- Laboratory Genetic Metabolic Diseases, Amsterdam Gastroenterology, Endocrinology, and Metabolism, Amsterdam Cardiovascular Sciences, Amsterdam UMC location University of Amsterdam, Amsterdam, Netherlands
Public attention and interest for longevity interventions are growing. These can include dietary interventions such as intermittent fasting, physical interventions such as various exercise regimens, or through supplementation of nutraceuticals or administration of pharmaceutics. However, it is unlikely that most interventions identified in model organisms will translate to humans, or that every intervention will benefit each person equally. In the worst case, even detrimental health effects may occur. Therefore, identifying longevity interventions using human data and tracking the aging process in people is of paramount importance as we look towards longevity interventions for the public. In this work, we illustrate how to identify candidate longevity interventions using population data in humans, an approach we have recently employed. We consider metformin as a case-study for potential confounders that influence effectiveness of a longevity intervention, such as lifestyle, sex, genetics, age of administration and the microbiome. Indeed, metformin, like most other longevity interventions, may end up only benefitting a subgroup of individuals. Fortunately, technologies have emerged for tracking the rate of ‘biological’ aging in individuals, which greatly aids in assessing effectiveness. Recently, we have demonstrated that even wearable devices, accessible to everyone, can be used for this purpose. We therefore propose how to use such approaches to test interventions in the general population. In summary, we advocate that 1) not all interventions will be beneficial for each individual and therefore 2) it is imperative that individuals track their own aging rates to assess healthy aging interventions.
Introduction
Increased life expectancy in human populations has been accompanied by increased rates of age-related diseases. This can include non-communicable age-related diseases, including cardiovascular diseases, neurodegenerative diseases, chronic kidney disease, and metabolic diseases such as obesity and type 2 diabetes. Advanced age also results in an increased susceptibility to communicable diseases, resulting in potentially severe clinical outcome upon influenza or COVID-19 infections. Aging has long been considered to be a passive process that could not be reverted or slowed down. However, it is now clear that the aging program is actively regulated (López-Otín et al., 2013), a discovery that has been accompanied by a paradigm shift in thinking; that regulating aging can reduce the burden of age-related diseases.
Although it has been a long time coming, the World Health Organization now defines aging as a disease in the latest version of the International Classification of Diseases (ICD-11, code ‘Ageing-related’ XT9T) (Khaltourina et al., 2020). The increased recognition of aging as a disease is meaningful for the development of future therapeutic interventions or strategies targeting aging and aging-related diseases (The Lancet Diabetes and End, 2018). Several classes of compounds that extend lifespan in model organisms hold great promise for clinical application in targeting human aging. In a recently published review, compounds including mTOR inhibitors, senolytics, metformin, acarbose, spermidine, NAD+ boosters, and lithium were deemed worthwhile to test in humans for their effects on healthspan (Partridge et al., 2020). Those compounds were selected given their reproducible pro-longevity effects in animal models, conserved mechanisms of action, significant amelioration of human biomarkers of aging, and potentially good safety profiles (Moskalev et al., 2016; Partridge et al., 2020). These factors therefore make up a primary set of criteria for evaluation of compounds for translatability to humans (Moskalev et al., 2016; Partridge et al., 2020).
Many longevity interventions have been described in model organisms, for example, the DrugAge database of aging interventions lists over 400 such compounds (Barardo et al., 2017; Janssens and Houtkooper, 2020). Yet, a major difficulty remains in gathering evidence that these compounds can influence the rate of biological aging in humans, other than by conducting an actual clinical trial. In this work, we advocate a parallel, non-mutually-exclusive route for selecting longevity interventions to test, starting from human population data and assessing rates of biological aging therein. We go on to illustrate how a variety of factors will influence a compound’s effectiveness as a longevity intervention, focusing on metformin as a case study. Finally, we describe how tracking the biological aging process can be used to test longevity interventions in the population, and is in fact a requisite for personalized longevity interventions.
Population Sourced Identification of Longevity Interventions
Recently, our team demonstrated that calculating the biological aging rate in a population can serve to identify factors associated with age deceleration (McIntyre et al., 2021). In this recent study, we used wearable device movement patterns to define a biological aging score, and checked for food components and drugs that were associated with age deceleration (McIntyre et al., 2021). We identified fiber, magnesium, and vitamin E as food components and the use of alpha blocker doxazosin as a pharmaceutical associated to younger biological age (McIntyre et al., 2021). Remarkably, we demonstrated that treating C. elegans with doxazosin could increase their aging health (measured by mobility and motility) in addition to their lifespan (McIntyre et al., 2021).
