- 1State Key Laboratory of Remote Sensing Science, Aerospace Information Research Institute, Chinese Academy of Sciences, Beijing, China
- 2University of Chinese Academy of Sciences, Beijing, China
- 3Nepal Open University, Lalitpur, Nepal
- 4Nepal Geographical Society, Kathmandu, Nepal
- 5Taylor Foundation for the Arts and Sciences, Atlanta, GA, United States
- 6Tulane University, New Orleans, LA, United States
Background: Despite examining the role of an association between particulate matter and lung cancer in low-income countries, studies on the association between long-term exposure to particulate matter and lung cancer risk are still contradictory. This study investigates the spatiotemporal distribution patterns of lung cancer incidence and potential association with particulate matter (PM2.5) in Bagmati province, Nepal.
Methods: We performed a spatiotemporal study to analyze the LC – PM2.5 association, using LC and annual mean PM2.5 concentration data from 2012 to 2021. The study assessed the global spatial autocorrelation test using global Moran's I, applied hotspot analysis. A bivariate statistical analysis was performed to evaluate the association, we also applied the geographically weighted regression model (GWR) to look for possible relationships.
Results: The annual mean crude incidence rate (CIR) and standardized incidence rate (SIR) were 5.16, and 6.09 respectively. The study reveals an increasing trend with notable municipal-level spatial variations. Bhaktapur municipality exhibits the highest CIR (243.88), followed by Panchkhal and Sunapati. Males consistently exhibit higher rates, particularly in middle-aged and older adult populations. Bhaktapur displayed the highest CIR in males (171.9) but very low in females (72). The spatial analysis identified concentration trends and hotspots developed in the Bhaktapur, Panchkhal, and Sunapati municipalities. The SIR showed fluctuating patterns of continuous rise until 2019, decrease in 2020, and rise again thereafter. Similar fluctuation association patterns were observed with PM2.5, the r-squared value consistently fluctuated during the study period.
Conclusion: In this study, we found an association between PM2.5 exposure and lung cancer incidence. The findings underscore the need for targeted public health interventions, highlighting the role of PM2.5. Future research is suggested to explore the relationship between lung cancer distribution and various environmental risk factors for effective control and prevention. Addressing air pollution could potentially reduce future lung cancer risk.
Introduction
Cancer is a major global health problem and rapidly increasing in the global south (1, 2). According to the World Cancer Report, lung cancer is one of the major causes of death in the world (3). In 2020, ~2.21 million new cases were identified, and 1.8 million people died due to lung cancer. For men, lung cancer is the most common cancer worldwide (4), with the adjusted standardized incidence rate (ASIR) and adjusted standardized mortality rate (ASMR) at 22.4 and 18.0 per 100,000, respectively. The study in Shandong province found that four health endpoints related to PM2.5 in males were higher than in females, and the health consequences of PM2.5 pollution appeared to be more severe in males compared to females (5). With increasing easy access to tobacco, pollution, and industrialization, lung cancer risk is rising in the global south continuously (6). Lung cancer risk distributed heterogeneously, depends on various geographical locations, mainly because of several risk factors, such as pollution concentration, people's lifestyle, smoking prevalence, topographic variation, and dietary habits (7).
According to the International Agency for Research on Cancer (IARC), 2,431 new lung cancer cases with 9.3 adjusted standardized incidence rates were reported in Nepal. A study in Nepal reported the ASIR and ASMR were 80.9 and 54.8 per 100,000, respectively, which is very high; it is visible that Nepalese people are vulnerable to cancer (8).
Cancer mortality rates are notably higher among men in Nepal's urban areas, with peak lung cancer incidence observed in the 70–74 age group (62). Lung cancer leads as the most common cancer in Nepal, with the crude incidence rate (CIR) rising significantly between 2003 and 2012, from 2.25 to 4.45 per 100,000 in males and from 1.52 to 2.85 in females (9). This type of cancer accounts for the highest number of cases in men and the third highest in women, representing the primary cancer-related fatality risk for males. In 2012, the incidence rate was recorded at 5.74 in males and 3.89 in females (9). The Nepal Health Research Council (NHRC) reported a lung cancer mortality rate of 41.6 per 100,000, with evidence indicating a gradual increase in recent years (10). The ASIR in Kathmandu is 18.1 for males and 10.4 for females, contrasting with lower rates in rural areas such as Rukum (13.2 in males and 5.2 in females) (62). Additionally, studies indicate a higher burden of lung cancer among males compared to females in Nepal (11, 52).
Lung cancer risk is known to be linked to pollution levels and population density. Studies such as Liao et al. (53) have demonstrated an association between PM2.5 concentration and lung cancer mortality, with multiple spatiotemporal studies identifying fine particulate matter (PM2.5) as a significant effect on human activities (12) and a contributor to lung cancer incidence (13, 14, 54). PM2.5 ranks among the top public health risk factors globally, linked to 4.58 million deaths annually (55). Nepal's air quality was ranked 162 out of 200 countries by the Environmental Performance Index (56), with Kathmandu among South Asia's most polluted cities. PM2.5 is considered a major pollutant, which has been associated with harming public health, and premature deaths contributed by LC (15). WHO reports indicate an average PM2.5 level of 140 μg/m3 in Nepal—ten times above the recommended limit (3). In Bagmati Province, high population density in urban areas increases outdoor activity exposure, elevating lung cancer risk among residents.
Studies suggest a unique relationship between environmental factors, geography, and disease patterns, highlighting the importance of spatially focused approaches for disease control and prevention (50). Geospatial technology is instrumental in epidemiological research, offering crucial insights into disease distribution and contributing to targeted intervention strategies (51). This study, therefore, aims to fill a significant research gap by examining the relationship between PM2.5 exposure and lung cancer incidence in Nepal's Bagmati Province, an area where spatial epidemiology studies are scarce, especially regarding lung cancer risk in the Himalayan context. We hypothesize that higher PM2.5 exposure correlates with elevated lung cancer incidence, and we explore this association across the province's lowest administrative levels. By identifying correlations and hotspot areas with significantly higher lung cancer trends, this research provides a foundation for more precise public health interventions and further studies into the environmental determinants of lung cancer in Bagmati Province.
Methods
Study area
Bagmati province, is most populated province, is situated in the central part of Nepal with latitudes 26°55′-28°23′N and longitudes 83°55′-86°34′E. It has an area of 20,300 sq. km, which is 13.79% of the country's total area. Among the total land, 27.29% is covered by forest. Bagmati province is bordered by Madhesh province and India in the south, China in the north, Gandaki province in the west, and Koshi province in the east (Figure 1). According to the administrative division, it has 13 districts, and 119 local levelcomposed of three metropolitan cities, one sub-metropolitan city, 41 municipalities, and 74 rural municipalities with 1,121 wards. Bagmati province has a 6,116,866 population, 3,068,182 females, and 3,048,684 males (16). The Bagmati province has the second-highest population in Nepal. Kathmandu is the capital city of Nepal, which is highly populated, with a population of about 2,041,578 in the district itself—believed to be ~3.1 million in the entire Kathmandu valley.
Bagmati Province, encompassing Nepal's capital (Kathmandu Valley), is the country's most populous and developed region, with high urban density and considerable migration flows that contribute significantly to elevated air pollution levels (57, 58). This combination of dense urban congestion and limited resources to support healthy living conditions has been linked to an increased risk of lung cancer, particularly in major cities (59). Non-communicable diseases (NCDs) are also on the rise, posing a growing public health concern (60). As Nepal continues to develop, the rising incidence of lung cancer has emerged as a critical challenge, significantly impacting public health in urbanized areas.
