- 1Bernard and Irene Schwartz Center for Biomedical Imaging, Department of Radiology, New York University Grossman School of Medicine, New York, NY, United States
- 2Center for Advanced Imaging Innovation and Research (CAI2R), Department of Radiology, New York University Grossman School of Medicine, New York, NY, United States
- 3Department of Radiology, New York University Grossman School of Medicine, New York, NY, United States
- 4Department of Orthopedic Surgery, New York University Grossman School of Medicine, New York, NY, United States
Introduction: Femoroacetabular Impingement (FAI) is a hip pathology characterized by impingement of the femoral head-neck junction against the acetabular rim, due to abnormalities in bone morphology. FAI is normally diagnosed by manual evaluation of morphologic features on magnetic resonance imaging (MRI). In this study, we assess, for the first time, the feasibility of using radiomics to detect FAI by automatically extracting quantitative features from images.
Material and methods: 17 patients diagnosed with monolateral FAI underwent pre-surgical MR imaging, including a 3D Dixon sequence of the pelvis. An expert radiologist drew regions of interest on the water-only Dixon images outlining femur and acetabulum in both impingement (IJ) and healthy joints (HJ). 182 radiomic features were extracted for each hip. The dataset numerosity was increased by 60 times with an ad-hoc data augmentation tool. Features were subdivided by type and region in 24 subsets. For each, a univariate ANOVA F-value analysis was applied to find the 5 features most correlated with IJ based on p-value, for a total of 48 subsets. For each subset, a K-nearest neighbor model was trained to differentiate between IJ and HJ using the values of the radiomic features in the subset as input. The training was repeated 100 times, randomly subdividing the data with 75%/25% training/testing.
Results: The texture-based gray level features yielded the highest prediction max accuracy (0.972) with the smallest subset of features. This suggests that the gray image values are more homogeneously distributed in the HJ in comparison to IJ, which could be due to stress-related inflammation resulting from impingement.
Conclusions: We showed that radiomics can automatically distinguish IJ from HJ using water-only Dixon MRI. To our knowledge, this is the first application of radiomics for FAI diagnosis. We reported an accuracy greater than 97%, which is higher than the 90% accuracy for detecting FAI reported for standard diagnostic tests (90%). Our proposed radiomic analysis could be combined with methods for automated joint segmentation to rapidly identify patients with FAI, avoiding time-consuming radiological measurements of bone morphology.
1. Introduction
Femoroacetabular impingement (FAI) is a common cause of hip pain in young adults with an estimated incidence of 54.4 per 1,00,000 person-years (1). FAI is characterized by impingement of the femoral head-neck junction against the acetabular rim during hip joint motion due to morphologic abnormalities of the proximal femur and acetabulum (2–4). There are two distinct pathoanatomic types of FAI, although mixed types are commonly detected at arthroscopy (5). Cam FAI is caused by decreased offset and asphericity of the femoral head-neck junction, while Pincer FAI is due to focal or generalized acetabular over-coverage (3, 4). Although the natural history of FAI is unknown, early diagnosis and appropriate surgical treatment of the condition has been shown to reduce symptoms and improve function, at least in the short-term (6).
Imaging plays an important role in the diagnosis of FAI as distinguishing the disorder from other causes of hip pain is challenging using clinical history and physical examination (7). Quantitative measures of bone shape on radiographs including the alpha angle for Cam impingement and the center edge angle for Pincer impingement are typically used for the initial diagnosis of FAI (3, 4). However, radiographic measures of bone shape may be influenced by technical factors during image acquisition (8–10), and three-dimensional (3D) bone morphology may not be reliably assessed on two-dimensional (2D) radiographs (11, 12). Thus, computed tomography (CT) is commonly used for pre-operative planning to provide the most accurate assessment of 3D bone shape (3, 4). While CT provides high spatial resolution and excellent tissue contrast for evaluating bone, it may result in potentially harmful ionizing radiation exposure to the pelvis (13).
Recent literature (3, 4, 14, 15) focused the attention of FAI diagnosis on 3D MR imaging, which can enable radiologists to detect the typical osseous pathological condition in FAI with accuracy, sensitivity and specificity around 90% (3, 4). These analyses are usually based on metrics arising from the shape of the hip structures or from range of motion simulations of the hip joint (6, 7, 15–17).
Radiomics has gained increasing popularity over the recent years as a diagnostic image analysis method to predict and characterize a wide variety of pathologic conditions (18–22). Radiomics involves the high-throughput extraction of quantitative features from medical imaging studies such as CT and MRI (19–21). The assumption of radiomics is that image features quantify crucial information regarding pathologic conditions through intra-region heterogeneity (19). Several studies have used radiomics to evaluate musculoskeletal diseases of soft tissue and bone (23). However, to our knowledge no previous work has investigated the use of radiomics to diagnose FAI (24). Thus, our study was performed to investigate the feasibility of using radiomics on 3D-MRI to distinguish between hips with and without symptomatic impingement in patients with FAI.
2. Material and methods
2.1. Image data
The study group consisted of 17 patients (13 females and four males with mean age of 37.1 ± 5.7 years) with unilateral FAI diagnosed at hip arthroscopy who underwent an MRI examination of the hip prior to surgery. One patient was diagnosed with isolated Cam FAI, while the remaining 16 patients were diagnosed with mixed Cam and Pincer FAI at arthroscopy. Three patients underwent a follow-up MRI examination one year after surgery. All MRI examinations were performed on a 3T scanner (Skyra, Siemens Healthineers, Erlangen, Germany) and included an axial dual echo T1-weighted 3D fast low angle shot (FLASH) sequence of the pelvis with Dixon fat-water separation and the following imaging parameters: repetition time = 10 ms, echo time = 2.4 ms and 3.7 ms, field of view = 32 cm, acquisition matrix = 320 × 320, and slice thickness = 1 mm.
For each MRI dataset, a fellowship-trained musculoskeletal radiologist with 20 years of clinical experience delineated regions of interest (ROIs) for the femur and acetabulum on each water-only image slice of the 3D-FLASH sequence using an open-source software viewer (ITK-SNAP v3.8.0; www.itksnap.org) (25).The ROIs were drawn using the automatic 3D seed based segmentation tool available in ITK-SNAP and then manually fine-tuned slice by slice in the three main visualization axes: axial, coronal, and sagittal.
Left and right hip femur and acetabulum ROIs for the 17 patients were subdivided into healthy joints (HJs) and joints with impingement (IJs) according to the surgical reports. The IJs of the three patients with follow-up MRI examinations were excluded as the femur and acetabulum were surgically remodeled during arthroscopy. This resulted in a total of 37 segmented femoral and acetabular ROIs, which included 17 HJs and 17 IJs from the pre-operative MRI examinations and three HJs from the post-operative MRI examinations. Figure 1 shows representative examples of segmented femoral and acetabular ROIs from HJs and IJs.
