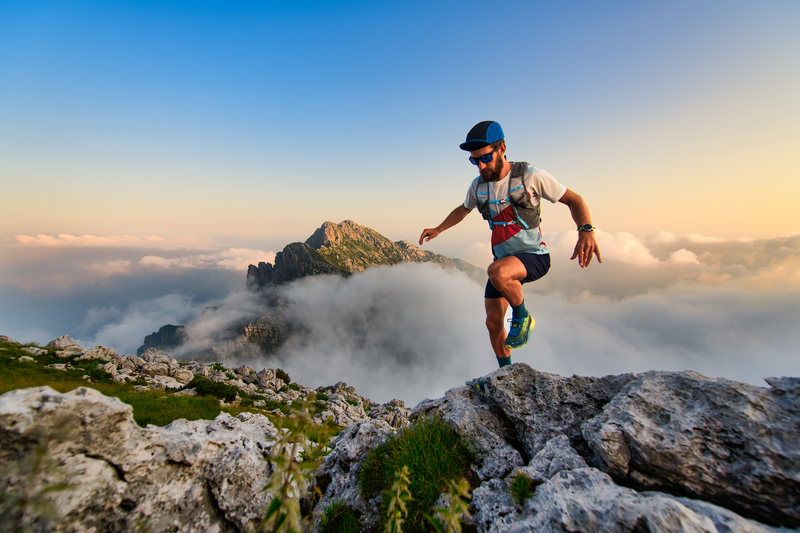
95% of researchers rate our articles as excellent or good
Learn more about the work of our research integrity team to safeguard the quality of each article we publish.
Find out more
ORIGINAL RESEARCH article
Front. Public Health , 21 February 2025
Sec. Public Health Policy
Volume 13 - 2025 | https://doi.org/10.3389/fpubh.2025.1546402
This article is part of the Research Topic Co-Creating Future Social Services View all 9 articles
Objective: The continuous increase in health care costs and the growing demand for health services among residents make it an urgent priority to improve the operational efficiency of the health system in Chinese society. In this study, data on the operational efficiency of tertiary public hospitals in Guangxi were analyzed to identify issues in hospital management within the context of performance assessment, which thereby enhanced the social service capacity of hospitals.
Methods: A comprehensive evaluation index system was constructed based on the “national monitoring indicators” of operational efficiency. The indicators were analyzed using data envelopment analysis with Banker, Charnes and Cooper (DEA-BCC) and Malmquist index models. The Tobit regression model was used to analyze the major factors affecting the efficiency of public hospitals.
Results: Between 2019 and 2022, the pure technical efficiency (PTE) of 61 tertiary public hospitals in Guangxi remained at a relatively high level. The results of the Malmquist index showed a downward trend. The technical progress (TC) indicator became the main factor affecting the decline in the operational efficiency of hospitals. Tobit regression analysis revealed that plenty of factors exerted a significant impact on the operating efficiency of hospitals. These factors included the number of beds, the ratio of outpatient and inpatient patients relative to total patient numbers, the proportion of discharged patients undergoing surgery among total patients, business expenditures and total annual revenue.
Conclusion: The scale of tertiary public hospitals in Guangxi is prominently unreasonable. It is necessary to raise the efficiency of resource utilization. The operation and management situation is not optimistic. Hospitals should accelerate the transformation of their development model, rationally allocate medical resources and shift from scale expansion to the improvement of quality and efficiency. Meanwhile, they should actively participate in establishing the hierarchical medical treatment system, controlling operating costs and reasonably increasing the proportion of personnel expenses to improve operational and management efficiency.
Some countries including China are facing the dual challenges of rising health care costs and low hospital efficiency. Specifically, China is currently dealing with an accelerating aging population, a slowing population growth rate and a shift in the disease spectrum, all of which are driving an increasing demand for health services of high quality. Public hospitals, the primary providers of social health services, bear tremendous pressure in response to the growing demand for these services. Research by Hu et al. (1) reported that the health resource allocation and service provision in China are inefficient. The inequitable distribution of medical resources has potentially threatened social stability. Therefore, policymakers need to improve the utilization efficiency of health resources to enhance the social service capabilities of hospitals. Strengthening hospital operations management is crucial to ensure efficient medical resource utilization, achieve refined hospital management and promote a transition from scale expansion to quality and efficiency-oriented development. In 2019, a unified performance assessment indicator system for tertiary public hospitals was set up for the first time, which marked the beginning of a nationwide performance evaluation of these hospitals. The performance assessment of tertiary public hospitals serves as a “guiding rod” for the development of public hospitals. It is of great significance to expedite the establishment of a tiered diagnosis and treatment system, build a modern hospital management system and enhance the operational management capabilities of hospitals. In 2020, the “Guiding Opinions on Strengthening the Operational Management of Public Hospitals” was issued by the National Health Commission of China and the National Administration of Traditional Chinese Medicine. This document highlighted that strengthening the operational management of public hospitals is an important means of leading high-quality hospital development with new development concepts, deepening comprehensive reforms in public hospitals and alleviating economic operational pressure in these hospitals. This policy further elevated the operational management of public hospitals to a strategic development level. Health resources are significantly fewer in the western region of China compared with other regions (2). In Western China, hospitals have weaker operational management capabilities. It is of great importance to promote national unity with many methods. Such methods involve using limited health resource inputs to meet the growing demand for health services while keeping pace with deepening medical reforms, updating internal hospital management systems, addressing internal operational management weaknesses (3) and fairly allocating health resources. This is also a key issue of common research interest in both the Chinese academia and political arena.
