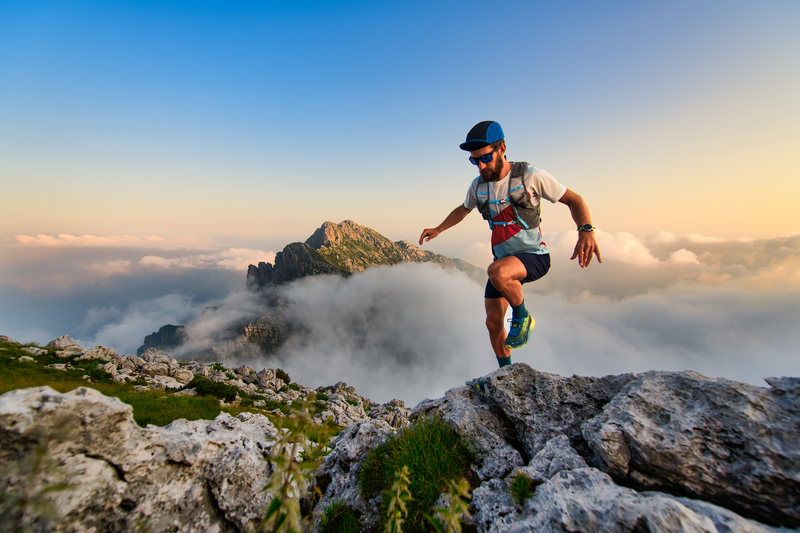
94% of researchers rate our articles as excellent or good
Learn more about the work of our research integrity team to safeguard the quality of each article we publish.
Find out more
ORIGINAL RESEARCH article
Front. Public Health , 22 October 2024
Sec. Public Health and Nutrition
Volume 12 - 2024 | https://doi.org/10.3389/fpubh.2024.1467339
This article is part of the Research Topic Transforming Food Systems: Addressing Malnutrition and Inequality in Low- and Middle-Income Countries View all 17 articles
Introduction: Carrying out studies using data from Food and Nutritional Monitoring is crucial given the current epidemiological scenario. This scenario reveals a coexistence of malnutrition and nutritional deficiencies alongside overweight and obesity, particularly among adult women in Brazil. Analyzing the microdata generated by the system is essential for accurately representing food and nutrition indicators in the country.
Objective: To analyze the association of food consumption markers and sociodemographic factors with changes in the Body Mass Index (BMI) among adult women.
Methodology: A cohort study with a final sample of 30,354 women evaluated through individual records between the years 2015 and 2019. Nutritional status was assessed using body mass index (BMI). Food consumption markers used were the ones available in the system, which referred to foods consumed the previous day. Linear regression was used to evaluate factors associated with BMI and multiple Poisson regression was used to estimate the relative risk of obesity incidence.
Results: There was an increase in BMI between 2015 and 2019. The average increase in BMI was lower in women who consumed, in the previous day, beans (β = −0,25; CI95%: −0.36; −0.13) and vegetables and/or legumes (β = −0.12; CI95%: −0.21; −0.04) and the increase was greater when they consumed hamburgers and/or sausages (β = 0.1; CI95%: 0.02; 0.19) adjusted by age, region and skin color. There was a 14% lower incidence of obesity in the group that consumed beans (RR = 0.86; 95% CI: 0.79; 0.93), and a 9% lower incidence when they consumed filled cookies, candies or treats (RR = 0.91; 95% CI 0.86; 0.96). The incidence of obesity was 11% higher in the group that consumed sweetened drinks (RR = 1.11; 95% CI: 1.05; 1.18) and 9% higher in the group that consumed instant noodles, packaged snacks or savory biscuits on the day before the 2015 registration (RR = 1.09; 95% CI: 1.03; 1.15).
Conclusion: There was an increase in BMI in the population of adult women, rising nationally between 2015 and 2019, with the notable influence of diet on this change.
The Brazilian Food and Nutrition Monitoring System (SISVAN) is a health information system, which functions as a technological tool for monitoring the food and nutritional situation of the Brazilian population receiving primary health care through the Unified Health System [Sistema Único de Saúde—SUS] (1).
It is worth highlighting its notoriety as a primary health care information system and a strong predictor of trends in the analysis of health conditions and the food and nutritional situation in Brazil. Thus, when it comes to adult individuals, especially women, the system becomes particularly useful. It also serves as a management tool for health professionals and managers, making it possible to monitor, manage, analyze and plan actions, supporting decision-making and priorities aimed at promoting and preventing health at a population level, improving the organization and care of nutritional care (2, 3).