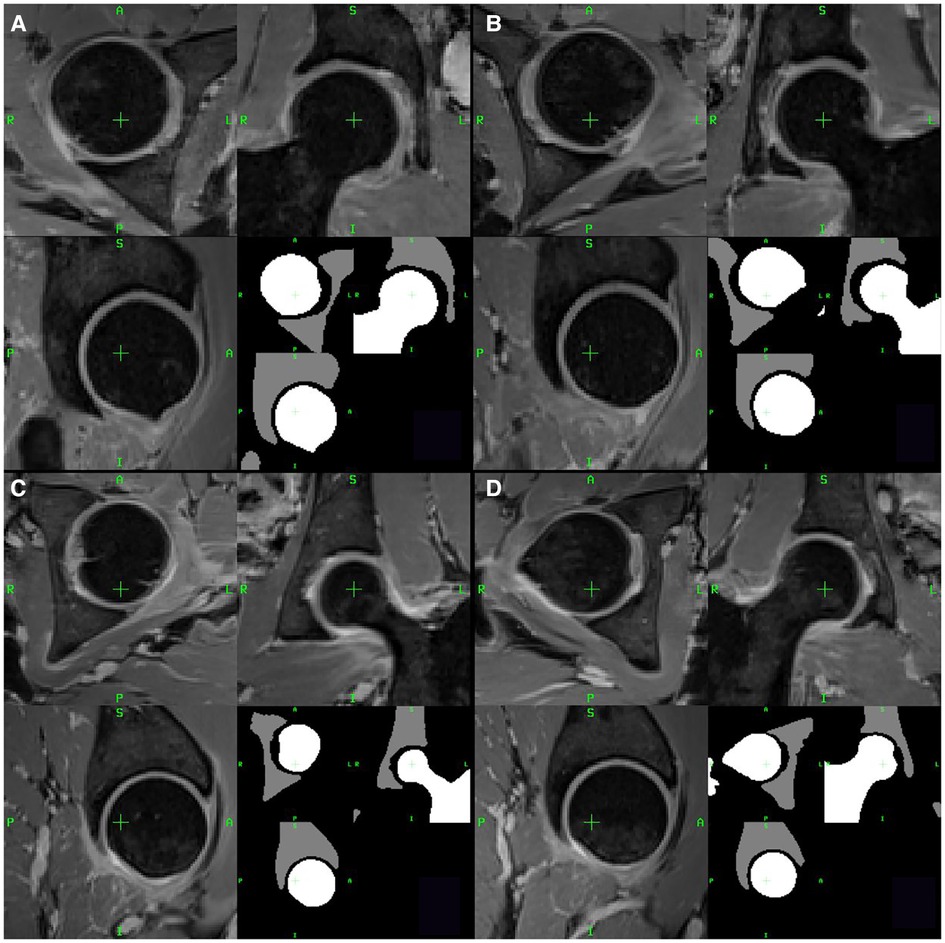
Figure 1. Two examples of healthy joints (A,B) and two examples of joints with impingement (C,D) from two representative patients with FAI. For each example, axial, coronal and sagittal views are shown. The lower right quadrant of each panel shows the segmented femur (white) and acetabulum (gray) ROIs.
2.2. Data augmentation
To increase sample size, a data augmentation method was used that provided rototranslated couples of images and ROIs that were sampled at different resolutions. Directly applying rototranslation and subsequently changing the resolution of the image could result in erroneously labeled pixels in the transformed ROIs due to the interpolation process after the rototranslation, or pixels affected by partial volume averaging. The developed data augmentation technique instead transformed every label map ROI in a collection of meshes, one per value of the map, and then transformed them along with the corresponding image. The transformations were applied in the non-gridded space of the meshes and then rasterized in the desired space. The output coordinate system could be also customized by setting origin, direction, resolution, and size of the output grid space. Data augmentation was implemented in ITK4 (26) and a containerized version of the software has been made freely available at https://hub.docker.com/r/erosmontin/daug.
As described in the workflow diagram in Figure 2, the 37 labeled and segmented femur and acetabulum ROIs were augmented by a factor of 60 for a total of 2,220 datasets. The 2,220 augmented datasets were obtained by creating randomly uniformed rototranslation between −5 and 5° in the first two Euler’s angles (left/right and anterior/posterior) and between −15 and 15° in the third Euler’s angle (inferior/superior), with random translations ranging between 5 and −5 mm. The resulting images were re-sampled using two output coordinate systems: a uniform grid of 1 mm side and a size of 120 voxels per dimension and an anisotropic grid of resolution 0.4 × 0.4 × 1.2 mm and matrix size of 320 × 320 × 120. In order to maintain the anatomical shape of the hips as realistic as possible, no scaling was applied to the datasets.
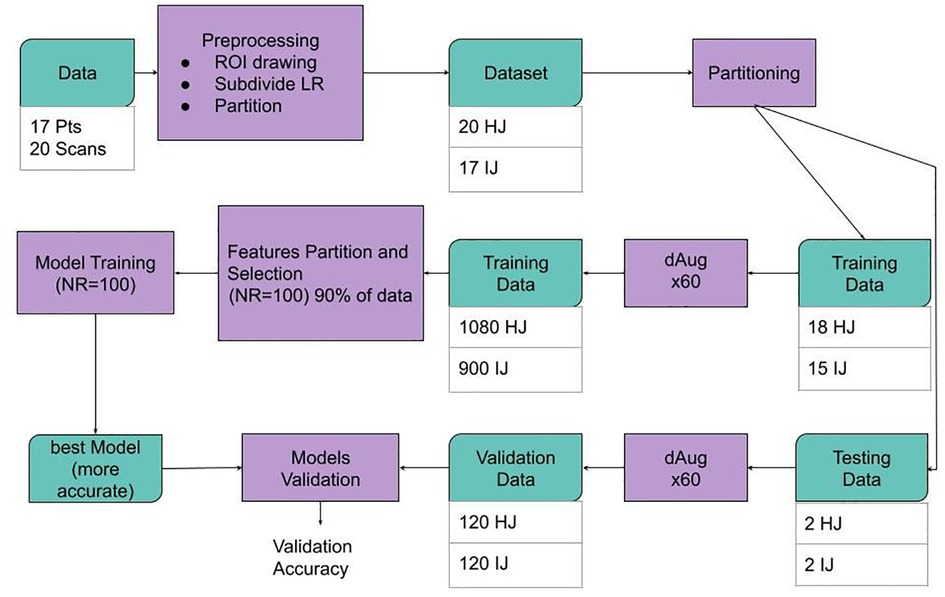
Figure 2. Schematic representation of the data workflow. Data was pre-processed, and images and regions of interest (ROIs) from a total of 3 datasets [18 healthy joints (HJs) and 15 joints with impingement (IJs)] were used for the model training phase, while four hold-out testing datasets (two healthy joints and two joints with impingement) were used for model evaluation. The size of the training and validation datasets was augmented by a factor of 60 using a data augmentation (dAug) method. 48 subsets of features were created from randomly selected 75% of the training data. For each subset of features, a KNN machine learning process was repeated 100 times and the most accurate model was selected for each case. Finally, the performance of the best model for each subset was assessed on the hold-out testing dataset.
2.3. Radiomic features extraction
For each couple consisting of an image and one associated femur or acetabulum ROI in the augmented dataset, 182 features were extracted using a previously described radiomic feature extractor (19), including 91 features for the femur and 91 features for the acetabulum. The 91 features could be classified into three main classes: (i) intensity and histogram based first order statistics (FOS) features, (ii) texture features, and (iii) shape and size features. A complete list of the 91 features extracted from the augmented datasets is summarized in Table 1.
