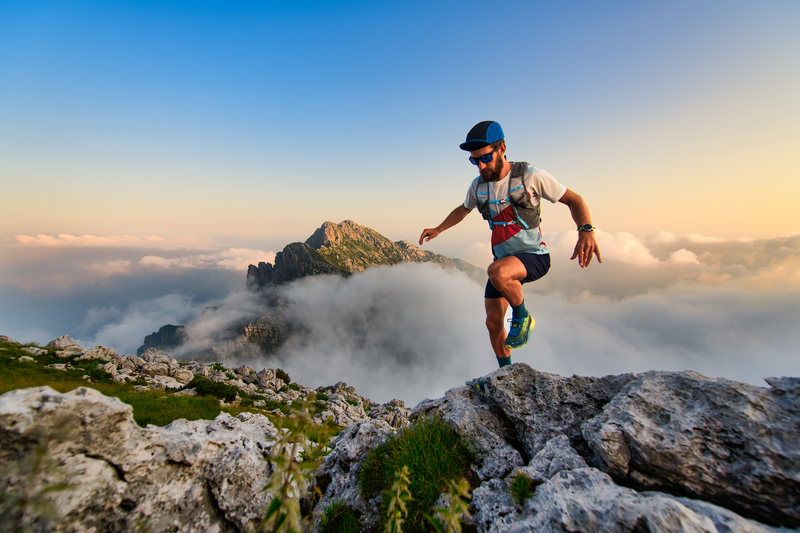
95% of researchers rate our articles as excellent or good
Learn more about the work of our research integrity team to safeguard the quality of each article we publish.
Find out more
STUDY PROTOCOL article
Front. Public Health , 14 March 2025
Sec. Children and Health
Volume 13 - 2025 | https://doi.org/10.3389/fpubh.2025.1467227
Introduction: This article outlines the rationale and methodology of the ENERGY expenditure of COmmuting to school study (the ENERGYCO study), a cluster-randomized controlled trial. The ENERGYCO study is divided into two phases: Phase I will aim; to assess the physical activity energy expenditure (PAEE) of different modes of commuting to school (i.e., walking, cycling, and motorized-vehicle) using indirect calorimetry in Spanish adolescents; and Phase II will aim to assess the effect of a school-based cycling intervention on resting metabolic rate and PAEE, as well as on other physiological, physical, and psychosocial outcomes on Spanish adolescents.
Method: For Phase I, a convenience sample of ~50 adolescents will be recruited. These participants will have their PAEE assessed in three different modes of commuting. Regarding phase II, a total of 300 adolescents from different schools in three Spanish cities will participate in this cluster randomized controlled trial. As many schools as necessary to meet the target sample will be included. In addition, each school will be randomized as either an intervention or control group. Participants from intervention schools will be asked to complete a school-based cycling intervention, while participants from control schools will be asked to continue their same habits for 8 weeks. The school-based cycling intervention will last for 8 weeks and will include Bikeability sessions, along with encouragement strategies to increase adherence to cycling to and from school.
Conclusion: The ENERGYCO study will provide novel insights into the PAEE associated with different modes of commuting to school using indirect calorimetry, as well as a comprehensive overview of how an 8-week school-based cycling intervention impacts resting energy expenditure, daily energy expenditure, and the physical and psychosocial health of adolescents.
Resting metabolic rate (RMR) constitutes the primary component of 24-h energy expenditure, representing the energy requirement for sustaining bodily functions while at rest in thermoneutral conditions (1). Given, fat-free mass (FFM) serves as a robust predictor of RMR in adolescent population (2, 3), exercise-induced weight loss is an important research topic since it leads to increases RMR by increasing the fat free mass component (4). However, studies in the adolescent population to modify the RMR have focused mainly on resistance or aerobic exercise (5, 6), without taking into account how regular physical activity (PA) might affect the RMR. Understanding PA energy expenditure (hereinafter referred to as PAEE) as the result of any muscular movement due to PA (7), the interaction of intensity, duration, and frequency of PA determines the total PAEE. In that sense, the American College of Sports Medicine states that exercise and health-oriented PA should be performed consistently to obtain its associated benefits. Nevertheless, nowadays there is an energy imbalance in the youth population, with high energy intake and low PAEE (8). This fact may have led to an increase in obesity in the young population worldwide, by 5.6% in girls and 7.8% in boys between 1975 and 2016 (9). In fact, the World Health Organization (WHO) has considered the overweight and obesity as a global public health concern (10). Although overweight and obesity have a multifactorial origin (9, 11), the literature recommends increasing PAEE through regular PA, as it is the most easily modifiable component of 24-h energy expenditure (11), to address the current situation. Therefore, interventions targeting young populations should aim to increase PAEE to restore or maintain energy balance (12).
In this regard, given that the young population commutes to and from school at least twice on a school day, promoting active modes of commuting, mainly walking or cycling (13), has been considered as an effective strategy to improve their health (14). Indeed, scientific evidence shows that active commuting to and from school (ACS) is associated with higher levels of total PA (15), during weekdays (16), and it even appears that those adolescents who walk to school also are more active in other contexts (17, 18). In fact, such is its impact on PA levels, that in the meta-analysis conducted by Campos-Garzón et al. (19), they indicated that actively commuting to and from school may help to complete 48% of the PA recommendations in young population. Nevertheless, few studies have analyzed the PAEE related to ACS (12, 20) and to the best of our knowledge, no study has analyzed how ACS impact on RMR. Moreover, while it is well known that walking and cycling are active modes of commuting to school, they can provide different health benefits (14). Cycling to school could be more related to physical fitness and physiological improvements such as, increasing in cardiorespiratory fitness levels and overall health (14), contributing to reducing the risk of cardiovascular diseases, obesity, diabetes or depression (14, 21–24). Therefore, although ACS has several associative benefits, it is important to know the differences between walking and cycling when promoting it (25).
Despite the limited number of studies analyzing PAEE related to ACS, a significant gap remains in accurately assessing PAEE during free-living behaviors. Indirect calorimetry is widely recognized as the gold standard for measuring PAEE, as it directly quantifies oxygen consumption and carbon dioxide production, providing continuous, real-time data that capture the complex metabolic responses during activity (26). This direct measurement offers a significant advantage over methods that rely on predictive equations or self-reported data, which often oversimplify the dynamic and context-specific nature of free-living behaviors (26). Previous studies have frequently relied on stationary or semi-portable indirect calorimetry systems that are not well-suited for field applications (27). Although portable metabolic systems have been developed to facilitate outdoor assessments, their high cost and operational complexity limit their widespread use in educational and field contexts (27). As an alternative, researchers have employed established equations, such as those proposed by Riebe et al. (28) and Pandolf et al. (29). However, these equations were derived under highly controlled laboratory conditions (e.g., typically on treadmills) and, consequently, do not account for the variability of environmental factors (e.g., changes in speed, terrain, and weather), which critically influence PAEE in real-life settings, particularly in ACS (30). Another common practice for analyzing PAEE related to ACS has been through estimates based on counts measured by accelerometers (20, 31). However, accelerometers may not be the most appropriate devices for measuring PAEE in the context of ACS (32). Moreover, a recent study compared the PAEE associated with walking to that of using an e-scooter (33). However, this study was conducted under laboratory conditions, making it impossible to account for environmental factors that could influence PAEE. It is well known that the availability of safe and accessible routes directly correlates with increased PA among adolescents, which could, in turn, lead to higher PAEE (34). Additionally, PA levels may depend on adolescents’ perceptions in their commuting environment, considering factors such as fear of accidents, infrastructure, and social norms related to active commuting behaviors (35). Therefore, it is crucial to compare the PAEE associated with different modes of commuting to school in free-living conditions. Furthermore, developing a context-specific equation that incorporates key commuting characteristics (e.g., speed and distance) would provide a more accurate estimation of PAEE associated with this behavior.
Furthermore, ACS interventions have primarily been conducted in children (i.e., from 6 to 11 years) rather than in adolescents (i.e., from 12 to 17 years) as well as promoting walking rather than cycling. These interventions have primarily focused on changes in the mode of commuting, frequency of commuting, or increased PA levels (36–39) rather than assessing the effects on 24-h energy expenditure components (i.e., RMR and PAEE). In addition, interventions to improve RMR or PAEE have mainly consisted of resistance or endurance trainings (5, 11), being these activities performed out of school. To the best of our knowledge the effect of a school-based cycling intervention on the RMR and daily PAEE of adolescents has not been studied to date. In fact, the assessment of the effects of a school-based cycling intervention could be particularly important to consider as a suitable strategy to increase the RMR and re-balance PAEE in the adolescent population. In addition, a school-based cycling intervention will not only produce effects at physiological level (i.e., RMR or PAEE), but it will also allow to deepen the effects of this mode of commuting to school at physical or psychosocial level in the adolescent population. It has been shown that cycling to school is associated with greater grip strength and vertical jump (40), but the association does not imply causality. Similar results occur at the psychosocial level, where cycling to school has been associated with greater well-being or increased social relations compared to use passive passively commuting to school in adolescents (41). Moreover, there is controversy as to whether cycling to school could improve young people’s body composition (14). Thus, a school-based cycling intervention is needed to increase and improve the evidence of the effects of cycling to school in physiological, physical, and psychosocial outcomes.
Therefore, this protocol describes the rationale and methodology of the ENERGY expenditure of school Commuting study (The ENERGYCO study). ENERGYCO project will address the gaps and limitations in the existing scientific literature regarding the PAEE that occurs during cycling to school. Considering the previous literature and existing gaps, the ENERGYCO project will be divided into two phases. Phase I will focus on using a portable metabolic system to assess PAEE associated with different modes of commuting (i.e., walking, cycling, motorized vehicles) under free-living conditions in Spanish adolescents. Additionally, Phase I will serve to develop a predictive equation that will be used in Phase II to measure PAEE associated with different modes of commuting to and from school. Additionally, considering the ongoing debate regarding the health benefits of ACS and the scarcity of studies examining the effects of school-based cycling interventions on RMR and PAEE, the Phase II will aim to assess the effects of a school-based cycling intervention on RMR and PAEE, as well as on other physiological (i.e., basal heart rate, blood pressure, and body temperature), physical (i.e., PA levels, anthropometric measures, body composition, physical fitness, and motor competence), and psychosocial outcomes (e.g., perceived barriers to active commute to school, self-esteem, and mental health) on Spanish adolescents. It is hypothesized that, in Phase I, using a portable metabolic system under free-living conditions will provide accurate and distinct measurements of PAEE associated with different commuting modes in Spanish adolescents. Specifically, we hypothesize that these measurements will highlight significant differences in PAEE across commuting modes, offering a more precise understanding of how commuting impacts adolescents’ physical activity levels. Additionally, we expect the data collected in Phase I to contribute to the development of a valid and reliable predictive equation for estimating PAEE during commuting to and from school. we hypothesize that a school-based cycling intervention will lead to a significant increase in both RMR and PAEE compared to a control group. We further hypothesize that the intervention will improve physiological outcomes (e.g., reductions in basal heart rate), physical outcomes (e.g., enhanced physical fitness and motor competence), and psychosocial outcomes (e.g., increased self-esteem) among Spanish adolescents.
The ENERGYCO study is a cluster randomized controlled trial to be conducted in high schools from three Spanish cities (Granada, Jaén, and Almería), where the schools will be the units of randomization, and individuals within the schools will be the units of analysis (participants). This project will consist of two main phases: Phase I will present a cross-sectional design which will take place before starting the Phase II. A cross-sectional approach will be adopted due to its suitability for capturing a snapshot of key variables of interest at a specific point in time, aligning with the objectives of this phase (i.e., comparing PAEE associated with different modes of commuting to school and developing predictive equations for measuring PAEE in Phase II). In contrast, for Phase II a cluster parallel-arm design will be employed to allocate interventions effectively to larger groups while accounting for potential intra-cluster variability, enhancing the external validity and generalizability of the results. The ENERGYCO study has been approved by the Review Committee for Research Involving Human Subjects at the University of Granada (Reference: 2496/CEIH/2021) and registered with a ClinicalTrials.gov ID: NCT06414668. In addition, the present study protocol has been written in accordance with the Standard Protocol Items: Recommendations for Interventional Trials (SPIRIT) statement (See Supplementary material 1).