The anthropometric assessment records of adult individuals assisted by the system are weight, height and waist circumference, where the cutoff points adopted for the Body Mass Index follow the recommendation of the World Health Organization (4). The index is calculated by dividing the weight in kilograms (kg) by the height in meters (m) squared (kg/m2), which becomes a satisfactory tool for predicting population risks (5). Food consumption markers assess foods that were consumed the previous day, identifying healthy or unhealthy behaviors, according to the recommendations of the food guide for the Brazilian population (6). There is a notable increase in BMI, which confirms the nutritional changes experienced in Brazil and around the world, with a decline in the prevalence of malnutrition and an increase in obesity and overweight individuals (7), and it is object of study among Brazilian adults (8), based on a comprehensive set of surveys between 1975 and 2019, exposing, over time, the classic “X” shape with the nutritional transition being greater and more representative among women, pointing to a decrease in underweight rates among men and women, inversely to the increase in the obesity trajectory among both sexes over the years.
Despite the relevance of the subject, this is the first Brazilian cohort study to date to analyze the association of food consumption markers and sociodemographic factors associated with obesity among adult women, using microdata from the Food and Nutrition Surveillance System, assessing the representativeness of the food and nutrition indicators produced by the system. Thus, the importance of conducting studies with data from this system is highlighted, especially considering the epidemiological scenario, which indicates a simultaneity between malnutrition and nutritional deficiencies, overweight and obesity in the adult population in general (9–11) and especially in women (12). Therefore, the objective of this study was to analyze the association of food consumption markers and sociodemographic factors associated with obesity among adult women.
This is an observational epidemiological study using an historical cohort of adult women from the recorded data on nutritional status and markers of food consumption of SISVAN at a national level, in the period between 2015 and 2019. Cohort studies are important for observing, in a previously defined sample, the incidence of a given disease or phenomenon related to health or illness in a temporal sequence of events over time. This type of study thus allows the identification of possible risk factors, understanding the development of diseases and supporting decisions in public health policies, in addition to serving as a basis for future studies (13). Cohort studies have been considered the most reliable, if not the only method for studying the incidence and natural history of diseases (14, 15). The choice of this period is due to the fact that 2015 was when the new food consumption forms were introduced, and 2019 was the last year before the start of the COVID-19 pandemic. In 2019, no measures to control and prevent the disease had been implemented. Since these measures influenced daily habits and regular access to health services, it is assumed that the data for the year used would represent the usual profile of the target population.
The research complied with the standards of the National Health Council and instructions of regulation number 884, December 13, 2011 (16), on the transfer of data contained in the national databases of information systems managed by the Health Care Department and the Brazilian Health Ministry. The study project was approved by the Human Research Ethics Committee of the University of Fortaleza (UNIFOR) on October 20, 2020 with protocol no. 4,348,452, and Certificate of Presentation for Ethical Assessment n° 31540320.9.1001.5052.
The research analyzed data from adult women from all over Brazil, identified through the National Health Card in the Food and Nutrition Surveillance System (SISVAN), with the analysis units being users of Primary Health Care whose anthropometric records were carried out in 2015 and then in 2019 in their macro-regions (North; Northeast; South; Southeast; Midwest) and Federation Units (FUs) (17).
The data analyzed come from SISVAN, provided by the Ministry of Health, as requested from the General Coordination of Food and Nutrition, the microdata provided are composed of monitoring records from SISVAN itself, its Web version which also includes the migration of data from the Bolsa Família Program; and through the citizen's electronic medical record and simplified data collection software, and the integration of SISVAN with the e-SUS Primary Care Strategy, where the migration of existing data in the Primary Care System (SISAB) to SISVAN database, according to a technical note from the Ministry of Health (18).
The inclusion criteria for this cohort study were, cumulatively: (a) registered in SISVAN, being female, aged between 20 and 59 years in 2015; (b) records of the same individuals in 2019; and (c) the completion of data regarding anthropometry and food consumption in the years 2015 and 2019. We considered exclusion criteria to be: (a) records linked to care programs for pregnant women; (b) duplicate records (food consumption and anthropometry recorded more than once a year), with only records of the last assessment for 2015 and 2019 being kept; (c) biologically implausible values with weight < 30 and ≥300 kg (19); and (d) observations with a height < 1.20 and ≥2.20 m (19). When verifying the incidence of obesity, an additional exclusion criterion was the individual having a BMI ≥ 30 kg/m2 in 2015, the year of the cohort's baseline. The sample consisted of 84,882 women followed up in PHC between 2015 and 2019. After filters and exclusions, and considering only the cases that were present in the databases, the final sample was 30,354 women. The process for obtaining the sample is represented in Figure 1.