The approach we describe, consisting of a retrospective analysis on the rate of aging, and identifying factors associated with age deceleration, is a general approach that can be employed throughout many large human cohort datasets. While our study used wearable device accelerometer data to determine a biological aging score, biological age can also be assessed using a wide variety of other aging scores, including DNA methylation-based, transcriptomics-based, proteomics-based, and metabolomics-based molecular aging clocks (Jylhävä et al., 2017; Zhavoronkov and Mamoshina, 2019; Galkin et al., 2020; Robinson and Lau, 2020), in addition to other phenotypic parameters, e.g. blood biochemistry markers (Levine et al., 2018). Fortunately, these types of datasets are increasingly becoming available in large and thoroughly characterized human population studies. Following this, calculating biological age for each individual, and comparing this to their calendar age, can identify individuals with decelerated aging rates. Finding what factors, whether nutritional supplements, pharmaceuticals, food components, exercise routines, or genetics, that associate with younger age, can provide candidate interventions for follow up in prospective studies (Figure 1).
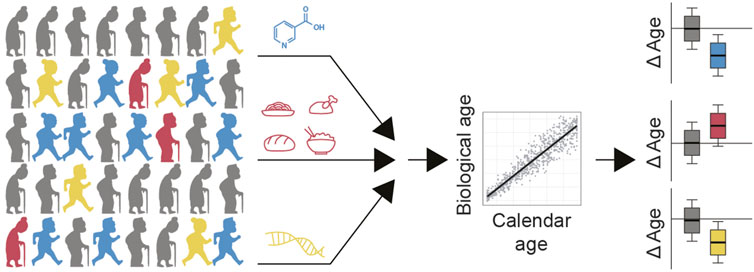
FIGURE 1. Strategy for identifying candidate longevity interventions in humans. Population level data would ideally contain information on treatments that people take, e.g. supplements and drugs, as well as their nutrition patterns and genetics. Calculating biological age of each participant and comparing this to their calendar age would identify which of these factors potentially slow aging (e.g. blue and yellow) compared to interventions which may accelerate aging (e.g. red). Factors that slow aging (blue, yellow) or accelerate aging (red), may comprise nutrients, drugs, exercise routines, other lifestyle factors and/or combinations thereof. These would become candidate therapies to test in a prospective cohort.
Studies, in addition to our own, have begun to apply such approaches and in doing so have identified candidate interventions with the potential to promote healthy aging in humans. For example, using an epigenetic aging clock, higher quality diets were related to decelerated aging (Kim et al., 2022). Furthermore, looking at telomere lengths and mortality data, it was found that Mediterranean diets may also decelerate aging (Crous-Bou et al., 2014). Using an epigenetic aging clock, it was also seen that certain mood stabilizers (including lithium carbonate, sodium valproate, and carbamazepine) are associated with decelerated aging (Okazaki et al., 2020). In line with this, studies in model organisms have demonstrated that this class of drugs can extend lifespan (Evason et al., 2008; Castillo-Quan et al., 2016; Nespital et al., 2021). Additionally, analysis of survival data alone can provide insights into factors positively affecting healthy longevity. For example, the antidiabetic drug metformin, discussed as a case-study below, was identified in such an analysis. Metformin users had lower mortality rates than non-diabetic age-matched controls, suggesting metformin was interveneing in the aging process (Bannister et al., 2014). Taken together, identification of decelerated aging factors through reanalysis of human datasets, whether drugs, diets, or otherwise, may offer a high likelihood of translatability to humans.
Population level data would ideally contain information on treatments that people take, e.g. supplements and drugs, as well as their nutrition patterns and genetics. Calculating biological age of each participant and comparing this to their calendar age would identify which of these factors potentially slow aging (e.g., blue and yellow) compared to interventions which may accelerate aging (e.g. red). Factors that slow aging (blue, yellow), or accelerate aging (red), may comprise nutrients, drugs, exercise routines, other lifestyle factors and/or combinations thereof. Those found to slow aging would become candidate therapies to test in a prospective cohort.
Considerations for Use of Longevity Compounds, a Case Study of Metformin
Metformin is a biguanide drug widely prescribed for the treatment of type 2 diabetes (T2D) worldwide (Adak et al., 2018). Metformin has been used in the clinic for over 6 decades and has an excellent safety record thus far. The medical history of metformin dates back to the 17th century, where the extracts of French lilac (Galega officinalis), which contains a metformin-like guanidine compound, was used as an herbal remedy to treat intense urination (Witters, 2001). This was the typical symptom of the disease that later came to be known as diabetes mellitus (Witters, 2001). In 1994, metformin was approved in the U.S. by the FDA to treat type 2 diabetes and is now the most prescribed anti-diabetic medication worldwide, taken by over 150 million people each year (He and Wondisford, 2015). Metformin is an effective glucose-lowering agent while also increasing peripheral sensitivity to insulin, thereby reducing the risk of all diabetes-related complications including death (Rena et al., 2017). Despite the long history and widespread use in the clinic, the modes of metformin’s action underlying these favorable effects are complex and remain elusive. Metformin is not metabolized in humans or animals and is eliminated unchanged by renal clearance (He and Wondisford, 2015). After oral administration, approximately 70% of metformin is absorbed from the small intestine and then delivered directly to the liver where it acts primarily to reduce hepatic gluconeogenesis (He and Wondisford, 2015). In addition to the liver, the intestines are also identified as an important target organ for metformin’s actions. Metformin can stimulate anaerobic glucose metabolism in enterocytes, suppress hepatic glucose production via liver-gut-brain crosstalk (Duca et al., 2015), and alter the gut microbiome in ways that contribute to antihyperglycemic effects, weight loss and inflammation suppression in individuals with T2D (Forslund et al., 2015; Wu et al., 2017).
