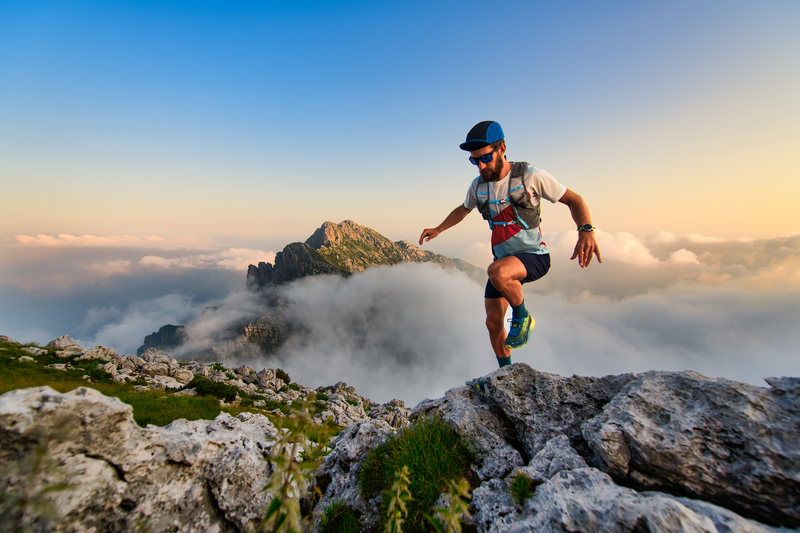
94% of researchers rate our articles as excellent or good
Learn more about the work of our research integrity team to safeguard the quality of each article we publish.
Find out more
MINI REVIEW article
Front. Antibiot. , 08 March 2024
Sec. Antibiotics in Clinical Settings
Volume 3 - 2024 | https://doi.org/10.3389/frabi.2024.1380380
This article is part of the Research Topic Rising Stars in Antibiotics View all 3 articles
Antimicrobial resistance in bacteria has been associated with significant morbidity and mortality in hospitalized patients. In the era of big data and of the consequent frequent need for large study populations, manual collection of data for research studies on antimicrobial resistance and antibiotic use has become extremely time-consuming and sometimes impossible to be accomplished by overwhelmed healthcare personnel. In this review, we discuss relevant concepts pertaining to the automated extraction of antibiotic resistance and antibiotic prescription data from laboratory information systems and electronic health records to be used in clinical studies, starting from the currently available literature on the topic. Leveraging automatic extraction and standardization of antimicrobial resistance and antibiotic prescription data is an tremendous opportunity to improve the care of future patients with severe infections caused by multidrug-resistant organisms, and should not be missed.
Antimicrobial resistance in bacteria has been associated with significant morbidity and mortality in hospitalized patients (Courvalin, 2016; Bassetti et al., 2017). Both development of severe infection and treatment of severe infection caused by multidrug-resistant (MDR) bacteria are active fields of clinical research through observational, surveillance studies assessing the epidemiology of MDR organisms, and through either observational studies or randomized controlled trials investigating efficacy and safety of old and new antibiotics for the treatment of MDR infections (Karaiskos et al., 2019; Kanj et al., 2022; Gill et al., 2024).
Collection of data for the studies mentioned above is usually performed manually, through collection of relevant demographics, clinical, therapeutic, microbiological, and prognostic information that is necessary for correctly evaluating epidemiology of MDR organisms and for assessing factors favorably or unfavorably impacting diagnosis or prognosis, by means of appropriate statistical models (Maraolo et al., 2021; Giacobbe et al., 2023a).
However, in the era of big data and of the consequent frequent (although not an absolute rule) need for large study populations, manual collection of data for research studies has become extremely time-consuming and sometimes impossible to be accomplished by overwhelmed healthcare personnel (Giacobbe et al., 2020). Against this backdrop, automated extraction of data from electronic health records (EHRs) and laboratory information systems (LISs) is attracting increasing attention for the possibility of rapidly and automatically collecting the large amounts of data necessary for training and evaluating complex statistical or machine learning models, at the same time relieving healthcare personnel from the difficult (or sometimes impossible) task of manually collecting thousands of variables (Puing et al., 2019). However, the automated extraction should be of high-quality, accurate, reproducible, and standardized, which could prove not so easy tasks, although essential in line with FAIR principles (Findability, Accessibility, Interoperability, and Reusability) (McEwen and Fedorka-Cray, 2002; Huys et al., 2007; Wilkinson et al., 2016).
In the present narrative review, we discuss relevant concepts pertaining to the automated extraction of antibiotic resistance and antibiotic prescription data from LISs and EHRs to be used in clinical studies, starting from the currently available literature on the topic (see Table 1).