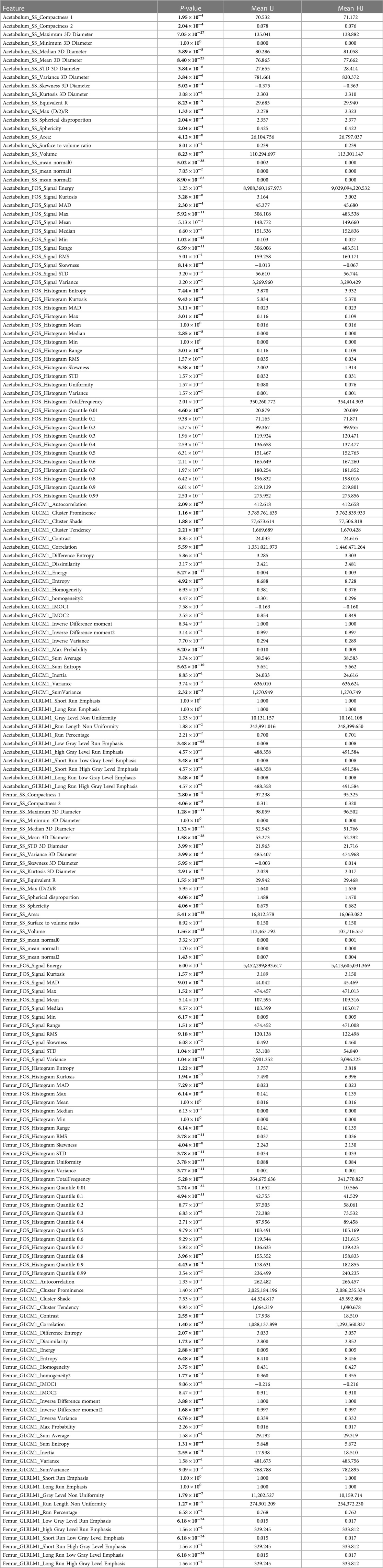
Table 1. List of radiomic features. For each feature, the p-value of the Wilcoxon rank-sum test is reported along with the mean values for the feature distribution for the health joints (HJs) and joints with impingement (IJs). Gray cells are associated with a statistically significant difference between the distribution of the feature values in the HJs and IJs. SS, shape and size; 3D, three-dimensional; GLCM, gray level co-occurrence matrices; GLRLM, gray level run length matrices; FOS, first-order-statistic; STD, standard deviation; MAD, mean absolute deviation; RMS, root mean square; IMOC, information measure of correlation; R, ray; D, diameter.
For each femur and acetabulum ROI, the 12 signal FOS features were extracted from the water-only 3D-FLASH grayscale image values in the ROIs. The following 25 histogram FOS features described the complexity of the shape of the histogram distribution of the grayscale values in the ROIs. The histogram settings for all feature classes were set to 32 bins with a marginal scale of 0.5 and minimum and maximum equal to 0 and 200, respectively. These first two subsets of features belonged to the FOS features. Texture features were based on the gray level co-occurrence matrices (GLCM) and gray level run length matrices (GLRLM) (27), calculated in 26 directions, one for every neighbor of a voxel in a 3D space with a radius set to one pixel. For each GLCM and GLRLM feature, the extracted features were averaged over the 26 directions to get 23 GLCM features and 11 GLRLM features per ROI. Lastly, 20 shape and size features were extracted from the ROI mesh of the femur and acetabulum separately.
The resulting 182 features were subdivided in 24 subsets with a variable number of features, divided by feature type and femur or acetabulum ROI. For each subset, a univariate ANOVA F-value analysis was applied to find the five most pertinent features based on p-values among those included. This yielded 24 additional F-contrast subsets with five features each, for a total of 48 subsets. The feature selection was repeated 100 times using 90% of the dataset and used the five most frequent features selected by the F-contrast rank.
2.4. Machine learning model training and evaluation
A K-nearest neighbor machine learning model was used to identify the features most pertinent to differentiate IJs from HJs. From the available data, 240 augmented datasets consisting of two HJs and two IJs were randomly selected as a hold-out testing dataset for model evaluation. The remaining 1,980 augmented datasets consisting of 900 datasets from 15 IJs and 1,080 datasets from 18 HJs were used for model training and validation. For each of the 48 feature subsets, a K-nearest neighbor model (k = 3) was trained and validated using 100-fold cross-validation with a 75/25 data split. During this selection process, the augmented images of one patient belonged only to one group either training or testing. The inputs of each model were the z-scored values of the radiomic features in the corresponding subset, and the outputs were the labels HJ and IJ. The trained model with the highest prediction accuracy was selected as the final model for the particular subset of features and was evaluated against the hold-out testing dataset to assess its performance in differentiating IJs from HJs. The process resulted in one trained model for each of the 48 subsets of features which was then evaluated against the testing dataset.
3. Results
Table 1 shows the mean values of each feature distribution in the femur and acetabulum for HJs and IJs and the corresponding p-values for the Wilcoxon rank sum tests comparing differences in values between groups. The results show that 116 features out of the total 182 features could differentiate IJs from HJs (p < 0.05, hereinafter indicated by *). Out of these 116 features, 45 features (39%) belonged to the intensity-based FOS group [16 signal (14%) and 29 histogram (25%)], 33 (28%) to the shape and size group, and 38 (33%) to the textural features group [28 GLCM (24%) and 10 GLRLM (9%)]. Among the 45 statistically significant FOS features, 24 features were from the femur (8 signal and 16 histograms), 21 from the acetabulum (8 signal and 13 histogram), and 20 were from both the femur and acetabulum (8 signal and 12 histogram).
Table 2 shows the diagnostic performance of the machine learning models for differentiating IJs from HJs using the hold-out testing dataset. For each subset of features, the accuracy, specificity, sensitivity, and AUC of the models were reported along with the number of features in the training subset. The table had 48 entries, 24 reporting the performance of the model trained using all the features in a specific subset and 24 entries reporting the performance of the model trained using only the five most pertinent features in the specific subset with the lowest F-contrast p-values. The top performing models analyzed all GLCM texture features from the femur and acetabulum followed by the models analyzing all intensity-based FOS features from the femur and acetabulum, all shape and size features from the femur and acetabulum, and all intensity-based histogram FOS features of the femur.
