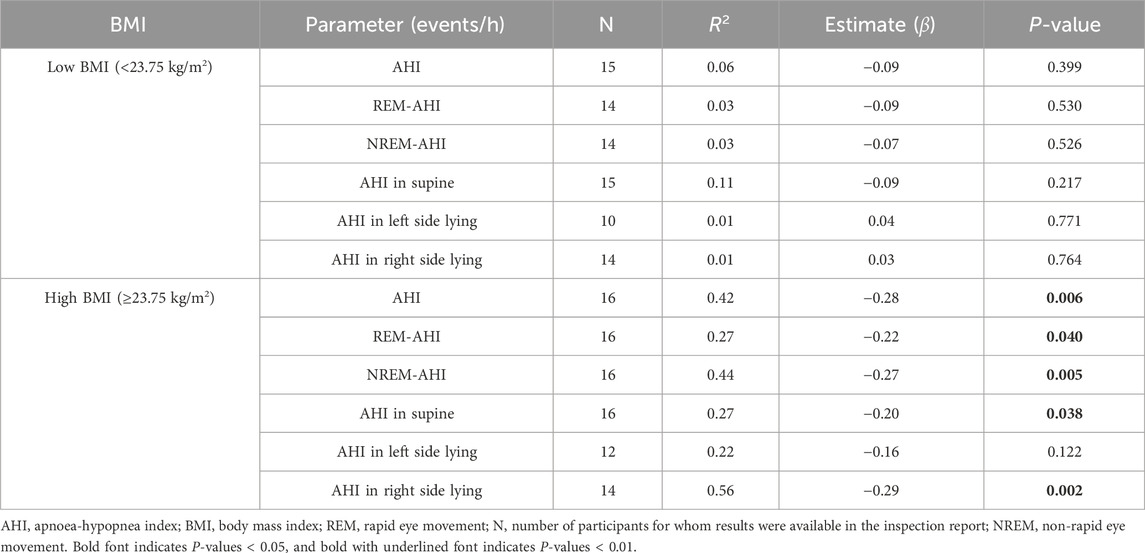
Table 7. Estimated effect of deep sleep% on AHI characteristics in the high- and low-BMI groups using the linear regression model.
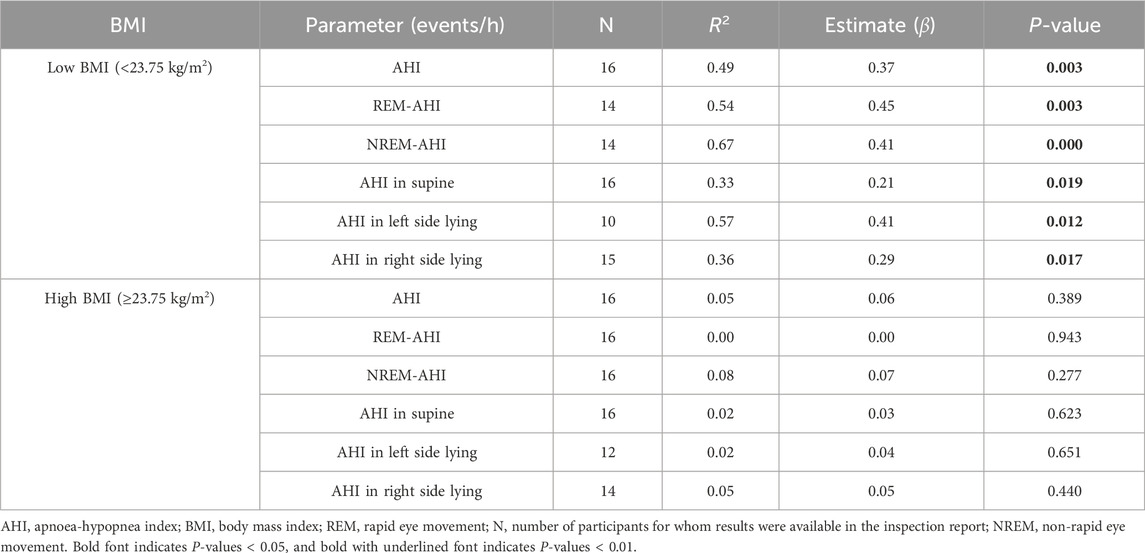
Table 8. Estimated effect of the number of awakenings during sleep on AHI characteristics in the high- and low-BMI groups using the linear regression model.
