- 1Neuroscience Training Program, Wisconsin Institutes for Medical Research, University of Wisconsin–Madison, Madison, WI, United States
- 2Department of Radiology, University of Wisconsin School of Medicine and Public Health, Madison, WI, United States
- 3Department of Medical Physics, University of Wisconsin School of Medicine and Public Health, Madison, WI, United States
- 4Department of Bacteriology, University of Wisconsin–Madison, Madison, WI, United States
- 5Gnotobiotic Animal Core Facility, Biomedical Research Model Services, University of Wisconsin–Madison, Madison, WI, United States
- 6Department of Biomedical Engineering, University of Wisconsin–Madison, Madison, WI, United States
- 7Department of Psychiatry, University of Wisconsin School of Medicine and Public Health, Madison, WI, United States
The gut microbiome profoundly influences brain structure and function. The gut microbiome is hypothesized to play a key role in the etiopathogenesis of neuropsychiatric and neurodegenerative illness; however, the contribution of an intact gut microbiome to quantitative neuroimaging parameters of brain microstructure and function remains unknown. Herein, we report the broad and significant influence of a functional gut microbiome on commonly employed neuroimaging measures of diffusion tensor imaging (DTI), neurite orientation dispersion and density (NODDI) imaging, and SV2A 18F-SynVesT-1 synaptic density PET imaging when compared to germ-free animals. In this pilot study, we demonstrate that mice, in the presence of a functional gut microbiome, possess higher neurite density and orientation dispersion and decreased synaptic density when compared to age- and sex-matched germ-free mice. Our results reveal the region-specific structural influences and synaptic changes in the brain arising from the presence of intestinal microbiota. Further, our study highlights important considerations for the development of quantitative neuroimaging biomarkers for precision imaging in neurologic and psychiatric illness.
Introduction
Interactions between commensal gut bacteria and the central nervous system (CNS) profoundly impact brain structure and function (1). Clinical and epidemiological studies have shown changes in gut microbiota are associated with neurologic, neurodegenerative, and psychiatric disorders (2–6) and collectively reflect the relationship between intestinal microbiota and neurologic and psychiatric health throughout the lifespan. Although the causal links between altered gut microbiota and neurologic and psychiatric illness remains unknown, cellular and molecular studies of germ-free (GF) and gnotobiotic animals have shown disruptions to neurogenesis (7), cortical myelination (8) and microglia-mediated synaptic pruning (9) as well as deficits in microglia maturation (9) and fear extinction learning (10). However, despite a growing understanding of the microbiota-gut-brain axis and their clinical correlates, our ability to characterize, quantify, and track microbiota-gut-brain interactions in clinical practice remains limited.
These limitations largely stem from the absence of clinically accessible measures of brain structure and function that are both neurobiologically salient and sensitive to the composition of gut microbiota. Many currently employed strategies use structural techniques (qT1, T2) to study the relationship between gut microbial composition and the brain, but these methods do not provide meaningful insights into neurobiology. Additionally, even though previous work has demonstrated strong associations between altered gut microbiomes and psychiatric illness, the small number of imaging studies exploring the link between the gut and the brain are confounded by an inability to distinguish neuropathological changes occurring as a result of the disease process from those potentially arising from the gut microbiota themselves (11). And critically, although studies of germ-free (GF) and gnotobiotic animals have uncovered the molecular and cellular effects of commensal gut bacteria on the brain, little is known about the contribution of gut microbiota to baseline measures of neural microstructure or brain function, which further hampers our collective ability to understand and ultimately assess the impact of altered gut microbiota in the disease state.
Guided by previous evidence demonstrating that GF and conventionally colonized mice possess significant differences in neurite morphology (12) and that alterations in synaptic density, a crucial marker of overall brain function, is associated with neurodevelopmental disorders such as autism spectrum disorder (ASD) and psychiatric illnesses such as schizophrenia and major depressive disorder (13–15), we performed 18F-SynVesT-1/NODDI (neurite orientation dispersion and density imaging) PET/MRI on GF and gnotobiotic mice to acquire both structural (neurite density and morphology) and synaptic measures of the brain that are both quantitative, complementary, and translationally relevant. Further, as a corollary, we sought to determine the degree to which resident gut microbes contribute to brain microstructure and synaptic density. Lastly, as both 18F-SynVesT-1 PET and NODDI MRI are techniques that can be performed on clinical timescales, our findings additionally highlight the clinical translational potential of 18F-SynVesT-1/NODDI PET/MRI for imaging the structural and synaptic correlates of the gut microbiome in human health and disease.
Materials and Methods
Animals
All experimental work was performed in accordance with animal protocols approved by the institutional animal care and use committee at our institution (Protocol Nos. M005899, M05599, and M05532). All GF C57BL/6 mice were maintained in a controlled environment in plastic flexible film gnotobiotic isolators under a strict 12 h light/dark cycle and received sterilized water and standard chow (LabDiet 5021; LabDiet, St Louis, MO) ad libitum. Sterility of GF animals was confirmed by incubating freshly collected fecal samples under aerobic and anaerobic conditions using standard microbiology methods and PCR analysis of the bacterial 16S DNA region. Humanized gnotobiotic (HG) mice were generated by oral gavage of GF mice with well-characterized human gut bacterial isolates (16) at 8 weeks of age, which contained a representative core community of eight species that are commonly found in the human microbiota (Anaerotruncus colihominis, Bacteroides caccae, Bacteroides thetaiotaomicron, Clostridium symbiosum, Collinsella aerofaciens, Coprococcus comes, Providencia stuartii and Ruminococcus torques). All HG animals are bred and maintained under specific pathogen free conditions and maintained on an irradiated standard chow diet (TD.2918; Envigo, Madison, WI). The successful transplantation of this community was confirmed using community profiling by sequencing (COPRO-Seq) (16). All animals were born and raised at our institution. The age of experimental animals were P55 germ-free (n = 5); gnotobiotic (n = 5); and P90 germ-free (n = 4) and gnotobiotic (n = 4). Only male animals were used to control for estrous effects. Imaging sample size estimations are based on a Markov Chain Monte Carlo approach (17) and informed by our previously published work (18–21) with power analyses indicating observed effect size values of d = 2.5 or greater given low standard deviation between within-group replicates (σ between 0.01 and 0.001), thus validating sample sizes of 4 or greater replicates per group.