Figure 1. Flowchart description for obtaining the final research sample (N = 30,354). Brazil, 2015 and 2019. Source: created by the authors.
Demographic, socioeconomic, food, and nutritional variables were used to characterize and analyze the sample. The demographic and socioeconomic variables used in this study were: geographic region (categorized as North; Northeast; Southeast; South; Central-West), age group (20–29; 30–39; 40–49; 50–59 years), and race/color (white; black; mixed-race; Asian; indigenous) (17).
The classification of nutritional status used by SISVAN is according to the Body Mass Index (BMI), in units of kg/m2. The cutoff points for BMI classification follow the World Health Organization (4) recommendation: obesity, when BMI ≥ 30 kg/m2.
Food consumption markers were collected using a screening form (20) proposed by seven markers, where the foods consumed during the previous day are noted, using an available list, with “yes,” “no,” or “don't know” response options. This enables the recognition of intake related to healthy or unhealthy eating, based on the proposal of the Food Guide for the Brazilian Population (6).
In line with the Food Guide for the Brazilian Population (6), the markers that characterize a healthy diet are: beans; fresh fruit; vegetables and/or legumes; and unhealthy diet are: hamburgers and/or sausages; sweetened drinks; instant noodles, packaged snacks or savory biscuits; and filled cookies, sweets, or treats (21).
The information used for this research came from the General Coordination of Food and Nutrition through the Ministry of Health. The data was organized in an Excel® spreadsheet in October 2022. The R Studio software was used for the process of filters and exclusions, to finalize the final database.
The variable “age group” was calculated based on age categorization, in complete years. The individual difference in BMI was calculated for each participant, based on the BMI value obtained in 2019 subtracted from the BMI value assessed in 2015. The frequency of BMI differences was observed by creating a histogram, which indicated a normal distribution.
The sociodemographic and anthropometric aspects of the sample were characterized using absolute and relative frequency. The average difference in BMI between the studied groups was calculated, and its association with sociodemographic aspects and food consumption was verified. The unadjusted association was estimated using simple linear regression, while the association adjusted for the sociodemographic variables of age, region and skin color was estimated using multiple linear regression. For the linear regression model, the variation in BMI was considered the outcome and the sociodemographic and food consumption aspects were considered exposure.
Food consumption markers were considered the exposure (collected in 2015) and the diagnosis of obesity was considered outcome (collected in 2019). It is noteworthy that cases of obesity prevalence were excluded in 2015.
The incidence rate of obesity was calculated by dividing the new cases in 2019 by the number of individuals at risk in 2015, multiplied by 100.
The incidence of obesity was compared between the groups using the relative risk estimate and its respective 95% confidence interval, obtained by multiple Poisson regression, adjusted for age, region and skin color, with a robust variance estimator. The analyses were carried out at a two-tailed α level of 0.05, with the aid of the Stata Statistical: version 11.2 (College Station, TX: StataCorp LLC).
In the macro-regions, it was observed that the Southeastern region (β = −0.18; 95% CI: −0.28; −0.09) showed a lower increase in BMI when compared to the Northeast, while the opposite occurred in the Central-Western region (β = 0.28; 95% CI: 0.13; 0.42). The increase in BMI occurred in the age groups of 30–39 years (β = −0.3; 95% CI: −0.38; −0.21), 40–49 (β = −0.45; 95% CI: −0.56; −0.34), and 50–59 years (β = −0.69; 95% CI: −0.84; −0.54), compared to the age group of 20–29 years (Table 1).
Table 1. Average difference in BMI units (kg/m2) and association with demographic and socioeconomic aspects among adult women registered in SISVAN (n = 30.354), Brazil, 2015–2019.
The average increase in BMI was lower in those who reported consuming beans the previous day (β = −0.25; 95% CI: −0.36; −0.13) and vegetables (β = −0. 12; 95% CI: −0.21; −0.04) and higher when consuming hamburgers and/or sausages (β = 0.1; 95% CI: 0.02; 0.19) adjusted by age, region and color of skin (Table 2).