Metformin is not only used in T2D management but also has gained significant attention as a potential geroprotective agent. Administration of metformin to various model organisms, including C. elegans (Onken and Driscoll, 2010) and mice (Martin-Montalvo et al., 2013), can mitigate aging, improve health and thereby increase lifespan through mechanisms beyond blood glucose regulation. The favorable effects of metformin on health and lifespan have been attributed to AMPK activation (Onken and Driscoll, 2010), mitohormesis (De Haes et al., 2014), the lysosomal pathway (Chen et al., 2017), and alterations of the microbiome, specifically by changing microbial folate and methionine metabolism (Cabreiro et al., 2013). In mice, metformin has been shown to extend lifespan and improve health in both short- and long-lived mouse models (Anisimov et al., 2005; Anisimov et al., 2008; Martin-Montalvo et al., 2013; Anisimov et al., 2015), yet the effects seen in long-lived mouse strains appear to be more modest. Some of these studies also suggest a greater health benefit of metformin in female mice (Anisimov et al., 2005; Anisimov et al., 2008; Anisimov et al., 2015). In spite of ample evidence that supports the protective effect of metformin against aging, a study conducted by the Aging Interventions Testing Program reported a non-significant effect of metformin on the lifespan of outbred mice (Strong et al., 2016). The authors suggested that the lack of benefit may be attributed to the doses used in the study (Strong et al., 2016). Given that the outbred mice have been deemed to be genetically heterogenous, these results also indicate that the lifespan response to metformin is subject to genetic variations. Therefore, individualized and precision approaches are needed to implement metformin in aging.
Nonetheless, a consensus exists that metformin targets and delays aging (Barzilai et al., 2016). Its administration in humans provides overall protection against age-related diseases, including but certainly not limited to diabetes, as well as, frailty in general. The geroprotective property of metformin can be evidenced by a wealth of retrospective observational studies, in which metformin use in diabetic patients is associated with reduced incidence of cancer (Currie et al., 2009; Libby et al., 2009; Landman et al., 2010; Lee et al., 2011; Monami et al., 2011; Ruiter et al., 2012; Tseng, 2012; Gandini et al., 2014), cardiovascular disease (Kooy et al., 2009; Wang et al., 2017), cognitive impairment (Cheng et al., 2014; Guo et al., 2014; Ng et al., 2014), and all-cause mortality (Turner, 1998; Bannister et al., 2014). Even when compared to age- and sex-matched non-diabetic groups, metformin treated diabetic patients had a slightly longer survival, despite the fact that diabetic patients were obese and had higher levels of morbidity at baseline (Bannister et al., 2014). A meta-analysis study has replicated these findings, reaching a similar conclusion on the protective effects of metformin against all-cause mortality and diseases of aging when compared to the general and non-diabetic population (Campbell et al., 2017). To explicitly answer the question concerning the anti-aging effects of metformin in non-diabetic individuals, the double-blinded, placebo-controlled multicenter trial Targeting Aging with Metformin (TAME) has been devised (Barzilai et al., 2016). TAME is the first so called ‘geroscience’ guided aging outcomes trial designed for metformin and plans to enroll ∼3,000 non-diabetic men and women aged 65–79 years for a 6-years trial period (Barzilai et al., 2016). In contrast to traditional FDA approved trials that only look for a single disease endpoint, TAME has a composite primary endpoint, consisting of stroke, heart failure, dementia, myocardial infarction, cancer, and death (Justice et al., 2018). This is in particular important to evaluate the efficacy of healthy aging drugs, considering the premise that slowing down aging will automatically postpone or eliminate multiple diseases of old age.
Metformin holds great potential to target aging and increase human healthspan. However, when it comes to the widespread use as an anti-aging therapy, some uncertainty still exists regarding whether metformin is effective and safe across the entire population for aging. One concern regards metformin responders versus non-responders, an issue that has been apparent among patients who take metformin for glycemic control (Florez, 2017). Genetic variation in the organic cation transporters that mediate the transport of metformin in body tissues are suggested to account for the differential responses to metformin (Yoon et al., 2013). In spite of this, understanding of the underlying mechanisms remains superficial. Another uncertainty concerns the interaction between metformin and lifestyle modification such as exercise. Although exercise and metformin independently improve metabolic health, and decrease the risk of age-related diseases, the combination may elicit unfavorable antagonistic effects on physiological function (Konopka et al., 2019). An additional consideration regards age of treatment. While metformin can be used safely and is the preferred initial therapy in many older adults with type 2 diabetes (Sue Kirkman et al., 2012), a study in C. elegans demonstrated that late-life treatment with metformin exacerbates aging-associated mitochondrial dysfunction and shortens lifespan (Espada et al., 2020). Furthermore, although metformin has been used with an excellent safety record for over 60 years, its long-term use is still associated with some potential side effects. Among them, gastrointestinal disturbances, such as diarrhea, nausea, vomiting, flatulence, and loss of appetite (Adak et al., 2018), occur most frequently. Very rarely, lactic acidosis may also occur upon metformin use, but the risk appears to be highly related to renal function (Connelly et al., 2017). Altogether, precision medicine approaches will be critical to implement metformin intervention as an anti-aging therapy in humans.