18F-SynVesT-1 Generation
18F-SynVesT-1 was generated as previously described (22). Briefly, cyclotron produced 18F-fluoride ions were first trapped onto a quaternary ammonium anion (QMA) exchange cartridge, eluted, dried, and mixed with a fresh prepared solution of 2.5 mg SynVesT-1 precursor, 5 mg copper triflate, and 8 μL of pyridine in 0.5 mL of dimethylacetamide; the reaction vial was then heated at 110°C for 20 min, cooled to 50°C and purified with a Luna C18 (2) 10 × 250 mm (5 μm) column. The purified product was collected into a dilution vial containing 40 mL of water and with a C18 cartridge, subsequently trapped, washed, and eluted with 1 mL of ethanol injection into a product vial containing 5 mL of 0.9% sodium chloride. The product purity was >99% and decay uncorrected yield was 16%.
PET/CT Imaging and PET ROI Analysis
At post-natal days 55 and 90 (P55 and P90), age- and sex-matched male GF mice and HG mice were imaged under isoflurane gas anesthesia on a small animal PET/CT scanner (Siemens Inveon Hybrid). CT was acquired for attenuation correction and anatomic localization. Dynamic PET imaging was carried out under anesthesia for 45 min. 5.5 MBq ± 0.4 (range 4.7–6.0) of 18F-SynVesT-1 was administered intravenously through the lateral tail vein. The 45-min 18F-SynVesT-1 PET acquisition was reconstructed into 1-min-per-frame images, starting at t = 60 s using 3-dimensional ordered-subset expectation maximization (OSEM, 2 iterations, 16 subsets) followed by a maximum a posteriori probability (MAP) algorithm. The PET and CT images were reconstructed at 128 × 128 × 159 (0.78 × 0.78 × 0.8 mm) and 480 × 480 × 635 (0.21 × 0.21 × 0.21 mm), respectively. PET images were converted to standardized uptake values (SUV). 3D regions of interest (ROIs) were manually placed over the whole brain and SUVmean (herein referred to as SUV) at each frame was recorded using MIM (MIM Software Inc., Cleveland OH). One GF mouse was excluded from the 18F-SynVesT-1 analysis due to substantial motion. SUVs at each time point were averaged within each mouse cohort and 18F-SynVesT-1 time-activity curves were generated for each cohort. The area under the curve (AUC) for GF and HG cohorts were calculated at the peak stabilized interval (4.5–10.5 min).
After 24 h, the same mice were administered 10.7 MBq ± 0.2 (range 10.4–11.1) of 18F-flurodeoxyglucose (FDG) through the lateral tail vein. Sixty minutes post injection, PET imaging was performed acquiring approximately 50 million counts per mouse. As with the 18F-SynVesT-1 PET data, 18F-FDG PET images were reconstructed using 3-dimensional ordered-subset expectation maximization (OSEM, 2 iterations, 16 subsets) followed by a maximum a posteriori probability (MAP) algorithm. CT attenuation, scatter and decay correction were applied to all datasets. The PET and CT images were also reconstructed at the spatial resolutions of 128 × 128 × 159 (0.78 × 0.78 × 0.8 mm) and 480 × 480 × 635 (0.21 × 0.21 × 0.21 mm), respectively.
The Waxholm MR atlas (23) was used to identify the hippocampus, neocortex, amygdala, ventral thalamus, lateral thalamus, globus pallidus, caudate putamen, hypothalamus, and accumbens. After upsampling the PET images to match the resolution of the CT images, brain masks were semi-automatically generated by thresholding the CT image in MATLAB to include brain tissue and were refined using subsequent image dilation and filing steps as needed. All brain masks were visually confirmed for each subject. The Waxholm MR atlas was individually registered to the CT brain masks of each subject using affine registration in MATLAB (imregtform). Then, the registered Waxholm atlases were used to compute SUV values for each brain region.