Table 2. Average difference in BMI units (kg/m2) and association with markers of food consumption among adult women registered in SISVAN (n = 30.354), Brazil, 2015–2019.
The incidence of obesity (BMI ≥ 30 kg/m2) and association with sociodemographic aspects among adult women (20–59 years old) registered in SISVAN was 11% lower in the Northern region (RR = 0.89; 95% CI: 0.82; 0.96) and 17% higher in the Southern region (RR = 1.17; 95% CI: 1.03; 1.32) when compared to the Northeastern region. Compared to the age group of 20–29 years, the risk of obesity was 20% higher in the age group between 40 and 49 years (RR = 1.28; 95% CI: 1.20; 1.37) and 32% lower in the age group aged 50–59 years (RR = 0.68; 95% CI: 0.60; 0.78). The incidence of obesity was 13% lower in Asian people (RR = 0.87; 95% CI: 0.78; 0.97) when compared to white people (Table 3).
Table 3. Incidence of obesity (BMI ≥ 30 kg/m2) and association with sociodemographic aspects among adult women registered in SISVAN (n = 30.354), Brazil, 2015–2019.
Regarding the incidence of obesity (BMI ≥ 30 kg/m2) and association with markers of food consumption among adult women aged 20–59 years registered in SISVAN, regardless of age, region, and skin color, we observed a 14% lower incidence when individuals said they ate breakfast the day before, compared to individuals who did not consume a breakfast (RR = 0.83; 95% CI: 0.73; 0.95). There was also a 15% lower incidence of obesity in the group that consumed a morning snack (RR = 0.85; 95% CI: 0.80; 0.90), 28% lower in the group that ate lunch (RR = 0.72; 95% CI: 0.58; 0.89), 19% lower in the group that had an afternoon snack (RR = 0.81; 95% CI: 0.76; 0.87) and 10% lower in the group that had an evening meal (RR = 0.9; 95% CI 0.83; 0.98). A 14% lower incidence of obesity was found in the group that consumed beans (RR = 0.86; 95% CI: 0.79; 0.93), and 9% lower when individuals said they consumed filled cookies, candies or treats (RR = 0.91; 95% CI: 0.86; 0.96), 11% higher in the group that consumed sweetened drinks (RR = 1.11; 95% CI: 1.05; 1.18) and 9% higher in the group who consumed instant noodles, packaged snacks or savory biscuits on the day before the 2015 records (RR = 1.09; 95% CI: 1.03; 1.15). In regard to the consumption of vegetables and/or legumes, we observed 7% higher risk of obesity in the unadjusted association (RR = 1.07; 95% CI: 1.01; 1.14) and 7% in the age-adjusted association (RR = 1.07; 95% CI: 1.01; 1.14), however, when analyzed, the association adjusted for age, region and skin color did not appear to be statistically significantly associated (RR = 1.06; 95% CI: 1.00; 1.13; Table 4).
Table 4. Incidence of obesity (BMI ≥ 30 kg/m2) and association with food consumption markers among adult women registered in SISVAN (n = 30.354), Brazil, 2015–2019.
In this cohort study, we identified sociodemographic factors and markers of food consumption associated with BMI and obesity. There was an increase in BMI between 2015 and 2019, which varied between regions and age groups. There was a lower average increase in BMI and a lower incidence of obesity in women who reported eating, in the previous day, foods like beans, vegetables, and/or legumes. There was a greater increase in BMI when they consumed hamburgers and/or sausages. The incidence of obesity was lower with the consumption of beans and filled cookies, candies or treats and higher among women who reported consuming sweetened drinks, instant noodles, packaged snacks or savory biscuits.
When analyzing the increase in BMI of the population of Brazilian adult women in association with demographic aspects, we observed that the Southeastern region showed a lower increase in BMI, compared to the Northeastern region, while the opposite occurred in the Central-Western region. Thus, the Central-Western region leads the increase in an overweight and obesity population in the period analyzed. Regarding this finding, the literature actually provides data on the increased prevalence of being overweight and obesity in this region (22, 23). With reference to the incidence of obesity and its association with sociodemographic aspects, we found a lower incidence in the Northern region and a higher incidence in the Southern region, when compared to the Northeastern region. It is noteworthy that nutritional status can be considered an indicator of health and quality of life, reflecting a development model; thus, the different socioeconomic realities in the Brazilian territory are reflected in the nutritional status, which varies between regions of the country (9, 10).
