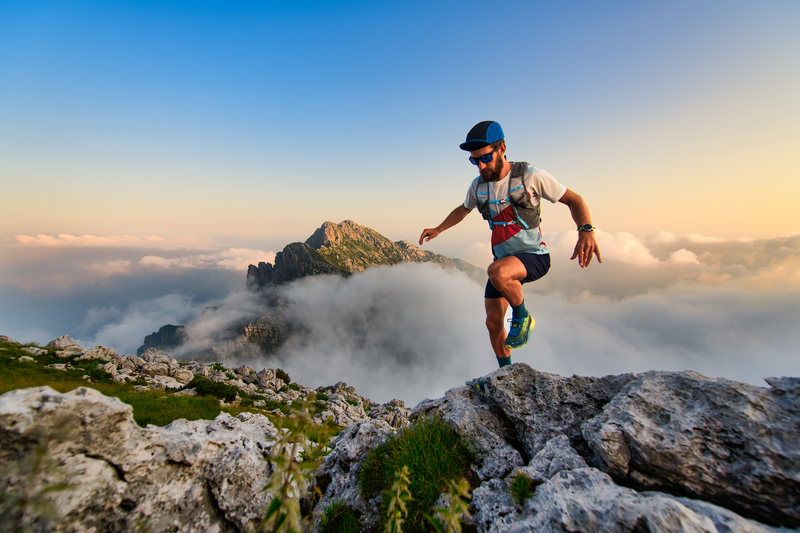
95% of researchers rate our articles as excellent or good
Learn more about the work of our research integrity team to safeguard the quality of each article we publish.
Find out more
ORIGINAL RESEARCH article
Front. Public Health , 04 April 2025
Sec. Public Health and Nutrition
Volume 13 - 2025 | https://doi.org/10.3389/fpubh.2025.1574499
This article is part of the Research Topic Transforming Food Systems: Addressing Malnutrition and Inequality in Low- and Middle-Income Countries View all 18 articles
Objective: Using longitudinal data from a study conducted in an area of high socioeconomic vulnerability in Brazil, we examined the long-term effects of food insecurity (FI) and social determinants on body weight gain (body weight, BMI) and metabolic risk (waist circumference - WC, waist-to-height ratio - WHtR) over 11 years of follow-up, conducted between 2011 and 2022.
Methods: Face-to-face household interviews were conducted using the Brazilian Food Insecurity Scale to measure FI, and anthropometric measurements of weight, height, and WC were taken. Data analysis was performed in STATA 15.0 using multilevel mixed-effects regression with covariate adjustment and predicted marginal means with marginal differences.
Results: Among the 210 individuals followed over 11 years, high prevalence of FI was observed (2011: 51.8%; 2022: 45.9%), central adiposity (2011: 83.8%; 2022: 88.6%), as well as a significant increase in the prevalence of high BMI (2011–2022: +16.7 p < 0.00), general obesity (2011–2022: +15.2 p < 0.00), and abdominal obesity (2011–2022: +0.5 p 0.02) over time. Multivariate analysis showed a positive association between BMI and body weight with mild, moderate, and severe FI after 8 and 11 years of follow-up among adults. A progressive increase in predicted body weight and BMI scores was observed among adults, with an increase of +5.6 (p 0.02) and + 2.3 (p 0.01) at the end of the follow-up, respectively, being higher in individuals with severe FI compared to those with food security. Among people ≥60 years old, WC and WHtR mean varied according to time and FI categories, with a positive association observed in mild and moderate FI, and an inverse association with severe FI at the end of the follow-up.
Conclusion: FI is a risk factor for long-term weight gain and obesity, particularly in vulnerable populations, highlighting the need for intersectoral public policies to ensure food and nutrition security, combat obesity, and combat the structural causes of poverty and FI.
The alarming rise in the prevalence of excess weight, including overweight and obesity, is a major global health crisis (1, 2). The population living with excess weight is concentrated mainly in low-and middle-income countries (LMICs) (3, 4). Environmental, social, nutritional, political, and commercial determinants of health and socioeconomic status contribute to the increased occurrence of excess weight in vulnerable populations in LMICs (5–7). Thus, people living in contexts of high socioeconomic vulnerability are at greater risk of excess weight and obesity (8, 9). Food insecurity (FI) is an economic and social condition characterized by limited or uncertain access to adequate food. FI is an indicator of socioeconomic vulnerability and inadequate access to adequate food (10). It remains a severe social and health problem worldwide, with a progressive rise in Latin America and the Caribbean, especially in South America (11–13).
FI is a potential risk factor for adult malnutrition (14, 15) and has been associated with obesity in many countries (14, 16–19), including Brazil (20–22). The association of FI-obesity is complex. This association is related, in particular, to malnutrition and the affordability of energy-dense foods (14, 23). The deprivation and difficulty in accessing adequate foods among people on FI lead to increased consumption of lower-cost foods with high caloric density, such as processed and ultra-processed foods (14, 23). These factors are intensified by social, economic, and regional inequalities (14), especially in LMICs (12). Poverty is a critical factor that contributes to FI and directly triggers the consumption of unhealthy diets. Consequently, FI and poverty contribute to the rise in the prevalence of excess weight and increased metabolic risk (12, 23, 24).
In Brazil, the Brazilian Institute of Geography and Statistics showed that 27.6% of Brazilian households (21.6 million Brazilians) were in FI in 2023, with 9.4% (7.4 million Brazilians) in moderate/severe FI (25). At the same time, according to the VIGITEL system, in 2023, 24.3% of adult Brazilians were living with obesity, with obesity rates being higher among adults with less education and in lower-income families, especially in the lower social classes (26).
Mitigating FI and obesity are targets of the Millennium Development Goals of the United Nations 2030 Agenda (27). Studies about the FI-obesity association have failed to explain the mediatory mechanisms of the relationship (14). The research cross-sectional models do not allow for exploring pathways and mechanisms underlying the relationship between FI and obesity, such as time of exposure to FI (14). Therefore, longitudinal studies are necessary.
In this article, using longitudinal data from an 11-year study conducted in an area of high socioeconomic vulnerability in Northeast Brazil, we examined the long-term effect of FI and social determinants on body weight gain and metabolic risk, using body weight, BMI, waist circumference, and waist-to-height ratio indicators in adults and people ≥60 years old.