Lung cancer data
Lung cancer data between 2012 and 2021 were collected from the Bhaktapur Cancer Hospital (BCH), one of the major government cancer hospitals in Bhaktapur city. The lung cancer data contains attributes including age, sex, address, and diagnosis date. The patient's permanent address is linked with the lung cancer data with a municipal map of Nepal in an ArcGIS environment. Out of 2,279 lung cancer cases, 95 were dropped due to missing information about the municipality, while the remaining 2,184 were successfully joined for further analysis. The municipality's age-specific populations were extracted from the NSO reports published by the Nepal government. The study used the municipality boundary map from the Department of Survey, Government of Nepal.
PM2.5 data
The study utilized annual mean PM2.5 concentration data from the Socioeconomic Data and Applications Center (SEDAC) dataset spanning 2012 to 2019. This study used particulate matter concentration data from 2012 to 2019. This dataset has included PM2.5 and its spatial distribution map with 0.1° × 0.1° resolutions (https://sedac.ciesin.columbia.edu/citations-db) (17). The dataset extracted PM2.5 data from the Global Annual PM2.5 Grids, MODIS, MISR and SeaWiFS Aerosol Optical Depth (AOD) with GWR dataset released by NASA (https://sedac.ciesin.columbia.edu/data/set/sdei-global-annual-gwr-pm2 − 5-modis-misr-seawifs-aod-v4-gl-03/data-download) annual-gwr-pm2.5-modis-misr-seawifs-aod) with a resolution of ~1 × 1 km. This dataset is almost similar to ground-monitored PM2.5 data with R2 = 0.81 and has been used in several studies (18, 19). Then, we calculated the annual 2012–2021 PM2.5 mean concentration for each municipality at the centroids of these polygons using a linear regression model. The same method has been applied in a previous study by van Donkelaar et al. (20). Finally, the study linked lung cancer data and PM2.5 from 2012 to 2021 for each of the 119 municipalities of Bagmati province.
Statistical analysis
For spatial statistical analysis, crude incidence rate (CIR) and age-adjusted standardized incidence rate (SIR) were calculated using the annual incidence rate. We used the national population of 2011 to calculate. Both rates were expressed per 100,000. In addition, to the Bagmati province, we also calculated CIR and SIR for Kathmandu Valley. The annual crude incidence rate was calculated by using the following equation:
Where, C = Total lung cancer cases
P = Total population
The age-adjusted incidence rate (SIR) was calculated e with a standard age structure. We used 18 specific age groups (i.e., 0–4 years, 5–9 years, . . . 85 years or older) to calculate it. The study used the Poisson model to examine the statistical significance, and statistical significance was defined as two-tailed p-values < 0.05 for this study (21), it has been used to compare and understand the temporal distribution (22). The standardization rate is in practice and suitable for comparing incidence rates in different years. First, we calculated the age-specific incidence rate then we standardized it with the national standard population. The annual age-standardized incidence rate was calculated by using the following equation:
Where, ci = Number of new lung cancer cases in the ith age group
Si = Standard population of the ith age group
yi = Risk population of the ith age group
S = Total standard population
n = the number of age groups (n = 18)
Spatial analysis
Crude incidence rate
Spatial analyses were conducted using GeoDa 1.6.7 and ArcGIS 10.5 (Esri Inc., California, USA) software (23). In GeoDa, we created a spatial weight file with a neighbored structure based on the K-nearest neighborhood criteria (five municipalities in this research), which was then imported to create spatially smoothed distribution maps. We computed the municipal-level CIR. The study employed a GIS mapping environment to visualize the lung cancer incidence rate over a decade. The study calculated the mean annual incidence rate using the GeoDa software by dividing the total number of lung cancer cases by the corresponding municipal population. The crude incidence municipal-level annual rates, CIRLC for the ith municipal in the year t was estimated as:
where XLCit represents the reported LC case counts at the municipality i (i = 1, 2, …, n = 2,184) for the year t (t = 2012, 2013, …, 2021), and Pit represents the population in municipality i for the year t.
Excess risk and rate
We also calculated excess risk to determine the lung cancer risk in Bagmati province. The excess risk can be determined by dividing the observed incidence in each municipality by the average incidence in all endemic locations. We calculated the excess rate for each municipality dividing total cancer cases by the corresponding municipal population each year. In the excess risk, value one is commonly used as a cut-off value, with less than one indicating lower incidence than predicted and more than one indicating higher incidence than expected. The municipal-level annual excess rate (ER)LC for the ith municipal in the year t was estimated as:
Where: LC(X) is the municipal level cases reported from the hospital (i) for the year (t), and (Y) is the population of the municipality (i) for the year (t) from 2012 to 2021, and census 2021 population is used for this calculation.
The study calculated a 10-year annual raw and excess risk map, which was subject to spatial autocorrelation analysis. Consequently, this map may not be accurately present the real distribution of lung cancer in the study area (24). To address this, we processed 10-year averaged incidence rates to generate a spatially smoothed lung cancer distribution map by correcting for spatial autocorrelation. We utilized the spatial empirical bayes method available in GeoDa for this purpose. Initially, we created a spatial weight file in GeoDa incorporating neighboring structures based on the K-nearest neighborhood criterion (five municipalities in this instance). This file was subsequently employed to produce the spatially smoothed distribution maps.
The study assessed the global spatial autocorrelation test using global Moran's I (25) and then applied hotspot analysis, spatially-weighted sum, bivariate statistical analysis, and geographically-weighted regression (GWR) model (22). As shown in the methodological framework (Figure 2), we first calculated the hotspot analysis based on municipal-level incidence rate to detect the geographical patterns of lung cancer incidence. After the hotspot analysis, we calculated the spatial weighted sum, applied to population-level risks of different municipalities by overlapping the population density (22). The following two stages attempted to explore the spatiotemporal correlations between PM2.5 distributions and lung cancer risk. A bivariate statistical analysis was performed to evaluate the association, while the geographically weighted regression model (GWR) was applied to look deeply into the possible relationships between different municipalities. A GWR model was used to examine the spatially varying association between lung cancer and PM2.5; it is shown with the standard deviations of the coefficient of determination (R2) at different municipalities. The study examined the GWR after applying the t-test to the linear regression.
The study used the Getis–Ord G* statistic, a scoring system derived from a significance test, to calculate whether features with high or low values tended to cluster within the study area. This software detects statistically relevant hotspots surrounded by other features with high values for each component in a dataset (26). The Z-score demonstrates the spatial clustering of features with high or low values. The formula is given below:
Where xj is the value for j, wij is the value of spatial value between I and j. n is equal to the total number of features and:
The value is the Z-score, so no need for additional calculation.
Then, we used the spatially-weighted sum tools to examine the health risks from particulate matter (14, 22), previous research adopted the classical approach to present the interactive outcomes of more factors by adding the value of the elements from one layer to another layer with area attributes (27). The study had calculated the lung cancer risk based on population density and we overlay annual PM2.5 with the population density map on the ArcGIS environment. The study used the population density and PM2.5 raster data to examine and identify the risk using overlap analysis.
The spatially weighted sum was calculated by overlapping the population densities and distributions of PM2.5 to calculate the health risks. This study used the Jenks natural breaks default in ArcGIS in this study. Natural break classes are based on natural groupings inherent in the data. Class breaks are identified as the best group with similar values and maximize class differences. The output is categorized into five levels and spatially weighted to show the population-level health risks at different municipalities.