In the Phase I of the ENERGYCO study, a convenience sample of 50 students (12–16 years old) will be recruited to assess the PAEE of different modes of commuting to school (i.e., walking, cycling, and motorized-vehicle). For Phase II, the ENERGYCO study will recruit a random sample of students (n = 300) from the three Spanish cities (i.e., Granada, Almería, and Jaén). The intervention aims to assess the effects of a school-based cycling intervention in the RMR and PAEE related to cycling to school, as well as its impact on others physiological, physical, emotional, and psychosocial outcomes in Spanish adolescents. The randomization process is presented in Figure 1.
Figure 1. School recruitment plan flow chart of the ENERGYCO study. *Expected number during the school recruitment process.
The trial management group will be composed of a steering committee of investigators responsible for overseeing all aspects of trial implementation. Their primary responsibilities include coordinating participant recruitment, ensuring compliance with study protocols, and monitoring data quality. Moreover, given the wide range of variables included in the ENERGYCO project, each investigator will be assigned a specific area of coordination: physiological variables (e.g., RMR and PAEE), physical variables (e.g., sedentary time and PA levels), psychosocial variables (e.g., self-esteem, and mental health), among others. Although the inclusion of additional oversight committees was considered, the manageable scope of this trial, combined with the expertise of the current steering committee, is considered sufficient to ensure proper trial governance and robust data quality control.
In Phase I, a convenience sample of approximately 50 students will be recruited. A call to action will be used to engage adolescents through the development of infographics and information about the aims of this phase, which will be disseminated through social media, personal contacts, or emails. Since these tests will be conducted outside the school timetable, no schools will be directly contacted. Instead, parents or legal guardians will be responsible for contacting the research team if their children are interested in participating in the study and they provide their consent. In this call to action, incentives, such as activity wristbands will be given away to participating adolescents. For Phase II, a specific selection protocol will be implemented through a random draw. A list of all public secondary schools in each of the participating cities of the government educational institutions in Andalucía will be obtained. The schools in the three cities (Granada, Almería, Jaén) will be contacted by phone, and if they are interested in participating, they will have to meet a series of inclusion and exclusion criteria presented in Table 1. During the call, a meeting will be arranged with interested schools to explain to the management team and teaching staff the main objective of the study, as well as to obtain official consent to participate in the project from the school. After that, the research staff with the help of Physical Education teachers will recruit participants and their families, by visiting selected classes and explaining the study to prospective adolescent participants and finally requesting the family’s consent. The inclusion and exclusion criteria for the participants are presented in Table 2. To complete the sample of students in the intervention group, several schools may be needed, as not all students will be able to participate in the school-based cycling intervention due to family reasons, distance, predisposition, etc. To encourage as many students as possible from the intervention school to participate (with their families’ consent) in the school-based cycling intervention, an incentive system will be planned similar to other studies (42, 43). This incentive will consist of a voucher to be redeemed for sports equipment, provided that the participant has completed the 8 weeks of intervention and presents valid data at both baseline and follow-up. Once finished the baseline assessment, the participating schools will be randomly assigned through a draw to either a control or experimental group.
For Phase II, at least 250 participants are needed to ensure an acceptable sample size, assuming 5% precision (α err prob) and a very high statistical power of 95%. (Error 1-β err prob) (44). This calculation has been made considering a study objective where the effect of the intervention program on the different health variables of young people is evaluated (e.g., RMR, EE, PA levels, body composition, muscle strength). In this study there will be two main comparison groups (experimental group and control group) and for this the ANCOVA statistical analysis will be performed: fixed effects, main effects, and interactions (F test) and with an effect size = 0.25. It is assumed that there will be dropouts during the school-based cycling intervention (≈15% in the experimental group), so ≈300 adolescents will be recruited from different schools in the three Spanish cities. Following the forecasts in Figure 1, 100 adolescents would be needed in each city. The groups randomization will be done at a 1:1 ratio at city level (i.e., from each city, 50 participants will be randomized to intervention and 50 to control groups), as other studies have done before (45). Nevertheless, due to the low prevalence of cycling to school in Spain (46), we will try to recruit as many schools as necessary in each city to reach the number of 100 adolescents.
In Phase I the PAEE of different modes of commuting will be quantified by analyzing each participant individually in different controlled environments. For phase II, after recruiting schools and participants, an eight-week school-based cycling intervention will be implemented. The choice of an eight-week duration was based on the results reported in the systematic review by Dorothea et al. (47) on the effects of cycling interventions in a school setting. For example, although interventions lasting longer than 8 weeks demonstrate positive changes in physical fitness (43, 48), they also exhibit lower adherence, with a higher dropout rate among participants post-intervention. Therefore, considering that interventions lasting 4 to 8 weeks show effects on self-efficacy (49) or PA levels (50) and report a lower dropout rate, an eight-week duration was chosen for the school-based cycling intervention. The intervention will consist of adolescents having to cycle to and school for eight consecutive weeks. To encourage participants to complete the intervention, they will take part in a Bikeability training and different encouragement strategies will also be used during this period. Moreover, the ENERGYCO school-based cycling intervention shows relevant factors described in the current National Education Law for the curriculum of Compulsory Secondary Education. This approach is based on strategies proposed by the Active Living by Design Community Action Model (51), which involves preparing through the development of interventions, promoting education, and motivating students to cycle anywhere, as well as organizing activities with incentives and programs to engage participants. Regarding the control groups, participants in these schools will be asked to continue their daily habits. In addition, at the end of the eight-week cycling intervention in schools, control schools will be given the opportunity to conduct the Bikeability sessions and will receive all the motivational strategies used for the participating adolescents.
In Phase I, participants will perform three different simulations (i.e., commute by motorized-vehicle, walking, and cycling [see Figure 2]): (1) a simulation of driving to school will be performed by motorized-vehicle. After all the calibration process of the Cosmed K5 (both, flow and gas analyzers will be calibrated before every gas exchange measurement), a face-mask attached to the K5 will be place and gas exchange will be check during a two-minute period before the measurement start. After the check phase, gas exchange recording start during a five-minute period, while participants remain seated. Then, the participants will simulate commute in a motorized-vehicle by remaining seated in a chair for another 15-minute period, having previously walked for 2 mins simulating that participants are moving toward the motorized-vehicle from home. After this first simulation, there will be a five-minute period of cooling down. The total time of this first test will be 27 min. (2) A simulation walking to school will be conducted in a closed circuit defined by the research staff. The same procedures mentioned before will be followed. Then, the participant will walk for 15 min (the participant will determine the speed and intensity of walking) and finish with a five-minute cool down; (3) Participants will simulate a cycling route. They will wait for five-minute after walking and they will start cycling during 15-minute period along the designated route. The simulation will finish with a five-minute cool down period. The total time used in the walking and cycling simulations will be 45 min. These times were chosen because an average walking speed of 4.4–4.6 km/h would not exceed the distance Spanish adolescents are willing to walk to their educational center (1,350 m) (52). While no study in Spanish adolescents indicates the distance, they are willing to cycle to school, we estimated a distance of 4,200 m, the threshold distance used for Australian adolescents (53), with which a speed of approximately 15 km/h would not exceed 15 min. Detailed visual information on the evaluation and calibration process of the Cosmed K5 (Cosmed, Rome, Italy) can be found at the following link.1 In order that one active mode of commuting (e.g., walking) does not affect the post exercise oxygen consumption, thus PAEE, of the subsequent active mode of commuting (e.g., cycling), participants will alternate randomly by first performing the simulation of walking or cycling. In addition, the alternation of starting on the bike and walking will be counterbalanced by having the same number of participants starting on the bike or walking. Finally, all data will be downloaded using PC OMNIA.9 software v3.30.79 (Cosmed, Rome, Italy).
Figure 2. Complete phase I protocol showing the three controlled environment simulations (i.e., commute by motorized-vehicle [from phase 2 to phase 2.2], walking [from phase 3 to phase 3.2], and cycling [from phase 4 to phase 4.1]).
In the Phase II, the procedures of the intervention will be tested as a pilot phase to ensure its feasibility and effectiveness. Prior to this, the research team will undergo joint training for internal evaluation. The pilot phase will be implemented in adolescents from a school in Granada over 2 days, with the involvement of the entire research team, lasting approximately 1 h per day. In this pilot phase, the organization of evaluations and the effectiveness of the tests used will be observed by the research group. Moreover, the school-based cycling intervention, which will be described in detail later, will be designed by research personnel trained as Physical Education teachers and graduated in Sport Sciences, and subsequently reviewed by research experts. During the baseline and follow-up of Phase II, the RMR, along with various health-related outcomes, will be assessed. These include individual, social, environmental, and safety factors associated with ACS (see Table 3). Figure 3 outlines the assessments to be conducted during the baseline and follow-up periods.
Assessment day 1 (2): this session will include anthropometry, blood pressure, muscular strength, and motor competence assessment at an indoor sport facility.
Assessment day 2 (2 h): in this session the cardiorespiratory fitness of the participants will be assessed. Once participants have finished the test, they will have to complete an online student-questionnaire. During this session, participants will receive a belt with an accelerometer (GT3X+ accelerometers; ActiGraph, Pensacola, FL) and a Global Positioning System (GPS; QStarz BT-Q1000XT; Travel Recorder, International Co, Ltd. Taipei, Taiwan), a heart rate monitor, including chest band and watch, (Polar Ignite 10, Polar Electro Oy, Kempele, Finland) for measuring their resting heart rate during the first weekend of the baseline week, and to use it during the cycling trips to and from school.
Assessment day 3 (1 h and a half): in this session the RMR of the participants will be assessed. Participants will have to come to the indicated facility in a postabsorptive state (10–12 h without consumption of caloric food or beverages) and by passive transport. Moreover, participants from the experimental group will be provided with two links, one to fill in weekly, after the Bikeability sessions week, providing information about their weekly cycling experience to and from school and their opinions on the motivational strategies, and another link with access to a questionnaire to be filled in by their parents.
RER will be measured using the Omnical metabolic cart (Maastricht Instruments, Maastricht, The Netherlands), a validated system for assessing volumes of oxygen consumption (VO2) and carbon dioxide production (VCO2) during resting conditions which a low measurement error for determining RER (1.7 ± 0.9%) (54). During the test, a ventilated plastic canopy will be connected to the instrument and placed over the participant, allowing the individual to breathe normally while the Omnical records CO2 and O2 levels in inhaled and exhaled air every 5 s. The device will be calibrated daily using a gas mixture of 18.0% O2 and 0.8% CO2. Data will be analyzed as the average VO2 (ml/min) and VCO2 (ml/min) over a 10-min stable period, aggregated by participant at baseline and follow-up. However, since the RMR measurement can only be performed at the research center in one of the participating cities, a contingency plan has been established in case participants from other cities cannot travel to the evaluation center. In such cases, the RMR will also be estimated for all participants using the Schmelze equation, which has demonstrated superior results compared to the gold standard among 12 equations, including a low measurement difference with the gold standard (−51.0 to 15.0 kcal/day), low % bias (−1.6 to 5.8%), an acceptable prediction percentage relative to the gold standard (40.7 to 59.0%), and a low RMSE (136.2 to 305.4 kcal/day) for adolescents with normal weight, overweight, or obesity (55).