We used data from the study “Effect of public policies on the food insecurity situation of families in the semi-arid northeast: cohort study, 2011–2022 (SANCuite cohort)” carried out by the Nutrition and Public Health Studies and Research Group (Núcleo PENSO), which monitored families in the municipality of Cuité, semi-arid region of the state of Paraíba, Brazil, for 11 years, between 2011 and 2022. The municipality selected for the research, Cuité, like approximately 70% of Brazilian cities; Cuité has approximately 20,000 inhabitants who face challenges related to water scarcity and the region’s low social and economic development (28). It is located in the semi-arid region of Northeast Brazil, in Paraíba, an area of extreme socioeconomic and climatic vulnerability. Due to these characteristics, the municipality was chosen to conduct the study.
The cohort began in 2011 (baseline), with a random and representative sample of the urban and rural population calculated based on an estimated prevalence of FI of 50%, a confidence interval of 95%, and a sampling error of 5%. Further details on the cohort baseline are available in Palmeira, Costa-Salles, and Perez-Escamilla (29). The follow-ups were performed in 2014, 2019, and 2022 via returns to the households of families surveyed at the baseline. The cohort consisted of adults (20–59 years old) and older people (≥ 60 years old). Pregnant women were excluded. At the end of data collection in 2022, 210 individuals were studied in all years of field research and comprised the sample of this study. The cohort of individuals had a sample loss of 40.7% (Figure 1).
Figure 1. Flowchart of the sample of individuals from the SANCuite cohort, Cuité, Brazil, 11 years of follow-up (2011–2022).
The primary outcome variables are body weight (kg), BMI (kg/m2), waist circumference (WC) (cm), waist-to-height ratio (WHtR), high BMI, general obesity, abdominal obesity risk, abdominal obesity, and central adiposity. Body weight, height, and WC were collected following WHO recommendations (30). All anthropometric measurements were performed in duplicate, and the mean of the two measurements was used for analysis.
High BMI (yes/no) was defined as the BMI of overweight or obesity (31). According to WHO recommendations (31), overweight and obesity were defined for adults as BMI between 25.0–29.9 kg/m2 and 30 kg/m2 and more, respectively; for people ≥60 years old, overweight was defined as BMI ≥ 27 kg/m2 (32) and obesity as ≥30 kg/m2 (31). General obesity (yes/no) was defined as a BMI of 30 kg/m2 and more (31). Abdominal obesity risk was defined according to cutoff points for WC as Low risk (WC < 94 for men and < 80 for women) and High risk (WC ≥ 94 for men and ≥ 80 for women) (33). Abdominal obesity (yes/no) was defined as WC ≥ 102 cm for men and ≥ 88 cm for women [33 Central adiposity (yes/no) was a WHtR >0.5 (34, 35)]. We used WC, WHtR, abdominal obesity risk, abdominal obesity, and central adiposity as proxy for metabolic risk.
The Multiple imputation technique imputed 11.4% (n = 24) of missing weight, WC, and BMI data. WC data collection began in the first follow-up of the cohort (2014) and was not collected at baseline; Thus, missing WC data (n = 210) at baseline (2011) were also imputed. Time, height, sex, and age were control variables in the imputation model for body weight and BMI, while time, sex, and age were used for WC.
The FI level was the independent variable used in the present study. This variable was measured using the Brazilian Food Insecurity Scale (EBIA), a method validated in Brazil in 2003 to assess household FI based on the dimension of access to food in the Brazilian population (36). The EBIA is a household experience-based scale adapted from the US Household Food Security Survey Module (36). We classified the household FI of individuals according to the sum of affirmative responses to EBIA items and household composition (36): Food security (FS) (score = 0); Mild FI (score = 1–5 in households with children/adolescents, 1–3 in adult-only households) Moderate FI (score = 6–9 in households with children/adolescents, 4–5 in adult-only households); Severe FI (score = 10–14 in households with children/adolescents, 6–8 in adult-only households).
The individuals were classified by sex (male or female), age group (adults or people ≥60 years old), skin color/race (white or black/mixed-race), high schooling (high school or more), and low schooling (up to elementary school). The households of the individuals were also characterized by housing area (urban or rural), access to cash transfer programs (Bolsa Família 2011–2021 and Auxílio Brasil 2021–2022), and monthly per capita income plus the value of cash transfer programs (above/below ½ the minimum wage). The Brazilian minimum wage was: 2011, R$ 545 ($US 340.6); 2014, R$ 724 ($US 308,1); 2019, R$ 998 ($US 253.3); and 2022, R$1212 ($US 253.3). We also use the “time” variable. The “time” variable describes the years of follow-up, which are: baseline (2011), 3 years (2014), 8 years (2019), and 11 years (2022). These covariates were used for the analyses and defined based on studies about the FI and the FI-obesity association (22, 37–39).
All statistical analyses were conducted using Stata 15.0 (40). Continuous variables are presented as means and categorical variables as proportions. Simple and average frequencies were estimated for each year of follow-up in the study: baseline (2011), 3 years (2014), 8 years (2019), and 11 years (2022). We applied the Cochran Q test to assess significant changes over time for category variables. Repeated Measures Analysis of Variance (ANOVA) was used to analyze how the means of the outcome variables vary over the follow-up period within each age group. The model analyzed was as follows: “by Age group, sort: ANOVA outcome-variable id time, repeated(time).” Mixed-effects Analysis was used to investigate how FI and time variables, both in their direct effects and interactions, influence the outcome variables.
We conducted a Multilevel Mixed-effects Regression of Body weight, BMI, WC, and WHtR with FI over time, adjusted by sex, income, and an interaction term between FI, age group, and time (FI#age#time). Next, the predicted marginal means for the outcome variables were estimated based on the time and FI variables, stratified by age group (adults and people ≥60 years old). The predicted means for each outcome were plotted separately. Differences between the means obtained over time were estimated, as well as comparisons between mild, moderate, and severe FI with the food security (FS) category, using the contrast command, and this allowed for the assessment of the effect of each level of FI and time on the outcome variables.
All multivariate models were estimated with robust variance adjustment and by considering unstructured covariance when tested, and the models did not exhibit collinearity. The sample size of this study can detect a true odds ratio of at least 2.42 with a 95% confidence level and 80% power (1-beta). Results were reported as significant with a p < 0.05.