MR Imaging Acquisition, Data Preprocessing, and Analysis
Following PET imaging, animals were transcardially perfused with 4% paraformaldehyde and brains were excised for ex vivo MRI acquisition with a 4.7T Agilent MRI system and a 3.5 cm diameter quadrature volume RF coil. Ex vivo imaging and analysis, including standard data preprocessing, and study template generation, was performed as previously described (24). Multi-slice, diffusion-weighted spin echo images were used to acquire 10 non-diffusion-weighted images (b = 0 s/mm2) and 75 diffusion-weighted images (25 images: b=800 s/mm2; 50 images: b = 2,000 s/mm2) using non-collinear, diffusion-weighting directions. Diffusion imaging was performed with an echo time of 24.17/2,000 ms, field of view = 30 × 30 mm2, and matrix = 192 × 192 reconstructed to 256 × 256 for an isotropic voxel size of 0.25 mm over 2 signal averages. Multi-shell diffusion data were fit with the Microstructure Diffusion Toolbox (25) to the NODDI ex vivo model. An additional compartment of isotropic restriction was included to account for potential fixative effects as recommended (26). Tract-based spatial statistics (TBSS) was performed as previously described (21) with an FA threshold of 0.2 applied for skeleton generation, a permutation test with n = 252, multiple comparisons correction and threshold-free cluster enhancement; p < 0.05 was utilized as a threshold for significance. Regions of interest (ROIs), including the left and right hippocampus, frontal association cortex, and amygdala, were selected a priori and defined with a DTI-based mouse brain atlas (27).
Statistical Analysis
Imaging sample size estimations are based on a Markov Chain Monte Carlo approach (17) and informed by our previously published work (18–21) with power analyses indicating observed effect size values of d = 2.5 or greater given low standard deviation between within-group replicates (σ between 0.01 and 0.001), thus validating sample sizes of 4 or greater replicates per group [significance level of 5% and power of 90% (11)]. Statistical differences between groups were performed using a two-tailed unpaired Student's t-test; p < 0.05 was established as the significance level. For MRI ROI analyses, a Student's t-test was used to determine the significance (p < 0.05) of ROI values between GF and gnotobiotic mice; statistically significant differences were determined after controlling for multiple comparisons with the Benjamini–Hochberg procedure (false discovery rate = 0.05).
Results
The Presence of a Functional Gut Microbiome Is Associated With Increased Neurite Density and Orientation Dispersion Compared to Germ-Free Mice
To determine whether commonly employed diffusion weighted imaging techniques are sensitive to alterations in brain microstructure due to the presence of a gut microbiome, we first performed DTI and NODDI on male GF and HG mice. Whole-brain voxelwise tract-based spatial statistics (TBSS) analysis comparing the HG mice to age and sex-matched GF mice was performed at P55 and P90. No significant differences in fractional anisotropy (FA) were identified; however, TBSS studies uncovered significant voxelwise changes in mean diffusivity (MD) and a reduction in neurite density (NDI) and orientation dispersion (ODI) in GF mice when compared to HG mice at the P55 time point (Figure 1). By P90, TBSS showed similar global increases in MD, but no significant difference in NDI or ODI between humanized gnotobiotic (HG) and GF animals.
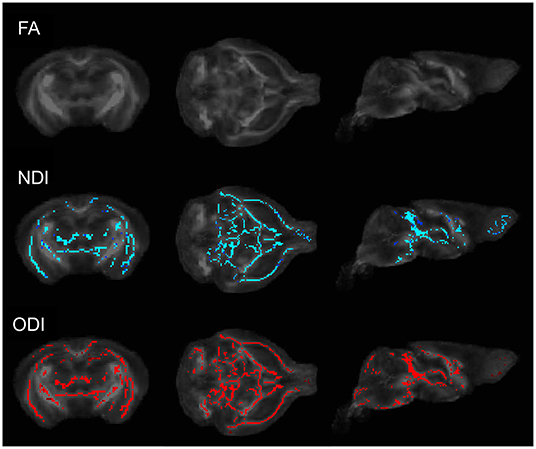
Figure 1. Coronal (left), axial (middle), and sagittal (right) TBSS plots of voxelwise changes in FA, NDI, and ODI between GF and HG P55 male animals. No significant voxelwise differences in fractional anisotropy (FA) were identified; however, TBSS analyses uncovered significant voxelwise reductions in both neurite density (NDI) and orientation dispersion (ODI) in GF mice when compared to HG mice. At P90, no significant voxelwise differences in NDI or ODI were detected between HG and GF animals.
We next performed a region of interest (ROI) analysis to test the contribution of the gut microbiome to brain microstructure in ROIs salient to neuropsychiatric and neurocognitive illness. Three regions were selected a priori for further analysis: the hippocampus, frontal association cortex, and amygdala. Mean values of DTI and neurite indices were computed within each ROI (left and right) for each individual subject for a total of 6 calculated ROIs per subject. Our results match the TBSS findings with statistically significant increases in MD, reduced NDI, and reduced ODI found in most regions of GF mice when compared to HG animals (unpaired t-test; p < 0.05) (Table 1). Differences were again more evident in animals at P55 than at P90.