PAEE related to different modes of commuting to school (i.e., walking, cycling, passive modes) will be measured using the Cosmed K5 portable metabolic system in Phase I. The Cosmed K5 has previously shown a good level of agreement compared to stationary metabolic carts at low cycling intensity (5.8% difference in VO2). At moderate intensities, it slightly underestimated VO2, VCO2, and energy expenditure (~6.6–6.9%). Additionally, during walking tests, the Cosmed K5 demonstrated high reproducibility, with a coefficient of variation of 4.5% for energy expenditure per kilometer and a concordance coefficient of 0.91. VO2 measurements were consistent, with low variability in the fraction of expired oxygen (coefficient of variation of 0.9%) (56). The Cosmed K5 is worn on the back with a harness and measures oxygen consumption (VO2) and carbon dioxide production (VCO2) in breath-by-breath mode. Before each assessment, the device will be calibrated using a reference gas mixture of 15.92% O2 and 5.03% CO2. Delay calibration for the breath-by-breath mode will also be performed (57). During the test, participants will breathe normally while commuting, and the Cosmed K5 will record data continuously. Data will be analyzed as the mean VO2 and VCO2 (ml/min) during each commuting mode. In Phase II, PAEE related to cycling will be estimated using predictive equations developed in Phase I. These equations will incorporate variables such as distance, speed, and heart rate, and will be cross-validated against PAEE measurements obtained with the Cosmed K5 to ensure accuracy. Therefore, data will be analyzed as the mean VO2 and VCO2 (ml/min) during each cycling trip to and from school, aggregated by participant during the intervention period.
Adolescents will self-report their gender, age, school grade, full postal address, socioeconomic status (including family education level and parents’ professions), extracurricular activities, and bicycle ownership through a structured questionnaire. Moreover, their parents will have to complete another questionnaire, where they will self-reported similar information to those previously self-reported by the adolescents. These data will be aggregated by participant and family, and they will be collected during baseline and follow-up assessments.
Heart rate will be monitored during three different commuting simulations in Phase I using the Polar Ignite H10 device (Polar Electro Oy, Kempele, Finland), which includes a chest strap and a watch. The Polar Ignite H10 has been considered the gold standard for measuring heart rate in sports conditions (with an RR interval mean bias ± limit of agreement of 0.2 ± 26.8 ms compared to a Holter monitor) (58). Data will be continuously recorded during the simulations and downloaded using the Polar app on a computer. The average heart rate (beats per minute) across the simulations will be calculated and aggregated by participant during baseline assessments. In Phase II, participants will measure their resting heart rate in the morning during the first weekend of both baseline and follow-up. Additionally, they will monitor their heart rate during each cycling trip to and from school throughout the eight-week intervention period. Data will be downloaded by the research staff using the Polar app after the follow-up period. The mean heart rate during cycling trips will be analyzed and aggregated by participant to examine trends over the intervention period.
Blood pressure will be evaluated using a standardized protocol for children (59), that employs the auscultatory method. A stethoscope will be placed on the elbow flexure over the radial artery to determine systolic blood pressure (SBP) and diastolic blood pressure (DBP). Measurements will be conducted individually for each participant and averaged across three repeated assessments at baseline and follow-up.
Body temperature will be measured using an iButton device (DS-1922 L, Thermochron; resolution: 0.0625°C; Maxim, Dallas, USA) placed on the left wrist with an epoch of 1 s. During sports conditions, the iButton device observed error estimates were within acceptable limits for the skin temperature method comparison, with typical errors of <0.3°C (showing an r coefficient of >0.999 under all assessed conditions) (60). Data will be analyzed using the Temperatus software2 (61) and aggregated as the mean temperature over the recording period for each participant at baseline and follow-up.
PA will be objectively assessed using an ActiGraph GT3x + accelerometer (ActiLife, version 6.11.7) worn on the hip for seven consecutive days during waking hours, excluding bathing, water activities, or sleeping. The accelerometer records acceleration in three axes, reporting the amount of activity performed at three intensity levels: light, moderate, and vigorous. Data will be processed using the open-source R package GGIR v.3.1–2. The processing pipeline includes: (i) auto-calibration of raw accelerometer data based on local gravitational acceleration (62), (ii) detection and imputation of non-wear time (63), and (iii) calculation of activity counts over 15-s epochs using the algorithm described by Neishabouri et al. (64) and facilitated in the open-source R package actilife counts (DOI: 10.5281/zenodo.7723333), and classifying MVPA as every epoch above the established cut-point for MVPA of 574 counts/15 s (65). Data will be aggregated by participant as the average daily time spent in MVPA during the baseline and follow-up assessments. In addition, PA will be self-reported using the Spanish Youth Activity Profile questionnaire a validated tool for estimating PA and sedentary behaviors at the group level in adolescents (kappa = 0.61–0.77; Intraclass Correlation Coefficient [ICC] = 0.77–0.89) (66). The Spanish version developed using a back-translation process, includes 19 items divided into three sections: (i) activity at school, (ii) activity out-of-school, and (iii) sedentary behaviors. The final variables to be analyzed will include average scores ranging from 1 to 5 for PA at school and PA outside of school, aggregated by participant during baseline and follow-up assessments.
Cardiorespiratory fitness will be assessed using the validated Course Navette field test (67). Adolescents will run between two lines separated by 20 meters, maintaining a rhythm dictated by audio signals from a pre-recorded CD. The initial speed will be 8.5 km/h, increasing by 0.5 km/h per minute. The test will end when participants fail to reach the lines according to the audio cues for at least two consecutive trials or stop due to fatigue. The equations by Léger et al. (67), previously validated in children and adolescents (r = 0.587) (68), will be used to estimate maximum oxygen consumption (VO2max), calculated individually for each participant during baseline and follow-up assessments. Muscle strength of the upper and lower limbs will be evaluated using standardized and validated protocols (69, 70). Upper limb strength will be measured using an analog dynamometer (Takey TKK5401) following the ALPHA fitness battery recommendations. Participants will perform the test with both hands. Lower limb strength will be assessed using the Standing Long Jump (SLJ) test, a valid and reliable tool for measuring lower limb power (70). Both handgrip strength and SLJ presents a high association with isokinetic parameters (r = 0.61 and 0.87; R2 = 0.39 and 0.76, respectively) (71). Additionally, the mid-thigh pull test (IMTP) will be conducted, an isometric test of maximum leg and back strength performed with an analog dynamometer (Takei TKK5402). This test has been previously validated by considering different knee and hip positions, showing moderate reliability between positions (r ranging from 0.666 to 0.739) and high reliability between sessions (r > 0.819) (72). For all strength tests, measurements will be conducted twice with a two-minute rest between trials. Data will be averaged across trials and aggregated by participant during baseline and follow-up assessments.
Perceived physical literacy will be evaluated using the Perceived Physical Literacy Instrument (PPLI). The PPLI has shown convergent validity ranging from 0.40 to 0.52, indicating that its three factors present adequate discriminant validity and moderate to good reliability (ICC = 0.62–0.79) (73). This tool consisting of nine items designed for adolescents and scored on a five-point Likert scale (1 = strongly disagree to 5 = strongly agree). The nine items are equally divided into three subscales: (a) knowledge and understanding (three items), (b) self-expression and communication with others (three items), and (c) sense of self and self-confidence (three items) (73). The final variable will be the mean score of the nine items aggregated by participant during baseline and follow-up.
Motor competence will be assessed using the Bruininks-Oseretsky Test (BOT-2; ICC >0.76 for all items) (74). The short form of the test will be used, consisting of 14 subtests that evaluate fine and gross motor skills. Fine motor tasks include Drawing, Lines through Winding Paths, Folding Paper, Copying a Square, Copying a Star, and Transferring Pennies. Gross motor tasks include Hopping on One Foot, Same-Side Synchronized Hopping, Heel-to-Toe Walking on a Line, Standing on One Leg and Balance Beam (Eyes Open), Stationary One-Legged Jumping, Catching a Ball with Both Hands, Dribbling a Ball, Alternating Hand Movements, Knee Push-Ups, and Sit-Ups. These tasks measure fine motor precision, bilateral coordination, balance, running speed, agility, upper body coordination, and strength (75). Results will be standardized using normative data from the BOT-2 Test Manual and aggregated by participant during baseline and follow-up assessments.
Anthropometric measurements will include body mass, height, Body Mass Index (BMI), and circumferences of the waist and neck. Body mass and height will be measured with participants wearing appropriate Physical Education class clothing (shorts and a short-sleeved shirt) and bare feet, using a SECA scale and stadiometer (model 799, Electronic Column Scale, Hamburg, Germany). Body mass will be recorded to the nearest 0.1 kg, and height will be measured in the Frankfort plane to the nearest 0.1 cm. Sitting height, used to estimate biological or somatic age, will also be measured using a stadiometer (Holtain Ltd., Crymmych, Pembs, United Kingdom) equipped with a sit-down drawer. BMI will be calculated as body mass divided by squared height (kg/m2) and classified into normal weight, overweight, or obesity categories based on Cole et al.’s cut-off points (76). Waist and neck circumference will be measured using the Executive thin line W606pm tape measure (Lufkin, Mexico). Two consecutive measurements will be taken for each circumference and averaged. All anthropometric measurements will be conducted during baseline and follow-up assessments.
Body composition will be assessed using Dual-energy X-ray Absorptiometry (DXA; Hologic Series Discovery QDR scanner [Bedford, MA], and APEX software, version 4.0.2). DXA is a scanner with high precision for determining body mass (ICC:0.999), lean mass (0.995), fat mass (0.998), and bone mineral content (0.996) (77). The DXA scan will provide data on fat-free mass, fat mass, lean mass index, fat mass index, body fat percentage, visceral adipose tissue, and bone mineral content. In addition, total body less head values will be reported, following the recommendations of the International Society of Clinical Densitometry for pediatric populations (78). Measurements will be conducted individually for each participant during baseline and follow-up assessments.
Perceived barriers to ACS will be assessed among participants using the BATACE Scale (79). Indeed, the BATACE scale has been considered a validated and reliable tool for evaluating barriers to ACS in Spanish adolescents (α ranged from 0.64 to 0.72; and ICC between test and retest ranged from 0.68 to 0.77) (79). The scale includes 18 items that address two key dimensions: environmental/safety factors and planning/psychosocial aspects. The resulting variable for analysis will be the average score across all items, ranging from one to four, where one indicates a low perception of barriers and four indicates a high perception of barriers. Scores will be aggregated by participant and collected during baseline and follow-up assessments. Parental perceived barriers to ACS will be evaluated using the PABACS (Parental Perception of Barriers toward ACS) questionnaire, a validated (rho ranged from 0.20 to 0.31) and reliable tool (ICC ranged between test and retest ranged from 0.51 to 0.55) (80). This questionnaire consists of 23 items divided into three scales: general barriers, walking barriers, and cycling barriers. The final variables for analysis will be the average scores for each scale and individual items, ranging from one (strongly disagree) to four (strongly agree). Data will be aggregated by parent during baseline and follow-up assessments.
Basic psychological needs satisfaction in ACS will be assessed using Basic Psychological Need Satisfaction in ACS Scale (BPNS-ACS) (81). The BPNS-ACS scale have shown a good internal consistency (α ranged from 0.81 to 0.92) and a high ICC between test and retest (ICC ranged from 0.76 to 0.81) (81). This scale consists of 12 items that explore participants’ thoughts and feelings about their usual mode of commuting to and from school. Participants will rate their agreement with each item on a five-point Likert scale, ranging from “strongly disagree” to “strongly agree.” The resulting variable will be the average score across all 12 items, aggregated by participant and collected during baseline and follow-up assessments. Motivation for ACS will be evaluated using the Behavioral Regulation in ACS (BR-ACS) questionnaire (82). This scale presents both a high internal consistency (α ranged from 0.70 to 0.91) and ICC (ranged from 0.70 to 0.76) (83). The BR-ACS consists of six factors and 23 items that assess different forms of motivation, including intrinsic motivation, integrated regulation, identified regulation, introjected regulation, external regulation, and amotivation. Participants will indicate their agreement with statements such as “I go or would go to and from school walking or cycling because.” on a five-point Likert-type scale, ranging from “not true for me” to “very true for me.” The final variables for analysis will include average scores for each of the six factors, aggregated by participant and measured during baseline and follow-up assessments.