The studied population showed a higher prevalence of females (87.6%), black/mixed-race individuals (70.0%), and urban residents (71.4%, 2011–2014: +2.9%). The proportion of people ≥60 years old, with higher education and income above ½ minimum wage, increased over the follow-up period. FI was also observed to persist over time, with a decrease from baseline (2011: 51.8%) during the first 8 years of follow-up (2019: 34.8%) and a subsequent increase in the final year (2022: 45.9%) (Table 1).
Table 1. Socioeconomic characteristics and food insecurity status in individuals over time, SANCuité cohort, Brazil, 11 years of follow-up (840 observations).
In Figure 2, the means of body weight, BMI, WC, and WHtR were plotted according to the years of follow-up, showing a significant increase in these scores over time in the adult group. A progressive increase in the prevalence of high BMI (+16.7%, p < 0.00) and general obesity (+15.2%, p < 0.00) was observed over time. A prevalence of over 70% of high abdominal obesity risk was observed in the population, with these rates being maintained throughout the follow-up period. The prevalence of abdominal obesity showed fluctuations in proportions, with an increase from baseline (53.3%) to after 8 years of follow-up (61.4%), followed by a reduction in the last follow-up (2022: 53.8%, p = 0.02). A prevalence of over 80% of central adiposity was also observed in the population, with these rates being maintained over time (Table 2).
Figure 2. Means of body weight, BMI and metabolic risk from 11 years of follow-up, by age groups. (A) Body weight; (B) Body mass index (BMI); (C) Waist circumference (WC); (D) Waist-to-height ratio. p calculated by repeated measures ANOVA. *p < 0.000. **p < 0.05.
Table 2. Prevalence of high BMI, obesity and metabolic risk in individuals over time, SANCuité cohort, Brazil, 11 years of follow-up (840 observations).
When comparing the means of body weight, BMI, WC, and WHtR according to FI categories, we found an association of Mild FI with body weight (p = 0.012) and BMI (p = 0.009) after 8 years of follow-up and an association of Severe FI with BMI after 8 years (p = 0.039) and 11 years of follow-up (p = 0.021), when compared to the reference group (FS at baseline). The means of WC and WHtR did not show an association with FI (Supplementary Table 1).
Table 3 presents the results of the multivariate mixed-effects analysis of the association between outcome variables and FI, including an interaction term with time by age group. Figure 3 displays the predicted means for body weight, BMI, WC, and WHtR over time by age group, considering the results of the association contrast.
Table 3. Multilevel mixed-effects regression of body weight, WC, waist-to-height, and body max index with food insecurity over time, SANCuité cohort, Brazil, 2011–2022 (840 observations).
Figure 3. Change in the predicted mean of BMI, body weight and metabolic risk over time according to food insecurity, by age groups: Cuité, Brazil, 11 years of follow-up. Mixed-effects logistic regression, margms predicted by age groups and contrast of predicted margins. Significant contrast results are shown (p < 0.05).
For body weight, a positive association was observed with all categories of FI in the later years of follow-up among adults. In contrast, for people ≥60 years old, this association was observed only with Moderate FI (Table 3). The predicted means for body weight in adults were higher in the Mild FI category (+3.9 kg at 8 years of follow-up, p 0.005) when compared to the Food security category. In the Severe FI category, this difference was even more significant and progressively increased in the later years of follow-up (+5.1 kg to 8 years, p 0.007, and + 5.6 kg to 11 years, p 0.02).
For BMI, the data also showed a positive association with all categories of FI in the later years of follow-up among adults. For people ≥60 years old, this association was observed only in the Mild FI category (Table 3). The predicted means for BMI in adults were 1.8 units higher in the Mild FI category (p 0.002) and 2.3 units higher in the Severe FI category (p 0.01) after 8 years of follow-up, compared to the Food Security category (Figure 3).
A positive association between WC and Mild and Severe FI was observed in the later years of follow-up among adults. In contrast, for people ≥60 years old, this association was observed with all categories of FI in the later years of follow-up (Table 3). The predicted means for WC showed no differences. A positive association between WHtR and Mild and Severe FI was observed in the later years of follow-up among adults. For people ≥60 years old, an association between WHtR and all categories of FI was found in the later years of follow-up (Table 3). The predicted means for WHtR in people ≥60 years old were lower in the Severe FI category (−0.13, p 0.04) compared to the Food Security category after 11 years of follow-up. Notably, all predicted means for WHtR were above 0.5, the cutoff point indicating central adiposity (Figure 3).
All these variations in means had their statistical significance confirmed by contrasts of predictive margins. Thus, our results indicate that, among adults in a state of FI, the predicted means for body weight and BMI progressively increased over time and with the severity of FI (Figure 3).
The results of this study, conducted with a population exposed to vulnerabilities and deprivation over 11 years in the semi-arid region of one LMIC, revealed weight gain and increases in average BMI scores, leading to a rise in the prevalence of obesity and the persistence of high metabolic risk and central adiposity in the studied population over time. Long-term exposure to mild and severe FI was associated with increased BMI and body weight in the adult population but not among people ≥60 years old.
The long-term effect of FI is a significant risk factor for body weight gain and increased metabolic risk. It serves as an essential determinant for the development of obesity, as observed in this longitudinal cohort study. Previous cross-sectional studies have reported an association between FI and adult obesity (16, 17, 41, 42). In the context of LMICs, longitudinal studies about the long-term effects of FI on obesity are scarce yet, especially in adults. Nevertheless, evidence from cross-sectional studies conducted in Mexico (18, 43), Brazil (20, 21, 44), Ethiopia (45), and Iran (19), suggested that individuals facing FI are more likely to develop overweight and obesity. This is particularly evident in regions and populations with low socioeconomic status and food access disparities.
In the US, longitudinal studies have demonstrated a positive association of household FI (46), high cumulative social risk (including FI) (47), and economic adversity (income, FI, debt, and housing insecurity) (48) with increases in BMI scores over time in children. On the other hand, in a context where the population involved in the research received financial support from government agencies to cope with FI, Huang et al. found no association between increased BMI and FI in economically vulnerable adolescents in Taiwan. In this case, the possible improvement in food security status blocked the impact on the increase in BMI over the time of the study (49).