4 Discussion
This study examined the relationships among BMI, sleep parameters (including AHI, sleep stages, and number of awakenings), and age in older adults who were in relatively good health and had no previous diagnosis of SAS. The prevalence of moderate or severe SAS was the same in the low- and high-BMI groups (both 68.8%). In all examined sleep parameters, AHI tended to increase with increasing BMI, with a significant increase during REM sleep. This study found the following differences between the high- and low-BMI groups: 1) in the high-BMI group, deep sleep% tended to decrease as AHI increased, with no significant correlation between the number of awakenings and AHI and 2) in the low-BMI group, there was a tendency for the number of awakenings to increase with age and AHI, although no significant correlation existed between deep sleep% and AHI.
We found that the prevalence of SAS (68.8%) in the low-BMI group was as high as that in the high-BMI group. This result is generally consistent with a small long-term longitudinal study conducted in San Francisco (Bliwise et al., 2010) and a large cross-sectional study conducted in Nagahama (Matsumoto et al., 2020). We also examined the sleep conditions in detail to determine the influence of BMI on SAS. Overall, the AHI tended to increase with increasing BMI in all states of sleep, with REM sleep and sleeping in the supine position showing the highest correlation with BMI; these findings are consistent with those of other studies (Oksenberg et al., 2010; Koo et al., 2018; Park et al., 2022). In addition, the REM-AHI was found to be the most strongly positively correlated with BMI, which was similar to findings reported in other studies (Acosta-Castro et al., 2018; Falla et al., 2023). In contrast, the current study showed that patients with SAS who have high BMI tend to have shallow sleep without awakenings and that the sleep of patients with SAS who have low BMI tends to be fragmented with awakenings, although deep sleep is achieved. Our findings regarding differences in sleep status between older adults with high and low BMI may significantly contribute to the literature, as we found no previous studies reporting these findings. However, our sample was as small as that of the San Francisco study; the fact that several of our findings are consistent with those of similar previous studies supports the validity of our findings regarding differences in sleep status.
Generally, increases in AHI have been attributed to airway narrowing due to excessive fat deposition (Peppard et al., 2013; White and Bradley, 2013; Pépin et al., 2016); however, this explanation is not applicable to patients with low BMI who have SAS. Other factors, such as tongue-based airway obstruction (Vanderveken et al., 2013; Strollo et al., 2014) and central nerve abnormalities (Oldenburg et al., 2007; Oldenburg et al., 2009; Cowie et al., 2015), are associated with SAS. Bliwise et al. (2010) noted in their discussion that the increase in AHI with ageing and weight loss could be due to muscle mass loss, but no muscle mass measurements or muscle-related motor function were reported. Our previous study, which included the same 32 participants as those in this study, showed that decline in motor function correlated with increased AHI (Tanaka et al., 2022) and worsening of SAS severity correlated with age in the low-BMI group. Loss of weight, muscle mass, and motor function with ageing is part of the typical pathophysiology of frailty (Bliwise et al., 2010; Clegg et al., 2013). The prevalence of SAS is higher in participants who are frail than in those who are not (Ensrud et al., 2009; Ensrud et al., 2012; Piovezan et al., 2013). Patients who are frail have also been reported to have an increased number and duration of awakenings than that observed in healthy older adults, consistent with the increased number of awakenings observed in the low-BMI group in this study (Ensrud et al., 2009). It has been well-documented that the number of awakenings during sleep increases with age (Knowles and MacLean, 1990; Edwards et al., 2010). Similarly, this study included participants older than 80 years, and only a few of them reported having a good sleep status, indicated by the number of awakenings and sleep depth. These findings suggest that patients with SAS who have low BMI analysed in this study may be frail due to physical ageing, including the loss of strength in respiratory muscles.