At present, data envelopment analysis (DEA) is the most extensively used method for studying and evaluating the operational efficiency of hospitals (4). It was proposed by Charnes, a renowned American operations researcher, and other scholars in 1978, and later introduced by Nunamaker to the field of hospital management (5). This method can consider a variety of input and output indicators simultaneously and avoid evaluation difficulties caused by different units of measurement. Internationally, scholars have examined hospital efficiency from various dimensions. In terms of research at the national level, Afonso and Aubyn (6) used DEA to assess the health efficiency of 30 Organization for Economic Cooperation and Development (OECD) countries and considered efficiency scores with environmental variables. Top et al. (7) evaluated the efficiency of health systems in 36 African countries. Regarding research within the same country but across different regions, Mazon (8) assessed the technical efficiency of public health expenditures in the municipalities of Santa Catarina and their connection with health management outcomes. Ngobeni (9) measured and compared the technical efficiency of healthcare delivery across the nine provinces of South Africa. Bates (10) used DEA and multiple regression analysis to empirically examine the impact of multiple market structure factors on the technical efficiency of hospital services in major United States (US) metropolitan areas. Ferreira et al. (11–13) applied extended DEA models like log-DEA and bootstrap to analyze the Portuguese health care system. From the perspective of health resource control: Zhang et al. (14) analyzed tertiary public hospitals in nine provinces along the Yellow River and found that the Coronavirus Disease 2019 (COVID-19) pandemic greatly affected technological changes in hospitals. They proposed that government departments reasonably control the flow of health resources and that hospitals enhance the application of technology. Li et al. (15) statically analyzed 30 hospitals in Shanxi and concluded considerable redundancy in hospital inputs, which suggested a need to transform hospital management models to improve operational efficiency. From the angle of scale efficiency (SE): He et al. (16) analyzed tertiary public hospitals in Heilongjiang and concluded that “SE” is the primary factor causing the imbalance of hospital development. There is a need to further improve operational efficiency and avoid the unregulated expansion of hospitals. Qin et al. (17) analyzed the cross-sectional data of public hospitals in Hunan by use of the DEA-Tobit model and emphasized the necessity of improving the efficiency of public hospitals and strictly controlling the scale of tertiary hospitals.
This study is the first to combine the “national monitoring indicators” from the “Performance Assessment of Tertiary Public Hospitals” to investigate and evaluate the operational efficiency of hospitals in the western region of China. To be specific, it makes the following contributions: Firstly, existing studies have all focused on the overall level of a region and provided limited guidance for individual hospitals despite having used the DEA or Malmquist model to evaluate public hospitals. This study breaks away from the integrative evaluation framework of other papers and evaluates Guangxi tertiary public hospitals from a micro perspective. It presents the evaluation results of 61 hospitals, identifies hospitals with significant issues, and offers valuable insights into the management practices of other hospitals. Secondly, qualitative (18) and quantitative (19, 20) approaches were combined to select indicators. This scientific method avoids the pitfalls of relying on a single approach. Thirdly, based on DEA, the Tobit model was employed to perform regression analysis on the factors influencing comprehensive efficiency (CE), pure technical efficiency (PET) and SE. This provides a more comprehensive analysis and control of real-world issues affecting the operational efficiency of public hospitals. The present study provides evidence-based support for gradually improving the operational management model of tertiary public hospitals in the western border regions of China and promoting their high-quality social health services.
The data were obtained from the statistical yearbooks and financial statements of 61 third-tier public hospitals located in the Guangxi Zhuang Autonomous Region and from the “Performance Evaluation of Third-Tier Public Hospitals” covering the years 2019–2022. The sample comprised 44 general hospitals and 17 specialized hospitals. Among them, 20 provincial hospitals, 36 municipal hospitals and five county-level hospitals were included. The sampled hospitals were systematically coded from H1 to H61.
According to an important empirical rule of DEA theory, the number of indicators in DEA studies should be considered concerning the number of decision-making units (DMUs). The inclusion of too many indicators in the model can lead to insensitive efficiency results, low differentiation and ineffective evaluations. Thus, the number of DMUs is supposed to be at least two to three times the sum of input and output indicators (19, 20). Based on the three-dimensional approach of human resource, physical resource and cost inputs developed by Ozcan et al. (21), an input indicator library related to operational efficiency from the “National Tertiary Public Hospital Performance Assessment Manual (2024 Edition)” was constructed in this study, as detailed in Table 1 (22–27). Cluster analysis was used to evaluate the indicators in the input indicator library (Figure 1), to minimize the collinearity effects among indicators of the same type (18, 28). Ultimately, it was concluded that the indicator representing the human resource input dimension is X3: the ratio of doctors to nurses; the physical resource input dimension is denoted by X4: the number of hospital beds; the cost input dimension is indicated by X8: average outpatient medical expenses per visit; X9 represents average outpatient drug expenses per patient. Output indicators were selected based on the “National Tertiary Public Hospital Performance Assessment Manual (2024 Edition) (29).” Revenue and expenditure structure and cost control were used as second-level indicators, and nine national monitoring indicators were taken as third-level indicators (Table 2). The DEAP 2.1 software was utilized for analyzing the input–output indicators of sample hospitals, and the operational efficiency of tertiary public hospitals in Guangxi was evaluated systematically. Additionally, indicators that may affect the static efficiency of hospitals were chosen based on the four dimensions of operational efficiency, sustainable development, medical quality and satisfaction from the “National Assessment” (Table 3). Tobit regression analysis was performed using the Stata 18 software (30–34).
Figure 1. Clustering results of input indicator library. The Y-axis from 1 to 12 corresponds to the indicators X1 to X12 in the indicator library, respectively.
Table 2. Input-output indicator system for operational efficiency of tertiary public hospitals in Guangxi.
Table 3. Estimation results of static efficiency of 61 third-tier public hospitals in Guangxi province using Tobit model.