Self-esteem will be assessed using the validated Spanish version of the Rosenberg Self-Esteem Scale (RSES), consisting of 10 items that evaluate self-respect and self-acceptance (84). This tool has demonstrated satisfactory psychometric properties, with Cronbach’s alphas ranged from 0.83 to 0.86, as well as factorial equivalence was validated using a structural equation model (CFI = 0.912; RMSEA = 0.079), indicating a high degree of invariance (84). In this case, participants will rate their agreement with each item on a four-point Likert scale ranging from one (“strongly disagree”) to four (“strongly agree”). Items 1, 3, 4, 7, and 10 measure positive self-esteem, while items 2, 5, 6, 8, and 9 measure negative self-esteem. The resulting variable will be the average score across all items, with higher scores indicating higher self-esteem. Data will be aggregated by participant and collected during baseline and follow-up assessments. Mental health will be evaluated using the PANASN questionnaire (85), a self-report tool with 20 items which presented a good internal consistency (α ranged from 0.75 to 0.76) and an acceptable temporal stability (ICC = 0.84). Ten items assess positive affect (e.g., “I am a cheerful person, I tend to get excited”), and 10 items assess negative affect (e.g., “I feel nervous”). Participants will complete the questionnaire based on how they usually feel and/or behave, using a three-point response scale: “Never” (i), “Sometimes” (ii), and “Often” (iii). The final variables for analysis will be the average scores for positive and negative affect, aggregated by participant and measured during baseline and follow-up assessments.
ACS will be evaluated using the PACO questionnaire3, a reliable and valid tool for young Spaniards (Kappa ranged from 0.42 to 0.94) (86, 87). The PACO questionnaire consists of four self-report items designed to assess the usual mode of commuting and the weekly frequency of commuting among children and adolescents. The four questions include: (i) “How do you usually get to school?” (ii) “How do you usually get home from school?” (iii) “How did you get to school each day?” and (iv) “How did you get home from school each day?” Participants will select their mode of commuting from predefined options such as walking, cycling, car, motorcycle, bus, public bus, metro/train/tram, or other (requiring mode specification). The main variables of interest are the percentage of usual cycling and active modes of commuting to and from school, along with the weekly number of cycling and active trips. Additionally, participants will report their distance from school and the time it takes to commute from home to school. Response options for distance range from less than 0.5 km to 5 km or more, while response options for travel time range from less than 15 min to 60 min or more (86). Other factors associated with ACS, such as independent mobility, will also be analyzed as secondary variables. Data will be aggregated by participant and collected during baseline and follow-up assessments. Overall, these questionnaires provide valuable data on modes of commuting, frequency of active commuting, distance covered, and time spent during the commute for both adolescents.
Cycling skills will be assessed using a specific observational checklist completed by the research staff after each participant’s performance during the Bikeability session. The checklist includes 18 cycling skills grouped into six categories: (i) starting off, pedaling, and changing gears; (ii) turning right; (iii) turning left; (iv) performing zigzags; (v) braking; and (vi) unexpected turning. Each skill is scored dichotomously (yes/no), and participants will receive a final score ranging from 0 to 18 points. Data will be aggregated by participant and collected during the Bikeability session. The checklist has been previously published, and it is available elsewhere (88).
Family socioeconomic status will be assessed using two complementary tools. Adolescents will self-report their family’s socioeconomic status through the Family Affluence Scale III (FAS III; r = 0.90 and ICC >0.76) (89). The resulting variables will include composite scores derived from the respective scales, reflecting the level of family affluence. Data will be aggregated by participants and family, and collected during baseline and follow-up assessments.
Distance from home to school will be determined using two objective methods. The first method calculates the shortest walking path between the participant’s home and school using Google Maps™ software (52). The second method combines GPS and Geographical Information System (GIS) data to establish the actual route taken by participants between home and school. Additionally, participants and parents will self-report their postal addresses, and the school’s postal address will also be obtained. These addresses will serve as reference points for both methods. The resulting variables will include the calculated shortest distance (km) and the actual route distance (km). Data will be aggregated by participant and collected during baseline and follow-up assessments.
ACS behavior will be objectively evaluated over seven consecutive days using a Q-STARZ BT-Q1000XT GPS device, manufactured by Int Co., Ltd. (Taipei, Taiwan). The GPS will record distance, speed, and trip duration for each commute performed by the participants. Additionally, environmental variables such as terrain slope will be measured and later used as confounders in the analysis. The GPS will be configured with a 15-s epoch to ensure precise data collection. This protocol, which combines GPS data with accelerometry for analyzing ACS behavior, has been previously validated by our research group (17, 20, 31). In this project, the combination will also be used to estimate PAEE associated with different modes of commuting to school. The main variables of interest include distance (km), average speed (km/h), and trip duration (minutes). Data will be aggregated by participant and collected during baseline and follow-up assessments.
Ambient and weather conditions will be assessed using two complementary methods. In Phase I, ambient temperature will be measured using iButtons (S-1922 L, Thermochron; resolution: 0.0625°C; Maxim, Dallas, USA). Participants will wear an iButton attached to their trousers to measure outdoor temperature. It is important to note that the iButton device has also demonstrated a very high correlation with a mercury thermometer (r > 0.999) for measuring ambient temperature (REF). Data from the iButtons will be analyzed using the Temperatus software (see Footnote 2) (90). In Phase II, temperature (maximum, minimum, and average), total rainfall, and average wind speed will be obtained from the National Weather Data Bank. This open-source database contains climatological information recorded by observatories across the country. Data will be collected from the weather station closest to each school for the five weekdays and the preceding weekend prior to completing the student questionnaire. These variables will be recorded at both baseline and follow-up. The main variables of interest include daily ambient temperature (°C), total rainfall (mm), and average wind speed (km/h). Data will be aggregated by participant and city.
School characteristics will be assessed using various measures. School size will be determined based on student enrollment, reflecting the total number of students enrolled during the study period. School socioeconomic status (SES) will be established using data from previous studies (91). Additionally, the socioeconomic status of the school neighborhoods will be objectively analyzed and classified as high or low using GIS. Moreover, the length of the cycling lane network within a 5.1 km street-network buffer around each school will be calculated using GIS (92). Additionally, school staff will be asked to provide information on the availability of bicycle racks either on school premises or near the main entrance. School engagement, which may influence the effects of the intervention, will be assessed through the perceptions of the research staff. Engagement will be categorized into three dimensions: teacher engagement (average staff perceptions of teacher involvement across all classes), student engagement (average staff perceptions of student participation across all classes), and overall school engagement (average of teacher and student engagement scores). Data for all variables will be collected during baseline and follow-up assessments and aggregated by participant and school.
The school cycling intervention program will include 1 week of training and familiarization with the bicycle using the Bikeability methodology (88), and the adolescents’ commuting to and from the school for 8 weeks with the support of encouragement strategies during the intervention. Bikeability sessions will have the objective of familiarizing the students with cycling to and from school. This is based on the fact that participants will know how to cycle, but many of them might not have cycled for a long time and have not cycled regularly. Moreover, Bikeability sessions will help the research staff to analyze if any student does not have sufficient capacity to carry out the intervention. The Bikeability methodology, previously tested and published as innovative material by our research group (88), will consist of two sessions over a one-week period delivered by the research group with the support of the Physical Education teacher during Physical Education class to acquire practical skills and understand how to cycle safely and effectively. The components and content of these two sessions focus on: (i) knowledge about road safety regulations, safety equipment for cyclists, hand signals while cycling, and the benefits of cycling as a means of transportation, and (ii) cycling-related skills. Prior to the first session, the Physical Education teacher will provide students with educational theoretical material to raise awareness about the benefits and usefulness of cycling as a mode of travel, knowledge about road safety rules for cyclists, knowledge about safety equipment for cyclists for both the cyclist and the bicycle, and hand signals while cycling in an urban context. The sessions conducted by the research group with the support of the Physical Education teacher are described below:
1. Closed Circuit Session (60 min—first day): A practical session will be conducted at the school facilities to learn or improve the fundamental cycling skills necessary for safe biking. Participants will practice how to properly use a helmet and inspect the bicycle before riding, as well as fundamental cycling skills through 10 activities and a cycling circuit. The content will include proper helmet fitting, bicycle safety check before riding, fundamental cycling skills for starting and pedaling, braking safely, shifting gears, and hand signals for changing direction.
2. Closed Circuit Session (60 min—second day): During the second session, the knowledge about the use and maintenance of bicycles will be reviewed, in addition to the cycling skills practiced in session one for their corresponding evaluation. It is important to note that the cycle skills results will be highly dependent on participants’ prior cycling skills, but this session will help to reinforce the skills of those who do not cycle regularly.
The participating schools will provide the necessary materials for the Bikeability sessions, such as signaling objects (cones and hoops) to build the circuits. Regarding the methodology, the sessions will encourage student participation, and the language used for communication will be inclusive and adapted to them. Active participation of the students will be promoted through questions, fostering dialog, and providing meaningful individual and group feedback. Additionally, based on previous interventions (45), some alternative strategies will be considered for implementing the Bikeability methodology: (i) half of the students will perform the activities first, followed by the other half; (ii) students from one class can lend their bicycles to students from another class; (iii) some secondary schools have their own bicycles that are offered free of charge to students; and (iv) local transport organizations or transportation can be asked to lend bicycles for the intervention. Detailed visual information on the Bikeability sessions can be found at the following link.4
The second part of the intervention will consist of an eight-week program cycling to and from school. The duration of 8 weeks for school cycling is appropriate in this setting according to a previous systematic review (47). During the eight-week intervention, adolescents will receive an encouragement strategy every week in the form of a challenge related to knowledge content about the importance of PA and having good physical condition for our health, benefits of ACS, knowledge of RMR, PAEE, and its importance, promotion of confidence and motivation for adolescents to travel safely in their environment. The functioning of these encouragement strategies, called Octopus Strategy, will consist of eight games that will be opened weekly as the intervention progresses. In each of the games a series of points will be obtained, and these will be accumulated so that there will be a competition between the different participating schools. The winner of the Octopus strategy will be the participant who completes all the games during the 8 weeks. It is important to highlight that although motivational strategies will be employed to support adherence to the intervention, they are not intended to address broader behavior change processes, such as altering participants’ attitudes or intentions toward active commuting. Thus, the Octopus strategy will aim to improve the adherence and motivation to the intervention (i.e., to cycle at least 3 days to and from the school). Table 4 shows a summary of the project’s objectives related to the Bikeability sessions and the contents to be developed in the encouragement strategies, during the eight-week intervention. More information about the different challenges proposed by our research group as an encouragement strategy in the Supplementary material and in the following link.5
Cycling is generally recognized as a safe and well-tolerated form of PA for adolescents. However, any potential adverse events will be carefully monitored throughout the intervention period. Minor adverse events not directly related to the intervention (e.g., seasonal allergies, weather) and those commonly associated with PA (e.g., fatigue) will not be systematically recorded unless they lead to a modification of the intervention protocol (e.g., the participant is unable to cycle to and from school at least 3 days/week). In such cases, the principal investigator will review the situation and decide whether the participant can continue in the study. On the other hand, in the event of any significant health-related issues, such as a serious injury, these incidents will be assessed by the research team. Again, the principal investigator will review the case and make informed decisions regarding participant safety, including whether the participant can remain in the study. If the participant manages to recover in time and is able to cycle to and from school 3 days/week, he/she will not be allowed to return to the intervention without providing medical clearance to support their resumption of PA. The safety and well-being of participants remain the primary focus of the research team. All adverse events will be systematically monitored, recorded, and reported in future manuscripts to ensure transparency and accountability. To achieve this, the research team will maintain a dedicated document to capture details such as the type, duration, and resolution of adverse events.