Testing Interventions in the Population
The case of metformin serves to illustrate that the efficacy of even the most promising longevity compounds will be susceptible to a variety of factors particular to the individual (Table 1). Furthermore, it remains unknown whether factors associated with decelerated aging will actually cause decelerated aging in humans. While conducting clinical trials such as the TAME study can directly address this question, this is an expensive (the TAME trial is estimated to cost 75 million USD (De Grey, 2019)) and time consuming option. While this may be the only way forward for drug interventions, other interventions, including supplements, diets, and exercises regimens, may allow for alternative forms of testing.
One possibility is to coordinate open-source public campaigns on such interventions in a systematic manner, to assess in the population whether a particular intervention is working (Figure 2). In theory, individuals may choose to take a safe nutraceutical, exercise regimen, or nutritional diet, which is suspected to slow the aging process. After a pre-defined period of administration, individuals assess their biological age, compared to their chronological age, to determine if the drug has a statistical effect at decelerating aging in the population tested (Figure 2). The most accessible biological aging score may be one based on accelerometers, as we have recently developed (McIntyre et al., 2021), since most individuals possess one in their mobile phones, smart watches, or fitness trackers.
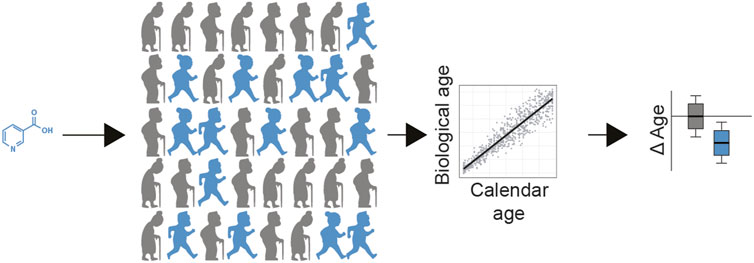
FIGURE 2. Population level testing of longevity interventions. In this example, a safe nutraceutical can be given to participants (blue = receiving treatment, grey = receiving placebo). After a predefined period of administration, individuals assess their biological age, compared to their chronological age, calculating deltaAge. This can be used to determine if the drug has a statistical effect at decelerating aging at the population level. In addition, personal data of the participants (e.g., sex, genetics, lifestyle, age, genetics, microbiome) can be used to help decipher which sub populations benefit the most from the treatment (not shown).
The advantage of the ‘public testing’ scenario described here, is that not only would individuals contribute to testing whether a particular intervention is having statistical effects at a population level, but they would also determine whether they themselves are ‘responders’ or ‘nonresponders’ to the intervention in question. Careful noting of relevant parameters at the onset of the study, including lifestyle factors, sex, age, intervention dose/frequency, and linking with commercially available ‘omics’ technologies such as genomics and microbiome measures, would eventually allow for the disentangling of factors involving efficacy. Ultimately, this would contribute to developing personalized approaches to treating aging for the future.
Conclusion
Here we 1) describe how biological age prediction can identify factors associated with decelerated aging in large cohort studies, 2) highlight that not all longevity interventions will equally benefit each user, using metformin as a case study, and 3) propose that large-scale controlled public testing of longevity interventions is a theoretical possibility, potentially even a necessity, towards implementing longevity interventions for the public. It is important to note that metformin is not alone in its potential drawbacks as a longevity intervention. For example, dietary restriction, one of the most widely accepted longevity interventions, has either beneficial or detrimental effects on mouse lifespan depending on genetic background (Liao et al., 2010). Furthermore, antioxidants, another popular intervention often praised for healthy aging benefits, can also prevent the benefits of physical exercise in humans (Ristow et al., 2009). Of important consideration too are sex differences. For example, it has been noted that lifespan extension in mice due to (genetically) reduced levels of insulin-like growth factor 1 (IGF-1) or mechanistic target of rapamycin (mTOR) signaling typically favors females, while pharmacological treatments (rather than genetic modifications) generally favors males (Austad and Bartke, 2015). Clearly, as longevity interventions reach the public, it will be of paramount importance to closely track the aging process. Moving forward, we advocate that 1) not all interventions will be beneficial for each individual and 2) it is imperative that individuals track their own aging rates to assess healthy aging interventions.
Data Availability Statement
The original contributions presented in the study are included in the article/Supplementary Material, further inquiries can be directed to the corresponding author.