In our study, when restricted to older adults with high BMI, AHI did not increase with ageing but rather decreased, although this finding was not statistically significant. This is in direct opposition to the finding for patients with SAS with low BMI, and to the best of our knowledge, this has not been reported by any study focused on the difference in sleep status according to BMI. The older adults with high BMI in our study might have been those with good health and maintained muscle mass. The SAS patients with high BMI in this study might have been older adults who have successfully maintained their health by preventing physical ageing, including loss of respiratory muscle strength.
Another important study finding was the lack of awareness of SAS among the study participants. SAS has been shown to increase not only mortality but also drowsiness, which can lead to decreased alertness and traffic accidents (Findley et al., 1989; Philip et al., 2008). Therefore, increasing the awareness of SAS is essential for early detection and treatment (Aurora et al., 2012; Martínez-García et al., 2012; Jennum et al., 2015; Dodds et al., 2020). Our study highlights the importance of considering SAS equally in older adults with low BMI as well as in those with high BMI. Since patients with SAS with low BMI are particularly likely to have associated frailty, it is necessary to consider snoring and other symptoms associated with SAS as indicators of frailty.
This study had some limitations. First, the study population was limited to the dataset obtained from the Yamanashi Healthy active long-living older people Biobank for healthy ageing biosciences survey, which was smaller than that in other SAS studies (Young et al., 2002; Jordan et al., 2014; Acosta-Castro et al., 2018; Matsumoto et al., 2020). However, we found that the basic characteristics of the study population, such as sleep stage and the AHI for each body position, were similar to those reported in larger studies, which partially confirms the validity of our results. Nonetheless, similar analyses with larger cohorts are required to further confirm the generalisability of our findings. Second, the participants in this study were limited to those from Yamanashi Prefecture; thus, it is necessary to verify whether the same results can be observed in other regions or countries. Third, standardising the use of common clothing throughout the year when measuring weights was impossible. This was because the study was conducted during the time of COVID-19 infection outbreak and ventilation and other measures were taken, particularly during the winter months. Therefore, asking older adults to change into uniform clothing was impossible. Although we asked them to undress as lightly as possible, a slight concern remains regarding the accuracy of BMI compared with physical examination. Lastly, WatchPAT, which was used in this study, is not equipped with a function to perform PSG; therefore, not only can it not detect central nervous system abnormalities that are thought to be associated with SAS but also the evaluation of REM and NREM sleep is an estimated evaluation not based on PSG. The use of PSG was avoided in this study in consideration of the burden on the older adults. We hope that the exploratory findings obtained with the less burdensome WatchPAT used in this study will be followed up with detailed validation studies using PSG and other instruments.
Data availability statement
The original contributions presented in the study are included in the article/Supplementary Material, further inquiries can be directed to the corresponding author.
Ethics statement
The studies involving humans were approved by ethics committee of yamanashi university. The studies were conducted in accordance with the local legislation and institutional requirements. The participants provided their written informed consent to participate in this study. Written informed consent was obtained from the individual(s) for the publication of any potentially identifiable images or data included in this article.
Author contributions
YT: Conceptualization, Data curation, Formal Analysis, Funding acquisition, Investigation, Methodology, Project administration, Resources, Supervision, Validation, Visualization, Writing–original draft, Writing–review and editing. NB-M: Writing–review and editing. TY: Writing–review and editing. KM: Writing–original draft, Writing–review and editing, Methodology, Supervision. SI: Data curation, Investigation, Writing–review and editing. TA: Investigation, Writing–review and editing. TK: Writing–review and editing. YI: Writing–review and editing. KS: Supervision, Writing–review and editing. DS: Supervision, Writing–review and editing.