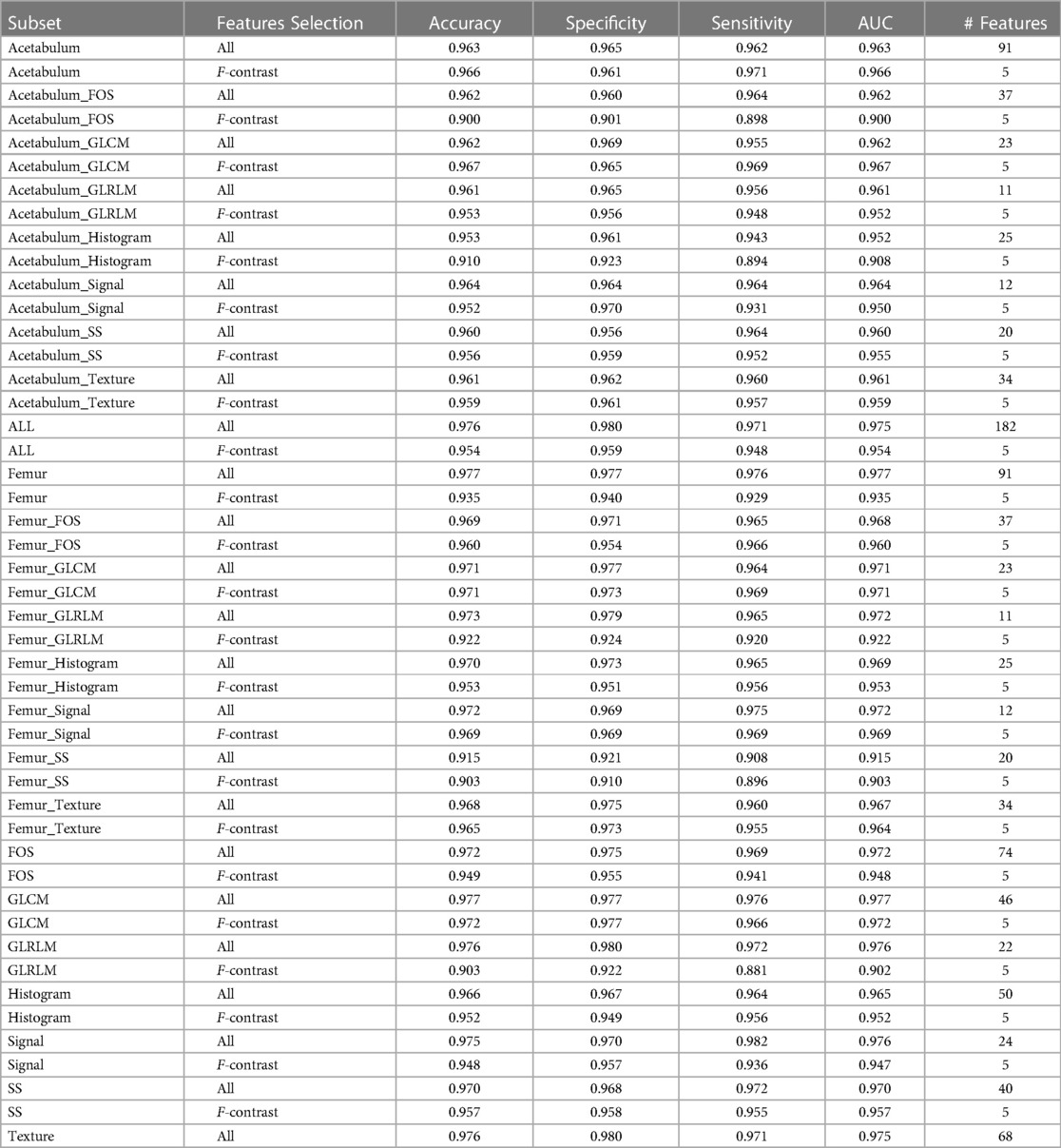
Table 2. Diagnostic performance of the machine learning models for differentiating IJs from HJs using each subset of features on the hold-out testing dataset. The complete list of features contained in each subsets can be seen in Supplementary Table S1.
The model trained with all GLCM texture features from the femur and acetabulum had the highest diagnostic performance for differentiating IJs from HJs with 0.977 accuracy, 0.977 specificity, 0.976 sensitivity, and 0.977 AUC. Three of the five features of this model with the lowest F-contrast p-values were related to GLCM of the femur (GLCM Max Probability*, GLCM1 Energy*, and GLCM1 Correlation*), while two were related to GLCM of the acetabulum (GLCM Correlation*, GLCM Inverse Variance). The F-contrast model using the five most pertinent features had 0.972 accuracy, 0.977 specificity, 0.966 sensitivity, and 0.972 AUC.
The model trained with all FOS features from the femur and acetabulum had 0.972 accuracy, 0.975 specificity, 0.969 sensitivity, and 0.972 AUC for differentiating IJs from HJs. The five most pertinent features of this model with the lowest F-contrast p-value were all related to the histogram of the femur (Histogram Quantile 0.99, Histogram Quantile 0.6, Histogram Uniformity*, Histogram Quantile 0.4, Histogram RMS*). The F-contrast model using these five features had 0.949 accuracy, 0.955 specificity, 0.941 sensitivity, and 0.948 AUC.
The model trained with all shape and size features from the femur and acetabulum had 0.970 accuracy, 0.968 specificity, 0.972 sensitivity, and 0.970 AUC for differentiating IJs from HJs. The five most pertinent features of this model with the lowest F-contrast p-values were all related to the shape and size of the femur (SS Area*, SS Mean 3D Diameter*, SS Median 3D Diameter*, Equivalent R*). The F-contrast model using these five features had 0.957 accuracy, 0.958 specificity, 0.955 sensitivity, and 0.957 AUC. As shown in Table 1, among the 40 shape and size features of the femur and acetabulum, 33 (83%) were significantly different between HJs and IJs.
The models trained with all intensity-based FOS histogram features from the femur had 0.972 accuracy, 0.969 specificity, 0.975 sensitivity, and 0.972 AUC for differentiating IJs from HJs. The five most pertinent features of this model with the lowest F-contrast p-values included the Femur Histogram Quantile 0.1*, Femur Histogram Total Frequency*, Femur Histogram Median, Femur Histogram Range*, and Femur Histogram Quantile 0.3.) The F-contrast model using these five features had 0.953 accuracy, 0.951 specificity, 0.956 sensitivity, and 0.953 AUC.
The model trained with the femur histogram features yielded an accuracy of 0.97 (0.97, 0.973, 0.965, 0.969). For the subset with the five most relevant features (F-contrast), these values became 0.953, 0.951, 0.956, and 0.953. In particular in the F-contrast subset included the femur Histogram Quantile 0.1*, femur Histogram Total frequency*, femur Histogram Median, femur Histogram Range*, and femur Histogram Quantile 0.3 features.
Figure 3 shows the diagnostic performance of the machine learning models for differentiating IJs from HJs during the 100-fold cross-validation training phase. Models trained with femur intensity-based FOS and GLCM texture features all had accuracies above 0.95, while most models trained with acetabular intensity-based FOS and GLCM texture features had accuracies under 0.95. The differences were more notable for the F-contrast models trained using the five most pertinent features with the lowest F-contrast p-values, where three of the four models with the highest accuracy used features from the femur. As shown in Table 2, differences in model performance were also confirmed using the hold-out testing dataset, where the model trained with 91 features from the femur had higher diagnostic performance (0.977 accuracy, 0.977 sensitivity, 0.976 specificity, and 0.977 AUC) when compared to models trained with all 182 features from the femur and acetabulum (0.976 accuracy, 0.980 specificity, 0.971 sensitivity, and 0.975 AUC) and models trained with 91 features from the acetabulum (0.963 accuracy, 0.965 specificity, 0.962 sensitivity, and 0.963 AUC). In particular, the model trained with the femur had higher accuracy compared to the ones trained with the acetabulum ones (Rank-sum test p < 0.05) even in the F-contrasted subset (bottom subplot).