On November 5, 2023, a literature search was conducted on PubMed using the keywords (EHR OR EHRs OR “electronic health record” OR EMR OR “electronic medical record” OR EPR OR “electronic personal record” OR “laboratory information system*”) AND (antibiotics OR antibiogram OR antibiotic OR antimicrobial OR “antimicrobial resistance” OR “antibiotic stewardship”) AND (“data extraction” OR “data extracted” OR “data retrieval” OR “information extraction” OR “information extracted” OR “information retrieval” OR “data mining”) NOT review and carefully evaluating the references of the articles retrieved. The resulting papers were manually screened by checking their title, abstract and full text. Inclusion criteria were: (i) publication after 2018; (ii) focus on antibiotic resistance or antibiotic prescription data from LISs or EHRs; and (iii) involvement of automatic data extraction from LISs or EHRs. We considered focus on genetic data as the only exclusion criterion. Given the availability of dedicated research in the literature (McEwen and Fedorka-Cray, 2002; Huys et al., 2007), a separate specific paragraph in the manuscript has been dedicated to automated extraction of antibiotic resistance data in veterinary medicine. Eventually, 13 articles were selected for discussion in the present review.
There are several possible methods to deal with information extraction from datasets, more specifically from EHRs. The wide range of adopted solutions is a direct consequence of the heterogeneity of the data structure: EHRs present a peculiar framework which varies by country and state, and may vary even by hospital within a limited geographic area (Ciampi et al., 2016). Moreover, EHRs often contain both structured and unstructured data, which require different extraction procedures, and may include missing data that need management (Tayefi et al., 2021).
Although different methodologies have increasingly become available for automatic extraction of data, manual extraction is still used much more frequently (Cuningham et al., 2020; Inglis et al., 2021; Tunio et al., 2023). Manual extraction is extremely time-consuming. However, it is applicable to any type and form of data. For this reason, it is frequently preferred to automatic extraction, since automatic extraction algorithms are heavily contingent on the data type and specialized personnel are needed to develop and use them.
Automatic extraction algorithms show substantial differences depending on whether the data handled are structured or unstructured. The automatic extraction of structured data requires a clear understanding of the schema of the sources, fields and relationships within data (Giacomini and Nappo, 2006). Specific queries are necessary to extract specific data. Then, the extracted data may undergo transformation and normalization to adhere to a standard format, and validation checks are implemented to ensure data integrity (e.g., checks for data type consistency and range validation). Against this background, challenges such as incomplete or inaccurate data are also frequently present (Austin et al., 2021). Therefore, the management of both missing values and data redundancies should also better be carefully defined and implemented a priori.
Algorithms dedicated to the extraction of information from unstructured data, which refers to data lacking a predefined, organized format like traditional databases, often present a more tangled framework. The absence of a rigid structure requires a more sophisticated approach, involving dedicated algorithms to navigate through the inherent variability and complexity present in unstructured data sources that present a richer and more intricate analytical context. This is because, to deal with data without a definable structure a priori, algorithms must be able to figure out, for example, where to find the information of interest, and this requires a deep understanding of the data (Zaman et al., 2020). For all articles retrieved and included in the present review, the unstructured data type was free text. The discipline that deals with understanding and managing free text data is called Natural Language Processing (NLP), which deals with making the natural language understandable to machines (Chowdhary, 2020). Many steps are often required to perform an appropriate extraction. Usually, the free texts dataset undergoes pre-processing, which involves cleaning, transforming, and organizing raw data to enhance quality and suitability for further processing. Typically, this step is followed by entity recognition and use of machine learning models. Challenges include ambiguity and variability, with variations in approaches (Casey et al., 2021; Reading Turchioe et al., 2022; Zhang et al., 2022).
As mentioned above, depending on the structure of the dataset and the specific data type, various tools can be used for the extraction of data, and different solutions can be adopted. For example, several data management tools were employed for the extraction of antibiotic resistance and antibiotic prescription data in the articles included in the present review, such as VigiLanz software, POLAR, MOMO, ETL (Extract, Transform, Load) module, SMILe and SQL. A more detailed description of these tools is available in Table 2 (Teodoro et al., 2012; Klass et al., 2013; Chao et al., 2018; Grundmeier et al., 2018; Hawes et al., 2018; Simões et al., 2018; Koller et al., 2019; Pearce et al., 2019; Brotherton et al., 2020; Vermassen et al., 2020; Yigzaw et al., 2020; Macy et al., 2021; Verberk et al., 2023b; VigiLanz, 2023; Medexter Healthcare).
To properly perform automatic information extraction, it is crucial to have a standardized data type whenever possible, in order to minimize ambiguity and enable interoperability and operational efficiency (Navigli and Velardi, 2005). In particular, the adoption of Standardized Clinical Terminology emerges as a pivotal element. This standardized terminology provides a common language to describe clinical information, minimizing ambiguity in the data and enabling optimal operational efficiency. According to the World Health Organization (WHO), a Standardized Clinical Terminology is a “compilation of terms used in the clinical assessment, management and care of patients, which includes agreed definitions that adequately represent the knowledge behind these terms and link with a standardized coding and classification system” and “Use of standardized terminology will result in better and safer patient care and more efficient health services” (Executive Board 118, 2006). Some of the papers included in the present narrative review adhere to defined terminology standards, employing a number of standardized languages to codify clinical terminologies and facilitate the automatic extraction process.
The Anatomical Therapeutic Chemical (ATC) Classification System, maintained by the WHO, organizes drugs into a hierarchy with five levels. At the top of the system, there are fourteen main groups, or 1st levels, categorized by anatomy or pharmacology. Each main group is further divided into 2nd levels, which can be either pharmacological or therapeutic groups. The 3rd and 4th levels further refine into chemical, pharmacological or therapeutic subgroups, while the 5th level specifically identifies the chemical substance (WHOCC, 2023). Therefore, since the ATC Classification is an international system used to classify drugs, in the context of the study conducted by Hawes et al. (2018), ATC codes were adopted to categorize antibiotics in a standardized way, thus providing a common basis for the data analysis.
Yigzaw et al. (2020) also employed the use of the ATC Classification System and, in addition, they used the International Classification of Primary Care, 2nd edition (ICPC-2). ICPC-2 is a classification system used to organize patient data and clinical activities in General/Family Medicine and primary care settings. This classification system helps the organization of various aspects, including the reason for the patient encounter, problems or diagnoses addressed, interventions undertaken and the structured arrangement of these data into a framework of episodes of care (WHO, 2003). In their work, Yigzaw et al. (2020) decided to use the ATC and ICPC-2 classification systems to systematically organize the acquired information, diagnosis and medical prescriptions from both the EHRs and the research databases used to store data.
The International Classification of Diseases, 9th Revision (ICD-9) has been developed by WHO with the aim to provide a standardized system to classify injuries, diseases and causes of death (ICD - ICD-9, 2023). The coding structure is represented with up to five digits, similarly to the 10th Revision (ICD-10) which, however, surpasses ICD-9 in greater detail and granularity of coded elements (ICD-10 Version: 2019). The coding structure of this newer revision is alphanumeric code with up to seven characters, enabling better accuracy to accommodate progress in technology and medical knowledge. These Standardized Clinical Terminologies have been exploited in the study of Chao et al. (2018), specifically ICD-10 codes are utilized to define a cohort of healthy individuals, excluding children with particular previous conditions. The ICD-10 codes are used also in the paper of Grundmeier et al. (2018), focusing on the task of switching the coding system from ICD-9 to ICD-10: descriptive words coupled with classification codes are used as input features because of the transition. The study highlights the complexity of accurately mapping between ICD-9 and ICD-10 codes, mainly for conditions where antibiotics are indicated. The one last of the papers analyzed where the ICD standard has been utilized is the study of Vermassen et al. (2020) where ICD-9 codes were used to define which patients had septic shock.
Another Standardized Clinical Terminology which is widely used and established is Systematized Nomenclature of Medicine - Clinical Terms (SNOMED CT), which is a complete clinical terminology developed for use in EHRs and other health information systems. It goes above and beyond coding diseases and procedures to represent clinical constructs and the links between them (SNOMED CT). SNOMED CT is a tree-like hierarchical structure where concepts are represented by univocal numeric codes. It can be employed in order to map and/or normalize various local terminologies and codes, facilitating a univocal understanding of these terms during the extraction process. In their work, Teodoro et al. (2012) discuss the usage of standard terminologies, including SNOMED CT along with WHO’s Anatomical Therapeutic Chemical (WHO-ATC) (WHOCC - ATC/DDD Index, 2023) and Universal Protein Resource (UniProt/NEWT) (UniProt, [[NoYear]]), in the ARTEMIS system. These terminologies have been mapped to the DebugIT Core Ontology and local concepts not covered within them are normalized against them using automatic classification tools.
Dealing with free text, it is not known a priori where the information of interest is located. Therefore, in addition to the extraction of natural language fields from the datasets of interest, NLP algorithms are typically implemented to properly use the information, as presented in several articles (Grundmeier et al., 2018; Vermassen et al., 2020; Inglis et al., 2021; Verberk et al., 2023b). EHRs developers are delivering an increasing number of tools, with the aim of supporting surveillance tasks, which usually utilize structured data (Hoffman and Podgurski, 2013), yet these kinds of tools are unable to manage unstructured data (such as free text).
Verberk et al. (2023a) initially described and validated a semi-automated surveillance algorithm for post-operative surgical site infections in multiple hospitals. In another paper (Verberk et al., 2023b), they proposed an improvement of the algorithm, including NLP as an add-on to free text clinical notes. To extract timely information, they implemented a list of keywords, considered as features, which also included the names of the antibiotics. This way, all texts of the clinical notes were compared with the list of keywords and each match was counted.
Vermassen et al. (2020) aimed to use NLP to identify patients with septic shock from clinical text notes from EHRs. The free text fields considered were “reason for admission”, “current medical history”, “daily notes”, “conclusion of admission”. Four dictionaries were created to apply NLP. The first dictionary contained only the term “septic shock”; the second dictionary contained terms related to infection; the third dictionary contained terms related to the need for vasopressors; the fourth dictionary contained terms related to increased lactate levels. The authors implemented two search strategies: (i) an explicit strategy, which only used the first dictionary, so patients were labeled as suffering from septic shock if this term was found in the text fields of the medical record; (ii) a combined strategy, where all four dictionaries were used and patients were labeled with septic shock if the explicit term was retrieved or if a dictionary-matching term for infection, vasopressors, or lactate was retrieved.
The aim of the study from Inglis et al. (2021) was to define machine learning models able to classify penicillin adverse drug events (ADRs) and evaluate the risk of true allergy, utilizing the free-text fields of EHRs. Once these fields were retrieved, the information about prescriptions and other crucial features was manually extracted.
Antibiotic data encompass a wide range of information, including but not limited to classes of antibiotics, mechanisms of action, resistance patterns, and efficacy of different antibiotics against specific pathogens. Understanding the complexities of antibiotics is crucial to make informed decisions in clinical practices and public health interventions. All but one of the papers contained antibiotic-related data, and all but one included general clinical data, while the other data types are strictly dependent on the specific purpose of the paper in question.
The threat of antibiotic resistance in the medical domain cannot be completely separated from that in the veterinary one. As widely demonstrated in numerous studies (Guardabassi et al., 2004; Lloyd, 2007; Allen et al., 2010; Graveland et al., 2010), animals have the ability to acquire and transmit MDR pathogens. This constitutes a potential channel for the exchange of antimicrobial resistance (AMR) with humans. The articles by Hur et al. (2022) and by Tharmakulasingam et al. (2023) explored the automatic extraction of antibiotic data in the veterinary field. It is worth noting that these studies focused deeply on automatic extraction, explaining in detail the tools created specifically for this purpose.
The study by Hur et al. (2022) aimed to describe the use, dose and common indications of antibiotic use, using NLP techniques to extract and analyze the information present in clinical records. In particular, NLP was applied to the free text fields of clinical notes, with the aim of extracting the relevant information mentioned above. The authors employed state-of-the-art NLP models, specifically VetBert (Hur et al., 2020) [the veterinary adaptation of ClinicalBert (Huang et al., 2020)]. This transformer model identified the reason for administering antimicrobials directly from the free text fields. By blending the information from structured data with the information extracted from the free-text fields through the above NLP techniques, the authors were able to calculate dose, duration, and indication for antimicrobial use.
The article by Tharmakulasingam et al. (2023) illustrates a novel way to predict development of AMR in bacteria. They used a 1D-Transformer, which helped to understand information about antibiotic use. A peculiarity of this model was indeed that an attempt was made to explain its predictions. The authors thus employed an explainable Transformer model, attempting to explain the model’s decisions in order to be understood and interpreted. Explainability (whenever completely interpretable models cannot be used or are less accurate in predictions) could be crucial, especially in medical fields, since trust in the model’s suggestions is essential for adoption by healthcare professionals. The article suggests using explainable AI (XAI) techniques, such as the Multi-Baseline Integrated Gradient approach, to enhance understanding of how the model reached its predictions and make it more transparent to users.
The automation of data extraction can strongly benefit from the possibility of standardization of health information systems as it could efficiently use the data communication structures between hospital systems and those of the Health Information Infrastructure (Ozaydin et al., 2020). First of all, it is necessary that the tools used for extraction are as unobtrusive as possible, often based on services that allow the exchange of data even between tools from technically different platforms (Gazzarata and Giacomini, 2016). However, it is not sufficient for there to be a technically effective possibility of transmitting data between different systems, it is essential that there is an understanding of the information at a higher level, therefore the use of technical interoperability tools, such as Fast Healthcare Interoperability Resources (FHIR) messages and similar (Wulff et al., 2021; Duda et al., 2022), but also knowledge sharing systems at a higher level (Blobel et al., 2023).
One of the key points to achieve a correct reuse of the data automatically extracted from health information systems is the adequate management of terminology. It was seen in previous sections how, in almost all projects taken into consideration in this review, standardized vocabularies were correctly used to manage the project’s internal terminology. However, at the same time it was clearly seen that the choice of vocabularies used is quite wide, due to the legitimate choice on the part of the specific communities that carried out each project to use that terminological collection that they consider most efficient for their purpose. Therefore, for a correct comparison between the results of individual projects, we believe it is extremely important to use the mapping services of international bodies such as the Unified Medical Language System (UMLS) centered in the USA https://www.nlm.nih.gov/research/umls/index.html, or the similar initiative set up in Europe such as Athena (https://athena.ohdsi.org/search-terms/start). However, these mappings must be maintained over time because the different vocabularies are frequently updated. Terminological services developed on international standards or machine learning technologies can be useful in these operations (Gazzarata et al., 2017; Kang et al., 2021).
Finally, the ability to create large, accurate, and standardized datasets of automatically extracted data concerning both antibiotic susceptibility (in bacteria causing the infection) and antibiotic use (in humans with the infection) should be coupled with improvements in the automatic extraction of other clinical features (e.g., information related to the acute phase of the disease, baseline comorbidities, other concomitant infectious and non-infectious conditions) (Giacobbe et al., 2023b; Giacobbe et al., 2023c). In turn, standardization of this process would allow to exploit the aid of either classical statistical models or up to date machine learning algorithms for accurately investigate clinically relevant associations between features and selected outcome of interests (e.g., diagnosis of a specific antimicrobial resistant infection, prognosis of a specific antimicrobial resistant infection), thereby improving identification of factors able to improve either diagnosis of treatment. Creating such large and accurate datasets through classical manual collection is likely nearly (or totally) impossible in the current big data era. Consequently, improving automatic extraction and standardization of antimicrobial resistance and antibiotic data is a tremendous opportunity for improving care of future patients with severe infections caused by MDR organisms, and should not be missed.
AC: Writing – original draft, Writing – review & editing. YM: Writing – original draft, Writing – review & editing. DG: Conceptualization, Writing – original draft, Writing – review & editing. SM: Conceptualization, Writing – review & editing. RG: Supervision, Writing – review & editing. NR: Supervision, Writing – review & editing. MG: Supervision, Writing – review & editing. MB: Supervision, Writing – review & editing.
The author(s) declare that no financial support was received for the research, authorship, and/or publication of this article.
Outside the submitted work, DRG reports investigator-initiated grants from Pfizer, Shionogi, and Gilead Italia, and speaker and/or advisor fees from Pfizer, Menarini and Tillotts Pharma. Outside the submitted work, MB reports research grants and/or personal fees for advisor/consultant and/or speaker/chairman from BioMérieux, Cidara, Gilead, Menarini, MSD, Pfizer, and Shionogi. Author RG is employed by Healthropy.
The remaining authors declare that the research was conducted in the absence of any commercial or financial relationships that could be construed as a potential conflict of interest.
The author(s) declared that they were an editorial board member of Frontiers, at the time of submission. This had no impact on the peer review process and the final decision.
All claims expressed in this article are solely those of the authors and do not necessarily represent those of their affiliated organizations, or those of the publisher, the editors and the reviewers. Any product that may be evaluated in this article, or claim that may be made by its manufacturer, is not guaranteed or endorsed by the publisher.
Allen H. K., Donato J., Wang H. H., Cloud-Hansen K. A., Davies J., Handelsman J. (2010). Call of the wild: antibiotic resistance genes in natural environments. Nat. Rev. Microbiol. 8, 251–259. doi: 10.1038/nrmicro2312
Austin P. C., White I. R., Lee D. S., van Buuren S. (2021). Missing data in clinical research: A tutorial on multiple imputation. Can. J. Cardiol. 37, 1322–1331. doi: 10.1016/j.cjca.2020.11.010
(2023) Finding Patients For Clinical Trials In Real Time | Clinical Surveillance Platform | Healthcare Intelligence & Decision Making Support Tools (VigiLanz). Available online at: https://vigilanz.com/the-platform/ (Accessed cited 2023 Dec 19).
Bassetti M., Poulakou G., Ruppe E., Bouza E., Van Hal S. J., Brink A. (2017). Antimicrobial resistance in the next 30 years, humankind, bugs and drugs: a visionary approach. Intensive Care Med. 43, 1464–1475. doi: 10.1007/s00134-017-4878-x
Blobel B., Ruotsalainen P., Oemig F., Giacomini M., Sottile P. A., Endsleff F. (2023). Principles and standards for designing and managing integrable and interoperable 5P medicine ecosystems. J. Personalized Med. 13, 1–23, 1579. doi: 10.3390/jpm13111579
Brotherton A. L., Rab S., Kandiah S., Kriengkauykiat J., Wong J. R. (2020). The impact of an automated antibiotic stewardship intervention for the management of Staphylococcus aureus bacteraemia utilizing the electronic health record. J. Antimicrob. Chemother. 75, 1054–1060. doi: 10.1093/jac/dkz518
Casey A., Davidson E., Poon M., Dong H., Duma D., Grivas A., et al. (2021). A systematic review of natural language processing applied to radiology reports. BMC Med. Inform Decis. Mak 21, 179. doi: 10.1186/s12911-021-01533-7
Chao Y. Y., Kociolek L. K., Zheng X. T., Scardina T., Patel S. J. (2018). Utilizing the electronic health record to construct antibiograms for previously healthy children with urinary tract infections. Infect. Control. Hosp. Epidemiol. 39, 1473–1475. doi: 10.1017/ice.2018.246
Chowdhary K. R. (2020). “Natural language processing,” in Fundamentals of Artificial Intelligence. Ed. Chowdhary K. R. (Springer India, New Delhi), 603–649. doi: 10.1007/978-81-322-3972-7_19
Ciampi M., Esposito A., Guarasci R., De Pietro G. (2016). “Towards interoperability of ehr systems: the case of Italy,” in Proceedings of the International Conference on Information and Communication Technologies for Ageing Well and e-Health. 133–138 (Rome, Italy: SCITEPRESS - Science and and Technology Publications). Available at: http://www.scitepress.org/DigitalLibrary/Link.aspx?doi=10.5220/0005916401330138.
Courvalin P. (2016). Why is antibiotic resistance a deadly emerging disease? Clin. Microbiol. Infect. 22, 405–407. doi: 10.1016/j.cmi.2016.01.012
Cuningham W., Anderson L., Bowen A. C., Buising K., Connors C., Daveson K., et al. (2020). Antimicrobial stewardship in remote primary healthcare across northern Australia. PeerJ 8, e9409. doi: 10.7717/peerj.9409
Duda S. N., Kennedy N., Conway D., Cheng A. C., Nguyen V., Zayas-Cabán T., et al. (2022). HL7 FHIR-based tools and initiatives to support clinical research: a scoping review. J. Am. Med. Inf. Assoc. 29, 1642–1653. doi: 10.1093/jamia/ocac105
Executive Board 118 (2006). eHealth: standardized terminology: report by the Secretariat. Available online at: https://iris.who.int/handle/10665/21530.
Gazzarata R., Giacomini M. (2016). A standardized SOA for clinical data sharing to support acute care, telemedicine and clinical trials. Eur. J. Biomed. Inf. 12, 49–57. doi: 10.24105/ejbi.2016.12.1.9
Gazzarata R., Monteverde M. E., Vio E., Saccavini C., Gubian L., Borgo I., et al. (2017). A terminology service compliant to CTS2 to manage semantics within the regional HIE. Eur. J. Biomed. Inf. 13, 43–50. doi: 10.24105/ejbi.2017.13.1.7
Giacobbe D. R., Marelli C., Cattardico G., Fanelli C., Signori A., Di Meco G., et al. (2023a). Mortality in KPC-producing Klebsiella pneumoniae bloodstream infections: a changing landscape. J. Antimicrob. Chemother. 78, 2505–2514. doi: 10.1093/jac/dkad262
Giacobbe D. R., Marelli C., Mora S., Guastavino S., Russo C., Brucci G., et al. (2023b). Early diagnosis of candidemia with explainable machine learning on automatically extracted laboratory and microbiological data: results of the AUTO-CAND project. Ann. Med. 55, 2285454. doi: 10.1080/07853890.2023.2285454
Giacobbe D. R., Mora S., Giacomini M., Bassetti M. (2020). Machine learning and multidrug-resistant gram-negative bacteria: an interesting combination for current and future research. Antibiotics (Basel) 9, 54. doi: 10.3390/antibiotics9020054
Giacobbe D. R., Mora S., Signori A., Russo C., Brucci G., Campi C., et al. (2023c). Validation of an automated system for the extraction of a wide dataset for clinical studies aimed at improving the early diagnosis of candidemia. Diagnostics (Basel) 13, 961. doi: 10.3390/diagnostics13050961
Giacomini M., Nappo A. (2006). An experience of microbiological data sharing. Methods Inf. Med. 45, 195–199. doi: 10.1055/s-0038-1634050
Gill C. M., Santini D., Nicolau D. P., ERACE-PA Global Study Group (2024). In vitro activity of cefiderocol against a global collection of carbapenem-resistant Pseudomonas aeruginosa with a high level of carbapenemase diversity. J. Antimicrob. Chemother. 79, 412–416. doi: 10.1093/jac/dkad396
Graveland H., Wagenaar J. A., Heesterbeek H., Mevius D., van Duijkeren E., Heederik D. (2010). Methicillin resistant Staphylococcus aureus ST398 in veal calf farming: human MRSA carriage related with animal antimicrobial usage and farm hygiene. PloS One 5, e10990. doi: 10.1371/journal.pone.0010990
Grundmeier R. W., Xiao R., Ross R. K., Ramos M. J., Karavite D. J., Michel J. J., et al. (2018). Identifying surgical site infections in electronic health data using predictive models. J. Am. Med. Inform Assoc. 25, 1160–1166. doi: 10.1093/jamia/ocy075
Guardabassi L., Schwarz S., Lloyd D. H. (2004). Pet animals as reservoirs of antimicrobial-resistant bacteria. J. Antimicrob. Chemother. 54, 321–332. doi: 10.1093/jac/dkh332
Hawes L., Turner L., Buising K., Mazza D. (2018). Use of electronic medical records to describe general practitioner antibiotic prescribing patterns. Aust. J. Gen. Pract. 47, 796–800. doi: 10.31128/AJGP-05-18-4570
Hoffman S., Podgurski A. (2013). Big bad data: law, public health, and biomedical databases. J. Law Med. Ethics 41, 56–60. doi: 10.1111/jlme.12040
Huang K., Altosaar J., Ranganath R. (2020)ClinicalBERT: modeling clinical notes and predicting hospital readmission. Available online at: http://arxiv.org/abs/1904.05342 (Accessed cited 2023 Dec 19).
Hur B., Baldwin T., Verspoor K., Hardefeldt L., Gilkerson J. (2020)Domain Adaptation and Instance Selection for Disease Syndrome Classification over Veterinary Clinical Notes. In: Proceedings of the 19th SIGBioMed Workshop on Biomedical Language Processing (Online: Association for Computational Linguistics). Available online at: https://aclanthology.org/2020.bionlp-1.17 (Accessed cited 2023 Dec 19). doi: 10.18653/v1/2020.bionlp-1
Hur B., Hardefeldt L. Y., Verspoor K. M., Baldwin T., Gilkerson J. R. (2022). Evaluating the dose, indication and agreement with guidelines of antimicrobial use in companion animal practice with natural language processing. JAC Antimicrob. Resist. 4, dlab194. doi: 10.1093/jacamr/dlab194
Huys G., Bartie K., Cnockaert M., Oanh D. T. H., Phuong N. T., Somsiri T., et al. (2007). Biodiversity of chloramphenicol-resistant mesophilic heterotrophs from Southeast Asian aquaculture environments. Res. Microbiol. 158, 228–235. doi: 10.1016/j.resmic.2006.12.011
ICD-10 Version: 2019. Available online at: https://icd.who.int/browse10/2019/en.
ICD - ICD-9 (2023). International classification of diseases, ninth revision. Available online at: https://www.cdc.gov/nchs/icd/icd9.htm.
Inglis J. M., Bacchi S., Troelnikov A., Smith W., Shakib S. (2021). Automation of penicillin adverse drug reaction categorisation and risk stratification with machine learning natural language processing. Int. J. Med. Inform. 156, 104611. doi: 10.1016/j.ijmedinf.2021.104611
Kang B., Yoon J., Kim H. Y., Jo S. J., Lee Y., Kam H. J. (2021). Deep-learning-based automated terminology mapping in OMOP-CDM. J. Am. Med. Inform Assoc. 28, 1489–1496. doi: 10.1093/jamia/ocab030
Kanj S. S., Bassetti M., Kiratisin P., Rodrigues C., Villegas M. V., Yu Y., et al. (2022). Clinical data from studies involving novel antibiotics to treat multidrug-resistant Gram-negative bacterial infections. Int. J. Antimicrob. Agents 60, 106633. doi: 10.1016/j.ijantimicag.2022.106633
Karaiskos I., Lagou S., Pontikis K., Rapti V., Poulakou G. (2019). The “Old” and the “New” Antibiotics for MDR gram-negative pathogens: for whom, when, and how. Front. Public Health 7, 151. doi: 10.3389/fpubh.2019.00151
Klass D. B., Klass A. P., Ring D. J., Goldsteen D. (2013). Method and system for monitoring patient care (United States: VigiLanz Corporation).
Koller W., Kleinoscheg G., Willinger B., Rappelsberger A., Adlassnig K. P. (2019). Augmenting analytics software for clinical microbiology by man-machine interaction. Stud. Health Technol. Inform. 264, 1243–1247. doi: 10.3233/SHTI190425
Lloyd D. H. (2007). Reservoirs of antimicrobial resistance in pet animals. Clin. Infect. Dis. 45 Suppl 2, S148–S152. doi: 10.1086/519254
Macy E., McCormick T. A., Adams J. L., Crawford W. W., Nguyen M. T., Hoang L., et al. (2021). Association between removal of a warning against cephalosporin use in patients with penicillin allergy and antibiotic prescribing. JAMA Netw. Open 4, e218367. doi: 10.1001/jamanetworkopen.2021.8367
Maraolo A. E., Corcione S., Grossi A., Signori A., Alicino C., Hussein K., et al. (2021). The impact of carbapenem resistance on mortality in patients with klebsiella pneumoniae bloodstream infection: an individual patient data meta-analysis of 1952 patients. Infect. Dis. Ther. 10, 541–558. doi: 10.1007/s40121-021-00408-8
McEwen S. A., Fedorka-Cray P. J. (2002). Antimicrobial use and resistance in animals. Clin. Infect. Dis. 34, S93–S106. doi: 10.1086/340246
Medexter Healthcare Infection control with momo. Available online at: https://www.medexter.com/products-and-services/clinical-solutions/microbiology-and-amr.
Navigli R., Velardi P. (2005). Structural semantic interconnections: a knowledge-based approach to word sense disambiguation. IEEE Trans. Pattern Anal. Mach. Intelligence 27, 1075–1086. doi: 10.1109/TPAMI.2005.149
Ozaydin B., Zengul F., Oner N., Feldman S. S. (2020). Healthcare research and analytics data infrastructure solution: A data warehouse for health services research. J. Med. Internet Res. 22, e18579. doi: 10.2196/18579
Pearce C., McLeod A., Patrick J., Ferrigi J., Bainbridge M. M., Rinehart N., et al. (2019). Coding and classifying GP data: the POLAR project. BMJ Health Care Inform. 26, e100009. doi: 10.1136/bmjhci-2019-100009
Puing A. G., Xie D., Adams-Huet B., Barros N., Yek C., Wallace B. S., et al. (2019). Impact of multidrug-resistant bacterial infections in solid-organ transplantation: the value of electronic health records-based registries and data extraction tools. Open Forum Infect. Dis. 6, S932–S933. doi: 10.1093/ofid/ofz360.2342
Reading Turchioe M., Volodarskiy A., Pathak J., Wright D. N., Tcheng J. E., Slotwiner D. (2022). Systematic review of current natural language processing methods and applications in cardiology. Heart 108, 909–916. doi: 10.1136/heartjnl-2021-319769
Simões A. S., Maia M. R., Gregório J., Couto I., Asfeldt A. M., Simonsen G. S., et al. (2018). Lapão LV. Participatory implementation of an antibiotic stewardship programme supported by an innovative surveillance and clinical decision-support system. J. Hosp. Infect. 100, 257–264. doi: 10.1016/j.jhin.2018.07.034
SNOMED CT U.S. National library of medicine. Available online at: https://www.nlm.nih.gov/healthit/snomedct/index.html.
Tayefi M., Ngo P., Chomutare T., Dalianis H., Salvi E., Budrionis A., et al. (2021). Challenges and opportunities beyond structured data in analysis of electronic health records. WIREs Comput. Stats 13, e1549. doi: 10.1002/wics.1549
Teodoro D., Pasche E., Gobeill J., Emonet S., Ruch P., Lovis C. (2012). Building a transnational biosurveillance network using semantic web technologies: requirements, design, and preliminary evaluation. J. Med. Internet Res. 14, e73. doi: 10.2196/jmir.2043
Tharmakulasingam M., Wang W., Kerby M., Ragione R. L., Fernando A. (2023). TransAMR: an interpretable transformer model for accurate prediction of antimicrobial resistance using antibiotic administration data. IEEE Access 11, 75337. doi: 10.1109/ACCESS.2023.3296221
Tunio S., Dzioba A., Dhami R., Elsayed S., Strychowsky J. E. (2023). Auto-substitutions to optimize perioperative antimicrobial prophylaxis: pre-post intervention study. Laryngoscope 133, 3403–3408. doi: 10.1002/lary.30740
UniProt. Available online at: https://www.uniprot.org/.
Verberk J. D. M., van der Kooi T. I. I., Hetem D. J., Oostdam E. W. M., Noordergraaf M., de Greeff S. C., et al. (2023a). Semiautomated surveillance of deep surgical site infections after colorectal surgeries – a multicenter external validation of two surveillance algorithms. Infect. Control. Hosp. Epidemiol. 44, 616–623. doi: 10.1017/ice.2022.147
Verberk J. D. M., van der Werff S. D., Weegar R., Henriksson A., Richir M. C., Buchli C., et al. (2023b). The augmented value of using clinical notes in semi-automated surveillance of deep surgical site infections after colorectal surgery. Antimicrob. Resist. Infect. Control 12, 117. doi: 10.1186/s13756-023-01316-x
Vermassen J., Colpaert K., De Bus L., Depuydt P., Decruyenaere J. (2020). Automated screening of natural language in electronic health records for the diagnosis septic shock is feasible and outperforms an approach based on explicit administrative codes. J. Crit. Care 56, 203–207. doi: 10.1016/j.jcrc.2020.01.007
WHO. (2003). Available online at: https://www.who.int/standards/classifications/other-classifications/international-classification-of-primary-care.
WHOCC (2023). Structure and principles. Available online at: https://www.whocc.no/atc/structure_and_principles/ (Accessed cited 2023 Dec 21).
WHOCC - ATC/DDD Index (2023). Available online at: https://www.whocc.no/atc_ddd_index/ (Accessed cited 2023 Dec 21).
Wilkinson M. D., Dumontier M., Aalbersberg I. J., Appleton G., Axton M., Baak A., et al. (2016). The FAIR Guiding Principles for scientific data management and stewardship. Sci. Data 3, 160018. doi: 10.1038/sdata.2016.18
Wulff A., Baier C., Ballout S., Tute E., Sommer K. K., Kaase M., et al. (2021). Transformation of microbiology data into a standardised data representation using OpenEHR. Sci. Rep. 11, 10556. doi: 10.1038/s41598-021-89796-y
Yigzaw K. Y., Budrionis A., Marco-Ruiz L., Henriksen T. D., Halvorsen P. A., Bellika J. G. (2020). Privacy-preserving architecture for providing feedback to clinicians on their clinical performance. BMC Med. Inform Decis. Mak 20, 116. doi: 10.1186/s12911-020-01147-5
Zaman G., Mahdin H., Hussain K., Rahman A. (2020). Information extraction from semi and unstructured data sources: A systematic literature review. ICIC Express Lett. 14, 593–603. doi: 10.24507/icicel.14.06.593
Keywords: antibiotic resistance, antimicrobial stewardship, automated extraction, EHR, LIS
Citation: Cappello A, Murgia Y, Giacobbe DR, Mora S, Gazzarata R, Rosso N, Giacomini M and Bassetti M (2024) Automated extraction of standardized antibiotic resistance and prescription data from laboratory information systems and electronic health records: a narrative review. Front. Antibiot. 3:1380380. doi: 10.3389/frabi.2024.1380380
Received: 01 February 2024; Accepted: 26 February 2024;
Published: 08 March 2024.
Edited by:
Stephen Henry Gillespie, University of St Andrews, United KingdomReviewed by:
Juan Vazquez-Lago, Servicio Gallego de Salud, SpainCopyright © 2024 Cappello, Murgia, Giacobbe, Mora, Gazzarata, Rosso, Giacomini and Bassetti. This is an open-access article distributed under the terms of the Creative Commons Attribution License (CC BY). The use, distribution or reproduction in other forums is permitted, provided the original author(s) and the copyright owner(s) are credited and that the original publication in this journal is cited, in accordance with accepted academic practice. No use, distribution or reproduction is permitted which does not comply with these terms.
*Correspondence: Daniele Roberto Giacobbe, ZGFuaWVsZXJvYmVydG8uZ2lhY29iYmVAdW5pZ2UuaXQ=
†These authors have contributed equally to this work and share first authorship
‡These authors have contributed equally to this work and share last authorship
Disclaimer: All claims expressed in this article are solely those of the authors and do not necessarily represent those of their affiliated organizations, or those of the publisher, the editors and the reviewers. Any product that may be evaluated in this article or claim that may be made by its manufacturer is not guaranteed or endorsed by the publisher.
Research integrity at Frontiers
Learn more about the work of our research integrity team to safeguard the quality of each article we publish.