The increase in BMI over the years in the present study is in line with findings from other nationwide research (8, 19). Therefore, the pattern of obesity in Brazil does not affect the population in a random or homogeneous manner, it tends to vary according to gender and socioeconomic status, as in other developing countries, with a higher prevalence of obesity among women (24, 25). Silva et al. (26) emphasize that women are more likely to experience higher levels of body weight, when these factors are directly associated with conditions such as income, marital status, physical activity, consumption of beans, consumption of alcoholic beverages, and smoking cigarettes, as these are all variables that have a greater relationship with gender inequality.
The number of data records in SISVAN also varied by macro-region. We found evidence that the Northeast presented a greater number of registered records of adult women who were attended to in PHC by the Unified Health System (SUS), in agreement with national studies, indicating improvement in the monitoring of nutritional status over the years in this region (10, 22, 27). This may be due to the monitoring of beneficiary families of the Bolsa Família program, with a greater number of records and monitoring in this region, as this program has greater coverage in the northeast region, due to the presence of higher poverty rates (28).
Despite the consumption of basic and traditional foods in the Brazilian diet, considered healthy markers, there is a high intake of foods with high energy value and low nutritional value, such as processed and ready-to-eat foods, contributing to the increase in being overweight and obesity in the population (29). Data found in this research, which have already been described in the literature, show an association between high consumption of sweetened and ultra-processed drinks, with the incidence of obesity and increased risk of NCDs (30, 31).
According to the Family Budget Survey, the acquisition of these foods rose from 12% in the 2002–2003 (32) survey to 16% in the 2007–2008 (33) survey and then 18.4% in 2017–2018 (34). Ultra-processed food consumption was responsible for ~57,000 premature deaths in Brazil or 10.5% of all premature deaths in adults aged 30–69 in Brazil (29, 35).
The results presented allowed us to identify healthy and unhealthy markers, as well as eating habits of women in Brazil monitored by SISVAN. Thus, we observed a lower increase in BMI in women who reported the habit of consuming at least five meals a day, including breakfast, morning snack, lunch, afternoon snack and evening meal, as well as consuming beans and vegetables and/or legumes the day before, and a higher increase when they consumed hamburgers and/or sausages. People who seem to split their meals have a regular supply of energy, maintenance of blood glucose levels and stabilization of metabolism, even when maintaining or reducing weight (36).
The incidence of obesity was lower with the consumption of beans and filled cookies, candies or treats and higher among women who reported consuming sweetened drinks, instant noodles, packaged snacks, or savory biscuits. We highlight in this research some unexpected findings regarding the incidence of obesity associated with the consumption of vegetables and/or legumes, where we observed a higher relative risk of the incidence of obesity when analyzed by unadjusted association and with the association adjusted by age, however, when we analyzed the association adjusted for age, region and skin color, we did not observe an association, while in regard to fruit intake, which should have some positive association with protective factors in the study, there was no significance in the analyses. Therefore, a possible justification would perhaps be a discrepancy caused by the lower prevalence of intake of these foods.
The intake of filled cookies, candies or treats, which was considered protective against obesity, was not expected. Some aspects should be discussed in relation to this finding. Firstly, the type of instrument used, which does not investigate food quantities consumed. It is possible that to detect the consumption of high amounts of this food group would be associated with a higher incidence of obesity. It should also be noted that within this food group, filled cookies may not necessarily be harmful, depending on the quantity and type of cookie, as the food industry has long been trying to end the impression that cookies are a villain of health and sell more of these products, reducing the presence of unhealthy ingredients and adding healthy ones (37, 38). Thus, the consumption of cookies has increased over the years and new production lines are emerging in the Brazilian food products market, which vary according to consumer demand, and include cookies with low fat content, added vitamins and minerals, with fiber, without gluten, are organic, lactose-free, and sugar-free, among others (28).
Likewise, it is important to point out that there is the consumption of beans, which emerges as a protective factor and their presence can counterbalance the harmful effects of some foods, in the case found here, filled cookies, candies and treats. Thus, the highest food frequencies, according to the 2008–2009 Family Budget Survey (33) and the 2017–2018 Family Budget Survey (34), which include rice and beans, show that Brazilians maintain a dietary pattern based on these two foods. Similar results on the consumption of beans as a marker of healthy eating in the Brazilian adult population were observed in previous population surveys (39, 40).