Funding
The author(s) declare financial support was received for the research, authorship, and/or publication of this article. This study was supported by the Japan Society for the Promotion of Science KAKENHI Grant-in-Aid for Scientific Research B) [grant number: 21H03351] and the Interdisciplinary Research Project at the University of Yamanashi. The study’s sponsor and the University of Yamanashi had the opportunity to review the entire project, including this paper. Personnel expenses for the first author were supported by the Tokyo Metropolitan Government’s Integrated Research Promotion Project for Living with Dementia.
Acknowledgments
The authors thank all participants and their families for participating in this study. The authors also wish to thank Yusuke Iwata, TA, Kozo Saito, Daisuke Ando, Masaki Omata, Tomokazu Matsuoka, Kyoichiro Tsuchiya, Katsue Suzuki-Inoue, Koichiro Ueki, Hirotaka Haro, Shuichi Koizumi, Kenji Kashiwagi, Zentaro Yamagata, and Masaru Iwasaki from the YHAB Health Data Survey Group 2020; SI and Atsuhito Nakao from the YHAB Sleep Health Group; and Kunio Miyake, Yoichi Shinozaki, and Hiroshi Kurosawa from the YHAB BioResources Analysis Group 2020 for their scientific advice and assistance with the necessary equipment. The authors also thank Keiko Muramatsu, Miho Saiki, Kaoru Hasegawa, Sachiko Amemiya, Megumi Nakata, Mai Fukasawa, Rika Tokoro, Yukiko Sakamoto, Mari Odagawa, and Naoko Hofuku for their assistance with the data management and secretarial work and Dr. Masatsugu Sasamoto and Dr. Misako Tanaka for their medical expertise and valuable advice.
Conflict of interest
The authors declare that the research was conducted in the absence of any commercial or financial relationships that could be construed as a potential conflict of interest.
Publisher’s note
All claims expressed in this article are solely those of the authors and do not necessarily represent those of their affiliated organizations, or those of the publisher, the editors and the reviewers. Any product that may be evaluated in this article, or claim that may be made by its manufacturer, is not guaranteed or endorsed by the publisher.
Supplementary material
The Supplementary Material for this article can be found online at: https://www.frontiersin.org/articles/10.3389/fragi.2024.1331448/full#supplementary-material
SUPPLEMENTARY FIGURE S1 | Normal quantile plots of BMI (A) and AHI (B), and prevalence of SAS according to BMI (C).
SUPPLEMENTARY TABLE S1 | Estimated effect of BMI on AHI parameters using the linear regression model.
SUPPLEMENTARY TABLE S2 | Comparisons between all participants in the high- and low-BMI groups.
SUPPLEMENTARY TABLE S3 | Comparisons between the participants in the high- and low-BMI groups with an AHI ≥15/h in the YHAB dataset.
SUPPLEMENTARY TABLE S4 | Correlation coefficients of indicators of age and sleep parameters in older adults.
Abbreviations
AHI, apnoea-hypopnoea index; BMI, body mass index; SAS, sleep apnoea syndrome; PSG, polysomnography; REM, rapid eye movement.