In US adults, Lohman et al. found a positive association between FI during adolescence and elevated BMI 16 years later in women (50). In a prospective cohort matched for age, sex, race/ethnicity, and BMI, Cheung et al. found that baseline FI was associated with more significant BMI gains after 3.2 years of follow-up in adults attending a community health center in Massachusetts (51). In a longitudinal study conducted in Minnesota, Hooper et al. demonstrated that individuals who lived in households with FI during adolescence had higher elevated BMI scores after 8 years of follow-up compared to those in households with food security and suggested that a household FI experienced during adolescence predisposes to the incidence of elevated BMI in adulthood (52).
Concerning this, the impacts of FI on body weight gain and metabolic risk depend on factors such as the duration and severity of exposure to FI and the socioeconomic and environmental context. Body weight gain and metabolic risk are highly associated with physical activity. Since FI is a condition of social vulnerability, it is important to raise the hypothesis that in families living with moderate and severe FI, regular physical activity may not be a priority when there is food deprivation. National data on the practice of physical activity in the capitals of Brazil showed that 37% of the individuals in the population studied did not achieve a sufficient level of physical activity, and it is important to highlight that physical activity decreases as the level of education decreases (26). Data from a systematic review of Brazilian studies on FI-Obesity raised the hypothesis of an association between FI and Obesity and NCDs precisely because of the presence of a sedentary lifestyle in contexts of social vulnerability (22).
Another factor highly associated with obesity is stress. Physiological responses to chronic stress can increase cortisol levels and influence body fat distribution, incredibly visceral adipose tissue. Excessive visceral fat accumulation in the abdominal region increases the risk of adverse health outcomes, such as obesity, cardiovascular disease, and diabetes (4, 8). Thus, prolonged stress associated with persistent FI can lead to changes in metabolic functioning, promoting abdominal fat accumulation. In addition, FI can impact the intestinal microbiota, which can also contribute to weight gain (4, 8, 53).
Over time, families exposed to prolonged FI develop and resort to strategies to ensure survival and access to food, consequently managing persistent FI. These strategies result in lower consumption of fresh and minimally processed foods and increased consumption of processed and ultra-processed foods (16, 53, 54); these food-related decisions are primarily based on the cost of foods (55). As a result, these families consume diets of low nutritional quality and with reduced dietary diversity (54, 56). Furthermore, the dynamics of FI experience over time, with prolonged periods of food deprivation and unpredictable eating patterns, contribute to the emergence of stress states that promote the development of obesity (57–59). These factors, combined with the chronic stress of living with food deprivation (60–62), may explain the tendency for individuals experiencing FI to gain weight over time. Thus, the dynamics of FI over time lead to the chronicity of exposure to risk factors associated with living in FI, contributing to the long-term effect of FI in the development of obesity and increased metabolic risk.
Therefore, our findings reveal insights into the long-term effects of FI on weight gain and metabolic risk and suggest mechanisms that may play a role in the association between FI and obesity.
This study has important implications for future weight management interventions in individuals in the context of FI and social inequalities. Obesity within the context of FI adds a layer of complexity to the current obesity epidemic (14). Thus, the long-term effects of FI on the risks of developing obesity among FI families should be considered. Therefore, the challenge of FI-obesity needs intersectoral care approaches. Health promotion, prevention, and treatment actions for overweight and obesity must be supported by intersectoral actions and policies to promote food and nutritional security and reduce social inequalities.
To face obesity in the context of FI and social inequalities involves considering a food system-level approach that efficiently addresses systemic and environmental determinants (63). The food environment provides the context for food choices; therefore, system changes are necessary to ensure that families can afford foods that promote a healthy diet. Concerning this issue, one of the primary objectives of facing obesity is to increase the consumption of fresh and minimally processed foods while reducing the consumption of processed and ultra-processed foods within the population. Achieving this requires coordinated actions and policies across agriculture, social assistance, and the economy to ensure the availability and accessibility of fresh and minimally processed foods for the population.
Brazil has a network of policies, programs, and actions aimed at reducing FI and obesity. Some examples include the National Food and Nutrition Policy (PNAN), which addresses obesity as both a risk factor and a disease and proposes improvements in food, nutrition, and health conditions, as well as ensuring the food and nutrition security of the Brazilian population (64), and the National Food and Nutrition Security Policy (PNSAN), an intersectoral policy with the perspective of adequate and healthy food, which treats obesity as a social problem and FI and proposes new ways to produce, market, and consume food to modify eating practices (65).
In this regard, a prominent program is the National School Feeding Program (PNAE), which provides free daily meals to students in Brazilian public schools. The meal preparation guidelines prioritize consuming fresh or minimally processed foods, purchasing products from family farming, and restricting ultra-processed foods (limited to 15%) (66). In addition, Brazil has invested in a set of guidance materials for the population and public managers, such as the Dietary Guidelines for the Brazilian population (67) and the Reference Framework for Food and Nutrition Education for Public Policies (68), as well as measures that promote access to adequate and healthy food, and consequently, the reduction of FI and obesity. These include the decree on the composition of the new Basic Food Basket and the reduction of taxes on products from the new Basic Food Basket, which excludes ultra-processed foods (69, 70).
Evidence shows that the food environment mediates the association between FI-obesity (51, 71), especially the accessibility of fresh produce in the neighborhood (72). Another critical aspect of the food environment concerns the places where people in FI purchase food. In Brazil, nationally, most food groups are predominantly purchased at supermarkets. Nonetheless, households experiencing moderate or severe FI rely more on small markets for essential items such as beans, rice, proteins, and ultra-processed foods. These findings highlight the need for public policies to improve vulnerable populations’ food access (73).
Therefore, policy efforts to mitigate obesity among families with FI should focus on the availability and affordability of neighborhood fresh produce and the social environment in which communities are embedded. Income access, stability, and food costs are key determinants of the FI-obesity relationship (62). Findings indicate that individuals facing FI-obesity food decisions are primarily based on food costs and influenced by family income levels, highlighting income as a barrier to healthy eating (51, 55). In this context, income redistribution policies and reducing prices for fresh and minimally processed foods are necessary.
Furthermore, evidence shows that FI reduces the effectiveness of interventions and treatments to reduce overweight and obesity (74). Thus, the findings of this study are essential for shaping care pathways for individuals living with overweight and obesity. The development of these care pathways should consider the effects of FI on the development of overweight and obesity. It should be grounded in an intersectoral approach, encompassing the sectors of social assistance, education, economy, and agriculture. In this sense, food and nutritional surveillance is crucial to face FI-obesity. Screening for FI has the potential to identify individuals and families at risk of increased BMI, as it serves as an indicator of inadequate access to proper alimentation and the risk of obesity, thereby enabling their inclusion in intersectoral care pathways.