In the ENERGYCO project, per-protocol and intention-to-treat (ITT) approaches, as well as sensitivity analyses, will be conducted. On one hand, the per-protocol approach will be used to present the main and secondary analysis. Participants from the intervention group who have cycled to and/or from school at least 3 days/week over 8 weeks will be included. Additionally, participants from both the intervention and control groups must have valid data at baseline and follow-up for the outcomes of interest (e.g., RMR and PAEE). On the other hand, the ITT approach will be used to present main and secondary analysis, but in the Supplementary material. This approach will include all participants in both the intervention and control groups who have valid data at baseline and follow-up or who have missing data at either baseline or follow-up. Finally, the potential impact of missing data will be assessed using various imputation models and sensitivity analyses. Specifically, we will conduct sensitivity analyses to determine whether the choice of imputation method affects the results. Additionally, no interim analyses will be performed.
Descriptive analyses of all study variables presented with the mean (standard deviation) and frequencies (percentage) will be performed. Subsequently, the normality of the variables will be analyzed using histograms and normality tests. In Phase I, for the comparison of PAEE between the different modes of commuting (i.e., walking, cycling, and motorized-vehicle), a repeated measures ANOVA will be performed using the per-protocol approach. In addition, Student t-test or Mann–Whitney tests will be performed to compare the fluctuation of PAEE according to speed or distance commuted between active modes of commuting (i.e., walking vs. cycling).Within Phase II, the effects of the school-based cycling intervention on RMR and other health-related outcomes will be studied using an analysis of covariance (ANCOVA). Post-intervention RMR and other-health related values will be included as dependent variables, group (i.e., experimental group vs. control group) as fixed factor, and baseline outcome data as covariates. To evaluate the effect of the intervention on the secondary outcomes (i.e., physiological, physical, and psychosocial outcomes), we will conduct the same analysis used for the main outcomes (i.e., ANCOVA analysis using the intention-to-treat approach). Moreover, to control the variability between schools, the analyses will include adjustments for contextual and organizational variables, such as the SES of the school area, the length of cycling lanes, the availability of bicycle parking, and the level of engagement of teachers and students in the intervention. The estimates will be present as raw and standardized (z-scores; i.e., mean differences between follow-up and baseline data divided by the standard deviation), as an indication of the change the post-intervention z-scores will be calculated relative to the baseline mean and standard deviation. Effect sizes will be categorized as small (0.2 SDs), medium (0.5 SDs), and large (0.8 SDs), based on Cohen (93). Finally, to analyze the influence of covariates on the association between the intervention and the main outcomes (i.e., RMR and PAEE), mediation analyses will be performed. The intervention will be included as the independent variable, and the main outcomes as the dependent variables. Covariates included in the mediation analysis will encompass body composition, physical function, cognitive determinants, perceived barriers or autonomy, built-environment characteristics, weather, among others. In the statistical analysis, a value of p < 0.05 will be considered statistically significant.
The ENERGYCO project will utilize three main systems to store and manage data. First, the Dropbox platform will be used to manage all project-related information, including monitoring documents, evaluation protocols, participant tracking sheets, adverse event control sheets, and raw data for all analyzed variables (both primary and secondary), organized into separate spreadsheets based on the variable being studied. Second, all data will be stored on an external hard drive, kept securely in the principal investigator’s office. The same data and folders stored in Dropbox will also be mirrored on this physical hard drive. Third, informed consent forms, paper-based questionnaires, and participant evaluation sheets will be collected and stored in paper format in the principal investigator’s office. To ensure participant anonymity, each individual will be assigned a unique code upon submitting their informed consent, which will be used in all data reports. The principal investigator will have continuous access to the final dataset and the research staff undertakes not to store on its computer any data related to the ENERGYCO project. Regarding data quality control, the process will include double-checking paper-based evaluation sheets and reviewing the ranges and valid values in the resulting spreadsheets. The research team will perform quality controls and archive the data following a pre-established protocol for both the baseline and follow-up phases. If any data quality issues are detected, the raw data stored in Dropbox or the hard drive will be consulted, along with the paper-based records, which will be reviewed as needed. It is important to highlight that the ENERGYCO project is committed to publishing its findings, whether positive, negative, or inconclusive, in peer-reviewed journals, at national and international conferences, and through public dissemination in local, regional, and national media outlets, as well as on social media platforms. Additionally, these results will be shared with participants and schools through individual reports for participants and comprehensive reports for the schools, including corresponding interpretations of the findings. Finally, eligibility for authorship will be determined by the principal investigator in accordance with the guidelines of the International Committee of Medical Journal Editors (ICMJE), which require substantial contributions to the conception, design, data acquisition, analysis, or interpretation, as well as drafting or critically revising the manuscript and approving the final version.
To the best of our knowledge, the ENERGYCO study will be the first study which will assess the PAEE related to different modes of commuting to school using indirect calorimetry. Moreover, the effects of an eight-week school-based cycling intervention on RMR and PAEE, as well as on other physiological (i.e., basal heart rate, blood pressure, and body temperature), physical (i.e., PA levels, anthropometric measures, body composition, physical fitness, and motor competence), and psychosocial outcomes(e.g., perceived barriers to active commute to school, self-esteem, and mental health) among Spanish adolescents aged will be assessed. The main objective of the study has potential individual implications for adolescents, as the school-based cycling intervention aims to explore its effects on RMR and daily PAEE, which may contribute to changes in PA levels and associated physical, psychosocial, and emotional aspects. However, individual variability and adherence challenges must be considered when interpreting the results. To support engagement, the study will provide easy-to-use encouragement strategies, such as infographics and motivational videos, to promote adherence to the intervention (i.e., cycling at least 3 days to and from school). While these materials may help sustain participation, their effectiveness in maintaining long-term engagement remains to be explored.
The findings of the ENERGYCO study will contribute to the current understanding of the potential effects of a school-based cycling intervention on RMR and daily PAEE among adolescents. Unlike most studies assessing ACS, PAEE, or PA levels, which often rely on self-reported tools, this study will use objective measurement techniques. Nevertheless, current research is incorporating objective devices such as GPS and accelerometry for more precise measurements (94). In this study, in addition to using these devices, indirect calorimetry technique will be employed, adding further value to the research. Furthermore, we will analyze the effects of a school-based cycling intervention on increasing RMR in adolescents, instead of focusing on a behavioral change. Indeed, most interventions in the school context have been focused on changing the behavior of adolescents (36–39), but even though such interventions are necessary, we still do not know in depth the effects on some health variables, especially on physiological outcomes such as RMR and PAEE. Therefore, the ENERGYCO study is expected to provide novel insights into the potential effects of a school-based cycling intervention on RMR and PAEE, along with other physiological, physical, and psychosocial outcomes. As research in this area remains limited, this study will contribute valuable data. Nevertheless, the findings should be interpreted in the context of the study’s duration, sample characteristics, and variability in adherence among participants. In this sense, and following the previous literature, interventions with a duration of 8 weeks could be enough to improve health markers in young people (95, 96).
Furthermore, studies focus on assessing the PAEE related to ACS in free living conditions have used accelerometer counts (20, 97) or heart rate (12) instead of indirect calorimetry. Indeed, as far as we know, two studies focus on young population used indirect calorimetry to measure the PAEE associated to different routine activities (98) or just walking in a treadmill (99), but both of them were carried out under laboratory conditions. The study by Schuna et al. (98) involved 106 American children and adolescents (6 to 18 years old) who completed a series of activities such as sitting, watching movies, coloring, climbing stairs, playing basketball, or jumping. Authors concluded that the lowest average PAEE values were associated with sitting and watching movies, while jumping was the activity that caused the highest average PAEE. While the study by Tudor-Locke et al. (99) evaluated the cadence produced by different walking speeds (an indirect measure of intensity) in young people aged 6 to 20 in a controlled trial. Participants completed multiple 5-min series on a treadmill, ranging from 0.80 km/h to 8.04 km/h. Steps were counted visually, and intensity was objectively measured using a portable metabolic system. Therefore, the ENERGYCO study will contribute to advancing knowledge on PAEE associated with different modes of commuting to school. To our knowledge, this will be the first study to objectively measure PAEE in adolescent commuters using indirect calorimetry in free-living conditions.
The ENERGYCO study aims to provide valuable insights for policymakers, educators, urban planners, and public health professionals by offering concrete recommendations for adapting and implementing similar interventions in different educational and urban settings. In the school context, integrating ACS into curricula through road safety education and awareness campaigns on the health benefits of cycling could enhance engagement. Policymakers should support these efforts by incorporating ACS modules into Physical Education programs and fostering collaborations between schools and local authorities to ensure safe commuting environments. From an urban planning perspective, expanding cycling infrastructure around schools, such as protected bike lanes, traffic-calming measures, and secure bicycle parking, should be prioritized, along with establishing urban design guidelines to improve the accessibility and safety of school routes. Public health initiatives should encourage community-based interventions, including bike-to-school programs, incentive schemes for cycling equipment, and partnerships with local businesses to create cycling-friendly environments. Regarding scalability and sustainability, after the completion of the ENERGYCO study, a comprehensive review of the collected data will be conducted to explore how a more cost-effective assessment approach could be developed. Given the logistical challenges of school settings, this analysis will focus on identifying ways to streamline data collection while maintaining methodological rigor, ensuring feasibility for future studies. The goal will be to refine the assessment of RMR, PAEE, and secondary outcomes in a real-world school environment. To maximize impact, the ENERGYCO study will disseminate its findings through infographics and policy briefs tailored to families, schools, municipalities, and public health entities, ensuring accessibility and usability for decision-makers.
It is important to mention that the ENERGYCO protocol presents some limitations and risks that may arise in the future. These limitations will be: (i) some participants may not attend the assessments, which could lead to a small sample size; (ii) the relatively small sample size in Phase I, which could potentially limit statistical power and generalizability. The sample size was determined based on previous studies using indirect calorimetry in similar research contexts. However, the complexity of the data collection process posed significant challenges for recruitment; (iii) important outcomes such as distance or barriers to cycling to school may also influence the recruitment process, resulting in a low-rate recruitment; (iv) there may be problems with the selection of schools. In Phase II, after the selection of schools, it is possible that there may not be enough students to form each of the groups to low prevalence of cycling to and from school in Spain (46); (v) the family is not involved in the intervention, uncertainties surrounding the help that the school can give to the research group for the success of the intervention (e.g., contact with adolescents). On the other hand, the ENERGYCO protocol presents several strengths. The study design is a cluster randomized controlled trial, as well as the duration of 8 weeks of the school-based cycling intervention, including strategies for maintaining adherence and motivation, and an incentive system. The use of instruments gold standard, such as the Omnical for assessing the RER, or the use of indirect calorimetry for measuring the PAEE related to different modes of commuting will provide accurate data for these variables.
