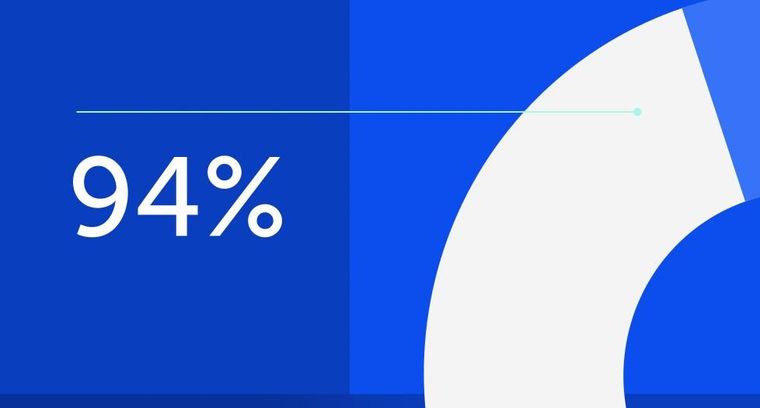
94% of researchers rate our articles as excellent or good
Learn more about the work of our research integrity team to safeguard the quality of each article we publish.
Find out more
EDITORIAL article
Front. Radiol., 12 February 2024
Sec. Neuroradiology
Volume 4 - 2024 | https://doi.org/10.3389/fradi.2024.1366704
This article is part of the Research TopicQuantitative Neuroradiology MethodsView all 6 articles
Editorial on the Research Topic
Quantitative neuroradiology methods
Neuroradiology has been and continues to be one of the fastest advancing imaging subspecialties, often driven by breakthroughs in artificial intelligence (AI) and pulse sequence advancements. These converging technologies hold immense promise for minimizing artifacts, leading to markedly improved diagnostic accuracy, as well as improved treatment decisions and prognostication.
Despite the increasing speed of MRI, motion remains a problem, particularly in younger subjects. Children's head motion during magnetic resonance imaging (MRI) poses a significant challenge, impacting image quality and diagnostic reliability. Analyzing motion patterns in pediatric patients with and without general anesthesia reveals differences, emphasizing the necessity of tailored approaches to mitigate artifacts and optimize image acquisition, and underscoring the importance of refining imaging protocols for enhanced clinical outcomes. The article by Eichhorn et al. presents relevant insights on motion artefacts and clinical implications.
In the very youngest patients, these motion mitigation techniques allow new avenues to be explored. Among these is the imaging of neonatal patients. Despite challenges in its application, diffusion kurtosis imaging (DKI) offers a unique window into the intricate microstructural changes within the neonatal spinal cord. Proposing a novel semi-automated pipeline for neonatal spinal cord imaging, Trò et al. illuminate DKI's superior sensitivity over traditional diffusion imaging methods, emphasizing its potential in elucidating early developmental anomalies and brain-spinal cord interconnections critical for long-term outcomes, and minimization of societal burden.
As patients mature, different types of disorders become prevalent. In the realm of blunt cerebrovascular injuries (BCVIs), emergent imaging modalities have transformed the landscape of detection and characterization. Evolving techniques, such as advanced magnetic resonance imaging (MRI) sequences and transcranial Doppler, have markedly improved the diagnosis and understanding of BCVIs, heralding a new era of precision imaging for trauma-related neurovascular injuries for these increasingly prevalent injuries (Bounajem et al.).
One of the first applications of AI, has been in image segmentation. AI has traditionally been limited by the size, and diversity of the databases, the networks are adapted from. Advances in weakly-supervised segmentation methodologies leveraging convolutional neural networks (CNNs) presents a potential ethical solution to the challenge of limited annotated datasets in medical imaging. The proposed pipeline by Rajapaksa and Khalvati utilizes relevance maps and localized perturbations, demonstrating enhanced accuracy in glioma brain tumor segmentation and showcases the potential of ethical AI solutions in neuroimaging tasks.
There are advances in MRI sequences, image processing, and data interpretation. The latter is where AI is most beneficial. AI, understandably is much in the news nowadays. The integration of AI in neuroradiology promises unparalleled insights into brain pathophysiology, refining treatment models, and augmenting diagnostic algorithms. Yet, this autonomous utilization raises critical ethical challenges. Issues of informed consent scope, data privacy risks, database biases, and the ethical responsibility accompanying AI-driven decisions cast a spotlight on the ethical landscape of neuroscience research and healthcare practices. The ethics of AI deserve far more attention in the medical literature, and we hope this issue will help guide that conversation (Khosravi and Schweitzer).
We hope with this editorial and Research Topic to synthesize the ethical dilemmas and technical innovations in neuroimaging advances and emphasize the necessity of establishing cohesive ethical standards. A balanced approach is advocated, one that harnesses the potential of advanced technologies while safeguarding patient privacy, ensuring informed consent, and considering database biases. By doing so, we prioritize ethical responsibilities in the pursuit to enhance the field of neuroimaging.
MB: Writing – original draft. MS: Writing – review & editing. TS: Writing – review & editing. YC: Writing – review & editing. ŞE: Writing – review & editing.
The authors declare that the research was conducted in the absence of any commercial or financial relationships that could be construed as a potential conflict of interest.
All claims expressed in this article are solely those of the authors and do not necessarily represent those of their affiliated organizations, or those of the publisher, the editors and the reviewers. Any product that may be evaluated in this article, or claim that may be made by its manufacturer, is not guaranteed or endorsed by the publisher.
Keywords: motion artefact, DKI-diffusion kurtosis imaging, BCVI-blunt cerebrovascular injury, CNN—convolutional neural network, ethics—clinical
Citation: Barzegar M, Schweitzer M, So TY, Chen Y and Ertürk ŞM (2024) Editorial: Quantitative neuroradiology methods. Front. Radiol. 4:1366704. doi: 10.3389/fradi.2024.1366704
Received: 7 January 2024; Accepted: 31 January 2024;
Published: 12 February 2024.
Edited and Reviewed by: Sotirios Bisdas, University College London, United Kingdom
© 2024 Barzegar, Schweitzer, So, Chen and Ertürk. This is an open-access article distributed under the terms of the Creative Commons Attribution License (CC BY). The use, distribution or reproduction in other forums is permitted, provided the original author(s) and the copyright owner(s) are credited and that the original publication in this journal is cited, in accordance with accepted academic practice. No use, distribution or reproduction is permitted which does not comply with these terms.
*Correspondence: Mojtaba Barzegar dHVtcy5yYWRpb2xvZ3lAZ21haWwuY29t
Disclaimer: All claims expressed in this article are solely those of the authors and do not necessarily represent those of their affiliated organizations, or those of the publisher, the editors and the reviewers. Any product that may be evaluated in this article or claim that may be made by its manufacturer is not guaranteed or endorsed by the publisher.
Research integrity at Frontiers
Learn more about the work of our research integrity team to safeguard the quality of each article we publish.