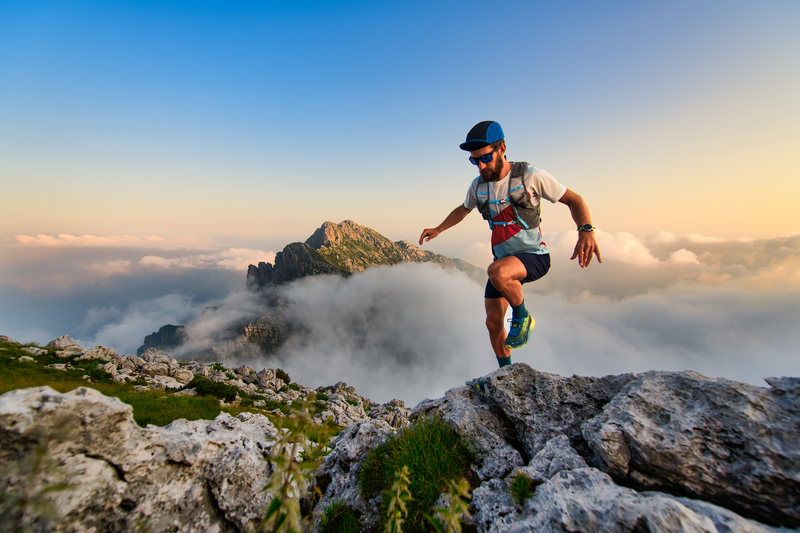
95% of researchers rate our articles as excellent or good
Learn more about the work of our research integrity team to safeguard the quality of each article we publish.
Find out more
SYSTEMATIC REVIEW article
Front. Public Health , 04 December 2024
Sec. Aging and Public Health
Volume 12 - 2024 | https://doi.org/10.3389/fpubh.2024.1476916
This article is part of the Research Topic Assistive Technologies in Aging and Disability View all 5 articles
Introduction: Monitoring daily activities in older adults using sensor technologies has grown significantly over the past two decades, evolving from simple tools to advanced systems that integrate Artificial Intelligence (AI) and the Internet of Things (IoT) for predictive monitoring. Despite these advances, there is still a need for a comprehensive review that addresses both technological progress and its impact on autonomous aging.
Objective: To conduct a systematic review of sensor technologies used to monitor the daily activities of independent older adults, focusing on sensor types, applications, usage contexts, and their evolution over time.
Methodology: A search was conducted in PubMed, Scopus, Web of Science, PsycInfo, and Google Scholar databases, covering studies published between 2000 and 2024. The 37 selected studies were assessed in terms of methodological quality and organized into four chronological stages, allowing for an examination of the progressive development of these technologies. Each stage represents an advance in sensor type, technological application, and implementation context, ranging from basic sensors to intelligent systems in multi-resident homes.
Results: Findings indicate a clear progression in the accuracy and applicability of sensors, which evolved from fall detection to predictive interventions tailored to each user’s needs. Furthermore, the taxonomic classification of studies shows how sensors have been adapted to monitor physical, cognitive, and social dimensions, laying the groundwork for personalized care.
Conclusion: Sensors represent a promising tool for promoting the independence and well-being of older adults, enabling proactive and personalized interventions in everyday settings. However, the lack of standardization in key parameters limits comparability between studies and highlights the need for consensus to facilitate the design of effective interventions that promote autonomous and healthy aging.
Population aging is a global phenomenon that presents significant challenges for healthcare and welfare systems. The World Health Organization (WHO) estimates that by 2050, one in six people will be aged 60 or older, doubling the current proportion of older adults (1). This shift entails an increase in longevity as well as a growing demand for care models that prioritize autonomy and quality of life for older adults. In this context, the traditional association of old age with illness or dependency is gradually shifting, particularly as older adults reach advanced ages while maintaining high levels of functionality and well-being. Thus, the current “active aging” paradigm underscores the importance of maximizing opportunities for health, participation, and security, fostering a view of aging focused on personal development and independence (2).