Following hotspot analysis, we computed bivariate statistical analysis to examine the spatial interrelation between PM2.5 and lung cancer incidence (28). This method is accessible for exploring relationships between different types of attribute data. We transferred the polygon data into the raster data before starting the spatial analysis. The polygon data were transferred at a 30 m resolution to raster data for spatial calculation and analysis in ArcGIS.
Finally, we employed the GWR model to analyze the spatially heterogeneous association of lung cancer with PM2.5. The GWR model fits the regression of each location and reports mapable regression parameters including the coefficient of determination i.e., R-squared assuming the relationship may vary in different locations (13). The following is the equation for a typical GWR of the ordinary least-square regression (OLS) model:
Where y is the lung cancer incidence rate of each municipality while x represents the environmental exposure i.e., PM2.5 in our case. The β0i indicates the location of the study unit.
Based on the geographical distribution of lung cancer incidences and health risks associated with PM2.5, our research investigated the spatial correlations between these health risks. Initially, a bivariate statistical analysis examined the overall spatial correlation between raster layers representing the distribution of PM2.5 and lung cancer incidence.
Results
Two thousand one hundred and eighty-four lung cancer cases were reported to the Bhaktapur Cancer Hospital during the study period. Among these, 1,315 cases were males, 867 females, and, 1 third gender. The annual mean CIR was 5.16. During the study period, the minimum CIR (3.02) was reported in 2012 while the maximum CIR (6.85) was reported in 2019 (Table 1). In the same period, the mean CIR of Kathmandu Valley was 5.53, the minimum was reported in 2012 and the maximum was reported in 2021. The minimum and maximum CIR were 3.86 and 6.84, respectively. Likewise, the age-standardized rates were calculated using the Nepal Census 2011. The annual mean SIR in Bagmati Province was 6.09. The minimum SIR, reported in 2012, was 3.58, while the maximum SIR, reported in 2019, was 8.09. The annual mean SIR in Kathmandu Valley was 6.56. The lowest SIR, 4.96, was observed in 2012, while the highest SIR, 7.81, was observed in 2021.
The annual analysis demonstrated several notable trends and fluctuations during the study periods (Figure 3). The number of lung cancer cases increased steadily from 74 in 2012 to 131 in 2021, with a notable increase of 77.03% in Kathmandu Valley. The crude rate, rises from 3.86 in 2012 to 6.84 in 2021, with an increment of 77.20%. In Bagmati province lung cancer cases increased from 119 in 2012 to 271 in 2021, showing a significant increase of 127.73%. The study found the crude rate rose from 3.02 in 2012 to 6.09 in 2021, showing an increase of 101.66% during the study period.
The results reveal marked spatial variation and heterogeneity in the distribution of CIR. Bhaktapur municipality exhibits the highest CIR (243.88), followed by Panchkhal and Sunapati with 146.39 and 129.62, respectively (Figure 4C). Bhaktapur municipality was the only municipality in the Kathmandu Valley with higher health risks. Conversely, municipalities such as Raksirang and Marin reported relatively low CIR at 3.85 and 3.47, respectively. Lung cancer cases are reported in higher numbers from Kathmandu, Lalitpur, and Bhaktapur, but due to the high density of the population, they had a low crude incidence rate. This detailed breakdown underscores the heterogeneity in lung cancer burden across municipalities, guiding targeted public health interventions and resource allocation based on specific incidence patterns. Specifically, within the province, the municipalities of Bhaktapur (one), Lalitpur (one), Ramechhap (three), Kabhrepalanchwok (six), Sindhupalchok (two), Nuwakot (one), and Makawanpur (one) exhibit a notable presence lung cancer. This distribution of CIR underscores the need for further investigation and targeted public health interventions in these identified areas to mitigate potential health challenges and improve overall community wellbeing.
The results further show spatial variations of CIR in terms of sex (Figures 4A, B). For instance, Bhaktapur displayed the highest CIR in males (171.9) but very low in females (72), The same pattern was found in another Panchkhal municipality of Kabhrepalanchwok (90.1) and females (56.3). Notably, Indrasarowar rural municipality exhibited a significantly higher CIR on females (51.7) compared to males (29.6). Further, the Meghang rural municipality of Nuwakot showed a higher CIR for females (47.4) compared to males (7.9).
The study found twenty-one municipalities with an excess risk higher than two, suggesting elevated risk relative to the municipality average (Figure 5). Two municipality Bhaktapur and Panchkhal had the highest excess risk among the other municipality, they had 6.78 and 4.78, respectively. The high level risk was distributed close to the Kabhrepalanchok, Sindhupalchwok, and Ramechhap districts. Most of the municipalities (73) has a moderate health risks, those municipalities have 0.5–2 excess risk, particularly concerning lung cancer, are observed across various municipalities in the Bagmati province of Nepal. The distribution of health risk of the study area underscores the detail investigation and started to lung cancer interventions to mitigate potential lung cancer risk.
The global spatial autocorrelation test using Moran's I showed positive spatial autocorrelation with Moran's I-value of 0.366, a Z-score of 8.25, and a p-value of 0.000 (Figure 6). This finding suggests a non-random distribution of lung cancer incidence rates within the study area. The positive Moran's I value indicates positive spatial autocorrelation, implying that neighboring municipalities tend to have similar lung cancer incidence rates.
The results of Getis-Ord Gi* spatial statistic were presented to identify concentration trends in lung cancer incidence in Bagmati province (Figure 7). The focal points were primarily clustered within the complex landscape in the vicinity of the Kabhrepalanchok, Bhaktapur, Sindhupalchok, and Ramechhap districts. The analysis revealed spatiotemporal variations in hotspot development over the study period. The coldspot was mainly observed around Chitawan, Makawanpur, and Rasuwa throughout the study periods. Notably, no significant geographical concentrations of either higher hotspots or coldspots were observed across Kathmandu and Lalitpur metropolitan cities after 2019. However, consistent coldspots were identified in Bharatpur metropolitan city each year. All identified coldspots exhibited 99 and 95% confidence levels.
The hot and cold spots experienced a minor contraction in 2 years (2012 and 2019) but expanded in the remaining 8 years. The coldspot was surrounded by secondary-level all year except in 2012. The hotspot exhibited elevated occurrences in 2013, 2016, and 2018 across all municipalities in Kabhrepalanchok and most municipalities in Sindhupalchwok and Ramechhap. Notably, Barhabishe municipality demonstrated a pronounced concentration pattern and a rise in lung cancer cases despite being categorized as a region with a lower health risk (very low) throughout the study period.
The results of the population-level health risk assessment show a very high risk in the three municipalities' different districts of Kathmandu Valley. The risk level coincided with the geographical concentration of both populations and PM2.5 in these areas as illustrated in Figure 8. Medium-level health risks were identified in municipalities such as Tokha, Kageshwori Manohara, Kirtipur, Mahalaxmi, and Bharatpur. Conversely, municipalities in Rasuwa, Dolakha, and Ramexap districts exhibited the lowest health risks. The Shailung, Doramba, And Ruby Valley of Bagmati province experienced the lowest health risks (very low) from PM2.5. The observed spatiotemporal variations in health risk within Bagmati province, particularly in Kathmandu Valley, indicated a concerning increasing trend.