References
Acosta-Castro, P., Hirotsu, C., Marti-Soler, H., Marques-Vidal, P., Tobback, N., Andries, D., et al. (2018). REM-associated sleep apnoea: prevalence and clinical significance in the HypnoLaus cohort. Eur. Respir. J. 52, 1702484. doi:10.1183/13993003.02484-2017
Aurora, R. N., Chowdhuri, S., Ramar, K., Bista, S. R., Casey, K. R., Lamm, C. I., et al. (2012). The treatment of central sleep apnea syndromes in adults: practice parameters with an evidence-based literature review and meta-analyses. Sleep 35, 17–40. doi:10.5665/sleep.1580
Baldwin, C. M., Griffith, K. A., Nieto, F. J., O’Connor, G. T., Walsleben, J. A., and Redline, S. (2001). The association of sleep-disordered breathing and sleep symptoms with quality of life in the Sleep Heart Health Study. Sleep 24, 96–105. doi:10.1093/sleep/24.1.96
Banno, K., and Kryger, M. H. (2007). Sleep apnea: clinical investigations in humans. Sleep. Med. 8, 400–426. doi:10.1016/j.sleep.2007.03.003
Bassetti, C., and Aldrich, M. S. (1999). Sleep apnea in acute cerebrovascular diseases: final report on 128 patients. Sleep 22, 217–223. doi:10.1093/sleep/22.2.217
Bliwise, D. L., Colrain, I. M., Swan, G. E., and Bliwise, N. G. (2010). Incident sleep disordered breathing in old age. J. Gerontol. A. Biol. Sci. Med. Sci. 65, 997–1003. doi:10.1093/gerona/glq071
Clegg, A., Young, J., Iliffe, S., Rikkert, M. O., and Rockwood, K. (2013). Frailty in elderly people. Lancet 381, 752–762. doi:10.1016/S0140-6736(12)62167-9
Cowie, M. R., Woehrle, H., Wegscheider, K., Angermann, C., d’Ortho, M.-P., Erdmann, E., et al. (2015). Adaptive servo-ventilation for central sleep apnea in systolic heart failure. N. Engl. J. Med. 373, 1095–1105. doi:10.1056/NEJMoa1506459
Dodds, S., Williams, L. J., Roguski, A., Vennelle, M., Douglas, N. J., Kotoulas, S.-C., et al. (2020). Mortality and morbidity in obstructive sleep apnoea-hypopnoea syndrome: results from a 30-year prospective cohort study. ERJ Open Res. 6, 00057–02020. doi:10.1183/23120541.00057-2020
Edwards, B. A., O’Driscoll, D. M., Ali, A., Jordan, A. S., Trinder, J., and Malhotra, A. (2010). Aging and sleep: physiology and pathophysiology. Semin. Respir. Crit. Care. Med. 31, 618–633. doi:10.1055/s-0030-1265902
Ensrud, K. E., Blackwell, T. L., Ancoli-Israel, S., Redline, S., Cawthon, P. M., Paudel, M. L., et al. (2012). Sleep disturbances and risk of frailty and mortality in older men. Sleep. Med. 13, 1217–1225. doi:10.1016/j.sleep.2012.04.010
Ensrud, K. E., Blackwell, T. L., Redline, S., Ancoli-Israel, S., Paudel, M. L., Cawthon, P. M., et al. (2009). Sleep disturbances and frailty status in older community-dwelling men. J. Am. Geriatr. Soc. 57, 2085–2093. doi:10.1111/j.1532-5415.2009.02490.x
Falla, C., Young, A., Pope, A., and O’Driscoll, D. M. (2023). Obstructive sleep apnea during REM sleep: effects on morning and evening blood pressure. Sleep 46, zsac259. doi:10.1093/sleep/zsac259
Fan, Z., Lu, X., Long, H., Li, T., and Zhang, Y. (2019). The association of hemocyte profile and obstructive sleep apnea. J. Clin. Lab. Anal. 33, e22680. doi:10.1002/jcla.22680
Feliciano, A., Linhas, R., Marçôa, R., Cysneiros, A., Martinho, C., Reis, R. P., et al. (2017). Hematological evaluation in males with obstructive sleep apnea before and after positive airway pressure. Rev. Port. Pneumol. 23, 71–78. doi:10.1016/j.rppnen.2016.12.001