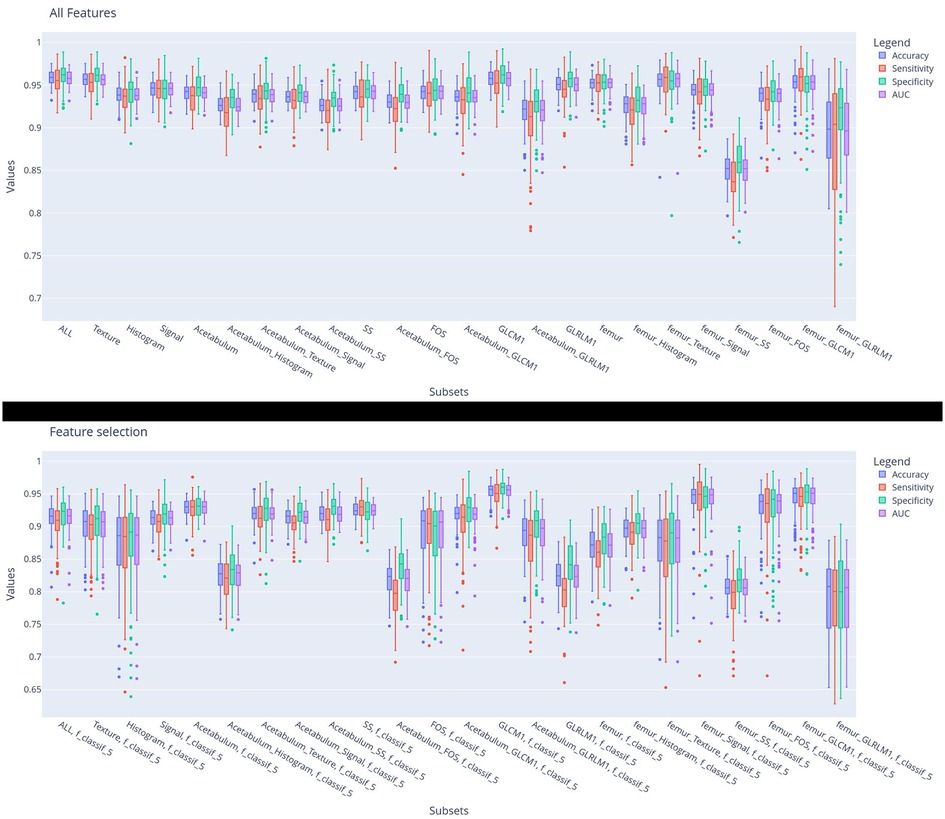
Figure 3. Diagnostic performance of the machine learning models for differentiating IJs from HJs using each subset of features during model training. The histogram bars represent the distribution of the prediction metrics during the 100-fold cross-validation.
Figure 4 shows the five most pertinent features with the lowest F-contrast p-values for each feature class, while Figure 5 shows z-scored values of each feature for the femur and acetabulum. For the femur, the five most pertinent features were three textural features (GLRLM Long Run Low Gray Level Emphasis*, GLRLM Short Run Low Gray Level Emphasis*, and GLRLM Low Gray Level Run Emphasis*) and two shape and size features (SS Area*, SS Volume*). The values of the three GLRLM features of the femur and the area and volume of the femur were higher in IJs than HJs. The importance of the three GLRLM features of the femur were further confirmed by the results in Table 2; Supplementary Table S1, which showed that the five most pertinent features with the lowest F-contrast p-values in the model trained with all 182 features from the femur and acetabulum included GLRLM Long Run Low Gray Level Emphasis*, GLRLM Short Run Low Gray Level Emphasis*, and GLRLM Low Gray Level Run Emphasis* of the femur.
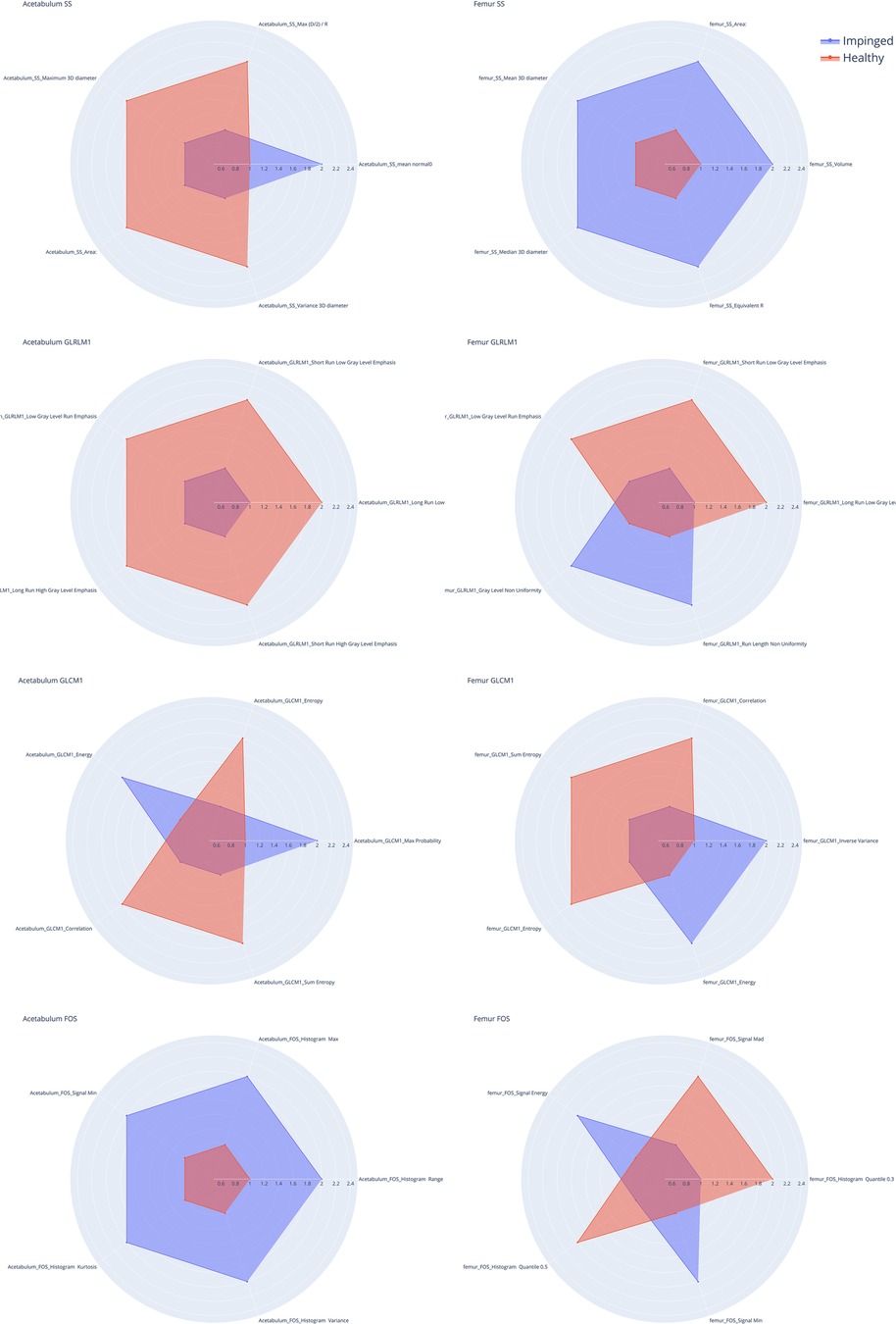
Figure 4. Radar charts for shape and size (SS), gray level Co-occurrence matrix (GLCM), gray level Run matrix (GLRLM) and intensity based first order statistic (FOS) of the acetabulum (left) and the femur (right). The five spokes represent the five most informative features in the group (F-contrast), the radial length of each spoke is proportional to the magnitude of the value of the associated feature. The spokes are normalized so that the difference between hip joints with impingement (IJ, blue line) and the healthy ones (HJ, orange line) is emphasized. For example, in the SS Acetabulum radar plot it is possible to see how four features values are higher for the healthy joints compared to the injured ones (first plot on the left) while the mean normal0 features values are higher in the injured acetabulum than in the healthy ones.