Another limitation attributed to the instrument used is that, in addition to not investigating quantity consumed, it does not investigate habituality of consumption, restricting itself to consumption from the previous day, which may prevent detecting other findings relating to the effects of different food groups on the BMI and the incidence of obesity. There is a need to delve deeper into the unexpected occurrences detected here, taking into account the limitations highlighted, in order to have a complete picture of the factors influencing BMI and the incidence of obesity. Although a quantitative investigation within the instrument's proposal seems unfeasible, it is necessary to think about possible strategies in order to improve the quality of research results that use this instrument.
From another perspective, Lourenço et al. (41) characterized the internal structure of the SISVAN form and analyzed the evidence of measurement invariance between Brazilian macro-regions, stages of life and over time. In this way, the authors clarify that the internal structure of the form adequately reflects its conceptual basis, with the stability of factors related to healthy and unhealthy eating in configuration, loads and scale in the sections investigated, enhancing its use in research in the SUS.
Given the results found, the coverage of the population-level dimension that assesses the markers related to healthy or unhealthy nutrition is highlighted, and enables food and nutritional monitoring and vigilance (42). The Food Guide for the Brazilian Population (6) provides information and recommendations on foods, meals and eating practices, based on the NOVA Classification, encouraging the intake of fresh and minimally processed foods.
This study has strengths and some limitations. Regarding its strengths, SISVAN represents the main information system at the interface with Food and Nutrition Security, being part of the third guideline of the National Food and Nutrition Policy (42). It is worth highlighting its reliability as a Primary Health Care information system and a strong predictor of trends in the analysis of health conditions and the food and nutritional situation in Brazil. It also serves as a management tool for health professionals and managers, improving the organization and care of nutritional care (1, 17).
SISVAN has been improved and updated over the years, improving its coverage and data quality, favoring early diagnosis, decision-making and the formulation and/or reformulation of public policies and health promotion actions in the APS, aimed at the country's food and nutritional needs (9, 22). It is important to highlight that the information present in this study's database is national data from basic health units in Brazil, which were collected using a standardized instrument recommended by the Ministry of Health (MS). Furthermore, the questions are objective and clear, and easy to apply by Primary Health Care professionals (41).
Among the limitations of this study, it is important to note the need for caution when generalizing the findings to the population of Brazilian women as a whole, since the sample represented by the SISVAN user population has peculiar characteristics, closer to the population benefiting from social programs. In addition, it is mentioned that the marker form is subject to recall biases or influenced by the fact that the interviewees are aware of socially accepted behaviors. There may also be failures in obtaining the data. Another limitation of the study lies in eating habits, especially when the previous day's consumption may be atypical, such as after holidays and weekends, where the eating routine is usually null. Therefore, the use of secondary data from SISVAN, which in turn comes from different sources, does not rule out the possibility of underreporting bias or errors in collection, filling in and typing in information management.
There was an increase in BMI in the population of adult women, rising nationally in the five national macro-regions in Brazil, between 2015 and 2019. The influence of diet on this evolution was noted. There was less variation in BMI among women who reported consuming beans, vegetables and/or legumes. The incidence of obesity was lower among those who consumed beans and filled cookies, candies or treats, and higher among those who consumed sweetened drinks, instant noodles, packaged snacks or savory biscuits.
Thus, our findings highlight SISVAN as a tool that facilitates the operationalization of a large database for Food and Nutrition Surveillance in Primary Health Care, and can support the implementation of similar studies, despite the various challenges reported in the literature over the years for its use, such as supply, use and coverage. Even so, the system has advanced, both in terms of its coverage, data quality and the formulation of policies and programs using its data. Finally, the need for more studies using data from this system on the nutritional status and food consumption of women treated in PHC in Brazil is highlighted.
The original contributions presented in the study are included in the article/supplementary material, further inquiries can be directed to the corresponding author.
AB: Formal analysis, Writing – original draft, Writing – review & editing. IA: Formal analysis, Writing – review & editing. LA: Formal analysis, Writing – original draft. AC: Conceptualization, Supervision, Writing – original draft, Writing – review & editing. DM: Formal analysis, Writing – original draft. HS: Conceptualization, Supervision, Writing – original draft, Writing – review & editing.