Author Contributions
GJ conceived of the perspective piece. YL, RM and GJ wrote the manuscript.
Funding
GJ is supported by a VENI grant from ZonMw, Talent Development and Innovation grants from the AGEM institute, and support from the Longevity Impetus Grant from Norn Group.
Conflict of Interest
The authors declare that the research was conducted in the absence of any commercial or financial relationships that could be construed as a potential conflict of interest.
Publisher’s Note
All claims expressed in this article are solely those of the authors and do not necessarily represent those of their affiliated organizations, or those of the publisher, the editors and the reviewers. Any product that may be evaluated in this article, or claim that may be made by its manufacturer, is not guaranteed or endorsed by the publisher.
References
Adak, T., Samadi, A., Ünal, A. Z., and Sabuncuoğlu, S. (2018). A Reappraisal on Metformin. Regul. Toxicol. Pharmacol. 92, 324–332. doi:10.1016/j.yrtph.2017.12.023
Anisimov, V. N., Berstein, L. M., Egormin, P. A., Piskunova, T. S., Popovich, I. G., Zabezhinski, M. A., et al. (2005). Effect of Metformin on Life Span and on the Development of Spontaneous Mammary Tumors in HER-2/neu Transgenic Mice. Exp. Gerontol. 40, 685–693. doi:10.1016/j.exger.2005.07.007
Anisimov, V. N., Berstein, L. M., Egormin, P. A., Piskunova, T. S., Popovich, I. G., Zabezhinski, M. A., et al. (2008). Metformin Slows Down Aging and Extends Life Span of Female SHR Mice. Cell Cycle 7, 2769–2773. doi:10.4161/cc.7.17.6625
Anisimov, V. N., Popovich, I. G., Zabezhinski, M. A., Egormin, P. A., Yurova, M. N., Semenchenko, A. V., et al. (2015). Sex Differences in Aging, Life Span and Spontaneous Tumorigenesis in 129/Sv Mice Neonatally Exposed to Metformin. Cell Cycle 14, 46–55. doi:10.4161/15384101.2014.973308
Austad, S. N., and Bartke, A. (2015). Sex Differences in Longevity and in Responses to Anti-aging Interventions: A Mini-Review. Gerontology 62, 40–46. doi:10.1159/000381472
Bannister, C. A., Holden, S. E., Jenkins-Jones, S., Morgan, C. L., Halcox, J. P., Schernthaner, G., et al. (2014). Can People with Type 2 Diabetes Live Longer Than Those without? A Comparison of Mortality in People Initiated with Metformin or Sulphonylurea Monotherapy and Matched, Non-diabetic Controls. Diabetes Obes. Metab. 16, 1165–1173. doi:10.1111/dom.12354
Barardo, D., Thornton, D., Thoppil, H., Walsh, M., Sharifi, S., Ferreira, S., et al. (2017). The DrugAge Database of Aging-Related Drugs. Aging Cell 16, 594–597. doi:10.1111/acel.12585
Barzilai, N., Crandall, J. P., Kritchevsky, S. B., and Espeland, M. A. (2016). Metformin as a Tool to Target Aging. Cel Metab. 23, 1060–1065. doi:10.1016/j.cmet.2016.05.011
Cabreiro, F., Au, C., Leung, K.-Y., Vergara-Irigaray, N., Cochemé, H. M., Noori, T., et al. (2013). Metformin Retards Aging in C. elegans by Altering Microbial Folate and Methionine Metabolism. Cell 153, 228–239. doi:10.1016/j.cell.2013.02.035
Campbell, J. M., Bellman, S. M., Stephenson, M. D., and Lisy., K. (2017). Metformin Reduces All-Cause Mortality and Diseases of Ageing Independent of its Effect on Diabetes Control: A Systematic Review and Meta-Analysis. Ageing Res. Rev. 40, 31–44. doi:10.1016/j.arr.2017.08.003
Castillo-Quan, J. I., Li, L., Kinghorn, K. J., Ivanov, D. K., Tain, L. S., Slack, C., et al. (2016). Lithium Promotes Longevity through GSK3/NRF2-dependent Hormesis. Cel Rep. 15, 638–650. doi:10.1016/j.celrep.2016.03.041
Chen, J., Ou, Y., Li, Y., Hu, S., Shao, L. W., and Liu, Y. (2017). Metformin Extends C. elegans Lifespan through Lysosomal Pathway. Elife 6. doi:10.7554/eLife.31268