The ENERGYCO study is designed to advance the understanding of cycling to school and its potential impact on physiological variables through an innovative two-phase structure. Phase I aims to quantify the energy demands of different commuting modes using out-of-laboratory gas exchange instruments, providing objective data on the physiological cost of cycling to school. The results from Phase I may offer valuable insights for schools, families, and practitioners, informing strategies to address sedentary behaviors and promote sustainable ACS, such as walking and cycling to school. Phase II will implement an eight-week school-based cycling intervention targeting Spanish adolescents. This phase will assess its potential impact on a range of outcomes, including physiological, physical, psychosocial, and emotional dimensions. By providing objective data on the short-term physiological effects of cycling to school, the ENERGYCO study aims to contribute to the evidence base supporting ACS strategies for adolescents. These results may help inform effective policies and practices, offering guidance to teachers, families, policymakers, and the clinical sector in implementing scalable and sustainable ACS-focused interventions. Finally, the ENERGYCO study aspires to contribute meaningfully to adolescent health and well-being, encouraging healthier and more active communities.
The ENERGYCO study has been approved by the Review Committee for Research Involving Human Subjects at the University of Granada (Reference: 2496/CEIH/2021) and registered with a ClinicalTrials.gov ID: NCT06414668. In addition, the present study protocol has been written in accordance with the Standard Protocol Items: Recommendations for Interventional Trials (SPIRIT) statement (See Supplementary material 1).
PC-G: Conceptualization, Investigation, Methodology, Project administration, Resources, Supervision, Writing – original draft, Writing – review & editing. VV-M: Investigation, Resources, Writing – original draft. JS-D: Investigation, Resources, Writing – original draft. MÁ-G: Investigation, Resources, Writing – review & editing. RS-A: Investigation, Writing – review & editing. AR-A: Investigation, Writing – review & editing. FL-C: Investigation, Resources, Writing – review & editing. UP: Investigation, Methodology, Resources, Writing – review & editing. JA: Methodology, Writing – review & editing. LM-C: Investigation, Writing – review & editing. DR-S: Investigation, Resources, Writing – review & editing. AR-O: Investigation, Resources, Writing – review & editing. MC-B: Investigation, Resources, Writing – review & editing. EÁ-L: Investigation, Resources, Writing – review & editing. FH-D: Conceptualization, Investigation, Methodology, Writing – review & editing. MH-C: Conceptualization, Investigation, Methodology, Writing – review & editing. SM: Conceptualization, Investigation, Methodology, Writing – review & editing. PC: Conceptualization, Investigation, Methodology, Writing – review & editing. YB-R: Conceptualization, Investigation, Methodology, Supervision, Writing – review & editing. EV-G: Conceptualization, Funding acquisition, Investigation, Methodology, Resources, Supervision, Writing – original draft, Writing – review & editing.
The author(s) declare that financial support was received for the research and/or publication of this article. This study is funded by grant PID2021.126126OA.I00, MICIU/AEI/ 10.13039/501100011033, and “ERDF/EU.” Also, this study obtained funding from the University of Granada, Plan Propio de Investigación 2016-Excellence actions: Unit of Excellence on Exercise and Health (UCEES). Moreover, JA is supported by the Juan de la Cierva Formación Grant (reference: FJC2020-044453-I) funded by MCIN/AEI/10.13039/501100011033 and “European Union NextGenerationEU/PRTR. In addition, AR-A is supported by the Spanish Ministry of Universities (FPU21/02460).
The authors would like to acknowledge the members of the ENERGYCO Study and all participating schools, students, parents, teachers, and school principals.
SM was employed by the company AGILE Research Ltd.
The remaining authors declare that the research was conducted in the absence of any commercial or financial relationships that could be construed as a potential conflict of interest.
The reviewer PR-V declared a shared affiliation with the author JA to the Handling Editor at the time of review.
All claims expressed in this article are solely those of the authors and do not necessarily represent those of their affiliated organizations, or those of the publisher, the editors and the reviewers. Any product that may be evaluated in this article, or claim that may be made by its manufacturer, is not guaranteed or endorsed by the publisher.
The Supplementary material for this article can be found online at: https://www.frontiersin.org/articles/10.3389/fpubh.2025.1467227/full#supplementary-material
1. ^https://www.youtube.com/watch?v=uI6nhUUB19Q
2. ^http://profith.ugr.es/temperatus?lang=en
3. ^http://profith.ugr.es/recursos-paco
4. ^https://drive.google.com/file/d/13vT0QELbFa_itJETI4hHWaBE4QI-f7-T/view?usp=drive_link
5. ^https://view.genial.ly/65a91b8a54833300148e0790/guide-mision-octopus
1. Lam, YY, and Ravussin, E. Analysis of energy metabolism in humans: a review of methodologies. Mol Metab. (2016) 5:1057–71. doi: 10.1016/j.molmet.2016.09.005
2. Molnár, D, and Schutz, Y. The effect of obesity, age, puberty and gender on resting metabolic rate in children and adolescents. Eur J Pediatr. (1997) 156:376–81. doi: 10.1007/s004310050618
3. Johnstone, AM, Murison, SD, Duncan, JS, Rance, KA, and Speakman, JR. Factors influencing variation in basal metabolic rate include fat-free mass, fat mass, age, and circulating thyroxine but not sex, circulating leptin, or triiodothyronine. Am J Clin Nutr. (2005) 82:941–8. doi: 10.1093/ajcn/82.5.941
4. Bray, GA, Frühbeck, G, Ryan, DH, and Wilding, JPH. Management of obesity. Lancet. (2016) 387:1947–56. doi: 10.1016/S0140-6736(16)00271-3
5. Alberga, AS, Prudhomme, D, Sigal, RJ, Goldfield, GS, Hadjiyannakis, S, Gougeon, R, et al. Does exercise training affect resting metabolic rate in adolescents with obesity? Appl Physiol Nutr Metab. (2017) 42:15–22. doi: 10.1139/apnm-2016-0244
6. Yu, WW, Lee, S, Arslanian, S, Tamim, H, and Kuk, JL. Effects of exercise on resting metabolic rate in adolescents with overweight and obesity. Child Obes. (2021) 17:249–56. doi: 10.1089/chi.2020.0280
7. Caspersen, CJ, Powell, KE, and Christenson, GM. Physical activity, exercise, and physical fitness: definitions and distinctions for health-related research. Public Health Rep. (1985) 100:126–31.
8. The GBD2015 Obesity Collaborators. Health effects of overweight and obesity in 195 countries over 25 years. N Engl J Med. (2017) 377:13–27. doi: 10.1056/NEJMoa1614362
9. Abarca-Gómez, L, Abdeen, ZA, Hamid, ZA, and Abu-Rmeileh, NM. Worldwide trends in body-mass index, underweight, overweight, and obesity from 1975 to 2016: a pooled analysis of 2416 population-based measurement studies in 128·9 million children, adolescents, and adults. Lancet. (2017) 390:2627–42. doi: 10.1016/S0140-6736(17)32129-3
10. Bull, FC, Al-Ansari, SS, Biddle, S, Borodulin, K, Buman, MP, Cardon, G, et al. World Health Organization 2020 guidelines on physical activity and sedentary behaviour. Br J Sports Med. (2020) 54:1451–62. doi: 10.1136/bjsports-2020-102955
11. Chu, DT, Minh Nguyet, NT, Dinh, TC, Thai Lien, NV, Nguyen, KH, Nhu Ngoc, VT, et al. An update on physical health and economic consequences of overweight and obesity. Diabetes Metab Syndr Clin Res Rev. (2018) 12:1095–100. doi: 10.1016/j.dsx.2018.05.004
12. Slingerland, M, Borghouts, LB, and Hesselink, MKC. Physical activity energy expenditure in Dutch adolescents: contribution of active transport to school, physical education, and leisure time activities. J Sch Health. (2012) 82:225–32. doi: 10.1111/j.1746-1561.2012.00691.x
13. Cook, S, Stevenson, L, Aldred, R, Kendall, M, and Cohen, T. More than walking and cycling: what is ‘active travel’? Transp Policy (Oxf). (2022) 126:151–61. doi: 10.1016/j.tranpol.2022.07.015
14. Larouche, R, Saunders, TJ, John Faulkner, GE, Colley, R, and Tremblay, M. Associations between active school transport and physical activity, body composition, and cardiovascular fitness: a systematic review of 68 studies. J Phys Act Health. (2014) 11:206–27. doi: 10.1123/jpah.2011-034
15. Khan, A, Mandic, S, and Uddin, R. Association of active school commuting with physical activity and sedentary behaviour among adolescents: a global perspective from 80 countries. J Sci Med Sport. (2021) 24:567–72. doi: 10.1016/j.jsams.2020.12.002
16. Kek, CC, García Bengoechea, E, Spence, JC, Mandic, S, Bengoechea, EG, Spence, JC, et al. The relationship between transport-to-school habits and physical activity in a sample of New Zealand adolescents. J Sport Health Sci. (2019) 8:463–70. doi: 10.1016/j.jshs.2019.02.006
17. Campos-Garzón, P, Stewart, T, Palma-Leal, X, Molina-García, J, Herrador-Colmenero, M, Schipperijn, J, et al. Are Spanish adolescents who actively commute to and from school more active in other domains? A spatiotemporal investigation. Health Place. (2024) 86:103211. doi: 10.1016/j.healthplace.2024.103211
18. Stewart, T, Duncan, S, and Schipperijn, J. Adolescents who engage in active school transport are also more active in other contexts: a space-time investigation. Health Place. (2017) 43:25–32. doi: 10.1016/j.healthplace.2016.11.009
19. Campos-Garzón, P, Sevil-Serrano, J, García-Hermoso, A, Chillón, P, and Barranco-Ruiz, Y. Contribution of active commuting to and from school to device‐measured physical activity levels in young people: a systematic review and meta‐analysis. Scandinavian J Med Sci Sports. (2023) 33:2110–24. doi: 10.1111/sms.14450
20. Campos-Garzon, P, Amholt, TT, Molina-Soberanes, D, Palma-Leal, X, Queralt, A, Lara-Sanchez, AJ, et al. Do physical activity and trip characteristics differ when commuting to and from school?: the PACO study. Travel Behav Soc. (2023) 33:100618. doi: 10.1016/j.tbs.2023.100618
21. Lubans, DR, Boreham, CA, Kelly, P, and Foster, CE. The relationship between active travel to school and health-related fitness in children and adolescents: a systematic review. Int J Behav Nutr Phys Act. (2011) 8:5. doi: 10.1186/1479-5868-8-5
22. Mendoza, JA, Watson, K, Nguyen, N, Cerin, E, Baranowski, T, and Nicklas, TA. Active commuting to school and association with physical activity and adiposity among US youth. J Phys Act Health. (2011) 8:488–95. doi: 10.1123/jpah.8.4.488
23. Ramirez-Velez, R, Garcia-Hermoso, A, Agostinis-Sobrinho, C, Mota, J, Santos, R, Correa-Bautista, JE, et al. Cycling to school and body composition, physical fitness, and metabolic syndrome in children and adolescents. J Pediatr. (2017) 188:57–63. doi: 10.1016/j.jpeds.2017.05.065
24. Villa-Gonzalez, E, Ruiz, JR, Ward, DS, Chillon, P, Villa-González, E, Ruiz, JR, et al. Effectiveness of an active commuting school-based intervention at 6-month follow-up. Eur J Pub Health. (2016) 26:272–6. doi: 10.1093/eurpub/ckv208
25. Mandic, S, Hopkins, D, García Bengoechea, E, Flaherty, C, Williams, J, Sloane, L, et al. Adolescents’ perceptions of cycling versus walking to school: understanding the New Zealand context. J Transp Health. (2017) 4:294–304. doi: 10.1016/j.jth.2016.10.007
26. Chen, KY, Smith, S, Ravussin, E, Krakoff, J, Plasqui, G, Tanaka, S, et al. Room indirect calorimetry operating and reporting standards (RICORS 1.0): a guide to conducting and reporting human whole-room calorimeter studies. Obesity. (2020) 28:1613–25. doi: 10.1002/oby.22928
27. Macfarlane, DJ. Open-circuit respirometry: a historical review of portable gas analysis systems. Eur J Appl Physiol. (2017) 117:2369–86. doi: 10.1007/s00421-017-3716-8
28. Riebe, D, Ehrman, JK, and Magal, M. American college of sports medicine. ACSM’s guidelines for exercise testing and prescription. 10th edn. Filadelfia, PA: Wolters Kluwer (2018).