The correlation matrix (Table 2) illustrates the spatial correlation analysis conducted from 2012 to 2021, which showed strong positive correlations among the annual incidence rates, indicating consistent spatial patterns in lung cancer prevalence over the study period. Specifically, correlations between successive years demonstrate high levels of consistency, with coefficients ranging from 0.712 to 0.925. This suggests a persistent spatial autocorrelation in lung cancer incidence rates, indicating that areas with high rates tend to remain clustered together over time. For instance, correlations were notably high in 2014 (0.913) and 2016 (0.925). The correlation between PM2.5 and lung cancer is relatively weak, with coefficients ranging from 0.125 in 2016 to 0.195 in 2018. This suggests a limited direct association between fine particulate matter pollution and lung cancer incidence. However, the spatial correlation between lung cancer incidence and PM2.5 concentrations in different municipalities was spatially distributed. Overall, the correlation pattern highlights the importance of considering both temporal trends and spatial relationships in understanding the dynamics of lung cancer incidence and its potential environmental determinants.
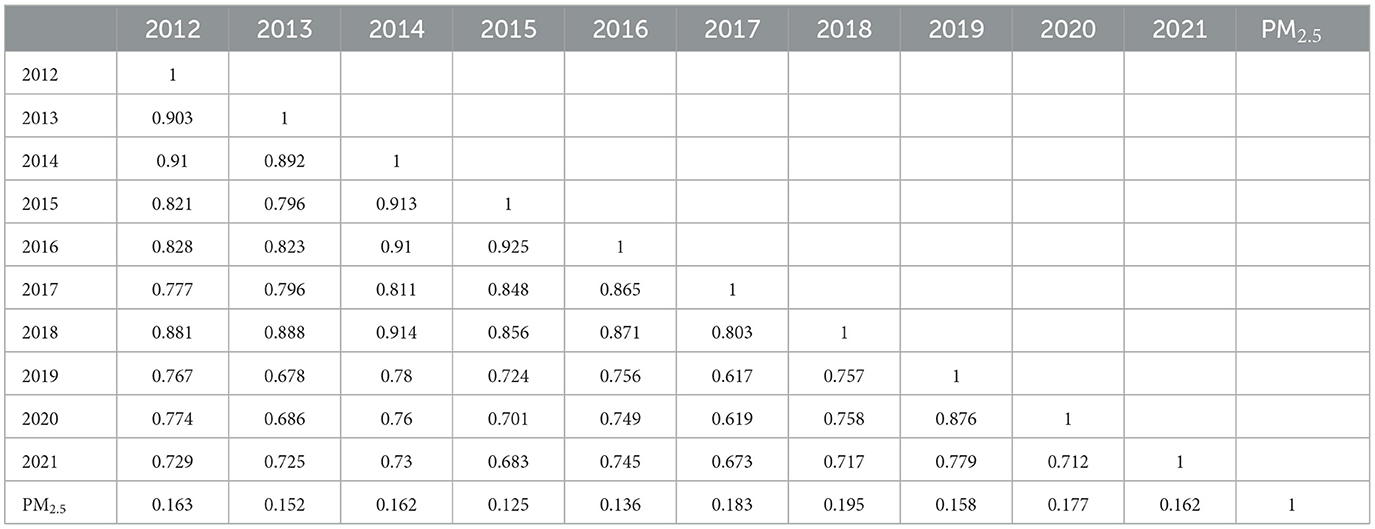
Table 2. Correlation matrix of the spatial correlation analysis among annual lung cancer incidence rates between years 2012–2021.
The geographically weighted regression GWR model is the advancement of the classical linear regression model, which means the ArcGIS adds several spatial analysis methods on the system, it adds the geographic coordinates into the classical linear regression model (29). In the study, before performing the GWR, we applied ordinary linear regression, to confirm the rationality of selecting the index from the extended GWR model. We applied the ordinary least square (OLS) method to validate the results. The R2 and adjusted R2 indicate the model fitting is better. The estimated coefficients of PM2.5 indicated lung cancer incidence and PM2.5 are significantly and positively related (B = 0.0387, P < 0.05). Therefore, from the OLS, it could be validated that the PM2.5 variables are reasonable, and we could continue to perform GWR. The GWR model best fits where values range from 0.5 to −0.5 values. Beyond this, the local GWR models were poorly fitted. Overall, the GWR analysis provides valuable insights into the localized relationships between explanatory variables and lung cancer incidence rates, contributing to our understanding of spatially varying risk factors and informing targeted intervention strategies.
The GWR analysis between cancer incidence and PM2.5 showed a fluctuating pattern of R-squared over the years (Figure 9). The R-squared values indicate the strength of the relationship between explanatory variables (PM2.5) and lung cancer incidence rates. The highest r squared was observed in 0.594 in 2018 indicating a period when PM2.5 exposure had a strong association with lung cancer risk. The result explains nearly 60% of the variance, while the GWR shows the lowest association between them in 2015. Conversely, the lowest R2 value of 0.204 in 2015 suggests a substantial decrease in the predictive power of PM2.5. The GWR analysis of lung cancer risk associated with PM2.5 in the study area during the study periods presents significant temporal variability in the strength of this association. The R2 values, representing the proportion of variance in lung cancer risk explained by PM2.5 levels, exhibit a moderate to strong relationship overall, with notable peaks and troughs. Despite these fluctuations, the generally high R2 values in recent years, particularly from 2018 to 2021, underscore the persistent and significant influence of PM2.5 on lung cancer risk in the region.
Discussion
This spatial epidemiological study provided updated lung cancer incidence rates (both CIR and SIR) in Bagmati province during the last 10 years based on Bhaktapur Cancer Hospital data. The study calculated and visualized the spatial and temporal distributions of lung cancer incidence and population-level health risks from PM2.5 across the Bagmati province. In addition, the study used the space-time geospatial approach to classify municipal-level health risks among the people by overlaying the population density and PM2.5 concentrations. We visualized the result in different maps through GeoDa and ArcGIS to show the association, spatial distributions, and temporal variation of lung cancer in the study area.
Our comprehensive analysis spanning a decade presents a detailed examination of the municipal-level spatial distribution patterns of lung cancer in Bagmati province. As illustrated in Table 1, lung cancer incidence rates reveal notable variations across different years. The standardized incidence rate peaked in 2019, indicating a heightened disease burden. Increased burden of cancer has also been reported in other recent studies. For example, National Health Research Council (NHRC), reported an incidence rate of 41.6 per 100,000 population (10). The age-standardized incidence rate for lung cancer in Nepal is reported at 12.3 per 100,000 population, surpassing incidence rates observed in neighboring countries like India yet lagging behind the higher rates documented in China (8). GLOBOCAN report (2022) highlights the risk of lung cancer, noting 2,431 new cases with an SIR of 9.3. Furthermore, research by Jha et al. (30) delineates a wide-ranging incidence rate for lung cancer in Nepal, spanning from 2.6 to 23.7 per 100,000 across diverse geographic regions of Nepal.
This study presents a detailed examination of lung cancer incidence in both genders. In males, there was an overall increasing trend in cancer incidence, but a notable reversal occurred after 2012, indicating a potential shift in the epidemiological landscape. In contrast, females exhibited a fluctuating trend from 2014 to 2016, showcasing periodic variations in cancer incidence. This observation contrasts with previous studies by Pun et al. (11) and Shrestha et al. (31), which also reported escalating cancer trends in Nepal. Recent studies in Nepal also reported the increasing trend of cancer in both males and females from 2003 to 2012. The study by Poudel et al. (9) showed that lung cancer was the major cancer throughout the last decade in males. A study in Nepal reported the same result; according to the study, lung cancer is increasing in urban areas of Nepal (11, 32). It was because of high pollution concentration in urban areas (33), household air pollution (34), unhealthy food, sophisticated lifestyle, tobacco consumption (8, 35), and insufficient medical health education (36). Furthermore, during the study period, the crude and standardized incidence rates of lung cancer in the study area increased from 2012 to 2016 but decreased in 2017 and 2020. Among all the municipalities of Bagmati province, Panchkhal, Bhumlu, Chautara-Samachokgadhi, Sunapati, and Ramechhap have an increased risk of lung cancer.