Cheng, C., Lin, C.-H., Tsai, Y.-W., Tsai, C.-J., Chou, P.-H., and Lan, T.-H. (2014). Type 2 Diabetes and Antidiabetic Medications in Relation to Dementia Diagnosis. Journals Gerontol. - Ser. A. Biol. Sci. Med. Sci. 69, 1299–1305. doi:10.1093/gerona/glu073
Connelly, P. J., Lonergan, M., Soto-Pedre, E., Donnelly, L., Zhou, K., and Pearson, E. R. (2017). Acute Kidney Injury, Plasma Lactate Concentrations and Lactic Acidosis in Metformin Users: A GoDarts Study. Diabetes Obes. Metab. 19, 1579–1586. doi:10.1111/dom.12978
Crous-Bou, M., Fung, T. T., Prescott, J., Julin, B., Du, M., Sun, Q., et al. (2014). Mediterranean Diet and Telomere Length in Nurses' Health Study: Population Based Cohort Study. BMJ 349, g6674. doi:10.1136/bmj.g6674
Currie, C. J., Poole, C. D., and Gale, E. A. M. (2009). The Influence of Glucose-Lowering Therapies on Cancer Risk in Type 2 Diabetes. Diabetologia 52, 1766–1777. doi:10.1007/s00125-009-1440-6
De Grey, A. D. N. J. (2019). TAME: A Genuinely Good Use of 75 Million Dollars. Rejuvenation Res. 22, 375–376. doi:10.1089/rej.2019.2274
De Haes, W., Frooninckx, L., Van Assche, R., Smolders, A., Depuydt, G., Billen, J., et al. (2014). Metformin Promotes Lifespan through Mitohormesis via the Peroxiredoxin PRDX-2. Proc. Natl. Acad. Sci. U.S.A. 111. doi:10.1073/pnas.1321776111
Duca, F. A., Côté, C. D., Rasmussen, B. A., Zadeh-Tahmasebi, M., Rutter, G. A., Filippi, B. M., et al. (2015). Metformin Activates a Duodenal Ampk-dependent Pathway to Lower Hepatic Glucose Production in Rats. Nat. Med. 21, 506–511. doi:10.1038/nm.3787
Espada, L., Dakhovnik, A., Chaudhari, P., Martirosyan, A., Miek, L., Poliezhaieva, T., et al. (2020). Loss of Metabolic Plasticity Underlies Metformin Toxicity in Aged Caenorhabditis elegans. Nat. Metab. 2, 1316–1331. doi:10.1038/s42255-020-00307-1
Evason, K., Collins, J. J., Huang, C., Hughes, S., and Kornfeld, K. (2008). Valproic Acid Extends Caenorhabditis elegans Lifespan. Aging Cell 7, 305–317. doi:10.1111/j.1474-9726.2008.00375.x
Florez, J. C. (2017). The Pharmacogenetics of Metformin. Diabetologia 60, 1648–1655. doi:10.1007/s00125-017-4335-y
Forslund, K., au, fnm., Hildebrand, F., Nielsen, T., Falony, G., Le Chatelier, E., et al. (2015). Disentangling Type 2 Diabetes and Metformin Treatment Signatures in the Human Gut Microbiota. Nature 528, 262–266. doi:10.1038/nature15766
Galkin, F., Mamoshina, P., Aliper, A., de Magalhães, J. P., Gladyshev, V. N., and Zhavoronkov, A. (2020). Biohorology and Biomarkers of Aging: Current State-Of-The-Art, Challenges and Opportunities. Ageing Res. Rev. 60, 101050. doi:10.1016/j.arr.2020.101050
Gandini, S., Puntoni, M., Heckman-Stoddard, B. M., Dunn, B. K., Ford, L., DeCensi, A., et al. (2014). Metformin and Cancer Risk and Mortality: A Systematic Review and Meta-Analysis Taking into Account Biases and Confounders. Cancer Prev. Res. 7, 867–885. doi:10.1158/1940-6207.CAPR-13-0424
Guo, M., Mi, J., Jiang, Q.-M., Xu, J.-M., Tang, Y.-Y., Tian, G., et al. (2014). Metformin May Produce Antidepressant Effects through Improvement of Cognitive Function Among Depressed Patients with Diabetes Mellitus. Clin. Exp. Pharmacol. Physiol., a–n. doi:10.1111/1440-1681.12265
He, L., and Wondisford, F. E. (2015). Metformin Action: Concentrations Matter. Cel Metab. 21, 159–162. doi:10.1016/j.cmet.2015.01.003
Janssens, G. E., and Houtkooper, R. H. (2020). Identification of Longevity Compounds with Minimized Probabilities of Side Effects. Biogerontology 21, 709–719. doi:10.1007/s10522-020-09887-7
Justice, J. N., Niedernhofer, L., Robbins, P. D., Aroda, V. R., Espeland, M. A., Kritchevsky, S. B., et al. (2018). Development of Clinical Trials to Extend Healthy Lifespan. Cardiovasc. Endocrinol. Metab. 7, 80–83. doi:10.1097/XCE.0000000000000159
Jylhävä, J., Pedersen, N. L., and Hägg, S. (2017). Biological Age Predictors. EBioMedicine 21, 29–36.
Khaltourina, D., Matveyev, Y., Alekseev, A., Cortese, F., and Ioviţă, A. (2020). Aging Fits the Disease Criteria of the International Classification of Diseases. Mech. Ageing Develop. 189, 111230. doi:10.1016/j.mad.2020.111230
Kim, Y., Huan, T., Joehanes, R., McKeown, N. M., Horvath, S., Levy, D., et al. (2022). Higher Diet Quality Relates to Decelerated Epigenetic Aging. Am. J. Clin. Nutr. 115, 163–170. doi:10.1093/ajcn/nqab201
Konopka, A. R., Laurin, J. L., Schoenberg, H. M., Reid, J. J., Castor, W. M., Wolff, C. A., et al. (2019). Metformin Inhibits Mitochondrial Adaptations to Aerobic Exercise Training in Older Adults. Aging Cell 18. doi:10.1111/acel.12880
Kooy, A., de Jager, J., Lehert, P., Bets, D., Wulffelé, M. G., Donker, A. J. M., et al. (2009). Long-term Effects of Metformin on Metabolism and Microvascular and Macrovascular Disease in Patients with Type 2 Diabetes Mellitus. Arch. Intern. Med. 169, 616. doi:10.1001/archinternmed.2009.20
Landman, G. W. D., Kleefstra, N., van Hateren, K. J. J., Groenier, K. H., Gans, R. O. B., and Bilo, H. J. G. (2010). Metformin Associated with Lower Cancer Mortality in Type 2 Diabetes. Diabetes Care 33, 322–326. doi:10.2337/dc09-1380
Lee, M.-S., Hsu, C.-C., Wahlqvist, M. L., Tsai, H.-N., Chang, Y.-H., and Huang, Y.-C. (2011). Type 2 Diabetes Increases and Metformin Reduces Total, Colorectal, Liver and Pancreatic Cancer Incidences in Taiwanese: A Representative Population Prospective Cohort Study of 800,000 Individuals. BMC Cancer 11. doi:10.1186/1471-2407-11-20
Levine, M. E., Lu, A. T., Quach, A., Chen, B. H., Assimes, T. L., Bandinelli, S., et al. (2018). An Epigenetic Biomarker of Aging for Lifespan and Healthspan. Aging 10, 573–591. doi:10.18632/aging.101414
Liao, C.-Y., Rikke, B. A., Johnson, T. E., Diaz, V., and Nelson, J. F. (2010). Genetic Variation in the Murine Lifespan Response to Dietary Restriction: From Life Extension to Life Shortening. Aging Cell 9, 92–95. doi:10.1111/j.1474-9726.2009.00533.x
Libby, G., Donnelly, L. A., Donnan, P. T., Alessi, D. R., Morris, A. D., and Evans, J. M. M. (2009). New Users of Metformin Are at Low Risk of Incident Cancer. Diabetes Care 32, 1620–1625. doi:10.2337/dc08-2175
López-Otín, C., Blasco, M. A., Partridge, L., Serrano, M., and Kroemer, G. (2013). The Hallmarks of Aging. Cell 153, 1194–1217. doi:10.1016/j.cell.2013.05.039
Martin-Montalvo, A., Mercken, E. M., Mitchell, S. J., Palacios, H. H., Mote, P. L., Scheibye-Knudsen, M., et al. (2013). Metformin Improves Healthspan and Lifespan in Mice. Nat. Commun. 4. doi:10.1038/ncomms3192
McIntyre, R. L., Rahman, M., Vanapalli, S. A., Houtkooper, R. H., and Janssens, G. E. (2021). Biological Age Prediction from Wearable Device Movement Data Identifies Nutritional and Pharmacological Interventions for Healthy Aging. Front. Aging 2. doi:10.3389/fragi.2021.708680
Monami, M., Colombi, C., Balzi, D., Dicembrini, I., Giannini, S., Melani, C., et al. (2011). Metformin and Cancer Occurrence in Insulin-Treated Type 2 Diabetic Patients. Diabetes Care 34, 129–131. doi:10.2337/dc10-1287
Moskalev, A., Chernyagina, E., Tsvetkov, V., Fedintsev, A., Shaposhnikov, M., Krut'ko, V., et al. (2016). Developing Criteria for Evaluation of Geroprotectors as a Key Stage toward Translation to the Clinic. Aging Cell 15, 407–415. doi:10.1111/acel.12463
Nespital, T., Neuhaus, B., Mesaros, A., Pahl, A., and Partridge, L. (2021). Lithium Can Mildly Increase Health during Ageing but Not Lifespan in Mice. Aging Cell 20. doi:10.1111/acel.13479
Ng, T. P., Feng, L., Yap, K. B., Lee, T. S., Tan, C. H., and Winblad, B. (2014). Long-term Metformin Usage and Cognitive Function Among Older Adults with Diabetes. Jad 41, 61–68. doi:10.3233/JAD-131901
Okazaki, S., Numata, S., Otsuka, I., Horai, T., Kinoshita, M., Sora, I., et al. (2020). Decelerated Epigenetic Aging Associated with Mood Stabilizers in the Blood of Patients with Bipolar Disorder. Transl. Psychiatry 10. doi:10.1038/s41398-020-0813-y