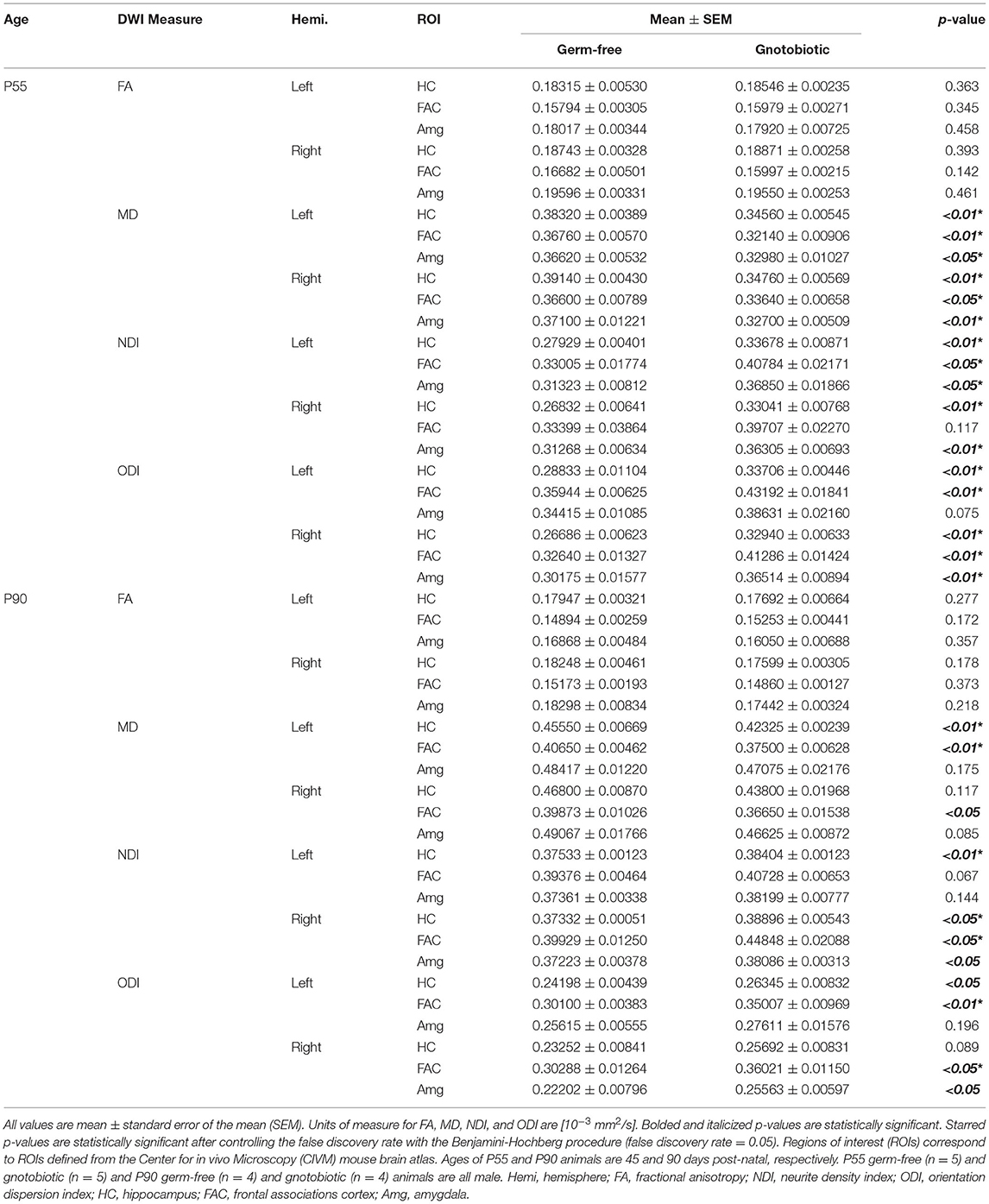
Table 1. Humanized gut microbiota contribute to significant changes in quantitative neural microstructure in salient regions implicated in neurologic and psychiatric illness.
The Presence of a Functional Gut Microbiome Is Associated With Decreased Synaptic Density Compared to Germ-Free Mice
The effect of gut microbiota on synaptic density was next analyzed via in vivo PET/CT imaging with 18F-SynVesT-1 at P90 (Figures 2A,B). 18F-FDG PET imaging was also performed to examine potential metabolic differences between GF and HG animals. Static 18F-FDG imaging was obtained 60 min after injection of the radiotracer; dynamic 18F-SynVesT-1 imaging was obtained over 45 min. The time-activity curve of 18F-SynVesT-1 shows a rapid increase, peaking at ~ 2 min, followed by clearance of the radiotracer by 20 min (Figure 2C). Area under the curve (AUC) analysis indicates significantly reduced SUV(mean) in HG mice compared to the GF mice (Figure 2D). There was no significant difference in the uptake of 18F-FDG (Figure 2E). In parallel to our diffusion-weighted imaging ROI analysis, the hippocampus, neocortex, and amygdala were selected a priori for 18F-SynVesT-1 binding ROI analysis, while the ventral thalamus, lateral thalamus, globus pallidus, caudate putamen, hypothalamus and accumbens were further selected for exploratory analysis. HG mice demonstrated reduced SV2A binding with significantly reduced SUV(mean) in all regions except for the caudate putamen (unpaired t-test; p < 0.05) (Table 2).
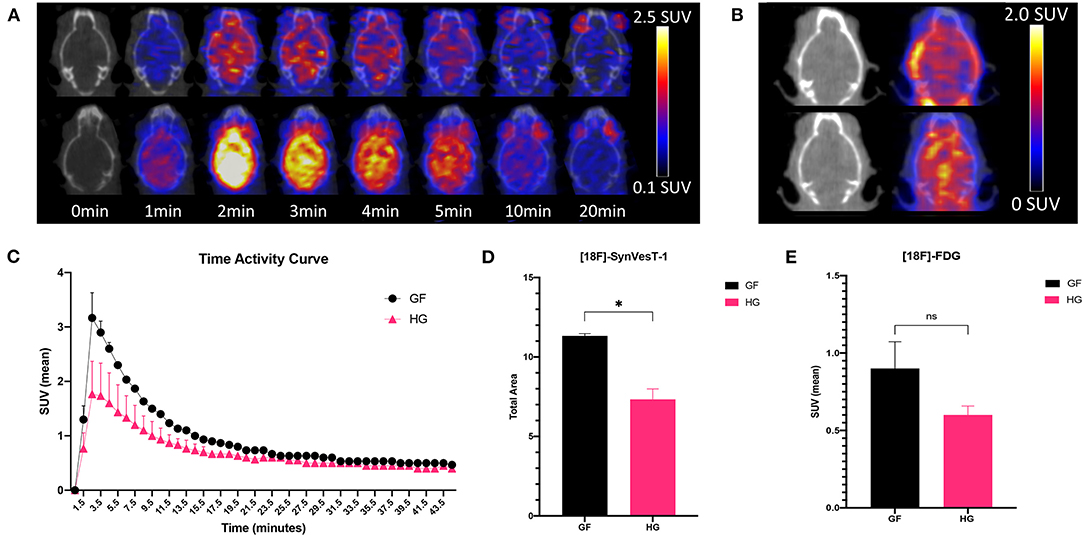
Figure 2. (A) Fused axial PET/CT images of male P90 HG (top row) and GF (bottom row) mice following the injection of 18F-SynVesT-1 demonstrating the qualitative higher uptake of the SynVesT-1 radiotracer in GF mice. (B) Static 18F-FDG images of HG (top row) and GF (bottom row). (C) Time-activity curves (mean ± SD) of male GF and HG P90 mice. The area under the curve was calculated for the peak stabilized interval (4.5–10.5 min) for 18F-SynVesT-1 (D) and 18F-FDG (E). HG, humanized gnotobiotic mice; GF, germ-free; *p< 0.05.
Discussion
Gut bacteria significantly influence numerous molecular and cellular processes (7–9, 28–35) and are also associated with a broad spectrum of neuropsychiatric illnesses (36–40). With growing recognition of the salience of the gut microbiota in neurologic and neuropsychiatric health, there is not only an intense interest to more fully understand the gut microbiota-brain axis and its relationship to psychiatric illness but also to uncover the biological mechanisms that lead to the psychiatric disease state. However, significant barriers currently limit our ability to translate emerging scientific insights about gut microbiota effects on psychiatric health into clinical populations. These include a limited understanding of the contribution of gut microbiota to baseline measures of neural microstructure or brain function and the absence of a clinically accessible method to quantitatively evaluate the changes that occur in the brain due to alterations in the gut microbiota. To address these shortcomings, we have demonstrated the potential utility of 18F-SynVesT-1/NODDI PET/MRI as a novel multimodal neuroimaging method that can be utilized to acquire quantitative measures of structural (neurite density and morphology) and synaptic density changes attributable to the presence of an intact functional gut microbiome. We further show that GF mice harbor reduced neurite density and reduced orientation dispersion when compared to mice with a humanized gnotobiotic gut microbiome and additionally demonstrate GF mice possess increased synaptic density that is potentially attributable to poor synaptic pruning in the absence of a functional gut microbiome (9).
Although there are no directly comparable studies in the literature, our results confirm findings from previous animal studies that indicate that the gut microbiome is crucial for normal morphological development of neurons and synaptic pruning. Luczynski et al. (12) showed that the neurons of the hippocampus in germ-free mice had shorter neurites with less branching and thinner spines at a P63-70 time point, which is compatible with our findings of reduced neurite density and orientation dispersion in germ-free mice. Our PET findings are also compatible with recent work finding that germ-free mice over-express synapse-related genes, which leads to an abnormal increase in synapse formation (41). As previous neuroanatomical and cellular studies of the developing central nervous system are consistent with our neuroimaging findings, multiparametric PET/MR studies with 18F-SynVesT-1/NODDI are thus well-positioned to explore gut microbial influences on brain microstructure and synaptic density.
Our findings also raise important considerations for the neuroimaging of psychiatric illness and rigor and reproducibility of such research. A second but no less important conclusion of our results is the finding that gut colonization significantly influences quantitative neuroimaging measures of brain structure and function. Diffusion tensor imaging and emerging multicompartment diffusion imaging methods such as NODDI are widely used to study neural microstructure in neurologic and psychiatric illness; likewise, new SV2A synaptic PET radiotracers such as 11C-UCB-J and 18F-SynVesT-1 are rapidly emerging as important imaging tools to examine important biological correlates underlying the pathogenesis of schizophrenia and other psychiatric illnesses (42). Although we present and demonstrate 18F-SynVesT-1/NODDI PET/MRI as a multiparametric technique to capture salient structural and functional changes attributable to the presence of a functional gut microbiome, conversely and critically, it is also conceivable that psychiatric neuroimaging studies and imaging endophenotypes of psychiatric illness may be unknowingly affected by metagenomic effects that can further challenge efforts to identify and refine candidate neuroimaging biomarkers across a wide variety of diseases, especially those with a known connection to gut microbial dysbiosis.
Our study has limitations. One limitation is our abridged PET analysis. Ideally, binding potential of the SynVesT-1 tracer would be quantified in regions of interest through tracer kinetic modeling with metabolite correction following arterial blood sampling, which would be beyond the scope of a pilot study. In its place, we compared integrated time-activity curves at early time points due to fast tracer kinetics and expected high metabolite fractions at later time points as has been reported in other similar SV2A tracers (43). Likewise, reference region analysis using the centrum semiovale (white matter, as previously reported in human studies) is precluded in this murine study due to limited scanner resolution. Kinetic modeling with arterial blood sampling will be pursued in follow-up studies. An additional limitation is that our results do not provide insight into how future studies might discriminate the degree and extent observed structural and synaptic changes might be attributable to either the underlying disease process or unaccounted metagenomic contributions. Careful study design incorporating metagenomic data as potential study confounds will be needed to control and account for these important contributors to measured brain microstructure.
Conclusion
In conclusion, we present initial pilot data for 18F-SynVesT-1/NODDI PET/MRI as a clinically accessible multiparametric neuroimaging approach to acquire both structural and synaptic brain measures that are quantitative, complementary, and reflective of brain changes attributable to the presence of a gut microbiome. Our work, for the first time, importantly highlights the sensitivity of commonly employed neuroimaging techniques (e.g., diffusion MRI) to non-genetic and environmental factors like the gut microbiome, which can potentially have a crucial and outsized effect in neuroimaging studies of the brain. This raises the potential need to incorporate such factors into future study designs if we are to achieve precision imaging in diseases of the CNS and to build greater rigor and reproducibility into quantitative neuroimaging research. With growing evidence of the role of the gut microbiome in early neurodevelopment and neuropsychiatric illness, 18F-SynVesT-1/NODDI PET/MRI can be employed to not only to further examine the biological underpinnings of the microbiome-gut-brain axis, but also to improve clinical diagnostic accuracy, patient risk stratification, and therapeutic monitoring for neuropsychiatric disorders.
Data Availability Statement
The raw data supporting the conclusions of this article will be made available by the authors, without undue reservation.
Ethics Statement
The animal study was reviewed and approved by University of Wisconsin Institutional Animal Care and Use Committee (IACUC).
Author Contributions
SY, AP, and J-PY wrote the manuscript. SY, AP, JJ, BB, NS, and EV performed all experimental work. SY, AP, JW, TB, AM, FR, and J-PY interpreted the experimental data. J-PY is the guarantor of this work, and as such, had full access to all the data in the study and assumes responsibility for the integrity of the data and the accuracy of the data analyses. All authors contributed to the article and approved the submitted version.
Funding
This work was supported by the Department of Radiology at the University of Wisconsin School of Medicine and Public Health and by the Brain and Behavior Research Foundation (NARSAD) Young Investigator Grant. Additional support was provided by the Clinical and Translational Science Award (CTSA) program, through the NIH National Center for Advancing Translational Sciences (NCATS) (UL1TR002373). SY was supported by the NIH/NIA award number F30AG066329. The content is solely the responsibility of the authors and does not necessarily represent the official views of the NIH.
Conflict of Interest
The authors declare that the research was conducted in the absence of any commercial or financial relationships that could be construed as a potential conflict of interest.
Publisher's Note
All claims expressed in this article are solely those of the authors and do not necessarily represent those of their affiliated organizations, or those of the publisher, the editors and the reviewers. Any product that may be evaluated in this article, or claim that may be made by its manufacturer, is not guaranteed or endorsed by the publisher.
References
1. Sharon G, Sampson TR, Geschwind DH, Mazmanian SK. The central nervous system and the gut microbiome. Cell. (2016) 167:915–32. doi: 10.1016/j.cell.2016.10.027
2. Tang W, Meng Z, Li N, Liu Y, Li L, Chen D, et al. Roles of gut microbiota in the regulation of hippocampal plasticity, inflammation, and hippocampus-dependent behaviors. Front Cell Infect Microbiol. (2020) 10:611014. doi: 10.3389/fcimb.2020.611014
3. Bairamian D, Sha S, Rolhion N, Sokol H, Dorothée G, Lemere CA, et al. Microbiota in neuroinflammation and synaptic dysfunction: a focus on Alzheimer's disease. Mol Neurodegener. (2022) 17:19. doi: 10.1186/s13024-022-00522-2
4. Goyal MS, Venkatesh S, Milbrandt J, Gordon JI, Raichle ME. Feeding the brain and nurturing the mind: Linking nutrition and the gut microbiota to brain development. Proc Natl Acad Sci U S A. (2015) 112:14105–12. doi: 10.1073/pnas.1511465112
5. Duscha A, Gisevius B, Hirschberg S, Yissachar N, Stangl GI, Eilers E, et al. Propionic acid shapes the multiple sclerosis disease course by an immunomodulatory mechanism. Cell. (2020) 180:1067–80. doi: 10.1016/j.cell.2020.02.035
6. Reynders T, Devolder L, Valles-Colomer M, Van Remoortel A, Joossens M, De Keyser J, et al. Gut microbiome variation is associated to multiple sclerosis phenotypic subtypes. Ann Clin Transl Neurol. (2020) 7:406–19. doi: 10.1002/acn3.51004
7. Ogbonnaya ES, Clarke G, Shanahan F, Dinan TG, Cryan JF, O'Leary OF. Adult hippocampal neurogenesis is regulated by the microbiome. Biol Psychiatry. (2015) 78:e7–9. doi: 10.1016/j.biopsych.2014.12.023