29. Pandolf, KB, Givoni, B, and Goldman, RF. Predicting energy expenditure with loads while standing or walking very slowly. J Appl Physiol. (1977) 43:577–81. doi: 10.1152/jappl.1977.43.4.577
30. Olsen, JR, Leung, KYK, Nicholls, N, and Loo, BPY. Do neighbourhood characteristics matter in understanding school children’s active lifestyles? A cross-region multi-city comparison of Glasgow, Edinburgh and Hong Kong. Child Geogr. (2021) 19:488–504. doi: 10.1080/14733285.2020.1828826
31. Villa-Gonzalez, E, Rosado-Lopez, S, Barranco-Ruiz, Y, Herrador-Colmenero, M, Cadenas-Sanchez, C, Santos, MP, et al. Objective measurement of the mode of commuting to school using GPS: a pilot study. Sustainability (Switzerland). (2019) 11:1–12. doi: 10.3390/su11195395
32. Jeran, S, Steinbrecher, A, and Pischon, T. Prediction of activity-related energy expenditure using accelerometer-derived physical activity under free-living conditions: a systematic review. Int J Obesity Nature Publishing Group. (2016) 40:1187–97. doi: 10.1038/ijo.2016.14
33. Payne, C, Smith, SA, Sappal, A, Boorgula, R, and Taylor, KA. Are e-scooters active transport? Measured physical activity outputs of e-scooter riding vs walking. J Transp Health. (2025) 41:101963. doi: 10.1016/j.jth.2024.101963
34. Wei, YD, Xiao, W, and Garcia, I. Neighborhood environment, active commute, and healthy BMI in adolescents. Sustain For. (2021) 13:8286. doi: 10.3390/su13158286
35. Herrador-Colmenero, M, Villa-González, E, and Chillón, P. Children who commute to school unaccompanied have greater autonomy and perceptions of safety. Acta Paediatr. (2017) 106:2042–7. doi: 10.1111/apa.14047
36. Larouche, R, Mammen, G, Rowe, DA, and Faulkner, G. Effectiveness of active school transport interventions: a systematic review and update. BMC Public Health. (2018) 18:206. doi: 10.1186/s12889-017-5005-1
37. Pang, B, Kubacki, K, and Rundle-Thiele, S. Promoting active travel to school: a systematic review (2010-2016). BMC Public Health. (2017) 17:638. doi: 10.1186/s12889-017-4648-2
38. Sersli, S, Rothman, L, and Winters, M. Getting at mode share: comparing 3 methods of travel mode measurement for school travel research. J Sch Health. (2019) 89:365–72. doi: 10.1111/josh.12743
39. Villa-González, E, Barranco-Ruiz, Y, Evenson, KR, Chillón, P, Villa-Gonzalez, E, Barranco-Ruiz, Y, et al. Systematic review of interventions for promoting active school transport. Prev Med (Baltim). (2018) 111:115–34. doi: 10.1016/j.ypmed.2018.02.010
40. Cohen, D, Ogunleye, AA, Taylor, M, Voss, C, Micklewright, D, and Sandercock, GRH. Association between habitual school travel and muscular fitness in youth. Prev Med (Baltim). (2014) 67:216–20. doi: 10.1016/j.ypmed.2014.07.036
41. Nunes de Oliveira, B, Minatto, G, Galdino da Costa, BG, Veber Lopes, MV, and Samara da Silva, K. Association between psychosocial factors and active commuting to school in Brazilian adolescents. J Transp Health. (2020) 19:100964. doi: 10.1016/j.jth.2020.100964
42. Carlson, JA, Steel, C, Bejarano, C, Grimes, A, Shook, RP, Reddy, A, et al. Impacts of an earn-a-bike cycling education program on children’s time spent cycling. J Transp Health. (2020) 16:100826. doi: 10.1016/j.jth.2020.100826
43. Børrestad, LABB, Østergaard, L, Andersen, LB, Bere, E, Borrestad, LAB, Ostergaard, L, et al. Experiences from a randomised, controlled trial on cycling to school: does cycling increase cardiorespiratory fitness? Scand J Public Health. (2012) 40:245–52. doi: 10.1177/1403494812443606
44. Faul, F, Erdfelder, E, Lang, AG, and Buchner, A. G*power 3: a flexible statistical power analysis program for the social, behavioral, and biomedical sciences. Behav Res Methods. (2007) 39:175–91. doi: 10.3758/BF03193146
45. Chillon, P, Galvez-Fernandez, P, Huertas-Delgado, FJ, Herrador-Colmenero, M, Barranco-Ruiz, Y, Villa-Gonzalez, E, et al. A school-based randomized controlled trial to promote cycling to School in Adolescents: the PACO study. Int J Environ Res Public Health. (2021) 18:1–20. doi: 10.3390/ijerph18042066
46. Gálvez-Fernández, P, Herrador-Colmenero, M, Esteban-Cornejo, I, Castro-Piñero, J, Molina-García, J, Queralt, A, et al. Active commuting to school among 36,781 Spanish children and adolescents: a temporal trend study. Scand J Med Sci Sports. (2021) 31:914–24. doi: 10.1111/sms.13917
47. Schoenbach, DMI, Altenburg, TM, Marques, A, Chinapaw, MJM, Demetriou, Y, Schönbach, DMI, et al. Strategies and effects of school-based interventions to promote active school transportation by bicycle among children and adolescents: a systematic review. Int J Behav Nutr Phys Act. (2020) 17:138. doi: 10.1186/s12966-020-01035-1
48. Villa-Gonzalez, E, Ruiz, JR, Mendoza, JA, Chillon, P, Villa-González, E, Ruiz, JR, et al. Effects of a school-based intervention on active commuting to school and health-related fitness. BMC Public Health. (2017) 17:20. doi: 10.1186/s12889-016-3934-8
49. Huang, C, Dannenberg, AL, Haaland, W, and Mendoza, JA. Changes in self-efficacy and outcome expectations from child participation in bicycle Trains for Commuting to and from school. Health Educ Behav. (2018) 45:748–55. doi: 10.1177/1090198118769346
50. Mendoza, JA, Haaland, W, Jacobs, M, Abbey-Lambertz, M, Miller, J, Salls, D, et al. Bicycle trains, cycling, and physical activity: a pilot cluster RCT. Am J Prev Med. (2017) 53:481–9. doi: 10.1016/j.amepre.2017.05.001
51. Bors, P, Dessauer, M, Bell, R, Wilkerson, R, Lee, J, and Strunk, SL. The active living by design National Program. Am J Prev Med. (2009) 37:S313–21. doi: 10.1016/j.amepre.2009.09.027
52. Rodriguez-Lopez, C, Salas-Farina, ZM, Villa-Gonzalez, E, Borges-Cosic, M, Herrador-Colmenero, M, Medina-Casaubon, J, et al. The threshold distance associated with walking from home to school. Health Educ Behav. (2017) 44:857–66. doi: 10.1177/1090198116688429
53. Loh, V, Sahlqvist, S, Veitch, J, Thornton, L, Salmon, J, Cerin, E, et al. From motorised to active travel: using GPS data to explore potential physical activity gains among adolescents. BMC Public Health. (2022) 22:1512. doi: 10.1186/s12889-022-13947-7
54. Alcantara, JMA, Galgani, JE, Jurado-Fasoli, L, Dote-Montero, M, Merchan-Ramirez, E, Ravussin, E, et al. Validity of four commercially available metabolic carts for assessing resting metabolic rate and respiratory exchange ratio in non-ventilated humans. Clin Nutr. (2022) 41:746–54. doi: 10.1016/j.clnu.2022.01.031
55. Acar-Tek, N, Ağagündüz, D, Şahin, TÖ, Baygut, H, Uzunlar, EA, Zakkour, HK, et al. Validation of predictive equations for resting energy expenditure in children and adolescents with different body mass indexes. Nutr J. (2023) 22:39. doi: 10.1186/s12937-023-00868-3
56. Perez-Suarez, I, Martin-Rincon, M, Gonzalez-Henriquez, JJ, Fezzardi, C, Perez-Regalado, S, Galvan-Alvarez, V, et al. Accuracy and precision of the COSMED K5 portable Analyser. Front Physiol. (2018) 9:9. doi: 10.3389/fphys.2018.01764
57. Crouter, SE, LaMunion, SR, Hibbing, PR, Kaplan, AS, and Bassett, DR. Accuracy of the Cosmed K5 portable calorimeter. PLoS One. (2019) 14:e0226290. doi: 10.1371/journal.pone.0226290
58. Gilgen-Ammann, R, Schweizer, T, and Wyss, T. RR interval signal quality of a heart rate monitor and an ECG Holter at rest and during exercise. Eur J Appl Physiol. (2019) 119:1525–32. doi: 10.1007/s00421-019-04142-5
59. Moraes, LI, Nicola, TC, Jesus, JS, Alves, ER, Giovaninni, NP, Marcato, DG, et al. High blood pressure in children and its correlation with three definitions of obesity in childhood. Arq Bras Cardiol. (2013) 102:175–80. doi: 10.5935/abc.20130233
60. Smith, ADH, Crabtree, DR, Bilzon, JLJ, and Walsh, NP. The validity of wireless iButtons® and thermistors for human skin temperature measurement. Physiol Meas. (2010) 31:95–114. doi: 10.1088/0967-3334/31/1/007
61. Martinez-Tellez, B, Quesada-Aranda, A, Sanchez-Delgado, G, Fernández-Luna, JM, and Ruiz, JR. Temperatus® software: a new tool to efficiently manage the massive information generated by iButtons. Int J Med Inform. (2019) 126:9–18. doi: 10.1016/j.ijmedinf.2019.03.007
62. Migueles, JH, Rowlands, AV, Huber, F, Sabia, S, and van Hees, VT. GGIR: a research community–driven open source R package for generating physical activity and sleep outcomes from multi-day raw accelerometer data. J Meas Phys Behav. (2019) 2:188–96. doi: 10.1123/jmpb.2018-0063
63. van Hees, VT, Renström, F, Wright, A, Gradmark, A, Catt, M, Chen, KY, et al. Estimation of daily energy expenditure in pregnant and non-pregnant women using a wrist-worn tri-axial accelerometer. PLoS One. (2011) 6:e22922. doi: 10.1371/journal.pone.0022922
64. Neishabouri, A, Nguyen, J, Samuelsson, J, Guthrie, T, Biggs, M, Wyatt, J, et al. Quantification of acceleration as activity counts in ActiGraph wearable. Sci Rep. (2022) 12:11958. doi: 10.1038/s41598-022-16003-x
65. Evenson, KR, Catellier, DJ, Gill, K, Ondrak, KS, and McMurray, RG. Calibration of two objective measures of physical activity for children. J Sports Sci. (2008) 26:1557–65. doi: 10.1080/02640410802334196
66. Segura-Díaz, JM, Barranco-Ruiz, Y, Saucedo-Araujo, RG, Aranda-Balboa, MJ, Cadenas-Sanchez, C, Migueles, JH, et al. Feasibility and reliability of the Spanish version of the youth activity profile questionnaire (YAP-Spain) in children and adolescents. J Sports Sci. (2021) 39:801–7. doi: 10.1080/02640414.2020.1847488
67. Léger, LA, Mercier, D, Gadoury, C, and Lambert, J. The multistage 20 metre shuttle run test for aerobic fitness. J Sports Sci. (1988) 6:93–101. doi: 10.1080/02640418808729800
68. Ruiz, JR, Silva, G, Oliveira, N, Ribeiro, JC, Oliveira, JF, and Mota, J. Criterion-related validity of the 20-m shuttle run test in youths aged 13–19 years. J Sports Sci. (2009) 27:899–906. doi: 10.1080/02640410902902835
69. Moeskops, S, Oliver, JL, Read, PJ, Cronin, JB, Myer, GD, Haff, GG, et al. Within- and between-session reliability of the isometric Midthigh pull in young female athletes. J Strength Cond Res. (2018) 32:1892–901. doi: 10.1519/JSC.0000000000002566