Onken, B., and Driscoll, M. (2010). Metformin Induces a Dietary Restriction-like State and the Oxidative Stress Response to Extend C. elegans Healthspan via AMPK, LKB1, and SKN-1. PLoS One 5, e8758. doi:10.1371/journal.pone.0008758
Partridge, L., Fuentealba, M., and Kennedy, B. K. (2020). The Quest to Slow Ageing through Drug Discovery. Nat. Rev. Drug Discov. 19, 513–532. doi:10.1038/s41573-020-0067-7
Rena, G., Hardie, D. G., and Pearson, E. R. (2017). The Mechanisms of Action of Metformin. Diabetologia 60, 1577–1585. doi:10.1007/s00125-017-4342-z
Ristow, M., Zarse, K., Oberbach, A., Klöting, N., Birringer, M., Kiehntopf, M., et al. (2009). Antioxidants Prevent Health-Promoting Effects of Physical Exercise in Humans. Proc. Natl. Acad. Sci. U.S.A. 106, 8665–8670. doi:10.1073/pnas.0903485106
Robinson, O., and Lau, C.-H. E. (2020). Measuring Biological Age Using Metabolomics. Aging. doi:10.18632/aging.104216
Ruiter, R., Visser, L. E., van Herk-Sukel, M. P. P., Coebergh, J.-W. W., Haak, H. R., Geelhoed-Duijvestijn, P. H., et al. (2012). Lower Risk of Cancer in Patients on Metformin in Comparison with Those on Sulfonylurea Derivatives. Diabetes Care 35, 119–124. doi:10.2337/dc11-0857
Strong, R., Miller, R. A., Antebi, A., Astle, C. M., Bogue, M., Denzel, M. S., et al. (2016). Longer Lifespan in Male Mice Treated with a Weakly Estrogenic Agonist, an Antioxidant, an α‐glucosidase Inhibitor or a Nrf2‐inducer. Aging Cell 15, 872–884. doi:10.1111/acel.12496
Sue Kirkman, M., Briscoe, V. J., Clark, N., Florez, H., Haas, L. B., Halter, J. B., et al. (2012). Diabetes in Older Adults: A Consensus Report. J. Am. Geriatr. Soc. 60, 2342–2356. doi:10.1111/jgs.12035
The Lancet Diabetes & Endocrinology (2018). Opening the Door to Treating Ageing as a Disease. Lancet Diabetes Endocrinol. doi:10.1016/S2213-8587(18)30214-6
Tseng, C.-H. (2012). Diabetes, Metformin Use, and colon Cancer: a Population-Based Cohort Study in Taiwan. Eur. J. Endocrinol. 167, 409–416. doi:10.1530/EJE-12-0369
Turner, R. (1998). Effect of Intensive Blood-Glucose Control with Metformin on Complications in Overweight Patients with Type 2 Diabetes (UKPDS 34). Lancet. doi:10.1016/S0140-6736(98)07037-8
Wang, C.-P., Lorenzo, C., Habib, S. L., Jo, B., and Espinoza, S. E. (2017). Differential Effects of Metformin on Age Related Comorbidities in Older Men with Type 2 Diabetes. J. Diabetes its Complications 31, 679–686. doi:10.1016/j.jdiacomp.2017.01.013
Witters, L. A. (2001). The Blooming of the French Lilac. J. Clin. Invest. 108, 1105–1107. doi:10.1172/JCI14178
Wu, H., Esteve, E., Tremaroli, V., Khan, M. T., Caesar, R., Mannerås-Holm, L., et al. (2017). Metformin Alters the Gut Microbiome of Individuals with Treatment-Naive Type 2 Diabetes, Contributing to the Therapeutic Effects of the Drug. Nat. Med. 23, 850–858. doi:10.1038/nm.4345
Yoon, H., Cho, H.-Y., Yoo, H.-D., Kim, S.-M., and Lee, Y.-B. (2013). Influences of Organic Cation Transporter Polymorphisms on the Population Pharmacokinetics of Metformin in Healthy Subjects. AAPS J. 15, 571–580. doi:10.1208/s12248-013-9460-z
Keywords: aging, public, interventions, geroprotectors, biological age, chronological age
Citation: Liu YJ, McIntyre RL and Janssens GE (2022) Considerations Regarding Public Use of Longevity Interventions. Front. Aging 3:903049. doi: 10.3389/fragi.2022.903049
Received: 23 March 2022; Accepted: 05 April 2022;
Published: 25 April 2022.
Edited by:
Morten Scheibye-Knudsen, University of Copenhagen, DenmarkReviewed by:
Devin Wahl, Colorado State University, United StatesCopyright © 2022 Liu, McIntyre and Janssens. This is an open-access article distributed under the terms of the Creative Commons Attribution License (CC BY). The use, distribution or reproduction in other forums is permitted, provided the original author(s) and the copyright owner(s) are credited and that the original publication in this journal is cited, in accordance with accepted academic practice. No use, distribution or reproduction is permitted which does not comply with these terms.
*Correspondence: Georges E. Janssens, g.e.janssens@amsterdamumc.nl