Nepal had the highest age-specific lung cancer incidence rate in males during 2013–2015, among other cancers (31). These results also show that the risk factor of lung cancer is still not decreasing and has huge problematic risk characteristics that have not changed. The incidence rate in Bhaktapur and Panchkhal municipalities increased nearly three times from 2012 to 2021. The high risk in these municipalities might be due to screening and a high air pollution concentration. Several possible reasons may account for this phenomenon, including (1) accumulation of lung cancer from PM2.5 because of the geospatial attributes (37) and (2) increased urban areas and pesticide trade within a study area as part of the development activities and increase of agricultural production in Bagmati province (38, 39). A noteworthy observation is the consistent elevation of the incidence rate in males in urban areas. During this study period, the incidence rate in females in rural municipalities surpassed that of males. The lung cancer incidence exhibited analogous patterns in both genders within Bagmati province. However, a substantial incongruity emerged in the urban areas, where the LC was approximately twice that of rural areas, highlighting significant rural-urban variations.
The spatial autocorrelation analysis was performed PM2.5 and Spatial Empirical Bayes smoothed incidence rate using Moran's I index. This metric ranges from −1 to +1, where −1 indicates perfect negative spatial autocorrelation, +1 indicates perfect positive spatial autocorrelation, and 0 denotes a spatially random distribution (40). Our study revealed that Moran's I values for lung cancer risk were consistently higher than 0 in the study area, indicating the presence of spatial clustering in Bagmati province (Figure 7). This suggests a significant clustering of lung cancer risk in this province, highlighting the importance of further investigation into potential environmental or demographic factors contributing to this clustering phenomenon.
The lung cancer distribution has been attributed to population density, diverse topography, and pollution to lung cancer (23, 41, 42), most likely linked to pollution exposure (PM2.5) and the prevalence of risk in Bagmati province. PM2.5 is heterogeneously distributed; it has a high concentration in the Kathmandu Valley and Chure Siwalik region of Bagmati province. The study presented the same population distribution pattern (Figure 1). Kathmandu Valley has the highest population density. Thus, analyzing the municipal-level distribution of lung cancer risk provides a comprehensive understanding and helps to focus on the prevention strategy.
The hotspot experienced a continuous expansion from 2012 to 2018 but slightly shrinkage in 2019. Zhang and Tripathi (26) found that two regions (Chonburi and Chanthaburi) of Eastern Thailand were the major hot spots for lung cancer risk, situated near industrial areas. Our study identified the heterogeneous hotspots within Bagmati province; however, some municipalities like Bhaktapur and Panchkhal were identified as major HH clusters during the study periods. These areas are attributed to high concentrations of PM2.5 and pollution accumulation (37). This study highlights that it is important for effectively controlling lung cancer risk. Policymakers and healthcare authorities can strategically allocate resources and implement targeted intervention strategies by pinpointing these areas with heightened risk. This approach enables focused efforts to mitigate the impact of lung cancer in high-risk regions, thereby optimizing resource utilization and potentially improving health outcomes for affected populations (22, 43). Overall, identifying these hotspots is a valuable tool in guiding proactive measures aimed at reducing the burden of lung cancer within Bagmati province and enhancing the effectiveness of public health initiatives in combating this disease.
Incorporating municipal population density and PM2.5 into our analysis generated a comprehensive risk assessment. The population-level health risks attributed to PM2.5 are high for those who live in core urban areas with high concentrations of pollution, showing very high health risks in core city areas and high health risks in peripheral areas of Kathmandu Valley and Chitawan. The Kabhrepalanchwok, Nuwakot, and Ramechhap municipalities witnessed a significantly moderate concentration pattern and increased lung cancer risk. However, it was defined as a lower health risk area. This approach enhances the accuracy of identifying risk zones and provides valuable insights for targeted mitigation efforts. Our weighted sum overlay analysis offers a practical tool for prioritizing resource allocation and intervention strategies in high-risk areas by leveraging the strong evidence linking population density to lung cancer prevalence. Ultimately, it empowers policymakers and healthcare professionals with the information needed to address the burden of lung cancer within Bagmati province proactively.
Previous research has indicated that lung cancer and cardiovascular disease are associated with environmental factors, geographical location, and topography (23, 44). The study also presented spatiotemporal variations of lung cancer risk in Bagmati province associated with environmental factors (PM2.5) at the municipal level. The overall temporal distribution of lung cancer incidence rate is significant, but spatial correlations between lung cancer and PM2.5 distributions were not strongly significant. However, the study found differences at the municipal level compared to the Bagmati province. Furthermore, our study highlights the spatial heterogeneity in lung cancer risk at the municipal level across the study periods. Geographically weighted regression models can potentially enhance the explanatory power of risk factors to demonstrate spatial variations in lung cancer risk (23). This model can present the association between environmental variables and lung cancer risk, which will be effective in running the lung cancer intervention program in Bagmati province.
The study found a fluctuating temporal correlation in the study area between 2012 and 2021. The study investigated the spatial relationship between the distribution of PM2.5 and the incidence rate of lung cancer across the municipal level of Bagmati province using the GWR model. The findings indicate a noteworthy spatial association between PM2.5 and lung cancer, particularly in geographically concentrated areas with very high-risk municipalities. The risks of lung cancer incidence associated with fine particulate matter. Results show that PM2.5 emissions and concentrations have similar distribution patterns: high values existed in the urban areas with high population density and incidence. In contrast, the village area had a low incidence rate. These results underscore the potential impact of pollution activities on public health outcomes, emphasizing the need for targeted interventions and further investigation into mitigating factors in affected municipalities.
This research significantly enhances our understanding and contribution to the field of lung cancer and spatial epidemiology in Nepal by adding the spatiotemporal approach to the correlation with environmental and geographical perspectives. Adopting a health geography perspective to explore a smaller area's fundamental modifiable areal unit problem (MAUP) was much more effective in analyzing lung cancer (22, 45). This study strategically employed MAUP to study the municipality as the unit of analysis, providing valuable insights into the intricacies of lung cancer in Bagmati province. It was also an advantage in conducting environmental health-related research. Although socioeconomic status and healthcare accessibility may influence lung cancer, it is noteworthy that these elements were relatively evenly distributed across the study area.
However, this study was completed with several limitations. We acknowledge that a significant limitation of our study is the reliance on lung cancer incidence data from a single institution, Bhaktapur Cancer Hospital (BCH), which represents only a portion of the overall patient population in Bagmati Province and contributes ~20% to the Population-Based Cancer Registry (PBCR) system in Nepal (62). Bagmati Province has multiple institutions providing cancer care, and while including data from all hospitals would ideally yield a more comprehensive sample, access to these datasets was beyond our scope due to resource and logistical constraints.