In the context of DEA analysis, it is essential to conduct positive and standardized processing when interval and negative indicators within the input–output indicator system are addressed. The majority of articles in this field employ the reciprocal method to transform negative indicators into positive ones. This non-linear approach has the potential to alter the distribution characteristics of original data (35). Hence, the Min-Max reverse indicator formula (Equation 1) was used in this study to process negative indicators (36), where Xij stands for the original value of the j indicator for the i evaluation object. The medical surplus rate and asset-liability ratio were processed using the interval-type attribute standardization method with the standardization formula (Equation 2) (16). In the formula, [a,b] represents the optimal attribute interval; stands for the intolerable lower limit; denotes the intolerable upper limit, with converted maximum value being 1 and the minimum value being 0.
Common DEA static models include the Charnes, Cooper and Rhodes (CCR) model measuring constant returns to scale (CRS) and the Banker, Charnes and Cooper (BCC) model assessing variable returns to scale (VRS). Health production theory suggests that the production technology within health systems is characterized by VRS. Consequently, the BCC model was used in the current study for static analysis of sample hospitals (37). The BCC model enables the calculation of CE and returns to scale (RTS) in hospital operations. CE can be further decomposed into PTE and SE, which is represented by the equation CE = PTE × SE. The BCC model incorporates convexity constraints and reflects its assumption of VRS, which is opposite to the CCR model. Below is the linear programming formulation (Equation 3):
The optimal solution of the linear programming problem is described as follows:
If 0 = 1 and =0, =0, the DMU DMUj0 is DEA efficient. In this case, its production activities have SE and technical efficiency.
If 0 = 1 and s + >0, DMUj0 is slightly inefficient according to DEA. In this case, its production activities are not simultaneously efficient in terms of SE and technical efficiency.
When 0 < 1, DMUj0 is considered DEA inefficient. In this case, its production activities have no SE and technical efficiency.
In 1953, the Malmquist index was initially introduced by Sten Malmquist, a Swedish economist, to analyze variations in consumption indices across different periods (38). Subsequently, this concept was adapted by Caves for production analysis, to measure dynamic efficiency changes in production activities. On this basis, Fare and colleagues developed the Malmquist index by employing the geometric average of the indices from two consecutive periods to assess the trend of total factor productivity (TFP) changes from a specific period t to t + 1 (39). The model is represented as follows (Equation 4).
TFP is influenced simultaneously by two key factors: technical efficiency change (TEC) and technology progress (TC), which can be expressed as TFP = TC × TEC. Furthermore, TEC can be further broken down into the product of PTE change (PTEC) and SE change (SEC). Thus, the expression for TFP can also be articulated as TFP = TC × PTEC × SEC.
The Malmquist index has a threshold value of 1:
M = 1 indicates that efficiency remains unchanged.
M > 1 indicates that the efficiency in period t + 1 is on an upward trend compared to that in period t.
M < 1 indicates that the efficiency in period t + 1 is on a downward trend compared to that in period t.
In the process of performing regression analysis, continuous dependent variables may sometimes be constrained to a specific range due to truncation or censoring, which can result in inconsistent estimators. As defined by Davidson et al., truncation means the systematic exclusion of certain observations from the sample, whereas censoring refers to a scenario where no observations are excluded but some are limited to a particular threshold. Both truncated and censored variables are collectively termed restricted dependent variables (40, 41). Also called the censored or truncated regression model, the Tobit model is frequently employed in regression analyses involving restricted variables. Its fundamental form is represented as follows (Equation 5):
In this study, dependent variables including CE, PTE and SE exhibited a value range of (0, 1), and were categorized as censored data. Consequently, the Tobit model was employed for analyzing the influence factors for the static efficiency of sample hospitals and exploring both the direction and magnitude of these effects. The model can be formulated as follows (Equation 6):
Where EFFit represents the operational efficiency of the pilot hospital; α stands for the constant term; β denotes the coefficient for the impact of independent variables on efficiency; i refers to the serial number of each observation (1, 2, 3, …, 61); t indicates the year (2019, 2020, 2021 and 2022); ϵ is the random disturbance term.
As shown in Table 4, CE serves as an indicator of the production capacity of a hospital within the healthcare industry (42). From 2019 to 2022, the proportion of DEA-effective hospitals (those with a CE value of 1) among the 61 tertiary public hospitals in Guangxi was recorded at 49.18, 31.15, 36.07 and 39.34%, respectively, which demonstrated an initial decline followed by a gradual recovery trend. Only 12 hospitals (19.67%) maintained DEA-effectiveness throughout the four years, including six in Nanning, two in Liuzhou and one each in Wuzhou, Yulin, Baise and Laibin. Notably, over 80% of these hospitals did not operate on the efficient frontier, which indicated the failure of their resource inputs to achieve optimal output levels relative to best practices. PTE, which assumes CRS, quantifies the gap between the production capacity and the frontier of a hospital. This metric is an indicator of both technological proficiency and managerial competence. Over four years, 14 hospitals (22.95%) achieved a DEA value of 1 for PTE. Moreover, 27 hospitals experienced fluctuations in performance but successfully returned to the production frontier in 2022. The overall strong performance of the sampled hospitals was closely tied to the robust initiatives of the region that were aimed at advancing healthcare system reforms. Given the current level of technology, SE assesses the disparity between the actual and optimal production scale of a hospital. This metric is instrumental in determining whether a hospital should expand or contract its operations to enhance operational efficiency (43). During the investigation period, 36 hospitals (59.01%) exhibited a fluctuating decline in SE. The proportion of hospitals demonstrating DEA-inefficient SE was recorded at 50.82, 68.85, 63.93 and 60.66% over the four years. Of note, only 12 hospitals (19.67%) achieved a DEA value of 1 for SE throughout this period, which underscored the issue of irrational production scale within hospitals. Decreasing Returns to Scale (DRS) refers to the variation in output that occurs when the proportions of various production factors within a hospital are altered, while other conditions remain constant. In the past four years, the number of hospitals experiencing DRS was recorded as 31 (50.82%), 36 (59.02%), 39 (63.93%) and 35 (57.38%), respectively, which indicated a need for adjustments in their production scale.