By demonstrating the long-term effect of FI on increased body weight and BMI in a population experiencing socioeconomic vulnerability, this study highlights the need for a sustained and targeted set of intersectoral public policies to combat hunger, promote health, and reduce obesity in vulnerable areas.
We acknowledge that the limitations of this study may include the absence of predictive and confounding variables, such as physical activity, stress, sleep quality, blood pressure, lipid profile, and related biochemical markers associated with obesity. In our study, it was impossible to collect these variables due to logistical data collection issues in remote regions and the availability of financial resources. However, we emphasize that weight, height, and circumference measurements have been widely used in population studies precisely because of the feasibility of implementation in data collection, which justified the choice of these variables. Future longitudinal studies should consider these variables when examining and controlling the analysis of the FI-obesity association.
However, this is the first study to analyze the FI-obesity association longitudinally in a population of one LMIC. The study’s strengths include its longitudinal approach and the 11-year follow-up period, which allows for an in-depth analysis of changes over time, which helps to understand the long-term effects of FI on obesity. Another strength concerns the geographic and sociodemographic context in which the study was conducted, an area of high socioeconomic vulnerability in Northeastern Brazil. Finally, this study helps to address significant scientific gaps regarding the FI-obesity association, particularly in terms of its temporal dynamics. It may contribute to the development of public policies to mitigate this issue.
Food insecurity is a risk factor for weight gain and increased obesity and metabolic risk over time, particularly in populations in contexts of socioeconomic vulnerability. The long-term effects of FI significantly impact weight gain, eating behaviors, and psychosocial and metabolic factors. Therefore, this issue must be faced through intersectoral public policies focused on ensuring food and nutrition security and combating obesity. Furthermore, such policies must address the structural causes of poverty and FI to promote long-term health. Longitudinal cohort study can attest to the findings of the current study.
The raw data supporting the conclusions of this article will be made available by the authors, without undue reservation.
The studies involving humans were approved by the Comitê de Ética em Pesquisa do Hospital Universitário Alcides Carneiros da Universidade Federal de Campina Grande (CAAE: 30919314.6.0000.5182). The studies were conducted in accordance with the local legislation and institutional requirements. The participants provided their written informed consent to participate in this study.
JL: Conceptualization, Data curation, Formal analysis, Investigation, Methodology, Project administration, Resources, Software, Supervision, Validation, Visualization, Writing – original draft, Writing – review & editing. IM-C: Visualization, Writing – original draft, Writing – review & editing. RO-S: Data curation, Visualization, Writing – original draft, Writing – review & editing. AS: Data curation, Visualization, Writing – original draft, Writing – review & editing. PP: Conceptualization, Formal analysis, Funding acquisition, Investigation, Methodology, Supervision, Validation, Visualization, Writing – original draft, Writing – review & editing.
The author(s) declare that financial support was received for the research and/or publication of this article. This work was funded by the Chamada CNPq/MCTI/FNDCT No. 18/2021 - Universal and Edital No. 010/2021 - FAPESQ/PB - MCTIC/CNPq Programa de Infraestrutura para Jovens Pesquisadores/Programa Primeiros Projetos - PPP, in addition to funding for scholarships for postgraduate authors by the Coordenação de Aperfeiçoamento de Pessoal de Nível Superior (CAPES). The publication of this article was received financial support by the Chamada CNPq/MCTI/FNDCT No. 18/2021 - Universal and Edital n° 38/2022 (Promoção e Fortalecimento aos Programas de Pós-graduação consolidados em Saúde e bem-estar do Estado da Paraíba).
The authors declare that the research was conducted in the absence of any commercial or financial relationships that could be construed as a potential conflict of interest.
The authors declare that no Gen AI was used in the creation of this manuscript.
All claims expressed in this article are solely those of the authors and do not necessarily represent those of their affiliated organizations, or those of the publisher, the editors and the reviewers. Any product that may be evaluated in this article, or claim that may be made by its manufacturer, is not guaranteed or endorsed by the publisher.
The Supplementary material for this article can be found online at: https://www.frontiersin.org/articles/10.3389/fpubh.2025.1574499/full#supplementary-material
1. Lin, X, and Li, H. Obesity: epidemiology, pathophysiology, and therapeutics. Front Endocrinol. (2021) 12:706978. doi: 10.3389/fendo.2021.706978
2. World Health Organization. Obesity. Health topics (2024). Available online at: https://www.who.int/health-topics/obesity (Accessed February 2024).
3. World Obesity Federation. World obesity atlas 2024. (2024) 1–235. Available online at: https://data.worldobesity.org/publications/WOF-Obesity-Atlas-v7.pdf (Accessed February 2024).
4. Zhang, X, Ha, S, Lau, HCH, and Yu, J. Excess body weight: novel insights into its roles in obesity comorbidities. Semin Cancer Biol. (2023) 92:16–27. doi: 10.1016/j.semcancer.2023.03.008
5. Jastreboff, AM, Kotz, CM, Kahan, S, Kelly, AS, and Heymsfield, SB. Obesity as a disease: the Obesity Society 2018 position statement. Obesity. (2019) 27:7–9. doi: 10.1002/oby.22378
6. Ford, ND, Patel, SA, and Narayan, KM. Obesity in low- and middle-income countries: burden, drivers, and emerging challenges. Annu Rev Public Health. (2017) 38:145–64. doi: 10.1146/annurev-publhealth-031816-04460
7. Miranda, JJ, Barrientos-Gutiérrez, T, Corvalan, C, Hyder, AA, Lazo-Porras, M, Oni, T, et al. Understanding the rise of cardiometabolic diseases in low- and middle-income countries. Nat Med. (2019) 25:1667–79. doi: 10.1038/s41591-019-0644-7
8. Kwarteng, JL, Schulz, AJ, Mentz, GB, Israel, BA, and Perkins, DW. Independent effects of neighborhood poverty and psychosocial stress on obesity over time. J Urban Health. (2017) 94:791–802. doi: 10.1007/s11524-017-0193-7
9. Anekwe, CV, Jarrell, AR, Townsend, MJ, Gaudier, GI, Hiserodt, JM, and Stanford, FC. Socioeconomics of obesity, Socioeconomics of obesity. Curr Obes Rep. (2020) 9:272–9. doi: 10.1007/s13679-020-00398-7
10. Food and Agriculture Organization. The state of food insecurity in the world, economic crises – impacts and lessons learned. (2009) 1–59. Available online at: https://www.fao.org/3/i0876e/i0876e00.htm (Accessed February 2024).