This single-center approach may have several potential biases. Albeit, it is the leading cancer hospital in Nepal. Selection bias may be minimal due to the patient population at BCH has unique characteristics, such as distinct demographic or socioeconomic factors, that differ from those receiving treatment elsewhere. Referral bias could also impact generalizability, as BCH may treat more advanced or specific cases of lung cancer, characteristic of tertiary-care centers, which can influence the representation of prognostic factors. Additionally, underreporting or over reporting is possible since cases treated at other facilities are not captured, potentially skewing the observed incidence patterns. Lastly, temporal bias may affect data consistency over the study period due to evolving diagnostic criteria, treatment practices, and reporting standards.
Moreover, this study is subject to the modifiable areal unit problem (MAUP), where spatial analysis results can vary based on how geographic units, like municipalities, are defined and their sizes (61). The way municipalities are divided may influence the spatial patterns observed, potentially affecting the strength of associations between PM2.5 exposure and lung cancer risk. Moreover, we do not incorporate topographical and meteorological components to understand the dynamics of lung cancer, representing another limitation in this study. Although topographical phenomena are key influential factors in epidemiology, they contribute highly to lung cancer (46).
Other factors contributed to significantly higher lung cancer incidence in men than women in Bagmati Province, Nepal. Lung cancer risk among men is elevated due to (1). higher smoking rates (3), (2). occupational exposures, particularly in agriculture, construction, and brick manufacturing, significantly increase the risk of lung cancer due to exposure to hazardous chemicals, respirable crystalline silica, and poor working conditions, highlighting the urgent need for improved health and safety standards. Prajapati et al. (47) increased alcohol use, which amplifies tobacco's carcinogenic effects (48). While women face higher indoor air pollution exposure from cooking with solid fuels, traditional household roles and limited healthcare access may delay diagnosis, affecting lung cancer incidence reporting (49). It is important to note that the study results may be biased, potentially underrepresenting or over representing the actual relationships between PM2.5 exposure and lung cancer risk without controlling for these confounding factors. Although this study focuses solely on the association with PM2.5, these additional factors are important considerations in understanding gender disparities in lung cancer risk for future work.
Despite these limitations, our analysis still provides valuable insights into lung cancer trends and spatial associations with PM2.5 exposures within Bagmati Province. Using geographically weighted regression (GWR) and spatial autocorrelation analysis, we identified notable hotspots and trends that align with known population and environmental patterns. These findings, while limited to a subset of patients, offer a preliminary understanding that can guide future studies with expanded regional data access, potentially enhancing the generalizability of conclusions.
Conclusions
This study analyzed municipal-level lung cancer incidence in Bagmati province and explored the potential association with PM2.5. The result demonstrated a significant difference in the risk of lung cancer over the age and gender where male and older adult populations were more likely to get lung cancer compared with female and young age people. In terms of spatiotemporal distribution, marked spatial variations and heterogeneity were observed. The statistically significant hotspots were detected over the years. The heterogeneous association between lung cancer incidence and PM2.5 was observed and remained changing over the years. The results further showed the risk of lung cancer is increasing over the years. The findings revealed a concentrated pattern of environmental risks and health outcomes around the capital city, with notable spatiotemporal variations.
The government needs substantial information about the spatiotemporal distribution of LC to run an effective strategy for LC prevention. The spatiotemporal studies must be associated with several characteristics of environmental factors like PM2.5. In general, the results of this study are of great significance to public health issues in Nepal. The temporal and spatial analysis of lung cancer incidence provides important epidemiological clues for the investigation mechanism of lung cancer. It provides a scientific basis for the rational allocation of health resources. Based on these findings, it is important for mass early screening among people in high-risk areas of the study areas. Spatial information on diseases, as well as lung cancer, in Nepal is limited. This study can be the pathway of new research in Nepal under the geo-health theme.
Data availability statement
The datasets presented in this study can be found in online repositories. The names of the repository/repositories and accession number(s) can be found in the article/supplementary material.
Ethics statement
Ethical approval was not required for the study involving humans in accordance with the local legislation and institutional requirements. Written informed consent to participate in this study was not required from the participants or the participants' legal guardians/next of kin in accordance with the national legislation and the institutional requirements. The manuscript presents research on animals that do not require ethical approval for their study.
Author contributions
BN: Conceptualization, Visualization, Writing – original draft. BA: Methodology, Writing – review & editing. CC: Supervision, Writing – review & editing. MX: Formal analysis, Writing – review & editing. PT: Formal analysis, Writing – review & editing. SW: Formal analysis, Writing – review & editing. YY: Data curation, Writing – review & editing.
Funding
The author(s) declare financial support was received for the research, authorship, and/or publication of this article. This study was supported by the Open Fund of the State Key Laboratory of Remote Sensing Science (Grant No. OFSLRSS202309).
Acknowledgments
Neupane was supported by the University of Chinese Academy of Sciences and the ANSO Scholarship for Young Talents. We would like to thank you for this opportunity. We would like to extend our sincere gratitude to the Bhaktapur Cancer Hospital that have provided patient data for this research. The authors thank Dr. Basanta Paudel for his constructive comments and detailed advice.
Conflict of interest
The authors declare that the research was conducted in the absence of any commercial or financial relationships that could be construed as a potential conflict of interest.
Publisher's note
All claims expressed in this article are solely those of the authors and do not necessarily represent those of their affiliated organizations, or those of the publisher, the editors and the reviewers. Any product that may be evaluated in this article, or claim that may be made by its manufacturer, is not guaranteed or endorsed by the publisher.
References
1. Jones SB. Cancer in the developing world: a call to action. Br Med J. (1999) 319:505–8. doi: 10.1136/bmj.319.7208.505
2. Thun MJ, DeLancey JO, Center MM, Jemal A, Ward EM. The global burden of cancer: Priorities for prevention. Carcinogenesis. (2009) 31:100–10. doi: 10.1093/carcin/bgp263
3. WHO. World cancer report: cancer research for cancer development. In:C. Wild, E. Weiderpass, and B. Stewart, , editors. Vol. 1. Lyon: International Agency for Cancer Research (IARC) (2020).
4. Cao WR, Shakya P, Karmacharya B, Xu DR, Hao YT, Lai YS. Equity of geographical access to public health facilities in Nepal. BMJ Global Health. (2021) 6:6786. doi: 10.1136/bmjgh-2021-006786
5. Xu X, Zhang W, Shi X, Su Z, Cheng W, Wei Y, et al. China's air quality improvement strategy may already be having a positive effect: evidence based on health risk assessment. Front Public Health. (2023) 11:1250572. doi: 10.3389/fpubh.2023.1250572
6. Thandra KC, Barsouk A, Saginala K, Aluru JS, Barsouk A. Epidemiology of lung cancer. Wspolczesna Onkologia 25:45–52. doi: 10.5114/wo.2021.103829
7. Jemal A, Miller KD, Ma J, Siegel RL, Fedewa SA, Islami F, et al. higher lung cancer incidence in young women than young men in the United States. N Engl J Med. (2018) 378:1999–2009. doi: 10.1056/NEJMoa1715907
8. Shilpakar R, Paudel BD, Sharma R, Silwal SR, Sapkota R, Shrestha P, et al. (2022). Lung cancer in Nepal. J Thorac Oncol. 17:22–9. doi: 10.1016/j.jtho.2021.10.020
9. Poudel KK, Huang Z, Neupane PR, Steel R. Prediction of the cancer incidence in Nepal. Asian Pac J Cancer Prev. (2017) 18:165–8. doi: 10.22034/APJCP.2017.18.1.165
10. Jha AK, Chapagain S, Dhimal M, Subedi R, Kaphle U, Thakur C, et al. Nepal Health Research Council Population Based Cancer Registries. Kathmandu: Nepal Health Research Council (NHRC) (2018).
11. Pun CB, Pradhananga KK, Siwakoti B, Subedi K, Moore MA. Malignant neoplasm burden in Nepal - Data from the seven major cancer service hospitals for 2012. Asian Pac J Cancer Prev. (2016) 16:8659–63. doi: 10.7314/APJCP.2015.16.18.8659
12. Xu X, Zhang H, Chen J, Li Q, Wang X, Wang W, et al. Six sources mainly contributing to the haze episodes and health risk assessment of PM2.5 at Beijing suburb in winter 2016. Ecotoxicol Environ Saf. (2018) 166:146–56. doi: 10.1016/j.ecoenv.2018.09.069
13. Liu C, Chan KH, Lv J, Lam H, Newell K, Meng X, et al. Long-term exposure to ambient fine particulate matter and incidence of major cardiovascular diseases: a prospective study of 0.5 million adults in China. Environ Sci Technol. (2022) 56:13200–13211. doi: 10.1021/acs.est.2c03084
14. Wang CH, Chen N. A geographically weighted regression approach to investigating the spatially varied built-environment effects on community opportunity. J Trans Geogr. (2017) 62:136–47. doi: 10.1016/j.jtrangeo.2017.05.011
15. Xu X, Zhang W, Zhu C, Li J, Wang J, Li P, et al. Health risk and external costs assessment of PM2.5 in Beijing during the “Five-year Clean Air Action Plan.” Atmos Pollut Res. (2021) 12:101089. doi: 10.1016/j.apr.2021.101089
16. NSO. National Population and Housing Census 2021 (National Report) National Statistics Office, Vol. 1 (2021). Available at: www.censusnepal.cbs.gov.np (accessed March 6, 2024).
17. Hammer MS, Van Donkelaar A, Li C, Lyapustin A, Sayer AM, Hsu NC, et al. Global estimates and long-term trends of fine particulate matter concentrations (1998-2018). Environ Sci Technol. (2020) 54:7879–90. doi: 10.1021/acs.est.0c01764
18. Guo Y, Zeng H, Zheng R, Li S, Barnett AG, Zhang S, et al. The association between lung cancer incidence and ambient air pollution in China: a spatiotemporal analysis. Environ Res. (2016) 144:60–5. doi: 10.1016/j.envres.2015.11.004
19. Lavigne É, Bélair MA, Do MT, Stieb DM, Hystad P, van Donkelaar A, et al. Maternal exposure to ambient air pollution and risk of early childhood cancers: a population-based study in Ontario, Canada. Environ Int. (2017) 100:139–47. doi: 10.1016/j.envint.2017.01.004
20. van Donkelaar A, Martin RV, Brauer M, Kahn R, Levy R, Verduzco C, et al. Global estimates of ambient fine particulate matter concentrations from satellite-based aerosol optical depth: development and application. Environ Health Perspect. (2010) 118:847–55. doi: 10.1289/ehp.0901623
21. Flanders WD, Lally CA, Zhu B-P, Henley SJ, Thun MJ. Lung cancer mortality in relation to age, duration of smoking, and daily cigarette consumption: results from Cancer Prevention Study II 1. Cancer Res. (2003) 63.
22. Li J, Guo W, Ran J, Tang R, Lin H, Chen X, et al. Five-year lung cancer mortality risk analysis and topography in Xuan Wei: a spatiotemporal correlation analysis. BMC Public Health. (2019) 19. doi: 10.1186/s12889-019-6490-1
23. Ren H, Cao W, Chen G, Yang J, Liu L, Wan X, et al. Lung cancer mortality and topography: a Xuanwei case study. Int J Environ Res Public Health. (2016) 13:473. doi: 10.3390/ijerph13050473
24. Acharya BK, Cao C, Lakes T, Chen W, Naeem S. Spatiotemporal analysis of dengue fever in Nepal from 2010 to 2014. BMC Public Health. (2016) 16:1–10. doi: 10.1186/s12889-016-3432-z
25. Lei L, Huang A, Cai W, Liang L, Wang Y, Liu F, et al. Spatial and temporal analysis of lung cancer in Shenzhen, 2008–2018. Int J Environ Res Public Health. (2021) 18:1–13. doi: 10.3390/ijerph18010026
26. Zhang H, Tripathi NK. Geospatial hot spot analysis of lung cancer patients correlated to fine particulate matter (PM2.5) and industrial wind in Eastern Thailand. J Clean Prod. (2018) 170:407–24. doi: 10.1016/j.jclepro.2017.09.185
27. Cheng Q, Bonham-Carter G, Wang W, Zhang S, Li W, Qinglin X. A spatially weighted principal component analysis for multi-element geochemical data for mapping locations of felsic intrusions in the Gejiu mineral district of Yunnan, China. Comp Geosci. (2011) 37:662–9. doi: 10.1016/j.cageo.2010.11.001
28. Filzmoser P, Hron K, Reimann C. The bivariate statistical analysis of environmental (compositional) data. Sci Total Environ. (2010) 408:4230–8. doi: 10.1016/j.scitotenv.2010.05.011
29. Cao Q, Rui G, Liang Y. Study on PM2.5 pollution and the mortality due to lung cancer in China based on geographic weighted regression model. BMC Public Health. (2018) 18. doi: 10.1186/s12889-018-5844-4
30. Jha AK, Chapagain S, Dhimal M, Subedi R, Kafle U, Dikshit R. Population Based Cancer Registries at Kathmandu, Bhaktapur, Lalitpur, Siraha, Saptari, Dhanusha, Mohattari, West Rukumand East Rukum Districts, Nepal. (2020). Available at: http://103.69.126.140/bitstream/20.500.14356/740/2/RES00981-NHRC-2020.pdf (accessed May 4, 2024).
31. Shrestha G, Neupane P, Lamichhane N, Acharya BC, Siwakoti B, Subedi KP, et al. Cancer incidence in Nepal: a three-year trend analysis 2013-2015. Asian Pac J Cancer Care. (2020) 5:145–50. doi: 10.31557/apjcc.2020.5.3.145-150
32. Hashibe M, Siwakoti B, Wei M, Thakur BK, Pun CB, Shrestha BM, et al. Socioeconomic status and lung cancer risk in Nepal. Asian Pac J Cancer Prev. (2011) 12:1083–8.
33. Tuladhar A, Manandhar P, Shrestha KL. Assessment of Health Impact of PM2.5 Exposure by Using WRF-Chem Model in Kathmandu Valley, Nepal. Front Sustain Cities. (2021) 3:672428. doi: 10.3389/frsc.2021.672428
34. Raspanti GA, Hashibe M, Siwakoti B, Wei M, Thakur BK, Pun CB, et al. Household air pollution and lung cancer risk among never-smokers in Nepal. Environ Res. (2016) 147:141–5. doi: 10.1016/j.envres.2016.02.008
35. Piya MK. Epidemiological profile of lung cancer in a Nepalese population: a single-institution review. J Clin Oncol. (2019) 37(15_suppl):e13087. doi: 10.1200/JCO.2019.37.15_suppl.e13087
36. Khatiwoda SR, Dhungana RR, Sapkota VP, Singh S. Estimating the direct cost of cancer in Nepal: a cross-sectional study in a tertiary cancer hospital. Front Public Health. (2019) 7:160. doi: 10.3389/fpubh.2019.00160
37. Saud B, Paudel G. The threat of ambient air pollution in Kathmandu, Nepal. J Environ Public Health. (2018) 2018:1504591. doi: 10.1155/2018/1504591
38. Gc A. Trend of Pesticides Uses in Nepal. (2018). Available at: https://www.researchgate.net/publication/329153703 (accessed May 2, 2024).
39. Kandel P, Kharel K, Njoroge A, Smith BW, Díaz-Valderrama JR, Timilsina RH, et al. On-farm grain storage and challenges in Bagmati Province, Nepal. Sustainability. (2021) 13:7959. doi: 10.3390/su13147959
40. Goovaerts P, Jacquez GM. Accounting for regional background and population size in the detection of spatial clusters and outliers using geostatistical filtering and spatial neutral models: the case of lung cancer in Long Island, New York. Int J Health Geogr. (2004) 3. doi: 10.1186/1476-072X-3-14
41. Chen KC, Tsai SW, Shie RH, Zeng C, Yang HY. Indoor air pollution increases the risk of lung cancer. Int J Environ Res Public Health. (2022) 19:1164. doi: 10.3390/ijerph19031164
42. Yang T, Chen R, Gu X, Xu J, Yang L, Zhao J, et al. Association of fine particulate matter air pollution and its constituents with lung function: The China Pulmonary Health study. Environ Int. (2021) 156:106707. doi: 10.1016/j.envint.2021.106707
43. Yu P, Xu R, Wu Y, Huang W, Coelho ZS, Saldiva PHN, et al. Cancer mortality risk from short-term PM2.5 exposure and temporal variations in Brazil. J Hazard Mater. (2024) 473:134606. doi: 10.1016/j.jhazmat.2024.134606
44. Bradford DF, Stanley KA, Tallent NG, Sparling DW, Nash MS, Knapp RA, et al. Temporal and spatial variation of atmospherically deposited organic contaminants at high elevation in yosemite national park, California, USA. Environ Toxicol Chem. (2013) 32:517–25. doi: 10.1002/etc.2094
45. Li M, Shi X, Li X, Ma W, He J, Liu T. Epidemic forest: a spatiotemporal model for communicable diseases. Ann Am Assoc Geogr. (2019) 109:812–36. doi: 10.1080/24694452.2018.1511413
46. Yoshikawal T, Aoyagi Y, Kodama K, Kamijo T, Yonou H, Yokose T, et al. Topographical distribution of allelic loss in individual lung adenocarcinomas with lymph node metastases. Modern Pathol. (2004) 17:204–13. doi: 10.1038/modpathol.3800035
47. Prajapati R, Dahal A, Khanal A, Sharma P, Shrestha P, Kandel R, et al. Status of occupational health and safety in nepal: current scenario and strategies for improvement. J Multidiscip Res Adv. (2023) 1:61194. doi: 10.3126/jomra.v1i2.61194
48. Dhimal M, Bista B, Bhattarai S, Prasai L, Rani M, Agrawal N, et al. NoncommunicableDisease Risk Factors: STEPSSurvey Nepal 2019. (2020). Available at: https://www.who.int/docs/default-source/nepal-documents/ncds/ncd-steps-survey-2019-compressed.pdf (accessed April 13, 2024).
49. Ghimire S, Ghimire S, Singh DR, Sagtani RA, Paudel S. Factors influencing the utilisation of National health insurance program in urban areas of Nepal: Insights from qualitative study. PLOS Global Public Health. (2024) 4:3538. doi: 10.1371/journal.pgph.0003538
50. Moon G. Health geography. Int Encyclop Hum Geogr. (2009) 35–45. doi: 10.1016/B978-008044910-4.00338-2
51. Gregg Greenough P, Nelson EL. Beyond mapping: a case for geospatial analytics in humanitarian health. Conflict Health. (2019) 13:1–14. doi: 10.1186/s13031-019-0234-9
52. Pradhananga KK, Baral M, Shrestha BM. Multi-institution hospital-based cancer incidence data for Nepal: an initial report. Asian Pac J Cancer Prev. (2009) 10:259–62.
53. Liao W-B, Ju K, Zhou Q, Gao YM, Pan J. Forecasting PM2.5-induced lung cancer mortality and morbidity at county level in China using satellite-derived PM2.5 data from 1998 to 2016: a modeling study. Environ Sci Pollut Res. (2020) 27:22946–55. doi: 10.1007/s11356-020-08843-9
54. Dehghani M, Keshtgar L, Javaheri MR, Derakhshan Z, Conti GO, Zuccarello P, et al. The effects of air pollutants on the mortality rate of lung cancer and leukemia. Mol Med Rep. (2017) 15:3390–7. doi: 10.3892/mmr.2017.6387
55. Bu X, Xie Z, Liu J, Wei L, Wang X, Chen M, et al. Global PM2.5-attributable health burden from 1990 to 2017: estimates from the Global Burden of disease study 2017. Environ Res. (2021) 197:111123. doi: 10.1016/j.envres.2021.111123
56. CBS. Environment Statistics of Nepal, 2019. 1st ed., Vol. 1. Central Bureau of Statistics (2019).
57. Liang L, Gong P. Urban and air pollution: a multi-city study of long-term effects of urban landscape patterns on air quality trends. Sci Rep. (2020) 10:18618. doi: 10.1038/s41598-020-74524-9
58. Shaddick G, Thomas ML, Mudu P, Ruggeri G, Gumy S. Half the world's population are exposed to increasing air pollution. Npj Clim Atmos Sci. (2020) 3:23. doi: 10.1038/s41612-020-0124-2
59. Bakrania S. Urbanisation and Urban Growth in Nepal (GSDRC Helpdesk Research Report 1294). Birmingham: GSDRC; University of Birmingham (2015).
60. Adhikari S, Subedi S, Sharma P. Cancer treatment in Nepal: a historical background, development of treatment facilities, epidemiology and challenges for prevention and control of cancer. MOJ Proteomics Bioinform. (2012) 7:205–12. doi: 10.15406/mojpb.2018.07.00247
61. Wen TH, Lin NH, Lin CH, King CC, Su MD. Spatial mapping of temporal risk characteristics to improve environmental health risk identification: a case study of a dengue epidemic in Taiwan. Sci Tot Environ. (2006) 367:631–40. doi: 10.1016/j.scitotenv.2006.02.009
Keywords: lung cancer, spatiotemporal distribution, PM2.5, pollution, Bagmati province
Citation: Neupane BK, Acharya BK, Cao C, Xu M, Taylor PK, Wang S and Yang Y (2024) Lung cancer risk and its potential association with PM2.5 in Bagmati province, Nepal—A spatiotemporal study from 2012 to 2021. Front. Public Health 12:1490973. doi: 10.3389/fpubh.2024.1490973
Received: 04 September 2024; Accepted: 25 November 2024;
Published: 16 December 2024.
Edited by:
Giovanna Deiana, University of Sassari, ItalyReviewed by:
Evangelia Nena, Democritus University of Thrace, GreeceXianmang Xu, Qilu University of Technology, China
Copyright © 2024 Neupane, Acharya, Cao, Xu, Taylor, Wang and Yang. This is an open-access article distributed under the terms of the Creative Commons Attribution License (CC BY). The use, distribution or reproduction in other forums is permitted, provided the original author(s) and the copyright owner(s) are credited and that the original publication in this journal is cited, in accordance with accepted academic practice. No use, distribution or reproduction is permitted which does not comply with these terms.
*Correspondence: Min Xu, eHVtaW5AYWlyY2FzLmFjLmNu
†ORCID: Basanta Kumar Neupane orcid.org/0000-0001-9001-0162