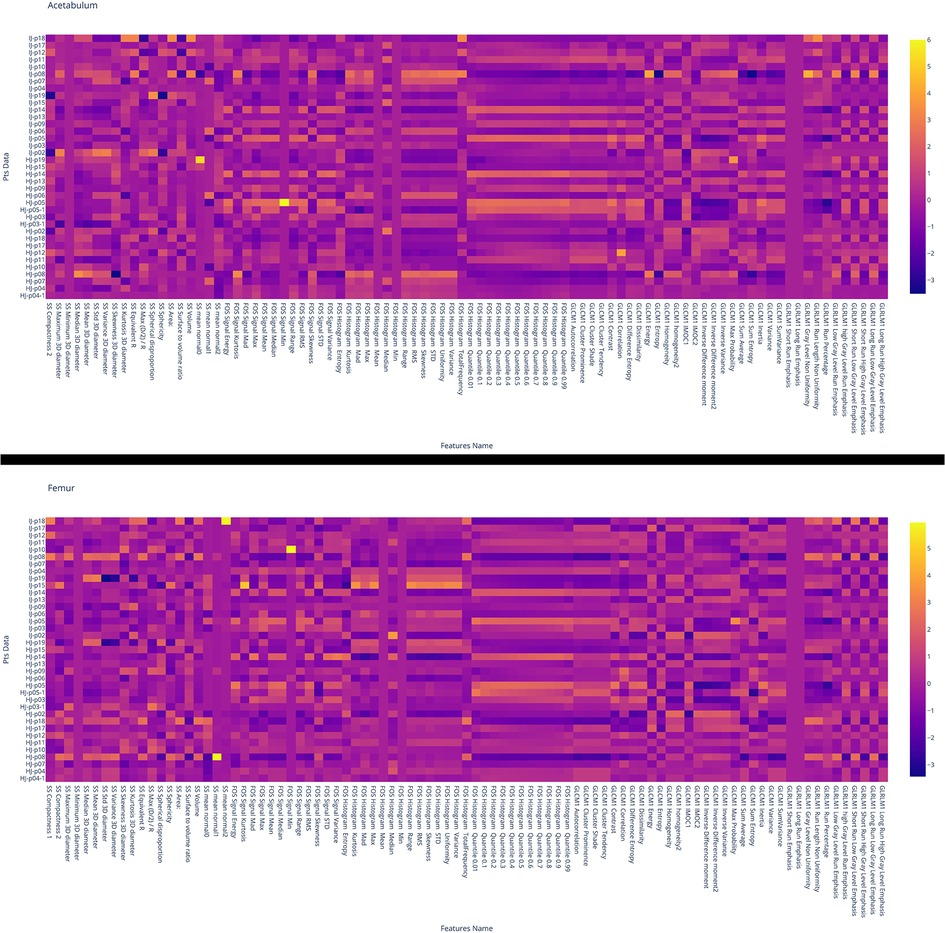
Figure 5. Heat map of the values of the features for the acetabulum (top) and femur (bottom). Each row corresponds to one patient and each column corresponds to one normalized (z-score) radiomic feature. HJ or IJ before the patient number refers to a healthy joint and joint with impingement, respectively. From the heat map it is possible to see how both femur and acetabulum GLCM Correlation feature is of higher value for HJ than IJ.
4. Discussion and conclusions
Our study was performed to investigate the feasibility of using radiomics of 3D-MRI to distinguish between hips with and without symptomatic impingement in patients with FAI. Our results showed some of the highest diagnostic performance for differentiating IJs from HJs using imaging studies reported in the literature. The top performing radiomic model in our study analyzed all GLCM texture features from the femur and acetabulum on 3D-MRI, followed by models analyzing all intensity-based FOS features from the femur and acetabulum, all shape and size features from the femur and acetabulum, and all histogram FOS features of the femur.
FAI is characterized by impingement of the femoral head-neck junction against the acetabular rim due to morphologic abnormalities of the proximal femur and acetabulum (2–4). In our study, the radiomic model trained with all shape and size features from the femur and acetabulum on 3D-MRI yielded the highest performance with 0.970 accuracy, 0.968 specificity, 0.972 sensitivity, and 0.970 AUC for differentiating IJs from HJs. The model had higher diagnostic performance for detecting FAI than currently used quantitative measures of bone shape on radiographs, CT, and MRI. For example, studies have shown that the alpha angle has sensitivities between 0.360 and 0.920 and specificities between 0.620 and 0.950 for detecting cam impingement (28–32), while the center edge angle has sensitivities between 0.820 and 0.842 and specificities between 0.390 and 1.00 for detecting pincer impingement (32, 33). Furthermore, a high prevalence of abnormal quantitative measures of proximal femur and acetabulum shape have been described in healthy subjects with no clinical evidence of FAI, which raises questions regarding the high specificities of these metrics reported in some studies (34).
Although FAI is a condition caused by morphological abnormalities of bone, our study found that the radiomic model analyzing all GLCM texture features of the femur and acetabulum on 3D-MRI had the highest diagnostic performance for differentiating IJs from HJs. GLCM features are calculated over the co-occurrence matrix, which highlights how spread out the image pixel signal intensity values are around a given pixel in a square matrix. If all the pixels in the ROI had the same grayscale value (i.e., pixel signal intensity values were homogeneous), the co-occurrence matrix would have only one bin containing that particular co-occurrence image intensity value set to 1 and all the other bins set to 0. The presence of multiple peaks in the co-occurrence implies heterogeneity in image pixel signal intensity. If the imaged tissue is mildly heterogeneous, the values in the co-occurrence matrix are less parse and more close to each other, whereas if the pixel values are completely random, the co-occurrence matrix will have sparser peaks (27). In our study, the model trained with all GLCM texture features from the femur and acetabulum had 0.977 accuracy, 0.977 specificity, 0.976 sensitivity, and 0.977 AUC for distinguishing between IJs and HJs. The five most pertinent features of this model were GLCM Max Probability, GLCM1 Energy, and GLCM1 Correlation of the femur and GLCM Correlation and GLCM Inverse Variance of the acetabulum. All these features were higher in the IJ than the HJ, indicating that FAI leads to a more heterogeneous distribution of image pixel signal intensity values. The femur and acetabulum primarily consist of trabecular and cortical bone, hematopoietic cells, and fat with little if any water content. As the water-only 3D-FLASH images used for radiomic analysis in our study reflect the presence of water within each image pixel, the greater heterogeneity of pixel signal intensity values in the IJs likely results in increased water content in some pixels. This may be due to subtle and non-uniform bone inflammation due to impingement of the femoral head-neck junction against the acetabular rim, which cannot even be detected in the image by the human eye.