The author(s) declare financial support was received for the research, authorship, and/or publication of this article. The study was funded by two Brazilian government grants: the Call to Action CNPq/MS/SAPS/DEPROS N° 27/2020—Research in Non-communicable Diseases and Risk Factors Associated AXIS I—EFFECTIVE STRATEGIES IN PRIMARY HEALTH CARE item 1.3.3—Obesity and Field of Study 5—Intervention studies to evaluate nutritional strategies based on the Food Guide for the Brazilian Population; process 442198/2020-5, and also by the call for proposals MS-SCTIE-Decit/CNPq n° 26/2019 from CNPq, of the Decit/SCTIE/MS and the General Coordinating Committee of Food and Nutritional Health Promotion of the Primary Health Care Executive Secretariat of the Ministry of Health.
To CAPES for the scholarship granted.
The authors declare that the research was conducted in the absence of any commercial or financial relationships that could be construed as a potential conflict of interest.
All claims expressed in this article are solely those of the authors and do not necessarily represent those of their affiliated organizations, or those of the publisher, the editors and the reviewers. Any product that may be evaluated in this article, or claim that may be made by its manufacturer, is not guaranteed or endorsed by the publisher.
1. Brasil. Ministério da Saúde. Portaria N° 1.156 de 31 de agosto de 1990: fica instituido o Sistema de vigilância alimentar e nutricional—SISVAN. Brasília: Diário oficial da União (1990).
2. Brasil. Ministério da Saúde. Secretaria de Atenção à Saúde. Departamento de Atenção Básica. Marco de referência da vigilância alimentar e nutricional na atenção básica. Brasília: Ministério da Saúde (2015). Available at: https://bvsms.saude.gov.br/bvs/publicacoes/marco_referencia_vigilancia_alimentar.pdf (accessed January 25, 2024).
3. Campos DSL, Fonseca PC. A vigilância alimentar e nutricional em 20 anos da Política Nacional de Alimentação e Nutrição. Cad Saúde Pública. (2021) 37:8–14. doi: 10.1590/0102-311x00045821
4. World Health Organization. Physical Status: the Use and Interpretation of Anthropometry. Geneva: WHO (1995).
5. Wu Y, Li D, Vermund SH. Advantages and limitations of the body mass index (BMI) to assess adult obesity. Int J Environ Res Public Health. (2024) 21:757. doi: 10.3390/ijerph21060757
6. Brasil. Ministério da Saúde. Secretaria de Atenção à Saúde. Departamento de Atenção Básica. Guia alimentar para a população brasileira. Brasília: Ministério da Saúde (2014). Available at: https://bvsms.saude.gov.br/bvs/publicacoes/guia_alimentar_populacao_brasileira_2ed.pdf (accessed January 25, 2024).
7. Popkin BM, Ng SW. The nutrition transition to a stage of high obesity and noncommunicable disease prevalence dominated by ultra-processed foods is not inevitable. Obes Rev. (2022) 23:1–18. doi: 10.1111/obr.13366
8. Conde WL, Silva IV, Ferraz FR. Undernutrition and obesity trends in Brazilian adults from 1975 to 2019 and its associated factors. Cad Saude Publica. (2022) 38:1–11. doi: 10.1590/0102-311xe00149721
9. Silva RPC, Vergara CMAC, Sampaio HAC, Vasconcelos Filho JE, Strozberg F, Ferreira Neto JFR, et al. Sistema de Vigilância Alimentar e Nutricional: tendência temporal da cobertura e estado nutricional de adultos registrados, 2008-2019. Epidemiologia e Serviços de Saúde. (2022) 31:1–13. doi: 10.1590/s1679-49742022000100019
10. Barbosa BB, Baltar VT, Horta RL, Lobato JCP, Vieira LJES, Gallo CO, et al. Food and Nutrition Surveillance System (SISVAN) coverage, nutritional status of older adults and its relationship with social inequalities in Brazil, 2008-2019: an ecological time-series study. Epidemiol Serv Saude. (2023) 32:1–16. doi: 10.1590/s2237-96222023000100034
11. Aguiar IWO, Carioca AAF, Barbosa BB, Adriano LS, Barros AQS, Kendall C, et al. Anthropometric indicators in traditional peoples and communities in Brazil: analysis of individual records from the Food and Nutrition Surveillance System, 2019. Epidemiol Serv Saude. (2023) 32:e2023543. doi: 10.1590/s2237-96222023000400005.pt
12. Fernandes RC, Merenda EKS, Antoniaconi JMV, Mandarino APG, Höfelmann DA. Sobrepeso e obesidade entre mulheres e associação com características demográficas e obstétricas entre usuárias de uma unidade de saúde especializada. Cad Saúde Coletiva. (2023) 31:1–12. doi: 10.1590/1414-462x202331010384
13. Almeida Filho N, Barreto ML. Epidemiologia & saúde: fundamentos, métodos, aplicações. Rio de Janeiro: Guanabara Koogan (2011).