Findley, L. J., Fabrizio, M. J., Knight, H., Norcross, B. B., LaForte, A. J., and Suratt, P. M. (1989). Driving simulator performance in patients with sleep apnea. Am. Rev. Respir. Dis. 140, 529–530. doi:10.1164/ajrccm/140.2.529
Heinzer, R., Marti-Soler, H., and Haba-Rubio, J. (2016). Prevalence of sleep apnoea syndrome in the middle to old age general population. Lancet Respir. Med. 4, e5–e6. doi:10.1016/S2213-2600(16)00006-0
Heinzer, R., Vat, S., Marques-Vidal, P., Marti-Soler, H., Andries, D., Tobback, N., et al. (2015). Prevalence of sleep-disordered breathing in the general population: the HypnoLaus study. Lancet Respir. Med. 3, 310–318. doi:10.1016/S2213-2600(15)00043-0
Hudgel, D. W., Lamerato, L. E., Jacobsen, G. R., and Drake, C. L. (2012). Assessment of multiple health risks in a single obstructive sleep apnea population. J. Clin. Sleep. Med. 8, 9–18. doi:10.5664/jcsm.1648
Jennum, P., Baandrup, L., Ibsen, R., and Kjellberg, J. (2015). Increased all-cause mortality with use of psychotropic medication in dementia patients and controls: a population-based register study. Eur. Neuropsychopharmacol. 25, 1906–1913. doi:10.1016/j.euroneuro.2015.08.014
Jordan, A. S., McSharry, D. G., and Malhotra, A. (2014). Adult obstructive sleep apnoea. Lancet 383, 736–747. doi:10.1016/S0140-6736(13)60734-5
Kapur, V. K., Auckley, D. H., Chowdhuri, S., Kuhlmann, D. C., Mehra, R., Ramar, K., et al. (2017). Clinical practice guideline for diagnostic testing for adult obstructive sleep apnea: an American Academy of Sleep Medicine clinical practice guideline. J. Clin. Sleep. Med. 13, 479–504. doi:10.5664/jcsm.6506
Knowles, J. B., and MacLean, A. W. (1990). Age-related changes in sleep in depressed and healthy subjects. A meta-analysis. Neuropsychopharmacology 3, 251–259.
Kondo, N., Kazama, M., Suzuki, K., and Yamagata, Z. (2008). Impact of mental health on daily living activities of Japanese elderly. Prev. Med. 46, 457–462. doi:10.1016/j.ypmed.2007.12.007
Koo, B. B., Patel, S. R., Strohl, K., and Hoffstein, V. (2018). Rapid eye movement-related sleep-disordered breathing: influence of age and gender. Chest 134, 1156–1161. doi:10.1378/chest.08-1311
Lam, J. C. M., Mak, J. C. W., and Ip, M. S. M. (2012). Obesity, obstructive sleep apnoea and metabolic syndrome. Respirology 17, 223–236. doi:10.1111/j.1440-1843.2011.02081.x
Martínez-García, M.-A., Campos-Rodríguez, F., Catalán-Serra, P., Soler-Cataluña, J.-J., Almeida-Gonzalez, C., De la Cruz Morón, I., et al. (2012). Cardiovascular mortality in obstructive sleep apnea in the elderly: role of long-term continuous positive airway pressure treatment: a prospective observational study. Am. J. Respir. Crit. Care. Med. 186, 909–916. doi:10.1164/rccm.201203-0448OC
Matsumoto, T., Murase, K., Tabara, Y., Minami, T., Kanai, O., Takeyama, H., et al. (2020). Sleep disordered breathing and metabolic comorbidities across sex and menopausal status in East Asians: the Nagahama Study. Eur. Respir. J. 56, 1902251. doi:10.1183/13993003.02251-2019
McArdle, N., Reynolds, A. C., Hillman, D., Moses, E., Maddison, K., Melton, P., et al. (2022). Prevalence of common sleep disorders in a middle-aged community sample. J. Clin. Sleep. Med. 18, 1503–1514. doi:10.5664/jcsm.9886