The trend of mean values for SE, PTE and CE from 2019 to 2022 is illustrated in Figure 2.
Table 4. Static decomposition of 61 tertiary public hospitals in Guangxi from 2019 to 2022 unit: [n(%)].
Overall, from a dynamic perspective, the Malmquist index decomposition indicators for sample hospitals from 2019 to 2022 demonstrated an initial increasing trend followed by a subsequent decline, as illustrated in Table 5 and Figure 3. The values for technical efficiency, TC, PTE and TFP that experienced a decrease from 2021–2022 compared to the period from 2020–2021 were recorded at 0.054, 0.373, 0.062 and 0.433, respectively. This suggests that resources are still significantly underutilized and a marked regression remains in comprehensive management and technological capabilities within the operation and management of tertiary public hospitals in Guangxi. Although SE was maintained at a level of 1, neglecting proper attention and effective control may result in subsequent issues concerning the rationality of the hospital production scale. From a microscopic perspective, 19 hospitals (31.15%) had TFP exceeding 1 over the observed years. Hospitals that demonstrated improvement solely attributable to the TC indicator included H6, H21, H32, H44, H50 and H59. Of the 42 hospitals exhibiting a TFP of less than 1, 13 (30.95%) experienced a decline in their TFP that was solely ascribed to a decrease in the TC indicator. Despite maintaining all other indicators at or above 1, six hospitals had a TFP of less than 1 owing to the influence of the TC indicator. The aforementioned situation reveals that the primary issue contributing to the decline in the operational management of third-tier public hospitals in Guangxi was significantly influenced by the TC indicator. Figure 4 displays this phenomenon in detail. Hospitals H9 and H35 had all indicators less than 1, which necessitated an increased focus on operational management.
Table 5. Malmquist index decomposition of 61 tertiary public hospitals in Guangxi from 2019 to 2022.
Figure 3. Dynamic efficiency value decomposition from 2019 to 2022 for Guangxi’s 61 tertiary public hospitals (EC stands for technical efficiency, TC for technological change, PTEC for pure technical efficiency, SE for scale efficiency, and TFP for total factor productivity).
Figure 4. Changes in TFP and relative efficiency in secondary tertiary hospitals in Guangxi province from 2019 to 2022.
In this study, the likelihood ratio (LR) test was conducted on the CE, PTE and SE values of 61 tertiary public hospitals in Guangxi. The p-values of CE, PTE and SE were 0.029, 0.000 and 0.023, respectively, all of which were below 0.05. This indicates that a mixed-effects Tobit model should be established. The results of the regression analysis on the influencing factors for CE indicated that variables such as bed size scale, the ratio of outpatient to inpatient patients and the percentage of discharged patients undergoing surgery within the dimension of functional positioning were statistically significant in the sample model. In specific terms, both the hospital scale and the ratio of outpatient to inpatient patients exerted a positive influence, whereas the percentage of discharged patients undergoing surgery had a negative effect. This suggests that tertiary hospitals should actively adjust their patient demographics and attach importance to treating patients requiring high-difficulty surgeries. From the perspective of operational efficiency, it was revealed in this study that business expenses exerted a negative influence on the CE of hospitals, whereas total annual revenue had a positive effect. These findings indicate that hospitals should prioritize controlling medical activity costs while increasing revenue and minimizing expenses. The results of the regression analysis on the factors influencing PTE indicated that the effects of all other indicators were not statistically significant except the bed size scale within the dimension of functional positioning. The results of the regression analysis on the factors influencing SE indicated that all the indicators within the dimension of functional positioning exhibited statistical significance for the sample model. Concretely, both the bed size scale and the ratio of outpatient to inpatient patients had a positive impact, while jurisdictional level and the percentage of discharged patients undergoing surgery among discharged patients exerted a negative influence. In the dimension of operational efficiency indicators, the business expenditure metric exhibited a negative impact, while the total annual revenue metric demonstrated a positive influence. The effects of sustainable development and satisfaction dimension indicators on SE showed no statistical significance. Please refer to Table 3 for further details.
The results of the BCC static model demonstrated that the fluctuation and decline in CE among sample hospitals were primarily driven by SE from 2019 to 2022. From the micro perspective of various DMUs, SE emerged as the main factor influencing overall efficiency. Among hospitals with a CE value of less than 1 during this period, those rendered DEA-ineffective because of invalid SE were numbered 27, 12, 30 and 29, respectively, for each year, which accounted for 87.10, 28.57, 76.92 and 78.38%, respectively. Except for the year 2020, these proportions consistently exceeded 75%. Furthermore, more than half of DEA-ineffective hospitals observed over these years operated under DRS, which indicated a certain risk of excessive expansion among tertiary public hospitals in Guangxi. This result was consistent with that reported by Kirigia and Asbu, who suggested that most hospitals suffer from low efficiency on account of inappropriate scale (44). A detailed analysis of specific survey data revealed that some hospitals saw a continuous increase in inputs like the number of healthcare personnel and actual open bed counts in several years. However, key output indicators including the proportion of medical service revenue, debt-to-asset ratio, medical surplus rate and the proportion of personnel expenditures did not improve alongside hospital size expansion or increased resource input. With the expansion of the hospital scale, operational costs also increased.
Therefore, it is critical to fully leverage the “conductor’s baton” role of performance assessment policies in tertiary public hospitals. This can promote a shift in the development model of public hospitals from scale expansion to quality and efficiency and in their management model from extensive administrative management to comprehensive performance management.