8. Hoban AE, Stilling RM, Ryan FJ, Shanahan F, Dinan TG, Claesson MJ, et al. Regulation of prefrontal cortex myelination by the microbiota. Transl Psychiatry. (2016) 6:e774. doi: 10.1038/tp.2016.42
9. Erny D, De Angelis ALH, Jaitin D, Wieghofer P, Staszewski O, David E, et al. Host microbiota constantly control maturation and function of microglia in the CNS. Nat Neurosci. (2015) 18:965–77. doi: 10.1038/nn.4030
10. Chu C, Murdock MH, Jing D, Won TH, Chung H, Kressel AM, et al. The microbiota regulate neuronal function and fear extinction learning. Nature. (2019) 574:543–8. doi: 10.1038/s41586-019-1644-y
11. Ong IM, Gonzalez JG, McIlwain SJ, Sawin EA, Schoen AJ, Adluru N, et al. Gut microbiome populations are associated with structure-specific changes in white matter architecture. Transl Psychiatry. (2018) 8:6. doi: 10.1038/s41398-017-0022-5
12. Luczynski P, Whelan SO, O'Sullivan C, Clarke G, Shanahan F, Dinan TG, et al. Adult microbiota-deficient mice have distinct dendritic morphological changes: differential effects in the amygdala and hippocampus. Eur J Neurosci. (2016) 44:2654–66. doi: 10.1111/ejn.13291
13. Valnegri P, Huang J, Yamada T, Yang Y, Mejia LA, Cho HY, et al. RNF8/UBC13 ubiquitin signaling suppresses synapse formation in the mammalian brain. Nat Commun. (2017) 8:1271. doi: 10.1038/s41467-017-01333-6
14. Coley AA, Gao W-J. PSD95: a synaptic protein implicated in schizophrenia or autism? Prog Neuropsychopharmacol Biol Psychiatry. (2018) 82:187–94. doi: 10.1016/j.pnpbp.2017.11.016
15. Holmes SE, Scheinost D, Finnema SJ, Naganawa M, Davis MT, DellaGioia N, et al. Lower synaptic density is associated with depression severity and network alterations. Nat Commun. (2019) 10:1–10. doi: 10.1038/s41467-019-09562-7
16. Kasahara K, Krautkramer KA, Org E, Romano KA, Kerby RL, Vivas EI, et al. Interactions between roseburia intestinalis and diet modulate atherogenesis in a murine model. Nat Microbiol. (2018) 3:1461–71. doi: 10.1038/s41564-018-0272-x
17. Allgoewer A, Mayer B. Sample size estimation for pilot animal experiments by using a Markov Chain Monte Carlo approach. Altern Lab Anim. (2017) 45:83–90. doi: 10.1177/026119291704500201
18. Barnett BR, Anderson JM, Torres-Velazquez M, Yi SY, Rowley PA, Yu J-PJ. Exercise ameliorates deficits in neural microstructure in a disc1 model of psychiatric illness. Magn Reson Imaging. (2019) 61:90–6. doi: 10.1016/j.mri.2019.05.021
19. Rowley PA, Guerrero-Gonzalez J, Alexander AL, Yu JPJ. Convergent microstructural brain changes across genetic models of autism spectrum disorder—a pilot study. Psychiatry Res - Neuroimaging. (2019) 283:83–91. doi: 10.1016/j.pscychresns.2018.12.007
20. Torres-Velazquez M, Sawin EA, Anderson JM, Yu J-PJ. Refractory diet-dependent changes in neural microstructure: implications for microstructural endophenotypes of neurologic and psychiatric disease. Magn Reson Imaging. (2019) 58:148–55. doi: 10.1016/j.mri.2019.02.006
21. Barnett BR, Torres-Velazquez M, Yi SY, Rowley PA, Sawin EA, Rubinstein CD, et al. Sex-specific deficits in neurite density and white matter integrity are associated with targeted disruption of exon 2 of the Disc1 gene in the rat. Transl Psychiatry. (2019) 9:82. doi: 10.1038/s41398-019-0429-2
22. Li S, Cai Z, Wu X, Holden D, Pracitto R, Kapinos M, et al. Synthesis and in vivo evaluation of a novel PET radiotracer for imaging of synaptic vesicle glycoprotein 2A (SV2A) in nonhuman primates. ACS Chem Neurosci. (2019) 10:1544–54. doi: 10.1021/acschemneuro.8b00526
23. Johnson GA, Badea A, Brandenburg J, Cofer G, Fubara B, Liu S, et al. Waxholm space: an image-based reference for coordinating mouse brain research. Neuroimage. (2010) 53:365–72. doi: 10.1016/j.neuroimage.2010.06.067
24. Yi SY, Stowe NA, Barnett BR, Dodd K, Yu JPJ. Microglial density alters measures of axonal integrity and structural connectivity. Biol Psychiatry Cogn Neurosci Neuroimaging. (2020) 5:1061–8. doi: 10.1016/j.bpsc.2020.04.008
25. Harms RL, Fritz FJ, Tobisch A, Goebel R, Roebroeck A. Robust and fast nonlinear optimization of diffusion MRI microstructure models. Neuroimage. (2017) 155:82–96. doi: 10.1016/j.neuroimage.2017.04.064
26. Alexander DC, Hubbard PL, Hall MG, Moore EA, Ptito M, Parker GJM, et al. Orientationally invariant indices of axon diameter and density from diffusion MRI. Neuroimage. (2010) 52:1374–89. doi: 10.1016/j.neuroimage.2010.05.043
27. Jiang Y, Johnson GA. Microscopic diffusion tensor atlas of the mouse brain. Neuroimage. (2011) 56:1235–43. doi: 10.1016/j.neuroimage.2011.03.031
28. O'Mahony SM, Clarke G, Borre YE, Dinan TG, Cryan JF. Serotonin, tryptophan metabolism and the brain-gut-microbiome axis. Behav Brain Res. (2015) 277:32–48. doi: 10.1016/j.bbr.2014.07.027