11. FAO, IFAD, UNICEF, WFP, WHO. The state of food security and nutrition in the world 2022. Repurposing food and agricultural policies to make healthy diets more affordable. Rome: FAO (2022).
12. FAO, IFAD, UNICEF, WFP and WHO. The State of Food Security and Nutrition in the World 2023. Urbanization, agrifood systems transformation and healthy diets across the rural–urban continuum. Rome: FAO (2023) 1–283.
13. Kantilafti, M, Giannakou, K, and Chrysostomou, S. Multimorbidity and food insecurity in adults: a systematic review and meta-analysis. PLoS One. (2023) 18:e0288063. doi: 10.1371/journal.pone.0288063
14. Carvajal-Aldaz, D, Cucalon, G, and Ordonez, C. Food insecurity as a risk factor for obesity: a review. Front Nutr. (2022) 9:1012734. doi: 10.3389/fnut.2022.1012734
15. Brandt, EJ, Mozaffarian, D, Leung, CW, Berkowitz, SA, and Murthy, VL. Diet and food and nutrition insecurity and cardiometabolic disease. Circ Res. (2023) 132:1692–706. doi: 10.1161/CIRCRESAHA.123.322065
16. Rezaei, M, Ghadamgahi, F, Jayedi, A, Arzhang, P, Yekaninejad, MS, and Azadbakht, L. The association between food insecurity and obesity, a body shape index and body roundness index among US adults. Sci Rep. (2024) 14:23631. doi: 10.1038/s41598-024-74108-x
17. Van der Velde, LA, Nyns, CJ, Engel, MD, Neter, JE, van der Meer, IM, Numans, ME, et al. Exploring food insecurity and obesity in Dutch disadvantaged neighborhoods: a cross-sectional mediation analysis. BMC Public Health. (2020) 20:569. doi: 10.1186/s12889-020-08611-x
18. Ponce-Alcala, RE, Ramirez-Garcia Luna, JL, Shamah-Levy, T, and Melgar-Quiñonez, H. The association between household food insecurity and obesity in Mexico: a cross-sectional study of ENSANUT MC 2016. Public Health Nutr. (2021) 24:5826–36. doi: 10.1017/S1368980021003153
19. Hamedi-Shahraki, S, Mir, F, and Amirkhizi, F. Food insecurity and cardiovascular risk factors among Iranian women. Ecol Food Nutr. (2021) 60:163–81. doi: 10.1080/03670244.2020.1812596
20. Schlüssel, MM, Silva, AA, Pérez-Escamilla, R, and Kac, G. Household food insecurity and excess weight/obesity among Brazilian women and children: a life-course approach. Cad Saude Publica. (2013) 29:219–26. doi: 10.1590/s0102-311x2013000200003
21. Domingos, TB, Sichieri, R, and Salles-Costa, R. Sex differences in the relationship between food insecurity and weight status in Brazil. Br J Nutr. (2022) 129:345–51. doi: 10.1017/S0007114522001192
22. Laurentino, JSL, Brito, RCS, Oliveira-Silva, RT, Soares, A, Pereira, TC, Lima, EM, et al. Association between food insecurity and chronic noncommunicable diseases in Brazil: a systematic review. Rev Bras Epidemiol. (2024) 27:e240041. doi: 10.1590/1980-549720240041
23. Nkambule, SJ, Moodley, I, Kuupiel, D, and Mashamba-Thompson, TP. Association between food insecurity and key metabolic risk factors for diet-sensitive non-communicable diseases in sub-Saharan Africa: a systematic review and meta-analysis. Sci Rep. (2021) 11:5178. doi: 10.1038/s41598-021-84344-0
24. Ferreira, SRG, Macotela, Y, Velloso, LA, and Mori, MA. Determinants of obesity in Latin America. Nat Metab. (2024) 6:409–32. doi: 10.1038/s42255-024-00977-1
25. Brazilian Institute of Geography and Statistics. Continuous National Household Sample Survey (PNAD): food security. (2024) 1–26. Available online at: https://www.ibge.gov.br/liv102084.pdf (Accessed May 2024).
26. VIGITEL System. Surveillance of risk and protective factors for chronic diseases by telephone survey: estimates of frequency and sociodemographic distribution of risk and protective factors for chronic diseases in the capitals of the 26 Brazilian states and the Federal District in 2023. Brazilian Ministry of Health; (2023). 131. Available online at: https://www.gov.br/saude/pt-br/centrais-de-conteudo/publicacoes/svsa/vigitel/vigitel-brasil-2023-vigilancia-de-fatores-de-risco-e-protecao-para-doencas-cronicas-por-inquerito-telefonico (Accessed February 2024).
27. The Millennium Development Goals. (2023). Available online at: https://www.un.org.br/objetivos-de-desenvolvimento-do-milenio (Accessed March 2024).
28. Brazilian Institute of Geography and Statistics. Panorama of cities. (2023) 1–26. Available online at: https://cidades.ibge.gov.br/brasil/pb/cuite/panorama (Accessed February 2024).
29. Palmeira, PA, Salles-Costa, R, and Pérez-Escamilla, R. Effects of family income and conditional cash transfers on household food insecurity: evidence from a longitudinal study in Northeast Brazil. Public Health Nutr. (2019) 23:756–67. doi: 10.1017/S1368980019003136
31. World Health Organization. Obesity: Preventing and managing the global epidemic: Report of a WHO consultation. Report no. 894. Geneva: WHO (2000).
33. Klein, S, Allison, DB, Heymsfield, SB, Kelley, DE, Leibel, RL, Nonas, C, et al. Waist circumference and cardiometabolic risk: a consensus statement from shaping America's health: Association for Weight Management and Obesity Prevention; NAASO, the Obesity Society; the American Society for Nutrition; and the American Diabetes Association. Am J Clin Nutr. (2007) 15:1061–7. doi: 10.1093/ajcn/85.5.1197
34. National Institute for Health and Care Excellence. Obesity: identification, assessment and management. London: National Institute for Health and Care Excellencer (2023).
35. Associação Brasileira para o Estudo da Obesidade e da Síndrome Metabólica. Diretrizes brasileiras de obesidade 2016. 4th ed. São Paulo, SP: ABESO (2016).
36. Segall-Corrêa, AM, Marin-León, L, Melgar-Quiñonez, H, and Pérez-Escamilla, R. Refinement of the Brazilian household food insecurity measurement scale: recommendation for a 14-item EBIA. Rev Nutr. (2014) 27:241–51. doi: 10.1590/1415-52732014000200010
37. Dhurandhar, EJ. The food-insecurity obesity paradox: a resource scarcity hypothesis. Physiol Behav. (2016) 162:88–92. doi: 10.1016/j.physbeh.2016.04.025
38. Lignani, JDB, Palmeira, PDA, Antunes, MML, and Salles-Costa, R. Relationship between social indicators and food insecurity: a systematic review. Rev Bras Epidemiol. (2020) 23:e200068. doi: 10.1590/1980-549720200068
39. Te Vazquez, J, Feng, SN, Orr, CJ, and Berkowitz, SA. Food insecurity and cardiometabolic conditions: a review of recent research. Current nutrition reports. (2021) 10:243–54. doi: 10.1007/s13668-021-00364-2
41. Yau, A, White, M, Hammond, D, White, C, and Adams, J. Socio-demographic characteristics, diet and health among food insecure UK adults: cross-sectional analysis of the international food policy study. Public Health Nutr. (2020) 23:2602–14. doi: 10.1017/S1368980020000087
42. Fernandes, SG, Rodrigues, AM, Nunes, C, Santos, O, Gregório, MJ, de Sousa, RD, et al. Food insecurity in older adults: results from the epidemiology of chronic diseases cohort study 3. Front Med (Lausanne). (2018) 5:203. doi: 10.3389/fmed.2018.00203
43. Arredondo, A, Torres, C, Orozco, E, Pacheco, S, Huang, F, Zambrano, E, et al. Socio-economic indicators, dietary patterns, and physical activity as determinants of maternal obesity in middle-income countries: evidences from a cohort study in Mexico. Int J Health Plann Manag. (2019) 34:e713–25. doi: 10.1002/hpm.2684
44. Velásquez-Melendez, G, Schlüssel, MM, Brito, AS, Silva, AA, Lopes-Filho, JD, and Kac, G. Mild but not light or severe food insecurity is associated with obesity among Brazilian women. J Nutr. (2011) 141:898–902. doi: 10.3945/jn.110.135046
45. Yosef, T, Sebeta, A, Tekalign, E, Sisay, BG, Bekele, BB, Tesfaw, A, et al. Assessment of abdominal obesity prevalence and determinants among adults in Southwest Ethiopia: a cross-sectional study. Front Public Health. (2024) 12:1400066. doi: 10.3389/fpubh.2024.1400066
46. Metallinos-Katsaras, E, Must, A, and Gorman, K. A longitudinal study of food insecurity on obesity in preschool children. J Acad Nutr Diet. (2012) 112:1949–58. doi: 10.1016/j.jand.2012.08.031
47. Suglia, SF, Duarte, CS, Chambers, EC, and Boynton-Jarrett, R. Cumulative social risk and obesity in early childhood. Pediatrics. (2012) 129:e1173–9. doi: 10.1542/peds.2011-2456
48. Schuler, BR, Vazquez, CE, Kobulsky, JM, and Dumenci, L. Adversity and child body mass index in fragile families over 15 years: do type and timing matter? SSM Popul Health. (2022) 19:101197. doi: 10.1016/j.ssmph.2022.101197
49. Huang, YC, Tan, CX, Lee, CT, and Tsai, MC. Relationships between food insecurity, self-efficacy, and changes in body mass index among the youth in Taiwan: analysis from a longitudinal cohort survey. Children. (2024) 11:663. doi: 10.3390/children11060663
50. Lohman, BJ, Neppl, TK, Lee, Y, Diggs, ON, and Russell, D. The association between household food insecurity and body mass index: a prospective growth curve analysis. J Pediatr. (2018) 202:115–120.e1. doi: 10.1016/j.jpeds.2018.05.052
51. Cheung, HC, Shen, A, Oo, S, Tilahun, H, Cohen, MJ, and Berkowitz, SA. Food insecurity and body mass index: a longitudinal mixed methods study, Chelsea, Massachusetts, 2009-2013. Prev Chronic Dis. (2015) 12:E125. doi: 10.5888/pcd12.150001
52. Hooper, L, Mason, SM, Telke, S, Larson, N, and Neumark-Sztainer, D. Experiencing household food insecurity during adolescence predicts disordered eating and elevated body mass index 8 years later. J Adolesc Health. (2022) 70:788–95. doi: 10.1016/j.jadohealth.2021.11.026
53. Bateson, M, and Pepper, GV. Food insecurity as a cause of adiposity: evolutionary and mechanistic hypotheses. Philos Trans R Soc Lond Ser B Biol Sci. (2023) 378:20220228. doi: 10.1098/rstb.2022.0228
54. Taylor, EA, Foster, JS, and Mobley, AR. Examining factors related to the food insecurity-obesity paradox in low-income mothers and fathers. Food Nutr Bull. (2021) 42:309–16. doi: 10.1177/03795721211011133
55. Puddephatt, JA, Keenan, GS, Fielden, A, Reaves, DL, Halford, JCG, and Hardman, CA. 'Eating to survive': a qualitative analysis of factors influencing food choice and eating behaviour in a food-insecure population. Appetite. (2020) 147:104547. doi: 10.1016/j.appet.2019.104547
56. Langer, B, Gupta, RK, Kumari, R, and Mahajan, R. Food insecurity and its association with dietary diversity: a cross-sectional study in rural households in Jammu. Indian J Community Med. (2024) 49:70–5. doi: 10.4103/ijcm.ijcm_811_22
57. Arenas, DJ, Thomas, A, Wang, J, and DeLisser, HM. A systematic review and Meta-analysis of depression, anxiety, and sleep disorders in US adults with food insecurity. J Gen Intern Med. (2019) 34:2874–82. doi: 10.1007/s11606-019-05202-4
58. Gooding, HC, Walls, CE, and Richmond, TK. Food insecurity and increased BMI in young adult women. Obesity (Silver Spring). (2012) 20:1896–901. doi: 10.1038/oby.2011.233
59. Ling, J, Robbins, LB, and Xu, D. Food security status and hair cortisol among low-income mother-child dyads. West J Nurs Res. (2019) 41:1813–28. doi: 10.1177/0193945919867112