The Malmquist dynamic analysis results indicated that the TFP of 61 public tertiary hospitals in Guangxi was primarily influenced by a combination of TC, TEC and SEC. From a positive perspective, it can be observed that 31.59% of the hospitals experienced an increase in TFP solely due to improvements in the TC indicator. Conversely, from a negative standpoint, 30.95% of the hospitals saw a decline in TFP exclusively owing to changes in the TC indicator. It is worth noting that none of the TEC, PTEC and SEC independently contributed to a decline in the TFP of these hospitals. Despite the progress made by hospitals H2, H11, H14, H23, H25 and H30 in other indicators, the decline in the TC index led to a decrease in TFP. This indicates that the TC index is crucial for improving the operational efficiency of tertiary public hospitals in Guangxi. It suggests that some hospitals are encountering challenges like outdated operational management techniques and methods, and the inefficient use of funds. This result was in line with that reported by Chen (45), who believed that the TC indicator should be the priority direction for improvement to improve the operational efficiency of hospitals. Through field investigations, it has been found that tertiary public general hospitals in Guangxi mostly have no separate operational departments. Instead, the financial department is primarily responsible for managing hospital operations. This arrangement makes it challenging to assign specific responsibilities to corresponding departments or individuals during actual work processes, which results in low operational efficiency and difficulties in implementing refined management practices. In addition, some hospitals are confronted with issues where existing information systems fail to satisfy operational management needs. For the time being, a majority of hospital operation management information system modules concentrate mainly on basic functions such as accounting, cost management and asset management. Only a few hospitals possess modules for risk control, operational analysis and project management related to scientific research and education. More than that, compatibility issues also exist between different systems within hospitals that pose technical barriers to the implementation of refined management practices.
In light of the aforementioned issues, on the one hand, healthcare institutions ought to establish a structured operational management system and standardize the creation of operational management departments to enhance operational efficiency. On the other hand, they must advance the informatization of operational management and facilitate a transition from the traditional “process-driven” model to a “dual-driven” approach integrating both data and processes.
According to the analysis of the Malmquist dynamic model, six (31.58%) provincial-level hospitals had a TFP of greater than 1 from 2019 to 2022. At the municipal level, 11 hospitals (57.89%) achieved a TFP of greater than 1. At the county level, only two hospitals (10.53%) obtained a TFP of greater than 1. This reflects the unbalanced operational development among hospitals of different jurisdictional levels. From a micro perspective, the M index indicated that five tertiary public hospitals in Nanning four in Guilin, three in Liuzhou and two each in Hechi and Yulin across various cities had a technical efficiency value of greater than 1. Beyond that, Wuzhou, Beihai, Laibin and Guigang each have one such hospital. The hospitals in the remaining cities experienced no growth in their TEC indicators. This further demonstrates the imbalance in the operational development of tertiary public hospitals across different regions of Guangxi. The exploration of underlying factors showed that Guangxi exhibits a relatively weak economic foundation featuring disparities in economic development across multiple regions and an imbalance in health financial investment. These conditions inevitably lead to differences in the operational development of hospitals. During the observation period, Guangxi grappled with the COVID-19 pandemic, which had varying effects across different areas. The responsibilities associated with epidemic prevention and control, nucleic acid testing tasks, the consumption of protective materials and labor expenses for dispatched medical staff differ among hospitals of varying levels as well. These external factors further influence hospital operations to differing degrees. A study by Androutsou and Lupu also demonstrated that COVID-19 exerted a huge impact on the operational efficiency of hospitals (46, 47).
As a result, hospitals should start from the actual situation of resource allocation to enhance resource utilization rates. Concurrently, they must strengthen cost management and accurately control the “revenue generation” and “cost saving” of hospitals to maximize operational management benefits. In addition, it is suggested that hospital management departments consciously balance the disparities in inputs among hospitals within a region, formulate rational regional health plans, and supervise and regulate hospital operational efficiency (48).
The Tobit regression analysis results demonstrated that the proportion of surgical patients discharged from hospitals had a negative impact on the operational efficiency of 61 third-level public hospitals in Guangxi. This finding contradicts conventional empirical judgments. Nevertheless, it is important to note that all the sample hospitals included in this study are classified as third-level or above public hospitals in China, and specifically defined as institutions primarily focused on treating complex and challenging diseases and emergencies. Therefore, they need to receive patients with higher treatment difficulty (49). An investigation into the quadruple-level surgery situation of sample hospitals revealed that Hospital H7 consistently had the highest proportion of level 4 surgeries from 2019 to 2022, with ratios of 42.79, 41.71, 37.11 and 41.74%, respectively. The hospital with the lowest proportion was H42 in 2019, with a ratio of 0.05%, while Hospital H58 had the lowest proportion from 2020 to 2022, with ratios of 0.14, 0.42 and 0.37%. In 2019, 2020 and 2022, only Hospital H7 in Guangxi reached the full score value (≥ 40%) for level 4 surgeries as per the “National Performance Assessment Full Mark Value” for tertiary public hospitals. In 2021, however, no hospitals in Guangxi achieved this full score value. This indicates that the patient intake structure of hospitals and their capacity to provide high-difficulty medical services still have room for adjustment. The business expenditure indicator also had a reverse effect on the operational efficiency of sample hospitals. The business activity expenses of hospitals encompass labor wages, fixed asset depreciation, costs of drugs and consumables, official expenses, etc. When controlling business expenditures, hospitals should also abide by national guidelines and reasonably increase personnel expenditures to retain talents. Research data indicate that the hospital with the highest proportion of personnel expenditures was consistently H6 over the four years, with values of 70.52, 72.05, 74.76 and 74.12%. In 2019, the hospital with the lowest proportion of personnel expenditures was H36, with a ratio of 28.3%. In 2020, the hospital with the lowest proportion of personnel expenditures was H53, with a ratio of 29.51%. Between 2021 and 2022, the hospital with the lowest proportion of personnel expenditures was H35, with ratios of 28.65 and 31.44%, respectively. The number of hospitals failing to reach the “national performance assessment full mark value (38.09%)” for tertiary public hospitals in the four years were 34 (55.74%), 31 (51.67%), 28 (46.67%) and 18 (30.00%), respectively. While the personnel expenditures of various hospitals continued to increase, a significant portion of hospitals remained unable to achieve the full mark value set by the national assessment.