Our study has shown that it is possible to create machine learning models to differentiate IJ from HJ with a high diagnostic performance using only a small subset of radiomic features on 3D-MRI. For each feature class, there was a relatively small decrease in model performance when using the five most pertinent features with the lowest F-contrast p-values compared to the full model analyzing all features from the femur and acetabulum. For example, the F-contrast model for GLCM texture features had 0.972 accuracy, 0.977 specificity, 0.966 sensitivity, and 0.972 AUC for differentiating IJs from HJs compared to 0.977 accuracy, 0.977 specificity, 0.976 sensitivity, and 0.977 for the full model. Radiomic models analyzing a smaller number of features are better suited for widespread use in clinical practice as they are quicker and easier to create and are likely more reproducible across different MRI scanners, sequences, and imaging parameters.
Our study had several limitations. One limitation was the small number of subjects used for model training and evaluation. The problem of model training with a small number of subjects was overcome by using a novel data augmentation framework to create pseudo-plausible image data that magnified the pattern in the features space between the IJs and HJs. Furthermore, our models were created using a simple K-nearest neighbor method to focus attention on the information content of the image features rather than the accuracy of the models per se. However, the relative simplicity of our machine learning approach may improve the reproducibility of the models and indirectly determines the lower bound of model performance as sensitivity and specificity could likely be improved with use of more sophisticated machine learning methods and larger training datasets. A final limitation was that our study could not assess model generalizability as model training and evaluation was performed using homogenous image datasets acquired on the same MRI scanner with the same sequence and imaging parameters.
In conclusion, our study has documented the feasibility of using radiomics of 3D-MRI to distinguish between hips with and without symptomatic impingement in patients with FAI. Our radiomic models analyzed intensity-based FOS features, shape and size features, and texture features and had some of the highest diagnostic performance for differentiating IJs from HJs using imaging studies reported in the literature. Additional studies are needed to investigate the use of more sophisticated machine learning approaches and larger training datasets to optimize model performance and to evaluate model generalizability using more heterogeneous patient populations imaged with different MRI scanners and imaging protocols.
Data availability statement
The raw data supporting the conclusions of this article will be made available by the authors, without undue reservation.
Ethics statement
The studies involving human participants were reviewed and approved by Institutional Review Board (IRB). The patients/participants provided their written informed consent to participate in this study.
Author contributions
EM and RL conceived the study and wrote the manuscript. EM developed the radiomic pipeline and the data augmentation software. RK drew the regions of interest on the MR images, TY recruited the patients and provided the diagnostic information. All authors contributed to the article and approved the submitted version.
Funding
This work was supported by NIH R01 AR070297 and performed under the Rubric of the Center for Advanced Imaging Innovation and Research (CAI2R, www.cai2r.net), an NIBIB National Center for Biomedical Imaging and Bioengineering (NIH P41 EB017183).
Conflict of interest
The authors declare that the research was conducted in the absence of any commercial or financial relationships that could be construed as a potential conflict of interest.
Publisher's note
All claims expressed in this article are solely those of the authors and do not necessarily represent those of their affiliated organizations, or those of the publisher, the editors and the reviewers. Any product that may be evaluated in this article, or claim that may be made by its manufacturer, is not guaranteed or endorsed by the publisher.
Supplementary material
The Supplementary Material for this article can be found online at: https://www.frontiersin.org/articles/10.3389/fradi.2023.1151258/full#supplementary-material.
References
1. Hale RF, Melugin HP, Zhou J, LaPrade MD, Bernard C, Leland D, et al. Incidence of femoroacetabular impingement and surgical management trends over time. Am J Sports Med. (2021) 49(1):35–41. doi: 10.1177/0363546520970914
2. Griffin DR, Dickenson EJ, O'Donnell J, Agricola R, Awan T, Beck M, et al. The warwick agreement on femoroacetabular impingement syndrome (FAI syndrome): an international consensus statement. Br J Sports Med. (2016) 50(19):1169–76. doi: 10.1136/bjsports-2016-096743
3. Albers CE, Wambeek N, Hanke MS, Schmaranzer F, Prosser GH, Yates PJ. Imaging of femoroacetabular impingement-current concepts. J Hip Preserv Surg. (2016) 3(4):245–61. doi: 10.1093/jhps/hnw035
4. Schmaranzer F, Kheterpal AB, Bredella MA. Best practices: hip femoroacetabular impingement. AJR Am J Roentgenol. (2021) 216(3):585–98. doi: 10.2214/AJR.20.22783
5. Beck M, Kalhor M, Leunig M, Ganz R. Hip morphology influences the pattern of damage to the acetabular cartilage: femoroacetabular impingement as a cause of early osteoarthritis of the hip. J Bone Joint Surg Br. (2005) 87(7):1012–8. doi: 10.1302/0301-620X.87B7.15203
6. Clohisy JC, St John LC, Schutz AL. Surgical treatment of femoroacetabular impingement: a systematic review of the literature. Clin Orthop Relat Res. (2010) 468(2):555–64. doi: 10.1007/s11999-009-1138-6
7. Reiman MP, Goode AP, Cook CE, Holmich P, Thorborg K. Diagnostic accuracy of clinical tests for the diagnosis of hip femoroacetabular impingement/labral tear: a systematic review with meta-analysis. Br J Sports Med. (2015) 49(12):811. doi: 10.1136/bjsports-2014-094302
8. Tannast M, Fritsch S, Zheng G, Siebenrock KA, Steppacher SD. Which radiographic hip parameters do not have to be corrected for pelvic rotation and tilt? Clin Orthop Relat Res. (2015) 473(4):1255–66. doi: 10.1007/s11999-014-3936-8
9. Tannast M, Zheng G, Anderegg C, Burckhardt K, Langlotz F, Ganz R, et al. Tilt and rotation correction of acetabular version on pelvic radiographs. Clin Orthop Relat Res. (2005) 438:182–90. doi: 10.1097/01.blo.0000167669.26068.c5
10. Siebenrock KA, Kalbermatten DF, Ganz R. Effect of pelvic tilt on acetabular retroversion: a study of pelves from cadavers. Clin Orthop Relat Res. (2003) 407:241–8. doi: 10.1097/00003086-200302000-00033
11. Harris MD, Kapron AL, Peters CL, Anderson AE. Correlations between the alpha angle and femoral head asphericity: implications and recommendations for the diagnosis of cam femoroacetabular impingement. Eur J Radiol. (2014) 83(5):788–96. doi: 10.1016/j.ejrad.2014.02.005