29. Braniste V, Al-Asmakh M, Kowal C, Anuar F, Abbaspour A, Tóth M, et al. The gut microbiota influences blood-brain barrier permeability in mice. Sci Transl Med. (2014) 6:158. doi: 10.1126/scitranslmed.3009759
30. Möhle L, Mattei D, Heimesaat MM, Bereswill S, Fischer A, Alutis M, et al. Ly6Chi monocytes provide a link between antibiotic-induced changes in gut microbiota and adult hippocampal neurogenesis. Cell Rep. (2016) 15:1945–56. doi: 10.1016/j.celrep.2016.04.074
31. Matcovitch-Natan O, Winter DR, Giladi A, Aguilar SV, Spinrad A, Sarrazin S, et al. Microglia development follows a stepwise program to regulate brain homeostasis. Science. (2016) 353:aad8670. doi: 10.1126/science.aad8670
32. Gacias M, Gaspari S, Santos PMG, Tamburini S, Andrade M, Zhang F, et al. Microbiota-driven transcriptional changes in prefrontal cortex override genetic differences in social behavior. Elife. (2016) 5:e13442. doi: 10.7554/eLife.13442
33. Bercik P, Denou E, Collins J, Jackson W, Lu J, Jury J, et al. The intestinal microbiota affect central levels of brain-derived neurotropic factor and behavior in mice. Gastroenterology. (2011) 141:599–609. doi: 10.1053/j.gastro.2011.04.052
34. Bercik P, Park AJ, Sinclair D, Khoshdel A, Lu J, Huang X, et al. The anxiolytic effect of Bifidobacterium longum NCC3001 involves vagal pathways for gut-brain communication. Neurogastroenterol Motil. (2011) 23:1132–9. doi: 10.1111/j.1365-2982.2011.01796.x
35. Desbonnet L, Clarke G, Traplin A, O'Sullivan O, Crispie F, Moloney RD, et al. Gut microbiota depletion from early adolescence in mice: implications for brain and behaviour. Brain Behav Immun. (2015) 48:165–73. doi: 10.1016/j.bbi.2015.04.004
36. Eltokhi A, Janmaat IE, Genedi M, Haarman BCM, Sommer IEC. Dysregulation of synaptic pruning as a possible link between intestinal microbiota dysbiosis and neuropsychiatric disorders. J Neurosci Res. (2020) 98:1335–69. doi: 10.1002/JNR.24616/v3/response1
37. Sullivan R, Wilson DA, Feldon J, Yee BK, Meyer U, Richter-Levin G, et al. The international society for developmental psychobiology annual meeting symposium: impact of early life experiences on brain and behavioral development. Dev Psychobiol. (2006) 48:583–602. doi: 10.1002/dev.20170
38. Bilbo SD, Levkoff LH, Mahoney JH, Watkins LR, Rudy JW, Maier SF. Neonatal infection induces memory impairments following an immune challenge in adulthood. Behav Neurosci. (2005) 119:293–301. doi: 10.1037/0735-7044.119.1.293
39. Goehler LE, Park SM, Opitz N, Lyte M, Gaykema RPA. Campylobacter jejuni infection increases anxiety-like behavior in the holeboard: possible anatomical substrates for viscerosensory modulation of exploratory behavior. Brain Behav Immun. (2008) 22:354–66. doi: 10.1016/j.bbi.2007.08.009
40. Rogers GB, Keating DJ, Young RL, Wong M-L, Licinio J, Wesselingh S. From gut dysbiosis to altered brain function and mental illness: mechanisms and pathways. Mol Psychiatry. (2016) 21:1–11. doi: 10.1038/mp.2016.50
41. Luck B, Engevik MA, Ganesh BP, Lackey EP, Lin T, Balderas M, et al. Bifidobacteria shape host neural circuits during postnatal development by promoting synapse formation and microglial function. Sci Rep. (2020) 10:1–18. doi: 10.1038/s41598-020-64173-3
42. Onwordi EC, Halff EF, Whitehurst T, Mansur A, Cotel M-C, Wells L, et al. Synaptic density marker SV2A is reduced in schizophrenia patients and unaffected by antipsychotics in rats. Nat Commun. (2020) 11:246. doi: 10.1038/s41467-019-14122-0
Keywords: gut microbiome, synaptic density, brain microstructure, SynVesT-1, NODDI, PET/MRI
Citation: Yi SY, Pirasteh A, Wang J, Bradshaw T, Jeffery JJ, Barnett BR, Stowe NA, McMillan AB, Vivas EI, Rey FE and Yu J-P (2022) 18F-SynVesT-1 PET/MR Imaging of the Effect of Gut Microbiota on Synaptic Density and Neurite Microstructure: A Preclinical Pilot Study. Front. Radiol. 2:895088. doi: 10.3389/fradi.2022.895088
Received: 12 March 2022; Accepted: 04 May 2022;
Published: 23 May 2022.
Edited by:
Michael Iv, Stanford University, United StatesReviewed by:
Martina Di Stasi, University of Naples Federico II, ItalyCamilla Russo, University of Naples Federico II, Italy
Copyright © 2022 Yi, Pirasteh, Wang, Bradshaw, Jeffery, Barnett, Stowe, McMillan, Vivas, Rey and Yu. This is an open-access article distributed under the terms of the Creative Commons Attribution License (CC BY). The use, distribution or reproduction in other forums is permitted, provided the original author(s) and the copyright owner(s) are credited and that the original publication in this journal is cited, in accordance with accepted academic practice. No use, distribution or reproduction is permitted which does not comply with these terms.
*Correspondence: John-Paul J. Yu, anAueXVAd2lzYy5lZHU=
†These authors share first authorship