Therefore, hospitals should actively align with the tiered diagnosis and treatment system by adjusting the admission structure for surgical patients and gradually referring common and frequently occurring diseases, as well as patients in the stable or recovery phase, to lower-level medical institutions. They should also progressively lower the proportion of outpatient visits for common ailments at urban tertiary general hospitals. This is aligned with the performance assessment goals for national tertiary public hospitals (27). Meanwhile, hospitals should vigorously develop new technologies and projects, keep enhancing their capacity to provide high-complexity medical services, strengthen their radiating capabilities and improve the quality and level of social services.
This study has several limitations: First, it only secured data from 2019 to 2022, which is a relatively short period. Nonetheless, the findings remain beneficial to assessing the short-term impact of hospital inefficiency. It is necessary to monitor data over a greater number of years for further validation in the future. Second, the evaluation was confined to tertiary public hospitals, and the authors had no access to data from secondary or primary hospitals. Thus, it is essential to evaluate the operational efficiency of hospitals at other levels in future research. Third, only hospitals in Guangxi were evaluated, which limited the generalizability of the findings. This limitation is common in research, but hospitals from this province were fortunately included in this study. Moreover, the province studied represents the western regions of China in terms of average economic and social development levels. Therefore, the findings remain applicable to public hospitals in the western regions of China.
In the present study, the operational efficiency of 61 hospitals in Guangxi was analyzed, and the factors influencing the operational efficiency of hospitals were explored from macro and micro perspectives. The results indicated that tertiary public hospitals in Guangxi have relatively low overall operational efficiency, with most facing the issue of excessive scale expansion. Tobit regression analysis showed that bed size scale, the proportion of discharged patients undergoing surgery, business expenditures and total annual revenue affected hospital operational efficiency to a large extent. Public hospitals should consider the economic conditions of the region while seeking development, and ensure both efficient operation and sustainable development.
The original contributions presented in the study are included in the article/Supplementary material, further inquiries can be directed to the corresponding authors.
JH: Conceptualization, Data curation, Formal analysis, Funding acquisition, Investigation, Methodology, Project administration, Resources, Software, Supervision, Validation, Visualization, Writing – original draft, Writing – review & editing. HY: Conceptualization, Data curation, Formal analysis, Project administration, Resources, Supervision, Validation, Writing – original draft, Writing – review & editing. YW: Conceptualization, Formal analysis, Investigation, Project administration, Software, Validation, Writing – review & editing. HW: Conceptualization, Data curation, Investigation, Methodology, Software, Writing – review & editing. XL: Formal analysis, Investigation, Project administration, Resources, Validation, Writing – review & editing. BW: Data curation, Funding acquisition, Methodology, Resources, Supervision, Visualization, Writing – review & editing. MZ: Conceptualization, Investigation, Methodology, Project administration, Resources, Software, Supervision, Visualization, Writing – review & editing. PZ: Conceptualization, Data curation, Formal analysis, Funding acquisition, Investigation, Project administration, Resources, Supervision, Visualization, Writing – review & editing.
The author(s) declare financial support was received for the research, authorship, and/or publication of this article. This article has received strong support from the “Research on the Application of Medical Quality Indicators in the Performance Assessment of Tertiary Public Hospitals in Guangxi under the Background of High-Quality Development (approval number: YLZLXZ24G055)” project funded by the Hospital Management Research Institute of the National Health Commission of China.
Gratitude is extended to the School of Humanities and Social Sciences at Guangxi Medical University for providing the research platform support, and special thanks go to the Guangxi Medical Service Management Guidance Center for their data support.
The authors declare that the research was conducted in the absence of any commercial or financial relationships that could be construed as a potential conflict of interest.
The author(s) declare that no Gen AI was used in the creation of this manuscript.
All claims expressed in this article are solely those of the authors and do not necessarily represent those of their affiliated organizations, or those of the publisher, the editors and the reviewers. Any product that may be evaluated in this article, or claim that may be made by its manufacturer, is not guaranteed or endorsed by the publisher.
The Supplementary material for this article can be found online at: https://www.frontiersin.org/articles/10.3389/fpubh.2025.1546402/full#supplementary-material
1. Sun, M, Ye, Y, Zhang, G, Xue, Y, and Shang, X. Measuring the efficiency of public hospitals: a multistage data envelopment analysis in Fujian Province, China. Front Public Health. (2023) 11:1091811. doi: 10.3389/fpubh.2023.1091811
2. Liu, H, Zhang, A, and Kou, L. Research on the equity of health resource allocation in China's ethnic minority autonomous prefectures. Chin J Prev Med. (2024) 25:245–50. doi: 10.16506/j.1009-6639.2024.02.019
3. Nong, Y, Zhang, X, and Xu, H. Operational efficiency and influencing factors of tertiary public hospitals in Hainan Province. Med Soc. (2024) 37:84–9. doi: 10.13723/j.yxysh.2024.02.014
4. Emrouznejad, A, and Yang, GA. Survey and analysis of the first 40 yearsof scholarly literature in DEA:1978–2016. Soc econ plan Sci. (2018) 61:4–8. doi: 10.1016/j.seps.2017.01.008
5. Nunamaker, TR. Measuring routine nursing service efficiency:a comparison of cost per patient day and data envelopment analysis models. Health Serv Res. (1938) 18:183–208.
6. Afonso, A, and Aubyn, MS. Assessing health efficiency across countries with a two-step and bootstrap analysis. Appl Econ Lett. (2011) 18:1427–30. doi: 10.1080/13504851.2010.541149
7. Top, M, Konca, M, and Sapaz, B. Technical efficiency of healthcare systems in Africancountries:an application based on data envelopment analysis. Health Policy Technol. (2020) 9:62–8. doi: 10.1016/j.hlpt.2019.11.010
8. Mazon, LM, Freitas, SFT, and Colussi, CF. Financing and management:thetechnical efficiency in public health expenditures of small-scale municipalitiesin the state of Santa Catarina. Ciênc Saúde Colet. (2021) 26:1521–32. doi: 10.1590/1413-81232021264.09712019
9. Ngobeni, V, Breitenbach, MC, and Aye, GC. Technical efficiency ofprovincial public healthcare in South Africa. Cost Eff Resour Alloc. (2020) 18:3. doi: 10.1186/s12962-020-0199-y
10. Bates, GE. Sensitivity analysis of hospital efficiency under alternative output/input and peer groups: a review. Int J Knowl Transfer Utilis. (2006) 65:1–29.
11. Aragon, Y, Daouia, A, and Thomas-Agnan, C. Nonparametric frontier estimation: a conditional quantile based approach. Economet Theor. (2005) 21:358–89. doi: 10.1017/S0266466605050206
12. Ferreira, DC, Marques, RC, and Nunes, AM. Pay for performance in health care: a new best practice tariff-based tool using a log-linear piecewise frontier function and a dual–primal approach for unique solutions. Oper Res Int J. (2021) 21:2101–46. doi: 10.1007/s12351-019-00502-3
13. Ferreira, DC, Nunes, AM, and Marques, RC. Operational efficiency vs clinical safety, care appropriateness, timeliness, and access to health care. J Prod Anal. (2020) 53:355–75. doi: 10.1007/s11123-020-00578-6
14. Zhang, W, Li, H, and Kong, Y. Research on the operational efficiency of hospitals in the Yellow River Basin based on DEA-Malmquist index model. Hospital Manag China. (2022) 42:14–7.
15. Li, N, Li, M, and Yang, W. Evaluation of operational efficiency of tertiary general hospitals in Shanxi Province based on DEA model. Chin Hosp. (2020) 24:21–3. doi: 10.19660/j.issn.1671-0592.2020.06.06
16. He, L, Qi, Y, and Bi, J. Evaluation of hospital operation efficiency based on performance appraisal of tertiary public hospitals: a case study of Heilongjiang Province. China Health Econ. (2023) 42:65–70.
17. Qin, Y, Hu, Z, and Zhou, L. Research on the efficiency and influencing factors of public hospitals in Hunan Province——Based on DEA-Tobit model. Health Econ Res. (2022) 39:50–3. doi: 10.14055/j.cnki.33-1056/f.2022.02.016
18. Hurst, J, and Hughes, MJ. Performance Measurement and Performance Management in OECD Health Systems. Disponível em www1[J].oecd.org. OECD Health Systems. Labour Market and Social Policy -Occasional Papers, (2001), 47:1–60.
19. Chames, A, Cooper, W, and Rhodes, E. Measuring the efficiency of decision making units. Eur J Oper Res. (1978) 2:429–44. doi: 10.1016/0377-2217(78)90138-8
20. Liu, S, Zhang, H, and Chen, L. Study on the operational efficiency of public hospitals and private hospitals in Guangdong Province based on DEA-Malmquist index. Modern Prev Med. (2019) 46:3920–4.
21. Ozcan, YA. Performance measurement using data envelopment analysis (DEA)[J]. Health care benchmarking and performance evaluation: An assessment using data envelopment analysis (DEA). (2014) 15–47.
22. Ravaghi, H, Afshari, M, Isfahani, P, Mahboub-Ahari, A, and Bélorgeot, VD. Hospital efficiency in the eastern mediterranean region: a systematic review and meta-analysis. Front Public Health. (2023) 11:1085459. doi: 10.3389/fpubh.2023.1085459
23. Hofmarcher, MM, Paterson, I, and Riedel, M. Measuring hospital efficiency in Austria--a DEA approach. Health Care Manag Sci. (2002) 5:7–14. doi: 10.1023/a:1013292801100
24. Pirani, N, Zahiri, M, Engali, KA, and Torabipour, A. Hospital efficiency measurement before and after health sector evolution plan in southwest of Iran: a DEA-panel data study. Acta Inform Med. (2018) 26:106–10. doi: 10.5455/aim.2018.26.106-110
25. Lindaas, NA, Anthun, KS, and Magnussen, J. New public management and hospital efficiency: the case of Norwegian public hospital trusts. BMC Health Serv Res. (2024) 24:36. doi: 10.1186/s12913-023-10479-7
26. Lai, S, Xie, J, and Xu, H. Efficiency and influencing factors of public hospitals in Guangdong Province based on DEA-Tobit method. Chin J Soc Med. (2024) 41:758–62.
27. Yu, X, Xu, J, Liu, J, and Huang, X. Study on the operational efficiency and influencing factors of public hospitals in a province based on the DEA-Tobit model. Health Soft Sci. (2024) 38:46–50.