Mohsenin, V., and Valor, R. (1995). Sleep apnea in patients with hemispheric stroke. Arch. Phys. Med. Rehabil. 76, 71–76. doi:10.1016/s0003-9993(95)80046-8
Nadeem, R., Singh, M., Nida, M., Waheed, I., Khan, A., Ahmed, S., et al. (2014). Effect of obstructive sleep apnea hypopnea syndrome on lipid profile: a meta-regression analysis. J. Clin. Sleep. Med. 10, 475–489. doi:10.5664/jcsm.3690
Newman, A. B., Foster, G., Givelber, R., Nieto, F. J., Redline, S., and Young, T. (2005). Progression and regression of sleep-disordered breathing with changes in weight: the Sleep Heart Health Study. Arch. Intern. Med. 165, 2408–2413. doi:10.1001/archinte.165.20.2408
Nittman, S. D., Ayas, N. T., MacDonald, M. M., Malhotra, A., Fogel, R. B., and White, D. P. (2004). Using a wrist-worn device based on peripheral arterial tonometry to diagnose obstructive sleep apnea: in-laboratory and ambulatory validation. Sleep 27, 923–933. doi:10.1093/sleep/27.5.923
Oksenberg, A., Arons, E., Nasser, K., Vander, T., and Radwan, H. (2010). REM-related obstructive sleep apnea: the effect of body position. J. Clin. Sleep. Med. 6, 343–348. doi:10.5664/jcsm.27875
Oldenburg, O., Bitter, T., Weimer, M., Langer, C., Horstkotte, D., and Piper, C. (2009). Pulmonary capillary wedge pressure and pulmonary arterial pressure in heart failure patients with sleep-disordered breathing. Sleep. Med. 10, 726–730. doi:10.1016/j.sleep.2008.08.004
Oldenburg, O., Lamp, B., Faber, L., Teschler, H., Horstkotte, D., and Töpfer, V. (2007). Sleep-disordered breathing in patients with symptomatic heart failure: a contemporary study of prevalence in and characteristics of 700 patients. Eur. J. Heart Fail. 9, 251–257. doi:10.1016/j.ejheart.2006.08.003
Park, I., Kokudo, C., Seol, J., Ishihara, A., Zhang, S., Uchizawa, A., et al. (2022). Instability of non-REM sleep in older women evaluated by sleep-stage transition and envelope analyses. Front. Aging Neurosci. 14, 1050648. doi:10.3389/fnagi.2022.1050648
Parra, O., Arboix, A., Bechich, S., García-Eroles, L., Montserrat, J. M., López, J. A., et al. (2000). Time course of sleep-related breathing disorders in first-ever stroke or transient ischemic attack. Am. J. Respir. Crit. Care. Med. 161, 375–380. doi:10.1164/ajrccm.161.2.9903139
Pépin, J. L., Timsit, J. F., Tamisier, R., Borel, J. C., Lévy, P., and Jaber, S. (2016). Prevention and care of respiratory failure in obese patients. Lancet Respir. Med. 4, 407–418. doi:10.1016/S2213-2600(16)00054-0
Peppard, P. E., Young, T., Barnet, J. H., Palta, M., Hagen, E. W., and Hla, K. M. (2013). Increased prevalence of sleep-disordered breathing in adults. Am. J. Epidemiol. 177, 1006–1014. doi:10.1093/aje/kws342
Philip, P., Sagaspe, P., Taillard, J., Chaumet, G., Bayon, V., Coste, O., et al. (2008). Maintenance of Wakefulness test, obstructive sleep apnea syndrome, and driving risk. Ann. Neurol. 64, 410–416. doi:10.1002/ana.21448
Phillips, B. G., Hisel, T. M., Kato, M., Pesek, C. A., Dyken, M. E., Narkiewicz, K., et al. (1999). Recent weight gain in patients with newly diagnosed obstructive sleep apnea. J. Hypertens. 17, 1297–1300. doi:10.1097/00004872-199917090-00009
Piovezan, R. D., Poyares, D., and Tufik, S. (2013). Frailty and sleep disturbances in the elderly: possible connections and clinical implications. Sleep. Sci. 6, 175–179. doi:10.1016/j.arr.2015.07.003
Strollo, P. J., Soose, R. J., Maurer, J. T., de Vries, N., Cornelius, J., Froymovich, O., et al. (2014). Upper-airway stimulation for obstructive sleep apnea. N. Engl. J. Med. 370, 139–149. doi:10.1056/NEJMoa1308659
Tanaka, Y., Ando, T., Mochizuki, K., Igarashi, S., Tsuchiya, K., Saito, K., et al. (2022). Super-multifactorial survey YHAB revealed high prevalence of sleep apnoea syndrome in unaware older adults and potential combinatorial factors for its initial screening. Front. Aging. 3, 965199. doi:10.3389/fragi.2022.965199
Vanderveken, O. M., Maurer, J. T., Hohenhorst, W., Hamans, E., Lin, H.-S., Vroegop, A. V., et al. (2013). Evaluation of drug-induced sleep endoscopy as a patient selection tool for implanted upper airway stimulation for obstructive sleep apnea. J. Clin. Sleep. Med. 9, 433–438. doi:10.5664/jcsm.2658
White, L. H., and Bradley, T. D. (2013). Role of nocturnal rostral fluid shift in the pathogenesis of obstructive and central sleep apnoea. J. Physiol. 591, 1179–1193. doi:10.1113/jphysiol.2012.245159
Yaggi, H. K., Concato, J., Kernan, W. N., Lichtman, J. H., Brass, L. M., and Mohsenin, V. (2005). Obstructive sleep apnea as a risk factor for stroke and death. N. Engl. J. Med. 353, 2034–2041. doi:10.1056/NEJMoa043104
Yamada, Y., Nishizawa, M., Uchiyama, T., Kasahara, Y., Shindo, M., Miyachi, M., et al. (2017). Developing and validating an age-independent equation using multi-frequency bioelectrical impedance analysis for estimation of appendicular skeletal muscle mass and establishing a cutoff for sarcopenia. Int. J. Environ. Res. Public Health 14, 809. doi:10.3390/ijerph14070809
Young, T., Palta, M., Dempsey, J., Skatrud, J., Weber, S., and Badr, S. (1993). The occurrence of sleep-disordered breathing among middle-aged adults. N. Engl. J. Med. 328 (17), 1230–1235. doi:10.1056/NEJM199304293281704
Keywords: apnoea-hypopnea index, apnoea syndromes, body mass index, sleep stage, older adults, ageing
Citation: Tanaka Y, Baba-Mori N, Yonaga T, Mochizuki K, Igarashi S, Ando T, Kohda T, Ito Y, Soejima K and Sakurai D (2024) Sleep status of older adults with sleep apnoea syndrome may vary by body mass index. Front. Aging 5:1331448. doi: 10.3389/fragi.2024.1331448
Received: 01 November 2023; Accepted: 15 April 2024;
Published: 01 May 2024.
Edited by:
Kieran Reid, Brigham and Women’s Hospital and Harvard Medical School, United StatesReviewed by:
Luis Alberto Gobbo, São Paulo State University, BrazilJason Aziz, Tufts Medical Center, United States
Copyright © 2024 Tanaka, Baba-Mori, Yonaga, Mochizuki, Igarashi, Ando, Kohda, Ito, Soejima and Sakurai. This is an open-access article distributed under the terms of the Creative Commons Attribution License (CC BY). The use, distribution or reproduction in other forums is permitted, provided the original author(s) and the copyright owner(s) are credited and that the original publication in this journal is cited, in accordance with accepted academic practice. No use, distribution or reproduction is permitted which does not comply with these terms.
*Correspondence: Yuji Tanaka, eXVqaS50YW5ha2EuYTNAdG9ob2t1LmFjLmpw