70. Ruiz, JR, Castro-Piñero, J, España-Romero, V, Artero, EG, Ortega, FB, Cuenca, MM, et al. Field-based fitness assessment in young people: the ALPHA health-related fitness test battery for children and adolescents. Br J Sports Med. (2011) 45:518–24. doi: 10.1136/bjsm.2010.075341
71. Artero, EG, España-Romero, V, Castro-Piñero, J, Ortega, FB, Suni, J, Castillo-Garzon, MJ, et al. Reliability of field-based fitness tests in youth. Int J Sports Med. (2011) 32:159–69. doi: 10.1055/s-0030-1268488
72. Comfort, P, PaulA, J, McMahon, JJ, and Newton, R. Effect of knee and trunk angle on kinetic variables during the isometric Midthigh pull: test–retest reliability. Int J Sports Physiol Perform. (2015) 10:58–63. doi: 10.1123/ijspp.2014-0077
73. López-Gil, JF, Martínez-Vizcaíno, V, Tárraga-López, PJ, and García-Hermoso, A. Cross-cultural adaptation, reliability, and validation of the Spanish perceived physical literacy instrument for adolescents (S-PPLI). J Exerc Sci Fit. (2023) 21:246–52. doi: 10.1016/j.jesf.2023.03.002
74. Deitz, JC, Kartin, D, and Kopp, K. Review of the Bruininks-Oseretsky test of motor proficiency, second edition (BOT-2). Phys Occup Ther Pediatr. (2007) 27:87–102. doi: 10.1080/J006v27n04_06
75. Radanović, D, Đorđević, D, Stanković, M, Pekas, D, Bogataj, Š, and Trajkovic, N. Test of motor proficiency second edition (BOT-2) short form: a systematic review of studies conducted in healthy children. Child Aust. (2021) 8:787. doi: 10.3390/children8090787
76. Cole, TJ, Flegal, KM, Nicholls, D, and Jackson, AA. Body mass index cut offs to define thinness in children and adolescents: international survey. BMJ. (2007) 335:194. doi: 10.1136/bmj.39238.399444.55
77. Moreira, OC, De, OCEP, and De Paz, JA. Dual energy X-ray absorptiometry (DXA) reliability and intraobserver reproducibility for segmental body composition measuring. Nutr Hosp. (2018) 35:340–5. doi: 10.20960/nh.1295
78. Shuhart, CR, Yeap, SS, Anderson, PA, Jankowski, LG, Lewiecki, EM, Morse, LR, et al. Executive summary of the 2019 ISCD position development conference on monitoring treatment, DXA Cross-calibration and least significant change, spinal cord injury, Peri-prosthetic and orthopedic bone health, transgender medicine, and pediatrics. J Clin Densitom. (2019) 22:453–71. doi: 10.1016/j.jocd.2019.07.001
79. Molina-Garcia, J, Queralt, A, Estevan, I, Alvarez, O, and Castillo, I. Perceived bafflers to active commuting to school: reliability and validity of a scale. Gac Sanit. (2016) 30:426–31. doi: 10.1016/j.gaceta.2016.05.006
80. Huertas-Delgado, FJ, Molina-Garcia, J, Van Dyck, D, and Chinon, P. A questionnaire to assess parental perception of barriers towards active commuting to school (PABACS): reliability and validity. J Transp Health. (2019) 12:97–104. doi: 10.1016/j.jth.2018.12.004
81. Burgueno, R, Gonzalez-Cutre, D, Sevil-Serrano, J, Herrador-Colmenero, M, Segura-Diaz, JM, Medina-Casaubon, J, et al. Validation of the basic psychological need satisfaction in active commuting to and from school (BPNS-ACS) scale in Spanish young people. J Transp Health. (2020) 16:1–11. doi: 10.1016/j.jth.2020.100825
82. Burgueño, R, González-Cutre, D, Sevil-Serrano, J, Herrador-Colmenero, M, Segura-Díaz, JM, Medina-Casaubón, J, et al. Understanding the motivational processes involved in adolescents’ active commuting behaviour: development and validation of the Behavioural regulation in active commuting to and from school (BR-ACS) questionnaire. Transp Res Part F Traffic Psychol Behav. (2019) 62:615–25. doi: 10.1016/j.trf.2019.02.016
83. Burgueño, R, and Medina-Casaubón, J. Validity and reliability of the interpersonal behaviors questionnaire in physical education with Spanish secondary school students. Percept Mot Skills. (2021) 128:522–45. doi: 10.1177/0031512520948286
84. Gómez-Lugo, M, Espada, JP, Morales, A, Marchal-Bertrand, L, Soler, F, and Vallejo-Medina, P. Adaptation, validation, reliability and factorial equivalence of the Rosenberg self-esteem scale in Colombian and Spanish population. Span J Psychol. (2016) 19:E66. doi: 10.1017/sjp.2016.67
85. Thompson, ER. Development and validation of an internationally reliable short-form of the positive and negative affect schedule (PANAS). J Cross-Cult Psychol. (2007) 38:227–42. doi: 10.1177/0022022106297301
86. Segura-Díaz, JM, Rojas-Jiménez, Á, Barranco-Ruiz, Y, Murillo-Pardo, B, Saucedo-Araujo, RG, Aranda-Balboa, MJ, et al. Feasibility and reliability of a questionnaire to assess the mode, frequency, distance and time of commuting to and from school: the PACO study. Int J Environ Res Public Health. (2020) 17:1–14. doi: 10.3390/ijerph17145039
87. Chillón, P, Herrador-Colmenero, M, Migueles, J, Cabanas-Sánchez, V, Fernández-Santos, J, Veiga, O, et al. Convergent validation of a questionnaire to assess the mode and frequency of commuting to and from school. Scand J Public Health. (2017) 45:612–20. doi: 10.1177/1403494817718905
88. Salto-Ruiz, C, Aranda-Balboa, MJ, Gálvez-Fernández, P, Herrador-Colmenero, M, and Chillón, P. Proyecto de innovación educativa para la eso“manual de intervención bikeability. Habilidad Motriz: revista de ciencias de la actividad física y del deporte. (2019) 52:12–38.
89. Torsheim, T, Cavallo, F, Levin, KA, Schnohr, C, Mazur, J, Niclasen, B, et al. Psychometric validation of the revised family affluence scale: a latent variable approach. Child Indic Res. (2016) 9:771–84. doi: 10.1007/s12187-015-9339-x
90. Martinez-Tellez, B, Perez-Bey, A, Sanchez-Delgado, G, Acosta, FM, Corral-Perez, J, Amaro-Gahete, FJ, et al. Concurrent validity of supraclavicular skin temperature measured with iButtons and infrared thermography as a surrogate marker of brown adipose tissue. J Therm Biol. (2019) 82:186–96. doi: 10.1016/j.jtherbio.2019.04.009
91. Molina-Garcia, J, Queralt, A, Molina-García, J, and Queralt, A. Neighborhood built environment and socioeconomic status in relation to active commuting to School in Children. J Phys Act Health. (2017) 14:761–5. doi: 10.1123/jpah.2017-0033
92. Chillón, P, Molina-García, J, Castillo, I, and Queralt, A. What distance do university students walk and bike daily to class in Spain. J Transp Health. (2016) 3:315–20. doi: 10.1016/j.jth.2016.06.001
94. Campos-Garzón, P, Saucedo-Araujo, RG, Sevil-Serrano, J, Migueles, JH, Barranco-Ruiz, Y, and Chillón, P. A systematic review in device-measured physical activity during active commuting to/from school: practical considerations to assess when, where, and how much it occurs. Transp Rev. (2023) 43:888–913. doi: 10.1080/01441647.2023.2175276
95. Cataldi, S, Francavilla, VC, Bonavolontà, V, De Florio, O, Carvutto, R, De Candia, M, et al. Proposal for a fitness program in the school setting during the COVID 19 pandemic: effects of an 8-week CrossFit program on psychophysical well-being in healthy adolescents. Int J Environ Res Public Health. (2021) 18:3141. doi: 10.3390/ijerph18063141
96. Gmmash, A, Alonazi, A, Almaddah, M, Alkhateeb, A, Sabir, O, and Alqabbani, S. Influence of an 8-week exercise program on physical, emotional, and mental health in Saudi adolescents: a pilot study. Medicina (B Aires). (2023) 59:883. doi: 10.3390/medicina59050883
97. Tudor-Locke, C, Ainsworth, BE, Adair, LS, and Popkin, BM. Objective physical activity of Filipino youth stratified for commuting mode to school. Med Sci Sports Exerc. (2003) 35:465–71. doi: 10.1249/01.MSS.0000053701.30307.A6
98. Schuna, JM, Barreira, TV, Hsia, DS, Johnson, WD, and Tudor-Locke, C. Youth energy expenditure during common free-living activities and treadmill walking. J Phys Act Health. (2016) 13:S29–34. doi: 10.1123/jpah.2015-0728
Keywords: youth, active transport, cycling, resting metabolic rate, energy expenditure
Citation: Campos-Garzón P, Valle-Muñoz VM, Segura-Díaz JM, Ávila-García M, Saucedo-Araujo RG, Ruiz-Alarcón A, López-Centeno FD, Pérez De Arrilucea Le Floc’h UA, Alcantara JMA, Medel-Carbonell LM, Rodriguez-Sanchez D, Ramírez-Osuna A, Castillo-Barragán M, Águila-Lara E, Huertas-Delgado FJ, Herrador-Colmenero M, Mandic S, Chillón P, Barranco-Ruiz Y and Villa-González E (2025) ENERGY expenditure of COmmuting to school (ENERGYCO): protocol for a cluster randomized controlled trial. Front. Public Health. 13:1467227. doi: 10.3389/fpubh.2025.1467227
Received: 19 July 2024; Accepted: 20 February 2025;
Published: 14 March 2025.
Edited by:
Nicholas P. Hays, Nestle, SwitzerlandReviewed by:
Robinson Ramírez-Vélez, Public University of Navarre, SpainCopyright © 2025 Campos-Garzón, Valle-Muñoz, Segura-Díaz, Ávila-García, Saucedo-Araujo, Ruiz-Alarcón, López-Centeno, Pérez De Arrilucea Le Floc’h, Alcantara, Medel-Carbonell, Rodriguez-Sanchez, Ramírez-Osuna, Castillo-Barragán, Águila-Lara, Huertas-Delgado, Herrador-Colmenero, Mandic, Chillón, Barranco-Ruiz and Villa-González. This is an open-access article distributed under the terms of the Creative Commons Attribution License (CC BY). The use, distribution or reproduction in other forums is permitted, provided the original author(s) and the copyright owner(s) are credited and that the original publication in this journal is cited, in accordance with accepted academic practice. No use, distribution or reproduction is permitted which does not comply with these terms.
*Correspondence: Emilio Villa-González, ZXZpbGxhQHVnci5lcw==
Disclaimer: All claims expressed in this article are solely those of the authors and do not necessarily represent those of their affiliated organizations, or those of the publisher, the editors and the reviewers. Any product that may be evaluated in this article or claim that may be made by its manufacturer is not guaranteed or endorsed by the publisher.
Research integrity at Frontiers
Learn more about the work of our research integrity team to safeguard the quality of each article we publish.