12. Rhee C, Le Francois T, Byrd JWT, Glazebrook M, Wong I. Radiographic diagnosis of pincer-type femoroacetabular impingement: a systematic review. Orthop J Sports Med. (2017) 5(5):2325967117708307. doi: 10.1177/2325967117708307
13. Wylie JD, Jenkins PA, Beckmann JT, Peters CL, Aoki SK, Maak TG. Computed tomography scans in patients with young adult hip pain carry a lifetime risk of malignancy. Arthroscopy. (2018) 34(1):155–163.e153. doi: 10.1016/j.arthro.2017.08.235
14. Saied AM, Redant C, El-Batouty M, El-Lakkany MR, El-Adl WA, Anthonissen J, et al. Accuracy of magnetic resonance studies in the detection of chondral and labral lesions in femoroacetabular impingement: systematic review and meta-analysis. BMC Musculoskelet Disord. (2017) 18(1):83. doi: 10.1186/s12891-017-1443-2
15. Samim M, Eftekhary N, Vigdorchik JM, Elbuluk A, Davidovitch R, Youm T, et al. 3D-MRI Versus 3D-CT in the evaluation of osseous anatomy in femoroacetabular impingement using dixon 3D FLASH sequence. Skeletal Radiol. (2019) 48(3):429–36. doi: 10.1007/s00256-018-3049-7
16. Yan K, Xi Y, Sasiponganan C, Zerr J, Wells JE, Chhabra A. Does 3DMR provide equivalent information as 3DCT for the pre-operative evaluation of adult hip pain conditions of femoroacetabular impingement and hip dysplasia? Br J Radiol. (2018) 91(1092):20180474. doi: 10.1259/bjr.20180474
17. Lerch TD, Degonda C, Schmaranzer F, Todorski I, Cullmann-Bastian J, Zheng G, et al. Patient-Specific 3-D magnetic resonance imaging-based dynamic simulation of hip impingement and range of motion can replace 3-D computed tomography-based simulation for patients with femoroacetabular impingement: implications for planning open hip preservation surgery and hip arthroscopy. Am J Sports Med. (2019) 47(12):2966–77. doi: 10.1177/0363546519869681
18. Lambin P, Rios-Velazquez E, Leijenaar R, Carvalho S, van Stiphout RG, Granton P, et al. Radiomics: extracting more information from medical images using advanced feature analysis. Eur J Cancer. (2012) 48(4):441–6. doi: 10.1016/j.ejca.2011.11.036
19. Corino VDA, Montin E, Messina A, Casali PG, Gronchi A, Marchiano A, et al. Radiomic analysis of soft tissues sarcomas can distinguish intermediate from high-grade lesions. J Magn Reson Imaging. (2018) 47(3):829–40. doi: 10.1002/jmri.25791
20. Bologna M, Calareso G, Resteghini C, Sdao S, Montin E, Corino V, et al. Relevance of apparent diffusion coefficient features for a radiomics-based prediction of response to induction chemotherapy in sinonasal cancer. NMR Biomed. (2022) 35(4):e4265. doi: 10.1002/nbm.4265
21. Bologna M, Corino VDA, Montin E, Messina A, Calareso G, Greco FG, et al. Assessment of stability and discrimination capacity of radiomic features on apparent diffusion coefficient images. J Digit Imaging. (2018) 31(6):879–94. doi: 10.1007/s10278-018-0092-9
22. Gitto S, Cuocolo R, Albano D, Morelli F, Pescatori LC, Messina C, et al. CT And MRI radiomics of bone and soft-tissue sarcomas: a systematic review of reproducibility and validation strategies. Insights Imaging. (2021) 12(1):68. doi: 10.1186/s13244-021-01008-3
23. Fritz B, Yi PH, Kijowski R, Fritz J. Radiomics and deep learning for disease detection in musculoskeletal radiology: an overview of novel MRI- and CT-based approaches. Invest Radiol. (2023) 58(1):3–13. doi: 10.1097/RLI.0000000000000907
24. Mascarenhas VV, Caetano A, Dantas P, Rego P. Advances in FAI imaging: a focused review. Curr Rev Musculoskelet Med. (2020) 13(5):622–40. doi: 10.1007/s12178-020-09663-7
25. Yushkevich PA, Piven J, Hazlett HC, Smith RG, Ho S, Gee JC, et al. User-guided 3D active contour segmentation of anatomical structures: significantly improved efficiency and reliability. Neuroimage. (2006) 31(3):1116–28. doi: 10.1016/j.neuroimage.2006.01.015
26. McCormick M, Liu X, Jomier J, Marion C, Ibanez L. ITK: enabling reproducible research and open science. Front Neuroinform. (2014) 8:13. doi: 10.3389/fninf.2014.00013
27. Haralick RM. Statistical and structural approaches to texture. P IEEE. (1979) 67(5):786–804. doi: 10.1109/PROC.1979.11328
28. Lohan DG, Seeger LL, Motamedi K, Hame S, Sayre J. Cam-type femoral-acetabular impingement: is the alpha angle the best MR arthrography has to offer? Skeletal Radiol. (2009) 38(9):855–62. doi: 10.1007/s00256-009-0745-3
29. Sutter R, Dietrich TJ, Zingg PO, Pfirrmann CW. How useful is the alpha angle for discriminating between symptomatic patients with cam-type femoroacetabular impingement and asymptomatic volunteers? Radiology. (2012) 264(2):514–21. doi: 10.1148/radiol.12112479
30. Barrientos C, Barahona M, Diaz J, Branes J, Chaparro F, Hinzpeter J. Is there a pathological alpha angle for hip impingement? A diagnostic test study. J Hip Preserv Surg. (2016) 3(3):223–8. doi: 10.1093/jhps/hnw014
31. Mascarenhas VV, Rego P, Dantas P, Caetano AP, Jans L, Sutter R, et al. Can we discriminate symptomatic hip patients from asymptomatic volunteers based on anatomic predictors? A 3-dimensional magnetic resonance study on cam, pincer, and spinopelvic parameters. Am J Sports Med. (2018) 46(13):3097–110. doi: 10.1177/0363546518800825
32. Roling MA, Mathijssen NMC, Bloem RM. Diagnostic sensitivity and specificity of dynamic three-dimensional CT analysis in detection of cam and pincer type femoroacetabular impingement. BMC Musculoskelet Disord. (2020) 21(1):37. doi: 10.1186/s12891-020-3049-3
33. Kutty S, Schneider P, Faris P, Kiefer G, Frizzell B, Park R, et al. Reliability and predictability of the centre-edge angle in the assessment of pincer femoroacetabular impingement. Int Orthop. (2012) 36(3):505–10. doi: 10.1007/s00264-011-1302-y
Keywords: radiomic, MRI, machine learning and AI, femoroacetabular impingement syndrome, features & signature, kNN (k nearest neighbor), hip joint, automatic diagnosis and prediction models
Citation: Montin E, Kijowski R, Youm T and Lattanzi R (2023) A radiomics approach to the diagnosis of femoroacetabular impingement. Front. Radiol. 3:1151258. doi: 10.3389/fradi.2023.1151258
Received: 25 January 2023; Accepted: 28 February 2023;
Published: 20 March 2023.
Edited by:
Dong Nie, University of North Carolina at Chapel Hill, United StatesReviewed by:
Zhixing Wang, University of Virginia, United StatesPeiyao Wang, University of North Carolina at Chapel Hill, United States
© 2023 Montin, Kijowski, Youm and Lattanzi. This is an open-access article distributed under the terms of the Creative Commons Attribution License (CC BY). The use, distribution or reproduction in other forums is permitted, provided the original author(s) and the copyright owner(s) are credited and that the original publication in this journal is cited, in accordance with accepted academic practice. No use, distribution or reproduction is permitted which does not comply with these terms.
*Correspondence: Eros Montin eros.montin@nyulangone.org
Specialty Section: This article was submitted to Artificial Intelligence in Radiology, a section of the journal Frontiers in Radiology