60. Leung, CW, Fulay, AP, Parnarouskis, L, Martinez-Steele, E, Gearhardt, AN, and Wolfson, JA. Food insecurity and ultra-processed food consumption: the modifying role of participation in the supplemental nutrition assistance program (SNAP). Am J Clin Nutr. (2022) 116:197–205. doi: 10.1093/ajcn/nqac049
61. Thomas, MK, Lammert, LJ, and Beverly, EA. Food insecurity and its impact on body weight, type 2 diabetes, cardiovascular disease, and mental health. Curr Cardiovasc Risk Rep. (2021) 15:15. doi: 10.1007/s12170-021-00679-3
62. Briggs, R, Rowden, H, Lagojda, L, Robbins, T, and Randeva, HS. The lived experience of food insecurity among adults with obesity: a quantitative and qualitative systematic review. J Public Health (Oxf). (2024) 46:230–49. doi: 10.1093/pubmed/fdae016
63. Heeren, FAN, Darcey, VL, Deemer, SE, Menon, S, Tobias, D, and Cardel, MI. Breaking down silos: the multifaceted nature of obesity and the future of weight management. Philos Trans R Soc Lond Ser B Biol Sci. (2023) 378:20220215. doi: 10.1098/rstb.2022.0215
64. National Food and Nutrition Policy. Ministry of Health, Secretariat of Health Care. Department of Primary Care. 1st ed. Brasília: Ministry of Health (2013). 84 p.
65. Dias, PC, Henriques, P, Anjos, LAD, and Burlandy, L. Obesidade e políticas públicas: concepções e estratégias adotadas pelo governo brasileiro. Cad Saude Publica. (2017) 33:e00006016. doi: 10.1590/0102-311X00006016
66. Resolution CD/FNDE No. 3, of February 4, 2025. Amends resolution CD/FNDE no. 6, of May 8, 2020, which provides for the provision of school meals to students in basic education under the National School Feeding Program (PNAE). Official gazette of the union. (2025). Available online at: https://www.in.gov.br/en/web/dou/-/resolucao-cd/fnde-n-3-de-4-de-fevereiro-de-2025-*-611861131 (Accessed March 2025).
67. Dietary Guidelines for the Brazilian population. Ministry of Health, Department of Primary Health Care, secretariat of health care. 2nd ed. Brasília: Ministry of Health (2014). 156 p.
68. Reference Framework for Food and Nutrition Education for Public Policies. Ministry of Social Development and Fight against hunger. Brasília, DF: MDS; National Secretariat for Food and Nutrition Security (2012). 68 p.
69. Decree No. 11.936, of March 5, 2024. Establishes the composition of the basic food basket within the scope of the National Food and nutrition security policy and the National Food Supply Policy. Official Gazette of the Union. (2024). Available online at: https://www.planalto.gov.br/ccivil_03/_ato2023-2026/2024/decreto/d11936.htm (Accessed March 2025).
70. Complementary Bill No. 68, of 2024. Establishes the tax on goods and services (IBS), the social contribution on goods and services (CBS), the selective tax (IS), and other provisions. Official gazette of the union. (2024). Available online at: https://www.planalto.gov.br/CCIVIL_03/Projetos/Ato_2023_2026/2024/PLP/plp-068.htm (Accessed March 2025).
71. Sanjeevi, N, Freeland-Graves, J, and Hersh, M. Food insecurity, diet quality and body mass index of women participating in the supplemental nutrition assistance program: the role of intrapersonal, home environment, community and social factors. Appetite. (2018) 125:109–17. doi: 10.1016/j.appet.2018.01.036
72. Ro, A, and Osborn, B. Exploring dietary factors in the food insecurity and obesity relationship among Latinos in California. J Health Care Poor Underserved. (2018) 29:1108–22. doi: 10.1353/hpu.2018.0082
73. de Oliveira, RT, Castro Junior, PCP, Suéte Matos, YAC, Ferreira, AA, and Salles-Costa, R. Food acquisition locations and food groups acquired according to levels of food insecurity in Brazil. Int J Environ Res Public Health. (2024) 21:1577. doi: 10.3390/ijerph21121577
Keywords: food insecurity, weight gain, obesity, social vulnerability, low socioeconomic status, cohort studies, Brazil
Citation: Laurentino JSL, Martins-Costa IMdO, Oliveira-Silva RTd, Santos ABMVd and Palmeira PdA (2025) Long-term effect of food insecurity on body weight gain and metabolic risk in a context of high socioeconomic vulnerability in a medium-income country: the SANCuité cohort, Brazil, 2011–2022. Front. Public Health. 13:1574499. doi: 10.3389/fpubh.2025.1574499
Received: 10 February 2025; Accepted: 24 March 2025;
Published: 04 April 2025.
Edited by:
Catarine Santos Da Silva, Universidade Federal do Rio Grande do Norte, BrazilReviewed by:
Diresibachew Haile Wondimu, The Addis Clinic, United StatesCopyright © 2025 Laurentino, Martins-Costa, Oliveira-Silva, Santos and Palmeira. This is an open-access article distributed under the terms of the Creative Commons Attribution License (CC BY). The use, distribution or reproduction in other forums is permitted, provided the original author(s) and the copyright owner(s) are credited and that the original publication in this journal is cited, in accordance with accepted academic practice. No use, distribution or reproduction is permitted which does not comply with these terms.
*Correspondence: Jackson Silva Lima Laurentino, amFja3Nvbm51dEBnbWFpbC5jb20=
Disclaimer: All claims expressed in this article are solely those of the authors and do not necessarily represent those of their affiliated organizations, or those of the publisher, the editors and the reviewers. Any product that may be evaluated in this article or claim that may be made by its manufacturer is not guaranteed or endorsed by the publisher.
Research integrity at Frontiers
Learn more about the work of our research integrity team to safeguard the quality of each article we publish.