16. Brasil. Portaria n° 884, de 13 de dezembro de 2011. Estabelece o fluxo para solicitação de cessão de dados dos bancos nacionais dos Sistemas de Informação. Brasília (DF): Diário Oficial da República Federativa do Brasil (2011). Available at: http://andromeda.ensp.fiocruz.br/etica/sites/default/files/documentos/PRT%20884_2011.pdf (accessed November 2, 2023).
17. Brasil. Ministério da Saúde. Secretaria de Atenção à Saúde. Departamento de Atenção Básica. Manual operacional para uso do sistema de vigilância alimentar e nutricional SISVAN—versão 3.0. Brasília: Ministério da Saúde (2017). Available at: http://sisaps.saude.gov.br/sisvan/public/file/ManualDoSisvan.pdf (accessed October 23, 2022).
18. Brasil. Ministério da Saúde. Nota técnica N° 51-SEI/2017-CGAA/DAB/SAS/MS. Brasília (DF): Diário Oficial da República Federativa do Brasil (2011). Available at: https://sisaps.saude.gov.br/esus/upload/docs/nt_51_sei_2017.pdf (accessed December 5, 2023).
19. Brasil. Ministério da Saúde. Secretaria de Vigilância em Saúde. Departamento de Análise em Saúde e Vigilância de Doenças Não Transmissíveis. Vigitel Brasil 2020: vigilância de fatores de risco e proteção para doenças crônicas por inquérito telefônico: estimativas sobre frequência e distribuição sociodemográfica de fatores de risco e proteção para doenças crônicas nas capitais dos 26 estados brasileiros e no Distrito Federal em 2020. Brasília: Ministério da Saúde (2021). Available at: https://www.gov.br/saude/pt-br/centrais-de-conteudo/publicacoes/publicacoes-svs/vigitel/relatorio-vigitel-2020-original.pdf (accessed August 25, 2023).
20. National Cancer Institute. Dietary Assessment Primer, Dietary Assessment Instrument Profiles. MD: National Institutes of Health (2023). Available at: https://dietassessmentprimer.cancer.gov/profiles/screeners/ (accessed January 5, 2024).
21. Brasil. Ministério da Saúde. Secretaria de Atenção à Saúde. Departamento de Atenção Básica. Orientações para avaliação de marcadores de consumo alimentar na atenção básica. Brasília: Ministério da Saúde (2015). Available at: https://bvsms.saude.gov.br/bvs/publicacoes/marcadores_consumo_alimentar_atencao_basica.pdf (accessed July 21, 2023).
22. Ricci JMS, Romito ALZ, Silva SA, Carioca AAF, Lourenço BH. Marcadores do consumo alimentar do Sisvan: tendência temporal da cobertura e integração com o e-SUS APS, 2015-2019. Ciência Saúde Coletiva. (2023) 28:921–34. doi: 10.1590/1413-81232023283.10552022en
23. Flores-Ortiz R, Malta DC, Velásquez-Meléndez G. Adult body weight trends in 27 urban populations of Brazil from 2006 to 2016: a population-based study. PLoS ONE. (2019) 14:1–10. doi: 10.1371/journal.pone.0213254
24. Instituto Brasileiro de Geografia e Estatística. Pesquisa nacional de saúde: 2019: atenção primária à saúde e informações antropométricas. Rio de Janeiro: IBGE (2020). Available at: https://biblioteca.ibge.gov.br/visualizacao/livros/liv101758.pdf (accessed March 2, 2024).
25. Triaca LM, Santos AMA, Tejada CA. Socioeconomic inequalities in obesity in Brazil. Econ. Hum. Biol. (2020) 39:100906. doi: 10.1016/j.ehb.2020.100906
26. Silva LA, Rodrigues CT, Braga MJ. Fatores socioeconômicos e comportamentais associados a desigualdade na obesidade de homens e mulheres no Brasil. Estudos Econômicos. (2023) 53:177–209. doi: 10.1590/1980-53575316lcm
27. Nascimento FA, Silva SA, