- 1Division of Medical Oncology, Department of Internal Medicine, The Ohio State University, Columbus, OH, United States
- 2Center for Biostatistics, The Ohio State University Wexner Medical Center, Columbus, OH, United States
- 3Division of Cancer Prevention and Control, Department of Medicine, College of Medicine, and The Ohio State University Comprehensive Cancer Center, The Ohio State University Columbus, OH, United States
- 4Pelotonia Institute for Immuno-Oncology, The Ohio State University James Comprehensive Cancer Center–James Cancer Hospital and Solove Research Institute, Columbus, OH, United States
- 5Departments of Molecular Genetics, Cancer Biology, and Genetics, The Ohio State University Comprehensive Cancer Center, Columbus, OH, United States
Introduction: Older adults with chronic disease prioritize functional independence. We aimed to describe the feasibility of capturing functional disability and treatment toxicity among older adults with lung cancer using a longitudinal comprehensive geriatric assessment (CGA) and molecular biomarkers of aging.
Methods: This prospective study included adults ≥60 years with any newly diagnosed non-small-cell lung cancer. Participants were recruited from central Ohio (2018–2020). Study assessments included the Cancer and Aging Research Group CGA (CARG-CGA), short physical performance battery (SPPB), and the blessed orientation-memory concentration (BOMC) test at baseline, 3, 6, and 12 months. Activities of daily living (ADLs) and instrumental ADLs (IADLs), quality of life (QoL, PROMIS 10), and treatment toxicity were captured monthly. Stool and blood were collected to characterize the gut microbiome and age-related blood biomarkers.
Results: This study enrolled 50 participants with an average age of 71.7 years. Ninety-two percent of participants were Caucasian, 58% were male, and all were non-Hispanic. Most had advanced stage (stage III/IV: 90%; stage I/II: 10%), with adenocarcinoma the predominant histologic subtype (68% vs. 24% squamous). First-line treatments included chemotherapy (44%), immune checkpoint inhibitors (ICIs, 22%), chemotherapy and ICIs (30%), or tyrosine kinase inhibitors (4%). The median baseline CARG toxicity score was 8 (range 2–12). Among patients with treatment-related toxicity (n = 49), 39 (79.6%) cases were mild (grade 1–2), and 10 (20.4%) were moderate to severe (≥ grade 3). Treatment toxicity was greater among those with a CARG score ≥8 (28.0% vs. 13.6%). Higher IADL independence, QoL, and SPPB scores at baseline were positively associated with Candidatus Gastranaerophilales bacterium, Lactobacillus rogosae, and Enterobacteria phage P4. Romboutsia ilealis, Streptococcus, and Lachnoclostridium sp An138 and T cell lag3 and cd8a were associated with worse IADLs, QoL, and SPPB scores at baseline.
Discussion: A longitudinal CGA and biomarker collection is feasible among older adults undergoing lung cancer treatment. Gut microbe and T cell gene expression changes correlated with subjective and objective functional status assessments. Future research will test causality in these associations to improve outcomes through novel supportive care interventions to prevent functional disability.
1 Introduction
Lung cancer is the leading cause of cancer-related death in the United States (United States) and is primarily a disease of older adults with a median age of 71 years at diagnosis (Bade and Dela Cruz, 2020). This is critical as older adults are the fastest-growing demographic in the United States, projected to make up almost two-thirds of all cancer diagnoses by 2030 (Smith et al., 2009; Hurria et al., 2013). Despite this knowledge, clinical trials primarily include younger, healthier adults experiencing cancer, leaving clinicians, patients, and families uncertain about treatment-related toxicity, disease response, and functional status (Vora and Reckamp, 2008).
Novel cancer drugs, such as immune checkpoint inhibitors (ICIs) and oral tyrosine kinase inhibitors (TKIs), have dramatically shifted the treatment course for advanced and early-stage non-small-cell lung cancer (NSCLC), further complicating the prediction of treatment tolerability among older adults. However, there is evolving evidence that predictive biomarkers and a comprehensive geriatric assessment (CGA) can identify older adults likely to benefit from cancer treatment, experience toxicity (Corre et al., 2016), suffer functional decline (Presley et al., 2022), and experience worse quality of life (Gomes et al., 2021; Presley et al., 2021). Past research typically involves a single CGA and subsequent outcomes assessments. The feasibility of a longitudinal assessment with repeated measures is unknown. Understanding how GA measures change over time and are associated with treatment toxicity and functional status is critical. Most older adults with serious illnesses, such as advanced cancer, prioritize remaining functionally independent over prolonged survival (Fried et al., 2002; Osoba et al., 2006; Li et al., 2022).
Functional decline and worsening disability among older adults with cancer are typically associated with increased mortality, loss of independent living, and substantial financial costs (Fried and Guralnik, 1997; Guralnik et al., 2002; Fong, 2019). Prior assessment tools, including the Eastern Cooperative Oncology Group (ECOG) performance status, were designed to predict toxicity; but, these tools were developed among patients receiving chemotherapy and not newer treatments such as ICIs and TKIs (Oken et al., 1982; Repetto et al., 2002; Hurria et al., 2011; Extermann et al., 2012; Corre et al., 2016). This is important because even a single cycle of chemotherapy can result in functional decline, depression, and low instrumental activities of daily living (IADL) scores in this vulnerable group (Hoppe et al., 2013). Furthermore, up to 40% of patients with advanced NSCLC experience functional disability at diagnosis (Presley et al., 2016). These facts underscore the need for an improved understanding of the relationship between functional status, treatment outcomes, and survival among patients with lung cancer receiving modern systemic treatments such as ICIs and TKIs (Wong et al., 2022).
The concomitant collection of aging biomarkers has also been absent in previous work. Biological aging of the immune system could impact tumor response to ICIs. Therefore, developing non-invasive tests to understand immune changes during lung cancer treatment is urgently needed. The aging immune system could mean disproportionate rates of autoimmune inflammatory conditions that can occur with ICI treatment, such as rash, thyroiditis, pneumonitis, colitis, arthritis, or hepatitis (Atchley et al., 2021). The gut microbiome is the one biomarker and therapeutic target that can both predict treatment toxicities and is modifiable to improve disease response. Recent findings suggest that certain microbes in the gut modulate the outcome of ICI treatments (Pitt et al., 2017; Routy et al., 2018). The quantification and characterization of immune changes in peripheral blood over time, in conjunction with changes in the gut microbiome, may provide information on how patients are likely to respond to a given treatment. The first step in examining this relationship among humans with solid tumors is longitudinal biospecimen collection and analysis to characterize and describe the diversity among older adults with lung cancer.
This study aimed to 1) test the feasibility of the Cancer and Aging Research Group (CARG) longitudinal CGA, objective physical function and cognitive evaluation, monthly patient-reported outcomes (PROs), and concomitant biospecimen collection among patients with newly diagnosed NSCLC and 2) evaluate associations between the CARG-CGA assessment scores, treatment toxicity, and changes in the gut microbiome and peripheral blood aging biomarkers.
2 Methods
2.1 Study design
This was a pilot prospective longitudinal cohort study of 50 adults ≥60 years of age with any stage of untreated or recurrent NSCLC. Eligible participants underwent a baseline assessment using the CARG CGA (Hurria et al., 2005; Nie et al., 2013). Briefly, this assessment included the CARG chemotherapy toxicity calculator, the Patient-Reported Outcome Measurement Information System (PROMIS 10 (Hays et al., 2009) Global Health Scale Short Form v1.1, and sociodemographic information. The CARG CGA includes self-reported functional status measures, comorbidity, medications, nutrition, psychological state, and social support. A clinical research coordinator administered the cognitive assessment, the Blessed Orientation-Memory-Concentration test (BOMC test (Tuch et al., 2021)), and the short physical performance battery (SPPB) (Guralnik et al., 1994; Kawas et al., 1995). Participants also underwent longitudinal peripheral blood draws and stool sample collection. Participants then received standard-of-care lung cancer treatment or participated in a clinical trial as decided by their treating physician.
2.2 Eligibility criteria
Participants were recruited, consented, and enrolled in the FITNESS study at The Ohio State University Comprehensive Cancer Center (OSUCCC)–James Thoracic Oncology clinic. Eligible patients were ≥60 years of age and intended to receive systemic treatment at the OSUCCC for either a new or recurrent NSCLC diagnosis. Participants were required to have decision-making capacity and willingness to sign informed consent. Patients with any medical conditions, including but not limited to unstable angina pectoris, cardiac arrhythmia, psychiatric illness, or cognitive impairment, without a legally authorized representative (LAR) that would verify compliance with study procedures were excluded.
2.3 Study procedures
Participants underwent a baseline assessment before initiating lung cancer treatment. This process included sociodemographic and disease assessment; CARG toxicity tool calculation (Hurria et al., 2011); stool and blood sample collection; and completion of the CARG CGA, the BOMC, the SPPB (Almugbel et al., 2022), and the timed up-and-go (TUG) (Nakano et al., 2021).
The type of treatment and drug doses (planned and actual) were recorded along with any supportive care medications given throughout treatment. Grade 1–5 toxicities (National Cancer Institute Common Terminology Criteria for Adverse Events, NCI CTCAE V5) (Kluetz et al., 2016), hospitalizations, dose delays and reductions, and drug discontinuation were captured. The toxicity assessment and chemotherapy drug and dose information were collected by a study coordinator at the beginning of every treatment cycle, reviewed and attributed by two certified oncologists. Vital signs, labs, and health-related quality of life questionnaires, including the PROMIS 1028 and European Organization for Research and Treatment of Cancer (EORTC) Quality of Life Questionnaire for Lung Cancer (QLQ-LC-13) (Bergman et al., 1994), were collected at visit 2 (day 90) and visit 3 (day 180). Patients underwent additional monthly functional status assessments (FSA), monthly biospecimen banking, and an additional CGA at visit 3.
Baseline assessments were repeated at the end-of-treatment (EOT) visit. These occurred up to 45 days after completing treatment or 365 days after treatment initiation—whichever occurred first. Due to the varying length of treatment plans and different staging at the time of diagnosis, participants prescribed only four cycles of chemotherapy (adjuvant treatment) were only eligible to participate in the baseline and EOT visits. Visit 1 (baseline), visit 2 (day 90), and visit 3 (day 180) allowed a ±30-day window of time, letting patients enroll up to 30 days after treatment initiation. Disease response was calculated using Response Evaluation Criteria in Solid Tumors (RECIST) 1.1 criteria by two medical oncology fellows using chest cat scan (CT) imaging (Eisenhauer et al., 2009). Target lesions were followed throughout the study at each visit in which imaging was available. Tumor response was categorized into four groups: complete response, partial response, stable disease, and progressive disease.
Stool samples were collected at baseline and during participants’ oncology visits, roughly every 3–4 weeks. Patients were provided with a stool collection tub and collection instructions. Patients were asked to collect a sample within 24 h of returning to the clinic. Once the biospecimen sample reached the lab, it was aliquoted into cryovials and stored at −80°C. Samples were then processed in batches. DNA was extracted using the QIAGEN Power Fecal Pro kit. Sequencing was performed on the Illumina NovaSeq 6,000 platform to a depth of eight million reads per sample. Demultiplexed fastq files were cleaned, paired, and taxonomically assigned following the MetaPhlAn HUMAnN 3 Pipeline (Beghini et al., 2021).
Peripheral blood (40 mL) was collected at baseline, 6 months, and at either 1 year or the end of the patient’s treatment plan. Blood samples were only collected from participants who had not initiated treatment before visit one to control the purity of data. The samples were processed by The Ohio State University Leukemia Tissue Bank (LTB). Peripheral blood T cells were isolated via negative selection using the RosetteSep human T cell isolation cocktail (Stemcell Technologies). Tcell RNA was isolated using a Zymo Research quick-DNA/RNA miniprep kit. The quality and concentration of the resulting RNA was quantified on an Agilent TapeStation and analyzed using a custom NanoString codeset panel, OSU Senescence. The OSU Senescence panel is comprised of 74- T-cell markers with five loading controls. Gene expression markers were selected to measure subset-specific T-cell mRNA markers of cellular senescence and T-cell differentiation proliferation, and exhaustion as previously published (Rosko et al., 2019; Lucas et al., 2020; Fell et al., 2022). Additionally, certain transcription profiles in T cells such as LAG3 and CD8 transcription expression has been shown to be associated with inferior clinical outcomes in patients with myeloma (Lucas et al., 2020). T cells are exhausted when cells lose cytotoxic capacity, show sustained expression of inhibitory checkpoint molecules such as LAG3 and have distinct altered transcription profiles in CD8 subsets (Lucas et al., 2020). Therefore, LAG3 and CD8 transcription profiles were examined for associations with longitudinal IADL scores.
2.4 Statistical analysis
The primary aim of this study was to assess the feasibility of a longitudinal geriatric assessment collected concurrently with blood and stool biomarkers. Metrics included the number of blood and stool samples collected, the completion of PRO assessments, and patient withdrawal. Feasibility was defined a priori as the proportion of missing information at each time point, with success defined as at least 50% of participants able to complete study visits 2, 3, and four or EOT. Reasons for missed collections and study withdrawals were captured.
Descriptive statistics were used to summarize demographic information, with totals and percentages used for categorical data and medians and interquartile ratio (IQR) used for continuous data. When determining the immune-related adverse event (irAE) grade for a given time point, the grade of the most extreme irAE for that time point was used. For association with baseline CARG, the maximum-grade irAE experienced by the patient was considered.
Microbe and gene expression associations with the CGA were performed using longitudinal mixed-effects models using the {lme4} and {lmerTest} packages in R (Bates et al., 2015). A model was run for each combination of GA tool (i.e., SPPB or IADL), microbe, and gene. For associations between microbes and the selected GA tool, each GA tool was considered an independent variable and all other variables were considered dependent variables. Repeated measures in patients were handled using random effects for each patient. All other effects were fixed. The longitudinal nature of the data was accounted for by including a term for the number of days on the study associated with each measure. The models can be described by the following equation, in which the term Ai is the score (A) for each geriatric assessment(i), Mj is the relative abundance (M) for each microbe (j), D is days on study, C is immune checkpoint inhibitor, (1|Pk) is the intercept (1|P) for each patient (k):
For transcript and geriatric assessment tools, variables were centered and scaled before modeling. Geriatric assessments remained the independent variable and patient intercepts remain random effects. The effects of change over time are still controlled for by including days on study in the model. These models can be described with the following equation, where Ai is the score (A) geriatric assessment of interest (i), Gj is the expression (G) of the gene of interest (i), D is days on study, (1|Pk) is the intercept (1|P) for each patient (k):
Analyses were performed in R, version 4.1.
3 Results
3.1 Feasibility
The study enrolled 61 patients with 11 withdrawals prior to starting (18% dropout rate), resulting in 50 evaluable participants at baseline. Among the 11 patients who dropped out before the study began, the reasons for withdrawal included disease progression, declining performance status, unwillingness to complete the study assessments, and study burden, including increased visit time and survey fatigue. Among the 50 participants, one withdrew at visit one due to cognitive impairment without an LAR. Reasons for drop out prior to the 3-month time point were death (n = 3) and withdrawal (n = 4). Inclusion criteria required participants to be undergoing active treatment versus surveillance for longitudinal follow up; therefore, the three- and 6-month time points excluded an additional 11 participants as they had completed treatment (adjuvant chemotherapy). Excluding the 11 adjuvant chemotherapy participants, at 3-months, 28 of 31 eligible participants (90%) completed the visit two assessments. Excluding the 11 adjuvant chemotherapy patients, at 6 months, 23 of 25 eligible participants (92%) had completed the study assessments (study visit 3). Reasons for drop out prior to 6 months were death (n = 4) and withdrawal (n = 2). For study visit 4, 30 (97%) of the remaining 31 participants completed the assessments. Reasons for drop out prior to 1-year were death (n = 4) and withdrawal (n = 1). The overall study attrition rate was 38%, excluding those who wished to continue participation after refusing to complete at least one study visit. Common reasons for study drop out included death (22%), loss of interest in participation (14%), and cognitive impairment (2%)—The study CONSORT diagram in Figure 1A details study enrollment, follow-up, and dropout.
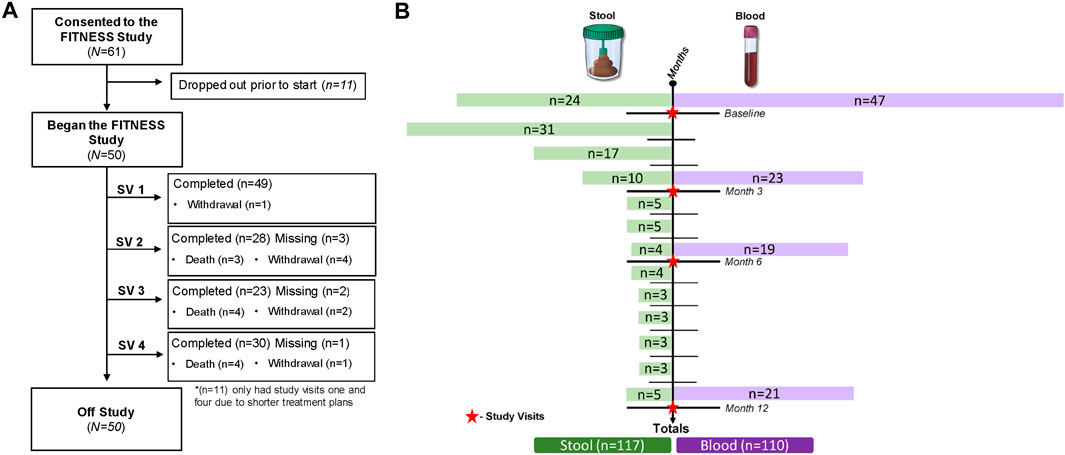
Figure 1. Study enrollment, follow-up, dropout, and biomarker collection. (A) CONSORT diagram; (B) longitudinal biomarker collection.
The collection of longitudinal gut microbiome samples posed challenges, including patient hesitancy, transportation difficulties for those with longer travel times, and constipation before the return-to-clinic visit. Despite these barriers, 113 stool samples were collected, including 24 baseline (treatment-naive) specimens. Of those, 23 (95.8%) participants provided longitudinal samples for correlative analysis. See Figure 1B for a detailed overview of longitudinal biomarker collection.
3.2 Patient characteristics
The mean age at baseline was 71.7 years (60–88 years). Most participants were Caucasian (92%), and 58% of patients were male. The most common histology was adenocarcinoma (70%), followed by squamous cell carcinoma (24%). Most cases were advanced stage (90% stage III/IV). For first-line treatment, 44% of patients received chemotherapy, 30% received a combination of chemotherapy and ICIs, 22% received ICIs alone, and 2 (4%) received TKIs. The mean CARG chemotherapy toxicity score at baseline was 7.5 (range 2–12), indicating an intermediate risk of treatment toxicity. Patient demographics and characteristics are further detailed in Table 1. Patient treatment timelines with the treatment types, treatment responses, and events, including toxicities and biomarker obtainment, are displayed in Figure 2.
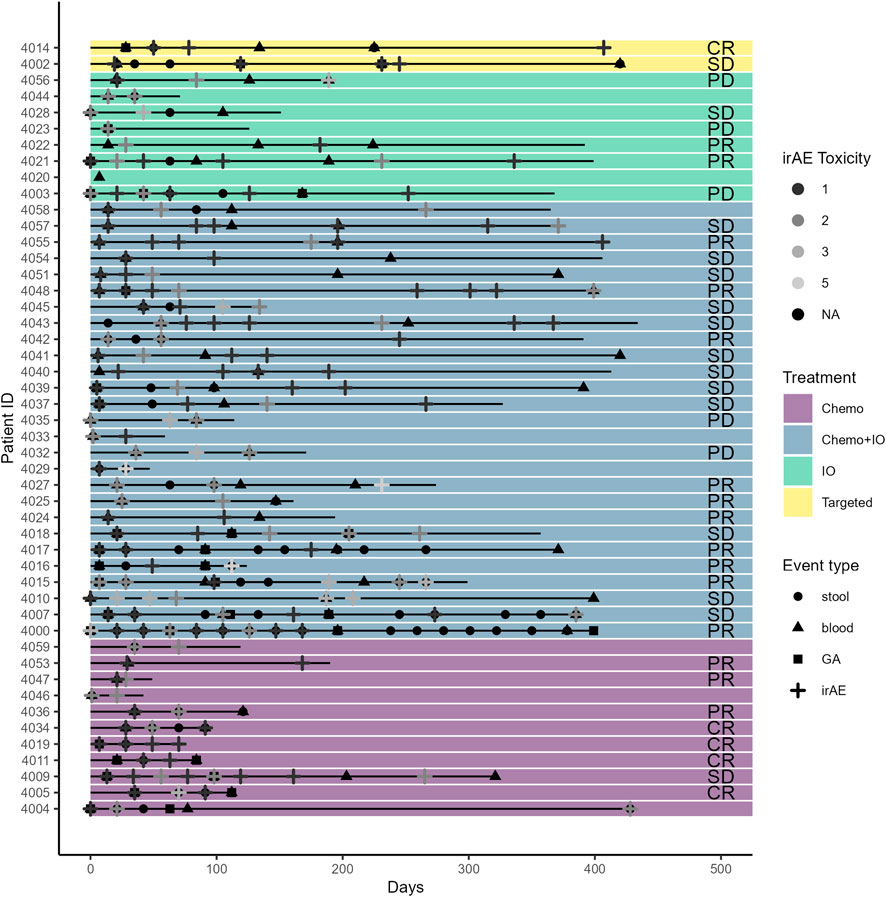
Figure 2. Treatment type, toxicity, geriatric assessment, and biospecimen timeline for study participants.
3.3 Comprehensive geriatric assessment and patient-reported outcomes
The CARG-CGA subset on IADLs (median: 14), Medical Outcomes Study (MOS) Physical Health (median: 61), Self-report Health Rating (median: 90), and the MOS Mental Health Inventory (median: 81) indicate relatively high levels of independence and mental health statuses among this cohort at baseline. Similarly, the PROMIS 10 average functional disability score (median: 24) and the EORTC-LC subset score (median: 17) indicated minimal to no baseline disability and minimal levels of symptom burden (Table 2; complete results including longitudinal data appear in Supplementary Table S4). While participants scored highly on the Social Support Medical Outcomes Survey (median: 96), their scores demonstrated a moderate impact to their usual levels of social activities (median: 58). The CARG-CGA Comorbidity Index showed that participants had an average of six pre-existing comorbid conditions present at baseline.
Using the CARG chemotherapy toxicity tool, 22 patients (47%) had a low or mild risk of chemotherapy toxicity, and 25 (53%) had a high risk of chemotherapy toxicity (Table 2). CARG toxicity scores were useful in predicting the presence of at least one grade 3 or higher adverse event (Figure 3). The majority (74%) of participants experienced a grade 1 or two treatment-related adverse events and 10 (20%) of participants experienced a grade 3 or higher adverse event. Participants who scored ≤7 on the CARG toxicity assessment tool (47%) had three participants with a ≥grade 3 while those that scored ≥8 (53%) included seven participants that experienced a ≥grade 3 (70%) that occurred during this study. Patient-reported outcomes measuring physical function (IADLs, PROMIS 10, MOS) among this cohort did not show high levels of disability while the SPPB suggests the presence of physical impairment (median: 9) and 19 participants (38%) had TUG scores that indicated a mild risk for falls. At baseline, participants showed a mild to moderate concern for cognitive impairment (BOMC (Tuch et al., 2021) median: 6) (Table 2).
3.4 Relationship between geriatric assessments and molecular biomarkers
Changes in GA tools over time were associated with changes in molecular features, including the gut microbiome and T-cell senescence markers. For example, Lactobacillus rogosae, was associated with increased (improving) IADL, QoL, and SPPB scores (Figure 4A; complete results with all p-values corrected for multiple hypothesis testing appear in Supplementary Table S1). In contrast, the microbes Romboutsia ilealis, Streptococcus, and Lachnoclostridium sp An138 were associated with decreased (worsening) IADL, QoL, and SPPB scores over time (Figure 4A). Similarly, T-cell lag3 and cd8a RNA expression over time were associated with decreased IADL scores (Figure 4B; complete results with all p-values corrected for multiple hypothesis testing appear in Supplementary Table S2, with results of an additional sensitivity analysis of these results using Spearman correlations appearing in Supplementary Table S3 and the full dataset appearing in Supplementary Table S4).
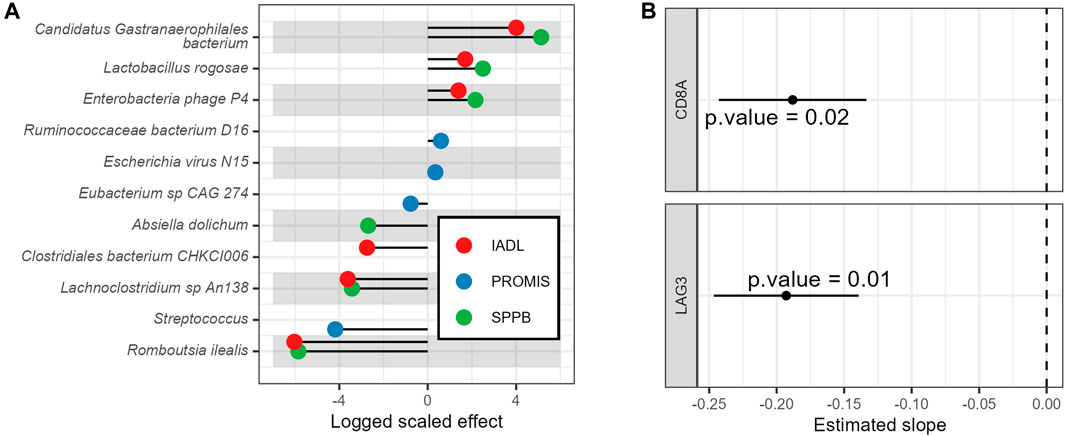
Figure 4. Associations of baseline vs. longitudinal geriatric assessments with molecular biomarkers. (A) Longitudinal associations of geriatric assessments with gut microbe relative abundances and (B) Longitudinal associations of IADL with T-cell gene expression. Displayed are the model’s estimated slopes with 95% confidence interval between genes and IADL.
4 Discussion
This study builds upon prior research supporting the utility of the CARG CGA in patients with lung cancer and describes the feasibility of longitudinal data and biospecimen capture in this patient population. Our comprehensive study design captured the GA longitudinally, included patients receiving the newest lung cancer treatments, and collected blood and stool samples for correlative biomarker analysis, resulting in a rich dataset. At baseline, patients reported minimal functional impairment and relatively high social support and exhibited normal levels of mobility as measured by the TUG. However, we uncovered mild to moderate levels of cognitive impairment using the BOMC and physical function impairment using the SPPB.
This study demonstrates the feasibility of instituting the CARG CGA longitudinally among older adults with lung cancer, with ≥50% of participants completing the surveys at each time point. The CARG toxicity calculator may also be useful in predicting treatment toxicity risk among older adults with NSCLC receiving newer treatments. We suspect withdrawal due to study burden (increased visit time and survey fatigue) was largely a result of the inclusion of recently diagnosed patients who were beginning treatment, contributing to general overwhelming feelings upon arrival at study visit 1.
We gained valuable insights into the preferred timing and modality of longitudinal GAs among older adults diagnosed with lung cancer. Due to patient status decline and death, we amended this study to include an additional GA at study visit 3, or roughly 6 months into treatment. We hypothesize that more frequent assessments would improve follow-up data capture for longitudinal data collection among participants with advanced disease. Additionally, we hypothesized that offering patients the option to complete study surveys virtually through a secure email link, allowing them to complete them independently rather than during their oncology visits, may mitigate survey fatigue and, ultimately, study dropout rates.
Prior research using GAs for various cancer types lacked physical performance metrics and relied solely on blood-based biomarkers (Antonio et al., 2018; Honecker et al., 2018; Loh et al., 2020). Furthermore, most current literature on older adults only included patients 70 or older, overlooking potential differences in chronologic versus physiologic aging (Schulkes et al., 2016). Physiologic aging is a complex process dependent on many biological and sociodemographic factors (Dziechciaz and Filip, 2014). To better account for biological aging-associated changes, this study included adults ≥60 years of age.
One limitation of this study is the dropout rate, leading to missing longitudinal data for many participants. The study follow-up length likely contributed to the high attrition rate due to death, as those who died while on the study had more advanced stages of the disease and thus longer treatment plans, permitting study enrollment for up to 12 months. In general, participants on shorter treatment plans were only asked to participate in the baseline and EOT visits and likely presented with early-stage disease with a lower risk of adverse events and disease complications. Another limitation of this data is the sample homogeneity. Thus, our findings may only be applicable to sociodemographic groups within this single-site center. While a proportion of participants declined or had poor adherence to stool sample collection, we gained valuable insights and strategies to enhance future gut microbiome studies in older adults with lung cancer. Patients who provided a baseline stool sample were likelier to submit a follow-up sample than those who did not participate at baseline. We hypothesize that reminding participants via phone 24–48 h before their clinic visit to collect stool samples, with incentives offered, could improve adherence and overall acceptability of sample procurement.
Future research should investigate whether modifying these biomarkers can impact treatment outcomes and establish causality in these associations. One upcoming randomized clinical trial (Geriatric Assessment and Management for Older Adults with NSCLC Receiving Chemotherapy Radiation Therapy (GAM-CRT (NCT06139627)), open to accrual, will examine the association between geriatric assessments and physician recommendations with grade 3–5 treatment toxicities. The GAM-CRT trial will continue to explore the relationship between microbial diversity and blood-based biomarkers with treatment-related toxicity and disease response. Additionally, the collection of PROs could be integrated into the electronic medical record (EMR) for improved care pathway implementation. While our institution has since incorporated PROs including the Cancer and Aging Research Group Chemo -Toxicity Calculator, The Geriatric 8 Screener, Generalized Anxiety Disorder −7 (GAD-7), Patient Health Questionnaire–9 (PHQ-9), and the European Quality of Life–5 Dimension (EQ-5D-5L) into the EMR, there are no current care pathways. This is an area for future development in which the GAM-CRT trial will help to distinguish these optimal recommendations such as referrals and other supportive care interventions.
In conclusion, our findings suggest that the CARG CGA can be successfully administered to newly diagnosed older adults with lung cancer prior to treatment initiation and at follow-up. However, assessments should be conducted at intervals of no longer than 3 months to improve longitudinal data collection. We found that baseline and longitudinal blood and stool samples were attainable and could be assessed for correlation with physical function measures, including the SPPB, and PROs, such as IADL impairment and quality of life. We plan to continue evaluating multidisciplinary GAs and their associations with correlative biomarkers and clinical outcomes to refine the gold standard for geriatric assessment procedures. Ultimately, the knowledge gained will improve therapeutic decision-making, risk assessment, patient prioritization, and quality of life for older adults with lung cancer.
Data availability statement
The data and code to regenerate all analyses is publicly available in the http://github.com/spakowiczlab/fitness repository, at DOI 10.5281/zenodo.11200745.
Ethics statement
The studies involving humans were approved by The Ohio State University Institutional Review Board (IRB) #2018C0069. The studies were conducted in accordance with the local legislation and institutional requirements. The participants provided their written informed consent to participate in this study.
Author contributions
MG: Investigation, Project administration, Writing–original draft, Writing–review and editing. RH: Data curation, Formal Analysis, Software, Validation, Visualization, Writing–review and editing. JB: Writing–review and editing, Data curation, Formal Analysis, Validation, Visualization. SJ: Formal Analysis, Writing–review and editing. NW: Writing–review and editing, Data curation. MN: Writing–review and editing, Conceptualization. CB: Writing–review and editing, Resources. EP: Writing–review and editing, Conceptualization. AR: Writing–review and editing, Funding acquisition, Resources. DS: Funding acquisition, Resources, Writing–review and editing, Data curation, Methodology, Validation, Visualization. CP: Funding acquisition, Methodology, Resources, Writing–review and editing, Conceptualization, Investigation, Project administration, Supervision, Writing–original draft.
Funding
The author(s) declare that financial support was received for the research, authorship, and/or publication of this article. This work was supported by the National Institute on Aging (CJP: 1K76AG074923-01, R03AG064374; DJS: K01AG070310), a Young Investigator Research Award in Immuno-oncology from the Lung Cancer Foundation of America (LCFA)-Bristol Myers Squibb (BMS)-International Lung Cancer Foundation (ILCF) (DJS), and the cancer research non-profit Pelotonia (CJP: Pelotonia Junior Investigator Award, K12). American Cancer Society Grant (RSG-23-1023205-01-CSCT) for DJS.
Acknowledgments
The authors thank Angela Dahlberg, Senior Editor in the Division of Medical Oncology at OSUCCC, for editing and proofreading this manuscript.
Conflict of interest
The authors declare that the research was conducted in the absence of any commercial or financial relationships that could be construed as a potential conflict of interest.
Publisher’s note
All claims expressed in this article are solely those of the authors and do not necessarily represent those of their affiliated organizations, or those of the publisher, the editors and the reviewers. Any product that may be evaluated in this article, or claim that may be made by its manufacturer, is not guaranteed or endorsed by the publisher.
Supplementary material
The Supplementary Material for this article can be found online at: https://www.frontiersin.org/articles/10.3389/fragi.2024.1268232/full#supplementary-material
SUPPLEMENTARY TABLE S1 | Longitudinal Models for Microbes and Geriatric Assessments 574.
SUPPLEMENTARY TABLE S2 | Longitudinal Models for Nanostring Results and Geriatric Assessments.
SUPPLEMENTARY TABLE S3 | Longitudinal Spearman Correlations for Select Microbes, Nanostring Results, and Geriatric Assessments.
SUPPLEMENTARY TABLE S4 | Complete Longitudinal Clinical Source Data.
References
Almugbel, F. A., Timilshina, N., Papadopoulos, E., Al-Showbaki, L., and Alibhai, S. M. H. (2022). The role of grip strength and short physical performance battery test in predicting chemotherapy-related outcomes in older adults with cancer. J. Geriatric Oncol. 13, 318–324. doi:10.1016/j.jgo.2021.12.002
Antonio, M., Saldana, J., Linares, J., Ruffinelli, J. C., Palmero, R., Navarro, A., et al. (2018). Geriatric assessment may help decision-making in elderly patients with inoperable, locally advanced non-small-cell lung cancer. Br. J. Cancer 118, 639–647. doi:10.1038/bjc.2017.455
Atchley, W. T., Alvarez, C., Saxena-Beem, S., Schwartz, T. A., Ishizawar, R. C., Patel, K. P., et al. (2021). Immune checkpoint inhibitor-related pneumonitis in lung cancer: real-world incidence, risk factors, and management practices across six health care centers in North Carolina. Chest 160, 731–742. doi:10.1016/j.chest.2021.02.032
Bade, B. C., and Dela Cruz, C. S. (2020). Lung cancer 2020: epidemiology, etiology, and prevention. Clin. Chest Med. 41, 1–24. doi:10.1016/j.ccm.2019.10.001
Bates, D., Mächler, M., Bolker, B., and Walker, S. (2015). Fitting linear mixed-effects models using lme4. J. Stat. Softw. 67, 1–48. doi:10.18637/jss.v067.i01
Beghini, F., McIver, L. J., Blanco-Miguez, A., Dubois, L., Asnicar, F., Maharjan, S., et al. (2021). Integrating taxonomic, functional, and strain-level profiling of diverse microbial communities with bioBakery 3. Elife 10, e65088. doi:10.7554/eLife.65088
Bergman, B., Aaronson, N. K., Ahmedzai, S., Kaasa, S., and Sullivan, M. (1994). The EORTC QLQ-LC13: a modular supplement to the EORTC core quality of life questionnaire (QLQ-C30) for use in lung cancer clinical trials. EORTC study group on quality of life. Eur. J. Cancer 30, 635–642. doi:10.1016/0959-8049(94)90535-5
Corre, R., Greillier, L., Le Caër, H., Audigier-Valette, C., Baize, N., Bérard, H., et al. (2016). Use of a comprehensive geriatric assessment for the management of elderly patients with advanced non–small-cell lung cancer: the phase III randomized ESOGIA-GFPC-GECP 08-02 study. J. Clin. Oncol. 34, 1476–1483. doi:10.1200/JCO.2015.63.5839
Dziechciaz, M., and Filip, R. (2014). Biological psychological and social determinants of old age: bio-psycho-social aspects of human aging. Ann. Agric. Environ. Med. 21, 835–838. doi:10.5604/12321966.1129943
Eisenhauer, E. A., Therasse, P., Bogaerts, J., Schwartz, L. H., Sargent, D., Ford, R., et al. (2009). New response evaluation criteria in solid tumours: revised RECIST guideline (version 1.1). Eur. J. Cancer 45, 228–247. doi:10.1016/j.ejca.2008.10.026
Extermann, M., Boler, I., Reich, R. R., Lyman, G. H., Brown, R. H., DeFelice, J., et al. (2012). Predicting the risk of chemotherapy toxicity in older patients: the chemotherapy risk assessment Scale for high-age patients (CRASH) score. Cancer 118, 3377–3386. doi:10.1002/cncr.26646
Fell, G., Rosko, A. A.-O., Abel, G. A.-O., Dumontier, C., Higby, K. J., Murillo, A., et al. (2022). Peripheral blood CD3(+) T-cell gene expression biomarkers correlate with clinical frailty in patients with haematological malignancies. Br. J. Haematol. 199 (1), 100–105. doi:10.1111/bjh.18336
Fong, J. H. (2019). Disability incidence and functional decline among older adults with major chronic diseases. BMC Geriatr. 19, 323. doi:10.1186/s12877-019-1348-z
Fried, L. P., and Guralnik, J. M. (1997). Disability in older adults: evidence regarding significance, etiology, and risk. J. Am. Geriatrics Soc. 45, 92–100. doi:10.1111/j.1532-5415.1997.tb00986.x
Fried, T. R., Bradley, E. H., Towle, V. R., and Allore, H. (2002). Understanding the treatment preferences of seriously ill patients. N. Engl. J. Med. 346, 1061–1066. doi:10.1056/NEJMsa012528
Gomes, F., Lorigan, P., Woolley, S., Foden, P., Burns, K., Yorke, J., et al. (2021). A prospective cohort study on the safety of checkpoint inhibitors in older cancer patients - the ELDERS study. ESMO Open 6, 100042. doi:10.1016/j.esmoop.2020.100042
Guralnik, J. M., Alecxih, L., Branch, L. G., and Wiener, J. M. (2002). Medical and long-term care costs when older persons become more dependent. Am. J. Public Health 92, 1244–1245. doi:10.2105/ajph.92.8.1244
Guralnik, J. M., Simonsick, E. M., Ferrucci, L., Glynn, R. J., Berkman, L. F., Blazer, D. G., et al. (1994). A short physical performance battery assessing lower-extremity function - association with self-reported disability and prediction of mortality and nursing-home admission. Journals Gerontology 49, M85–M94. doi:10.1093/geronj/49.2.m85
Hays, R. D., Bjorner, J. B., Revicki, D. A., Spritzer, K. L., and Cella, D. (2009). Development of physical and mental health summary scores from the patient-reported outcomes measurement information system (PROMIS) global items. Qual. Life Res. 18, 873–880. doi:10.1007/s11136-009-9496-9
Honecker, F., Harbeck, N., Schnabel, C., Wedding, U., Waldenmaier, D., Saupe, S., et al. (2018). Geriatric assessment and biomarkers in patients with metastatic breast cancer receiving first-line mono-chemotherapy: results from the randomized phase III PELICAN trial. J. Geriatr. Oncol. 9, 163–169. doi:10.1016/j.jgo.2017.09.009
Hoppe, S., Rainfray, M., Fonck, M., Hoppenreys, L., Blanc, J. F., Ceccaldi, J., et al. (2013). Functional decline in older patients with cancer receiving first-line chemotherapy. J. Clin. Oncol. 31, 3877–3882. doi:10.1200/JCO.2012.47.7430
Hurria, A., Gupta, S., Zauderer, M., Zuckerman, E. L., Cohen, H. J., Muss, H., et al. (2005). Developing a cancer-specific geriatric assessment: a feasibility study. Cancer 104, 1998–2005. doi:10.1002/cncr.21422
Hurria, A., Naylor, M., and Cohen, H. (2013). Improving the quality of cancer care in an aging population: recommendations from an iom report. JAMA 310, 1795–1796. doi:10.1001/jama.2013.280416
Hurria, A., Togawa, K., Mohile, S. G., Owusu, C., Klepin, H. D., Gross, C. P., et al. (2011). Predicting chemotherapy toxicity in older adults with cancer: a prospective multicenter study. J. Clin. Oncol. 29, 3457–3465. doi:10.1200/JCO.2011.34.7625
Kawas, C., Karagiozis, H., Resau, L., Corrada, M., and Brookmeyer, R. (1995). Reliability of the blessed telephone information-memory-concentration test. J. Geriatr. Psychiatry Neurol. 8, 238–242. doi:10.1177/089198879500800408
Kluetz, P. G., Chingos, D. T., Basch, E. M., and Mitchell, S. A. (2016). Patient-reported outcomes in cancer clinical trials: measuring symptomatic adverse events with the national cancer institute’s patient-reported outcomes version of the common Terminology criteria for adverse events (PRO-CTCAE). Am. Soc. Clin. Oncol. Educ. Book 35, 67–73. doi:10.1200/EDBK_159514
Li, D., Sun, C. L., Kim, H., Crook, C., Zhang, Y. H., Allen, R., et al. (2022). Patient-defined goals and preferences among adults with advanced neuroendocrine tumors. J. Natl. Compr. Canc Netw. 20, 1330–1337 e3. doi:10.6004/jnccn.2022.7059
Loh, K. P., Tooze, J. A., Nicklas, B. J., Kritchevsky, S. B., Williamson, J. D., Ellis, L. R., et al. (2020). Inflammatory biomarkers, geriatric assessment, and treatment outcomes in acute myeloid leukemia. J. Geriatr. Oncol. 11, 410–416. doi:10.1016/j.jgo.2019.03.014
Lucas, F., Pennell, M., Huang, Y., Benson, D. M., Efebera, Y. A., Chaudhry, M., et al. (2020). T cell transcriptional profiling and immunophenotyping uncover LAG3 as a potential significant target of immune modulation in multiple myeloma. Biol. Blood Marrow Transpl. 26, 7–15. doi:10.1016/j.bbmt.2019.08.009
Nakano, J., Fukushima, T., Tanaka, T., and Morishita, S. (2021). Physical function predicts mortality in patients with cancer: a systematic review and meta-analysis of observational studies. Support. Care Cancer 29, 5623–5634. doi:10.1007/s00520-021-06171-3
Nie, X., Liu, D., Li, Q., and Bai, C. (2013). Predicting chemotherapy toxicity in older adults with lung cancer. J. Geriatric Oncol. 4, 334–339. doi:10.1016/j.jgo.2013.05.002
Oken, M. M., Creech, R. H., Tormey, D. C., Horton, J., Davis, T. E., McFadden, E. T., et al. (1982). Toxicity and response criteria of the eastern cooperative oncology group. Am. J. Clin. Oncol. 5, 649–656. doi:10.1097/00000421-198212000-00014
Osoba, D., Hsu, M. A., Copley-Merriman, C., Coombs, J., Johnson, F. R., Hauber, B., et al. (2006). Stated preferences of patients with cancer for health-related quality-of-life (HRQOL) domains during treatment. Qual. Life Res. 15, 273–283. doi:10.1007/s11136-005-0580-5
Pitt, J. M., Vetizou, M., Gomperts Boneca, I., Lepage, P., Chamaillard, M., and Zitvogel, L. (2017). Enhancing the clinical coverage and anticancer efficacy of immune checkpoint blockade through manipulation of the gut microbiota. Oncoimmunology 6, e1132137. doi:10.1080/2162402X.2015.1132137
Presley, C. J., Arrato, N. A., Shields, P. G., Carbone, D. P., Wong, M. L., Benedict, J., et al. (2022). Functional trajectories and resilience among adults with advanced lung cancer. JTO Clin. Res. Rep. 3, 100334. doi:10.1016/j.jtocrr.2022.100334
Presley, C. J., Gomes, F., Burd, C. E., Kanesvaran, R., and Wong, M. L. (2021). Immunotherapy in older adults with cancer. J. Clin. Oncol. 39, 2115–2127. doi:10.1200/JCO.21.00138
Presley, C. J., Soulos, P. R., Tinetti, M., Montori, V. M., Yu, J. B., and Gross, C. P. (2016). Treatment burden of medicare beneficiaries with stage I non–small-cell lung cancer. J. Oncol. Pract. 13, e98–e107. doi:10.1200/JOP.2016.014100
Repetto, L., Fratino, L., Audisio, R. A., Venturino, A., Gianni, W., Vercelli, M., et al. (2002). Comprehensive geriatric assessment adds information to eastern cooperative oncology group performance status in elderly cancer patients: an Italian group for geriatric oncology study. J. Clin. Oncol. 20, 494–502. doi:10.1200/JCO.2002.20.2.494
Rosko, A. E., Huang, Y., Benson, D. M., Efebera, Y. A., Hofmeister, C., Jaglowski, S., et al. (2019). Use of a comprehensive frailty assessment to predict morbidity in patients with multiple myeloma undergoing transplant. J. Geriatr. Oncol. 10, 479–485. doi:10.1016/j.jgo.2018.05.015
Routy, B., Le Chatelier, E., Derosa, L., Duong, C. P. M., Alou, M. T., Daillère, R., et al. (2018). Gut microbiome influences efficacy of PD-1-based immunotherapy against epithelial tumors. Science 359, 91–97. doi:10.1126/science.aan3706
Schulkes, K. J., Hamaker, M. E., van den Bos, F., and van Elden, L. J. R. (2016). Relevance of a geriatric assessment for elderly patients with lung cancer-A systematic review. Clin. Lung Cancer 17, 341–349. doi:10.1016/j.cllc.2016.05.007
Smith, B. D., Smith, G. L., Hurria, A., Hortobagyi, G. N., and Buchholz, T. A. (2009). Future of cancer incidence in the United States: burdens upon an aging, changing nation. J. Clin. Oncol. 27, 2758–2765. doi:10.1200/JCO.2008.20.8983
Tuch, G., Soo, W. K., Luo, K. Y., Frearson, K., Oh, E. L., Phillips, J. L., et al. (2021). Cognitive assessment tools recommended in geriatric oncology guidelines: a rapid review. Curr. Oncol. 28, 3987–4003. doi:10.3390/curroncol28050339
Vora, N., and Reckamp, K. L. (2008). Non-small cell lung cancer in the elderly: defining treatment options. Semin. Oncol. 35, 590–596. doi:10.1053/j.seminoncol.2008.08.009
Keywords: lung cancer, functional decline, aging, biomarkers, microbiome, novel treatments
Citation: Grogan M, Hoyd R, Benedict J, Janse S, Williams N, Naughton M, Burd CE, Paskett ED, Rosko A, Spakowicz DJ and Presley CJ (2024) The FITNESS study: longitudinal geriatric assessment, treatment toxicity, and biospecimen collection to assess functional disability among older adults with lung cancer. Front. Aging 5:1268232. doi: 10.3389/fragi.2024.1268232
Received: 27 July 2023; Accepted: 06 May 2024;
Published: 07 June 2024.
Edited by:
Chiara Moretti, University of Gothenburg, SwedenReviewed by:
Jon Ambæk Durhuus, Hvidovre Hospital, DenmarkCarole Helissey, Hôpital d’Instruction des Armées Bégin, France
Copyright © 2024 Grogan, Hoyd, Benedict, Janse, Williams, Naughton, Burd, Paskett, Rosko, Spakowicz and Presley. This is an open-access article distributed under the terms of the Creative Commons Attribution License (CC BY). The use, distribution or reproduction in other forums is permitted, provided the original author(s) and the copyright owner(s) are credited and that the original publication in this journal is cited, in accordance with accepted academic practice. No use, distribution or reproduction is permitted which does not comply with these terms.
*Correspondence: Carolyn J. Presley, carolyn.presley@osumc.edu
†These authors have contributed equally to this work