Simultaneously, active aging requires tools that can support this comprehensive approach, assisting in health monitoring and management without compromising autonomy. Emerging technologies, particularly environmental sensors and wearable devices have shown great promise as solutions in this area (3). Initially, sensor use was limited to emergency detection and health event monitoring in highly dependent older adults, such as falls or dementia-related incidents. However, technological advancements have significantly expanded these applications, which now also benefit healthy and active older adults. Modern sensors enable continuous, non-invasive monitoring of physical, social, and cognitive activity parameters, providing data that enhances understanding of practices that contribute to the preservation of independence and quality of life in old age (4).
Furthermore, the integration of sensors with advanced technologies such as the Internet of Things (IoT) and Artificial Intelligence (AI) has further expanded their applications, enabling environmental monitoring at home in ways that adapt to the daily routines of older adults (5). These technologies capture data on daily activities, offering the opportunity for proactive assessment of changes in behavior and functional health. Unlike traditional monitoring models, which focus on reactive interventions, this approach allows for the anticipation of health issues and the customization of personalized interventions to meet the evolving needs of older adults (6, 7). By analyzing patterns of physical, social, and cognitive activity, AI can help identify practices and metrics associated with the preservation of functionality in autonomous older adults, thus optimizing preventive care approaches at this stage of life (8).
Within the framework of active aging, the use of these technologies provides benefits that go beyond health monitoring (9, 10). By capturing data that reflects the daily lives of older adults, these tools offer a holistic view of well-being, identifying practices that contribute to both physical health and personal development (11). The ability of these devices to analyze indicators of autonomy and adaptation is essential for promoting healthy and fulfilling aging. In particular, cognitive and social activities, such as interacting with others or engaging in intellectual pursuits, have been observed to play a crucial role in maintaining autonomy and reducing the risk of cognitive decline (12).
This study aimed to conduct a systematic review of sensor technologies used to monitor the daily activities of independent older adults, analyzing in depth not only the types of sensors used but also their applications, contexts of use, and specific objectives. Through a chronological organization in stages and an application taxonomy, this review provided a comprehensive view of the progress of these technologies, from basic detection tools to advanced predictive and personalized monitoring systems. This approach aimed to lay the groundwork for designing tailored interventions that promote independence and well-being in older adults, contributing to a better understanding of how these technologies can facilitate autonomous and healthy aging at home.
We conducted a systematic review of scientific literature published between 2000 and 2024, along with a narrative synthesis of the selected studies. The search took place between May and October 2023 and followed four main phases: literature search, article selection, assessment of methodological quality, and data extraction and interpretation. Researchers evaluated the quality of the studies using specific checklists tailored to each study design: the STROBE checklist for observational studies (13), the CONSORT checklist for clinical trials (14), and the PRISMA statement for systematic reviews (15). The review included only studies that met a minimum percentage of relevant quality items on their respective checklists.
Data sources included PubMed, Scopus, Web of Science, PsycINFO, and Google Scholar. The search strategy was structured using the Boolean terms “AND” and “OR” to connect terms across two main topics. Search terms included combinations such as: (ag* OR old* OR older*) AND (“free-living” OR “community-dwelling” OR home) AND (“activities of daily living” OR ADL OR “personal development” OR growth OR “cognitive activities” OR “physical activity” OR “social activity” OR care* OR recognize*) AND (tech OR wearable OR sens OR device OR app OR smartphone OR “smart home” OR “human activity recognition” OR HAR OR “artificial intelligence” OR “deep learning” OR “neural network*” OR “supervised learning” OR “unsupervised learning”).
The inclusion criteria were: (a) studies evaluating activities of daily living (ADL), instrumental activities of daily living (IADL), and aspects of personal development (physical, cognitive, and social) in older adults; (b) studies that employed monitoring technologies or sensors to assess ADL, IADL, personal development, or safety in older adults; (c) studies focusing on older adults without health conditions that significantly impaired mobility or autonomy; and (d) studies conducted in everyday living environments (“free-living”) where participants resided in their own homes.
The exclusion criteria included: (a) studies exclusively focused on fall prevention or detection without evaluating other physical, cognitive, or social aspects, except for early studies (2000–2005) that, although centered on fall detection, contributed relevant technological advances for algorithm and sensor development; (b) studies dedicated solely to monitoring physical activity without considering other dimensions; (c) studies analyzing assistive technologies without continuous monitoring; (d) studies conducted only in experimental settings without real-life applications; and (e) studies published before 2000.
Four researchers initially screened the studies, and a fifth evaluator resolved any discrepancies. They reviewed titles and abstracts first, followed by a thorough evaluation of the full texts to confirm that each study met the inclusion criteria.
The systematic review followed the PRISMA method to ensure a thorough and transparent process for selecting and evaluating relevant literature. Following recommendations, we expanded the search, identifying a total of 172 documents from various sources. After removing 92 duplicates, 80 unique documents remained for initial evaluation. In the preselection phase, we excluded 14 articles that did not meet relevance or methodological quality standards. The remaining 66 documents underwent full review, and we excluded 29 of these due to methodological limitations or insufficient data. This process resulted in 37 studies that met all inclusion criteria and were incorporated into the qualitative synthesis (see Figure 1).
To facilitate analysis, we organized the 37 studies into four chronological stages, reflecting advancements in sensor technologies for monitoring older adults. We evaluated each study’s methodological quality based on sample size, bias control, follow-up duration, and reproducibility, using the STROBE and CONSORT criteria. Supplementary Tables S1, S2 provide further detail: one outlines the specific criteria for each methodological quality item, and the other presents a detailed evaluation of each study according to these criteria.
The review reveals a clear and continuous progression in sensor development and applications for older adults, structured into four chronological stages (see Table 1 in the main article). These advancements enabled sensors to evolve from basic fall detection tools in home environments to complex predictive and personalized monitoring systems in multi-resident communities, enhanced by AI.
The focus and key technologies of each stage are detailed below:
Stage 1 (2000–2005): Early studies employed contact sensors and basic accelerometers, primarily focusing on fall detection and simple activity monitoring. This phase was crucial for testing sensor feasibility with older adults, enabling the capture of movement patterns and specific events. Although some studies, such as Kangas et al. (19), focused solely on fall detection, their inclusion in this review is justified by their contribution to early algorithm and sensor technology development, laying the foundation for continuous monitoring in everyday contexts.
Stage 2 (2006–2010): In this stage, the use of triaxial accelerometers and wireless networks advanced remote monitoring, allowing for applications like activity pattern and gait speed assessment. Sensors began capturing real-time information in smart homes, supporting immediate interventions for falls or mobility changes.
Stage 3 (2011–2015): This period saw the integration of advanced environmental sensors and combined accelerometers and gyroscopes, enabling applications focused on maintaining independence in daily activities and monitoring cognitive health. These systems supported continuous, detailed monitoring in homes and assisted living environments, allowing for the detection of changes in functionality and cognition among older adults.
Stage 4 (2016–2024): In this most recent stage, IoT and AI technologies expanded monitoring scope, emphasizing prediction and personalization. Studies in this period utilized advanced devices to detect and prevent falls and conduct predictive analyses on functional decline. Monitoring also extended to social aspects, capturing interaction patterns in multi-resident settings and enabling proactive, personalized support for older adults.
Table 1 summarizes these advancements across three key dimensions:
Type of Sensor: Progressing from contact sensors and basic accelerometers in Stage 1 to advanced IoT and AI devices in Stage 4.
Technological Applications: Evolving from fall detection in Stage 1 to predictive analysis and social monitoring in Stage 4.
Context of Use: Shifting from home environments in Stage 1 to multi-resident communities in Stage 4, allowing for adaptive and continuous monitoring.
Table 2 presents a taxonomy of sensor use in older adults, organized into three dimensions: population profile, monitoring objectives, and technological configuration. This structure illustrates how sensor applications adapt to different health contexts and specific population needs:
1. Target Population: Classification of studies based on healthy older adults, those with specific conditions (such as cognitive or mobility limitations), and groups in various settings (homes, assisted living facilities, hospitals).
2. Purpose of Use: Monitoring objectives, including daily activities (ADLs and IADLs), fall detection, cognitive and social well-being, and the management of specific health conditions.
3. Implementation Method: Details the technological configuration of sensors, specifying placement (such as wrist or environment), type of technology (wearables or environmental sensors), and sampling frequency.
This taxonomy provides a clear and organized reference that facilitates the identification of sensor applications across various daily life contexts, helping to understand how technology can adapt to the needs and characteristics of different groups of older adults.
The progression of sensor technology in monitoring older adults, organized into stages, demonstrates significant progress from basic devices to advanced technologies incorporating AI and IoT. This progression reflects not only improvements in the accuracy and reach of these technologies but also a shift toward a more comprehensive, personalized approach that encompasses the physical, cognitive, and social dimensions of aging. Recent studies underscore the importance of these advancements, showing that integrating sensors into everyday life can improve quality of life and support autonomy in aging (16).
The taxonomic classification presented in this review offers a new perspective by structuring sensor use according to ‘the target population,’ ‘purposes,’ and ‘implementation methods.’ This approach facilitates the grouping of studies according to specific monitoring objectives, older adults’ profiles, and application contexts, enabling the identification of usage patterns and highlighting areas for future research. Although early studies focused on older adults without complex conditions, the evolution of sensors has enabled greater personalization, adapting to subgroups with special needs, such as those with cognitive impairment or reduced mobility, and providing more in-depth monitoring oriented toward early intervention (17, 18).
From a practical standpoint, monitoring cognitive and social aspects represents an advance toward a holistic approach to health, going beyond an exclusively physical focus. Currently, researchers often infer cognitive activity indirectly, typically from movement patterns or social interactions (19, 20). Incorporating direct metrics on these activities would allow researchers and clinicians to gain a more detailed view of how cognitive and reflective practices contribute to personal development and healthy aging. Activities such as introspection and self-analysis have shown positive impacts on self-perception and adaptability in later life, which are associated with successful aging (21).
The variability in sensor configurations across studies also underscores the need to establish standards for parameters such as sampling frequency, device placement, and monitoring duration. Recent studies indicate that a lack of uniformity in these aspects limits the comparability and generalizability of findings (16, 17). In this regard, the adoption of uniform guidelines would not only improve the consistency of results but would also facilitate the application of these technologies in both clinical and everyday settings, allowing for more effective and accessible integration into health and social care systems for older people.
The progression of AI and IoT integration allows unprecedented levels of personalization in monitoring and adapting to real-time changes in older adults’ health status. This advancement holds significant implications for the future of healthcare, as it enables preventive interventions before critical events occur. Predictive capabilities are essential in the context of aging, allowing proactive planning that reduces reliance on intensive medical services and enhances the quality of life (12). However, using AI in monitoring also raises ethical and privacy concerns that must be addressed carefully, especially in multi-resident environments (18).
This review, while comprehensive, has certain limitations inherent to our methodology and approach. First, our reliance on studies published primarily in English and from the year 2000 onward may introduce selection bias, potentially excluding relevant research in other languages or earlier work that could offer additional historical context on sensor technology in aging populations (15).
Second, there is considerable variability in study design and reporting across the selected literature, especially regarding sensor placement, sampling frequencies, and data processing methods. These methodological inconsistencies limit the comparability of findings and the ability to generalize conclusions across studies. Standardizing parameters such as sensor placement and data processing would enhance data consistency, facilitating broader application of results in clinical and research settings (16, 17).
Third, given the rapid evolution of sensor technology, particularly with advances in Artificial Intelligence (AI) and the Internet of Things (IoT), some findings from this review may become quickly outdated, impacting the long-term relevance of our conclusions (18).
Lastly, while we aimed to capture a balanced view of applications in physical, cognitive, and social monitoring, the existing literature tends to emphasize physical activity monitoring, with less focus on cognitive and social activities. Although some studies infer cognitive and social engagement indirectly, the limited direct monitoring of these domains restricts our ability to fully assess their impact on functional independence and quality of life in older adults (4, 6).
In conclusion, combining chronological and taxonomic perspectives in this review provides a comprehensive understanding of sensor use in older adults. This analysis highlights the technological advancements achieved, as well as the pressing need to establish standards and ethical frameworks that maximize their utility and accessibility in both clinical and everyday applications. Sensor-based monitoring, tailored for the physical, cognitive, and social needs of older adults, presents a significant opportunity to transform older adults care, fostering truly autonomous, healthy, and enriching aging.
While this systematic review demonstrates clear progress in the use of sensors to promote autonomy and healthy aging, challenges remain that continue to limit their long-term implementation and effectiveness. The lack of consensus on technical standards regarding sensor configuration, placement, and data interpretation hinders the creation of a unified framework that allows meaningful comparisons across studies. Furthermore, reliance on indirect methods for assessing cognitive and reflective activities restricts the scope of data obtained, underscoring the need for innovations that capture these essential aspects of aging more directly and in greater detail.
Overall, the findings of this review emphasize the potential of sensors to transform monitoring in older adults into tools for proactive and personalized intervention. Achieving greater standardization and developing technologies that address cognitive and reflective dimensions of aging will represent essential steps toward fully harnessing these tools. As these advancements become more established, sensor use could offer an increasingly effective strategy to foster autonomous, resilient, and meaningful aging, aligned with the growing demands of aging societies.
The original contributions presented in the study are included in the article/Supplementary material, further inquiries can be directed to the corresponding author.
CR: Conceptualization, Formal analysis, Investigation, Methodology, Resources, Validation, Visualization, Writing – original draft, Writing – review & editing. MP-C: Conceptualization, Formal analysis, Methodology, Resources, Supervision, Validation, Writing – original draft, Writing – review & editing. PÁ-M: Conceptualization, Investigation, Supervision, Visualization, Writing – original draft, Writing – review & editing. VL-F: Conceptualization, Formal analysis, Investigation, Methodology, Supervision, Writing – original draft, Writing – review & editing.
The author(s) declare that financial support was received for the research, authorship, and/or publication of this article. This manuscript is part of the information-gathering phase of the project titled “Metric for monitoring the functional independence of healthy older adults,” funded by the Ministry of Science and Innovation from Spain funded (2022–2024), Ref. 2022/00216/001.
The authors declare that the research was conducted in the absence of any commercial or financial relationships that could be construed as a potential conflict of interest.
All claims expressed in this article are solely those of the authors and do not necessarily represent those of their affiliated organizations, or those of the publisher, the editors and the reviewers. Any product that may be evaluated in this article, or claim that may be made by its manufacturer, is not guaranteed or endorsed by the publisher.
The Supplementary material for this article can be found online at: https://www.frontiersin.org/articles/10.3389/fpubh.2024.1476916/full#supplementary-material
1. Organización Mundial de la Salud. Ageing and health. (2021). Available at: https://www.who.int/news-room/fact-sheets/detail/ageing-and-health (Accessed August 24, 2024).
2. López-López, R, and Sánchez, M. The institutional active aging paradigm in Europe (2002–2015). Gerontologist. (2020) 60:406–15. doi: 10.1093/geront/gnz094
3. Pramanik, P, Pal, S, and Choudhury, P. Beyond automation: the cognitive IoT. Artificial intelligence brings sense to the internet of things. Lecture Notes on Data Eng Commun Technol. (2018) 14:1–37. doi: 10.1007/978-3-319-70688-7_1
4. Kaye, JA, Maxwell, SA, Mattek, N, Hayes, TL, Dodge, H, Pavel, M, et al. Intelligent systems for assessing aging changes: home-based, unobtrusive, and continuous assessment of aging. J Gerontol B Psychol Sci Soc Sci. (2011) 66B:i180–90. doi: 10.1093/geronb/gbq095
5. González, I, Fontecha, J, Hervás, R, and Bravo, J. Estimation of temporal gait events from a single accelerometer through the scale-space filtering idea. J Med Syst. (2016) 40:1–10. doi: 10.1007/s10916-016-0612-4
6. Naccarelli, R, Casaccia, S, and Revel, GM. The problem of monitoring activities of older people in multi-resident scenarios: an innovative and non-invasive measurement system based on wearables and PIR sensors. Sensors. (2022) 22:1–23. doi: 10.3390/s22093472
7. Dorado-Chaparro, J, Ruiz Fernandez, J, Romero-Santofimia, MJ, Bolaños-Peño, C, Unzueta-Irurtia, L, García-Perea, MG, et al. Healthy and active ageing. Sensors. (2021) 21:1–40. doi: 10.3390/s21237938
8. Reiman, A, and Väyrynen, S. Holistic well-being and sustainable organisations–a review and argumentative propositions. Int J Sustain Eng. (2018) 11:321–9. doi: 10.1080/19397038.2018.1474397
9. Othaganont, P, Sinthuvorakan, C, and Jensupakarn, P. Daily living practice of the life-satisfied Thai elderly. J Transcult Nurs. (2002) 13:24–9. doi: 10.1177/104365960201300105
10. Cicirelli, G, Marani, R, Petitti, A, Milella, A, and D’orazio, T. Ambient assisted living: a review of technologies, methodologies and future perspectives for healthy aging of population. Sensors. (2021) 21:1–22. doi: 10.3390/s21103549
11. Alahi, E, Sukkuea, A, Tina, FW, Nag, A, Kurdthongmee, W, Suwannarat, K, et al. Integration of IoT-enabled technologies and artificial advancements and future trends. Sensors. (2023) 23:1–36. doi: 10.3390/s23115206
12. Rashidi, P, and Mihailidis, A. A survey on ambient-assisted living tools for older adults. IEEE J Biomed Heal Informatics. (2013) 17:579–90. doi: 10.1109/JBHI.2012.2234129
13. von Elm, E, Altman, DG, Egger, M, Pocock, SJ, Gøtzsche, PC, and Vandenbroucke, JP. The strengthening the reporting of observational studies in epidemiology (STROBE) statement: guidelines for reporting observational studies. J Clin Epidemiol. (2008) 61:344–9. doi: 10.1016/j.jclinepi.2007.11.008
14. Cobos-Carbó, A, and Augustovski, F. Declaración CONSORT 2010: actualización de la lista de comprobación para informar ensayos clínicos aleatorizados de grupos paralelos. Med Clin (Barc). (2011) 137:213–5. doi: 10.1016/j.medcli.2010.09.034
15. Urrutia, G, and Bonfill, X. PRISMA declaration: a proposal to improve the publication of systematic reviews and meta-analyses. Med Clin (Barc). (2010) 135:507–11. doi: 10.1016/j.medcli.2010.01.015
16. Hayes, TL, Abendroth, F, Adami, A, Pavel, M, Zitzelberger, TA, and Kaye, JA. Un obtrusive assessment of activity patterns associated with mild cognitive impairment. Alzheimers Dement. (2008) 4:395–405. doi: 10.1016/j.jalz.2008.07.004
17. Bourke, AK, O’Brien, JV, and Lyons, GM. Evaluation of a threshold-based tri-axial accelerometer fall detection algorithm. Gait Posture. (2007) 26:194–9. doi: 10.1016/j.gaitpost.2006.09.012
18. Christoforou, EG, Panayides, AS, Avgousti, S, Masouras, P, and Pattichis, CS. “An overview of assistive robotics and technologies for elderly care.” In Mediterranean conference on medical and biological engineering and computing. In: XV Mediterranean conference on medical and biological engineering and computing–MEDICON 2019: Proceedings of MEDICON 2019. Berlin: Springer; (2019). p. 971–976.
19. Kangas, M, Konttila, A, Lindgren, P, Winblad, I, and Jämsä, T. Comparison of low-complexity fall detection algorithms for body attached accelerometers. Gait Posture. (2008) 28:285–91. doi: 10.1016/j.gaitpost.2008.01.003
20. Orr, RJ, and Abowd, GD. The smart floor: a mechanism for natural user identification and tracking. Conf Hum Factors Comput Syst-Proc. (2000):275–6.
21. Sixsmith, A, and Johnson, N. A smart sensor to detect the falls of the elderly. IEEE Pervasive Comput. (2004) 3:42–7. doi: 10.1109/MPRV.2004.1316817
22. Mathie, MJ, Coster, ACF, Lovell, NH, and Celler, BG. Accelerometry: providing an integrated, practical method for long-term, ambulatory monitoring of human movement. Physiol Meas. (2004) 25:R1–R20. doi: 10.1088/0967-3334/25/2/R01
23. Tapia, EM, Larson, K, and Intille, S. “Activity recognition in the home setting using simple and ubiquitous sensors Emmanuel Munguia Tapia.” In: International conference on pervasive computing. Springer; (2004). p. 158–175.
24. Alemdar, H, and Ersoy, C. Wireless sensor networks for healthcare: a survey. Comput Netw. (2010) 54:2688–710. doi: 10.1016/j.comnet.2010.05.003
25. Chan, M, Campo, E, Estève, D, and Fourniols, JY. Smart homes - current features and future perspectives. Maturitas. (2009) 64:90–7. doi: 10.1016/j.maturitas.2009.07.014
26. Hagler, S, Austin, D, Hayes, TL, Kaye, J, and Pavel, M. Un obtrusive and ubiquitous in-home monitoring: a methodology for continuous assessment of gait velocity in elders. IEEE Trans Biomed Eng. (2010) 57:813–20. doi: 10.1109/TBME.2009.2036732
27. Virone, G, Alwan, M, Dalal, S, Kell, SW, Turner, B, Stankovic, JA, et al. Behavioral patterns of older adults in assisted living. IEEE Trans Inf Technol Biomed. (2008) 12:387–98. doi: 10.1109/TITB.2007.904157
28. Zouba, N, Bremond, F, and Thonnat, M. “An activity monitoring system for real elderly at home: validation study.” In: Proceedings-IEEE international conference on advanced video and signal based surveillance, AVSS 2010. IEEE; (2010). p. 278–285.
29. Dawadi, PN, Cook, DJ, Schmitter-Edgecombe, M, and Parsey, C. Automated assessment of cognitive health using smart home technologies. Technol Health Care. (2013) 21:323–43. doi: 10.3233/THC-130734
30. Fleury, A, Vacher, M, and Noury, N. SVM-based multimodal classification of activities of daily living in health smart homes: sensors, algorithms, and first experimental results. IEEE Trans Inf Technol Biomed. (2010) 14:274–83. doi: 10.1109/TITB.2009.2037317
31. Nef, T, Urwyler, P, Büchler, M, Tarnanas, I, Stucki, R, Cazzoli, D, et al. Evaluation of three state-of-the-art classifiers for recognition of activities of daily living from smart home ambient data. Sensors. (2015) 15:11725–40. doi: 10.3390/s150511725
32. Dasios, A, Gavalas, D, Pantziou, G, and Konstantopoulos, C. Hands-on experiences in deploying cost-effective ambient-assisted living systems. Sensors. (2015) 15:14487–512. doi: 10.3390/s150614487
33. Ni, Q, García Hernando, AB, and de la Cruz, I. The elderly’s independent living in smart homes: a characterization of activities and sensing infrastructure survey to facilitate services development. Sensors. (2015) 15:11312–62. doi: 10.3390/s150511312
34. Garcia-Moreno, FM, Bermudez-Edo, M, Garrido, JL, Rodríguez-García, E, Pérez-Mármol, JM, and Rodríguez-Fórtiz, MJ. A microservices e-health system for ecological frailty assessment using wearables. Sensors. (2020) 20:1–23. doi: 10.3390/s20123427
35. Muangprathub, J, Sriwichian, A, Wanichsombat, A, Kajornkasirat, S, Nillaor, P, and Boonjing, V. A novel elderly tracking system using machine learning to classify signals from mobile and wearable sensors. Int J Environ Res Public Health. (2021) 18:1–19. doi: 10.3390/ijerph182312652
36. Debes, C, Merentitis, A, Sukhanov, S, Niessen, M, Frangiadakis, N, and Bauer, A. Monitoring activities of daily living in smart homes: understanding human behavior. IEEE Signal Process Mag. (2016) 33:81–94. doi: 10.1109/MSP.2015.2503881
37. Moschetti, A, Fiorini, L, Esposito, D, Dario, P, and Cavallo, F. Recognition of daily gestures with wearable inertial rings and bracelets. Sensors (Switzerland). (2016) 16:1–18. doi: 10.3390/s16081341
38. Schrack, JA, Leroux, A, Fleg, JL, Zipunnikov, V, Simonsick, EM, Studenski, SA, et al. Using heart rate and Accelerometry to define quantity and intensity of physical activity in older adults. J Gerontol-Ser A Biol Sci Med Sci. (2018) 73:668–75. doi: 10.1093/gerona/gly029
39. Sepesy Maučec, M, and Donaj, G. Discovering daily activity patterns from sensor data sequences and activity sequences. Sensors. (2021) 21:920. doi: 10.3390/s21206920
40. Schrack, JA, Cooper, R, Koster, A, Shiroma, EJ, Murabito, JM, Rejeski, WJ, et al. Assessing daily physical activity in older adults: unraveling the complexity of monitors, measures, and methods. J Gerontol-Ser A Biol Sci Med Sci. (2016) 71:1039–48. doi: 10.1093/gerona/glw026
41. Vervoort, D, Vuillerme, N, Kosse, N, Hortobágyi, T, and Lamoth, CJ. Multivariate analyses and classification of inertial sensor data to identify aging effects on the timed-up-and-go test. PLoS One. (2016) 11:e0155984. doi: 10.1371/journal.pone.0155984
42. Gomez-Ramos, R, Duque-Domingo, J, Zalama, E, and Gómez-García-Bermejo, J. Daily human activity recognition using non-intrusive sensors. Sensors. (2021) 21:5270. doi: 10.3390/s21165270
43. Igarashi, T, Nihei, M, Inoue, T, Sugawara, I, and Kamata, M. Eliciting a User’s preferences by the self-disclosure of socially assistive robots in local households of older adults to facilitate verbal human–robot interaction. Int J Environ Res Public Health. (2022) 19:1–18. doi: 10.3390/ijerph191811319
44. Papagiannaki, A, Zacharaki, EI, Kalouris, G, Kalogiannis, S, Deltouzos, K, Ellul, J, et al. Recognizing physical activity of older people from wearable sensors and inconsistent data. Sensors. (2019) 19:1–19. doi: 10.3390/s19040880
45. Sasaki, JE, Hickey, A, Staudenmayer, J, John, D, Kent, JA, and Freedson, PS. Performance of activity classification algorithms in free-living older adults. Med Sci Sports Exerc. (2016) 48:941–50. doi: 10.1249/MSS.0000000000000844
46. Genovese, V, Mannini, A, and Sabatini, AM. A smartwatch step counter for slow and intermittent ambulation. Ieee Access. (2017) 5:13028–37. doi: 10.1109/ACCESS.2017.2702066
47. Paraschiakos, S, Cachucho, R, Moed, M, van Heemst, D, Mooijaart, S, Slagboom, EP, et al. Activity recognition using wearable sensors for tracking the elderly. User Model User-Adap Inter. (2020) 30:567–605. doi: 10.1007/s11257-020-09268-2
48. Rejeski, WJ, Marsh, AP, Brubaker, PH, Buman, M, Fielding, RA, Hire, D, et al. Analysis and interpretation of accelerometry data in older adults: the LIFE study. J Gerontol-Ser A Biol Sci Med Sci. (2016) 71:521–8. doi: 10.1093/gerona/glv204
49. Aramendi, AA, Weakley, A, Aztiria Goenaga, A, Schmitter-Edgecombe, M, and Cook, DJ. Automatic assessment of functional health decline in older adults based on smart home data. J Biomed Inform. (2018) 81:119–30. doi: 10.1016/j.jbi.2018.03.009
50. Gochoo, M, Tan, TH, Huang, SC, Liu, SH, and Alnajjar, FS. DCNN-based elderly activity recognition using binary sensors. Int Conf Electr Comput Technol Appl ICECTA 2017. (2017) 2018-Janua:1–5. doi: 10.1109/ICECTA.2017.8252040
Keywords: independent older adults, sensor technologies, activities, technological taxonomy, technological development, systematic review
Citation: Requena C, Plaza-Carmona M, Álvarez-Merino P and López-Fernández V (2024) Technological applications to enhance independence in daily activities for older adults: a systematic review. Front. Public Health. 12:1476916. doi: 10.3389/fpubh.2024.1476916
Received: 06 August 2024; Accepted: 13 November 2024;
Published: 04 December 2024.
Edited by:
Hasheem Mannan, University College Dublin, IrelandReviewed by:
Qi-Song Yan, Chongqing University of Science and Technology, ChinaCopyright © 2024 Requena, Plaza-Carmona, Álvarez-Merino and López-Fernández. This is an open-access article distributed under the terms of the Creative Commons Attribution License (CC BY). The use, distribution or reproduction in other forums is permitted, provided the original author(s) and the copyright owner(s) are credited and that the original publication in this journal is cited, in accordance with accepted academic practice. No use, distribution or reproduction is permitted which does not comply with these terms.
*Correspondence: Carmen Requena, Yy5yZXF1ZW5hQHVuaWxlb24uZXM=
Disclaimer: All claims expressed in this article are solely those of the authors and do not necessarily represent those of their affiliated organizations, or those of the publisher, the editors and the reviewers. Any product that may be evaluated in this article or claim that may be made by its manufacturer is not guaranteed or endorsed by the publisher.
Research integrity at Frontiers
Learn more about the work of our research integrity team to safeguard the quality of each article we publish.