28. Yu, X, Liu, M, and Gao, S. Evaluation of operational efficiency and influencing factors of Jilin provincial Hospital of Traditional Chinese Medicine. Med Soc. (2023) 36:33–9. doi: 10.13723/j.yxysh.2023.12.006
29. National Health Commission. Notice of the general Office of the National Health Commission on printing and distributing the performance appraisal operation manual for National Tertiary Public Hospitals (2024 edition): Guo Wei ban Yi Han [2024] no. 87 [EB/OL].(2024-03-21)[2024-03-21]. Available at: http://www.nhc.gov.cn/yzygj/s3594q/202403/94a97921a9b043e8b8e3315aed9f1627.shtml
30. Han, A, and Lee, KH. The impact of public reporting schemes and market competition on hospital efficiency. Healthcare (Basel). (2021) 9:1031. doi: 10.3390/healthcare9081031
31. Medarević, A, and Vuković, D. Efficiency and productivity of public hospitals in Serbia using DEA-Malmquist model and Tobit regression model, 2015-2019. Int J Environ Res Public Health. (2021) 18:12475. doi: 10.3390/ijerph182312475
32. Yousefi Nayer, M, Fazaeli, AA, and Hamidi, Y. Hospital efficiency measurement in the west of Iran: data envelopment analysis and econometric approach. Cost Eff Resour Alloc. (2022) 20:5. doi: 10.1186/s12962-022-00341-8
33. Guo, H, Zhao, Y, Niu, T, and Tsui, KL. Hong Kong Hospital Authority resource efficiency evaluation: via a novel DEA-Malmquist model and Tobit regression model. PLoS One. (2017) 12:e0184211. doi: 10.1371/journal.pone.0184211
34. Lin, CS, Chiu, CM, Huang, YC, Lang, HC, and Chen, MS. Evaluating the operational efficiency and quality of tertiary hospitals in Taiwan: the application of the EBITDA Indicator to the DEA method and TOBIT regression. Healthcare (Basel). (2021) 10:58. doi: 10.3390/healthcare10010058
35. Li, M, Chen, G, and Chen, Y. Research on index standardization method in comprehensive evaluation.Chinese. J Manag Sci. (2004) 12:45–8.
36. Chen, P. Comparison of linear dimensionless methods and positive methods of inverse indicators.Operations research and management (2021) 30:95–101.
37. Liu, Q. Research on the evaluation of the operation efficiency of the third batch of county-level public general hospitals in Guangxi based on three-stage DEA-Tobit model Guangxi Medical University (2018).
38. Wan, H, Ai, L, and Chen, J. Evaluation of the operational efficiency of the pilot hospitals of comprehensive reform of county-level public hospitals in Hubei Province. Hospital Manag China. (2016) 36:15–8.
39. Chen, L, Tang, L, and Ni, W. Total factor productivity analysis of Shanghai tertiary general hospitals from 2003 to 2014 based on Malmquist index. J Shanghai Jiao Tong Univ. 36:912–6.
40. Zhang, J, Li, J, and Chen, H. Research on the performance evaluation and influencing factors of scientific research and innovation in universities in the Yangtze River Delta———based on DEA-Malmquist-Tobit model. Sci Technol Manag Res. (2020) 40:80–7.
41. Tian, W. An empirical analysis of the relationship between inflation and economic growth.Statistics and decision-making (2019) 35:153–7.
42. Lan, Y, Ye, W, and Wang, D. The current situation and enlightenment of the reform of public hospitals in foreign countries.Management of rural health undertakings in China (2021) 31:473–6.
43. Guo, X. Research on the efficiency and influencing factors of public hospitals in China Shandong University (2012).
44. Kirigil, JM, and Asub, EZ. Technical and scale efficiency of publiccommunity hospitals in Eritrea: an exploratory study. Health Econ Rev. (2013) 3:6. doi: 10.1186/2191-1991-3-6
45. Cheng, J, Jia, C, and Zhang, Y. Measuring technical efficiency and productivity of secondary maternal and child health hospitals in Hubei, China: some evidences from Hubei Province of China 2019 to 2021. Inquiry. (2024) 61:469580241254543. doi: 10.1177/00469580241254543
46. Androutsou, L, Kokkinos, M, and Latsou, D. Assessing the efficiency and productivity of the hospital clinics on the island of Rhodes during the COVID-19 pandemic. Int J Environ Res Public Health. (2022) 19:15640. doi: 10.3390/ijerph192315640
47. Lupu, D, and Tiganasu, R. COVID-19 and the efficiency of health systems in Europe. Health Econ Rev. (2022) 12:14. doi: 10.1186/s13561-022-00358-y
48. Gao, K, Wang, Z, and Gao, S. Comprehensive evaluation of the operational efficiency of traditional Chinese medicine hospitals in China based on DEA model. Modern Prev Med. (2022) 49:690–694+705.
Keywords: Guangxi tertiary public hospital, performance appraisal, operational efficiency, DEA-Malmquist, Tobit returns
Citation: Huang J, Yuan H, Wu Y, Wang H, Liu X, Wei B, Zhang M and Zhu P (2025) Measurement of the operational efficiency of tertiary public hospitals in Western China: evidence from Guangxi from 2019 to 2022. Front. Public Health. 13:1546402. doi: 10.3389/fpubh.2025.1546402
Received: 16 December 2024; Accepted: 11 February 2025;
Published: 21 February 2025.
Edited by:
Andrzej Klimczuk, Warsaw School of Economics, PolandReviewed by:
Alexandre Morais Nunes, University of Lisbon, PortugalCopyright © 2025 Huang, Yuan, Wu, Wang, Liu, Wei, Zhang and Zhu. This is an open-access article distributed under the terms of the Creative Commons Attribution License (CC BY). The use, distribution or reproduction in other forums is permitted, provided the original author(s) and the copyright owner(s) are credited and that the original publication in this journal is cited, in accordance with accepted academic practice. No use, distribution or reproduction is permitted which does not comply with these terms.
*Correspondence: Pinghua Zhu, emh1cGluZ2h1YUBneG11LmVkdS5jbg==; Mengjian Zhang, emhhbmdtajAyQDE2My5jb20=
†These authors have contributed equally to this work
Disclaimer: All claims expressed in this article are solely those of the authors and do not necessarily represent those of their affiliated organizations, or those of the publisher, the editors and the reviewers. Any product that may be evaluated in this article or claim that may be made by its manufacturer is not guaranteed or endorsed by the publisher.
Research integrity at Frontiers
Learn more about the work of our research integrity team to safeguard the quality of each article we publish.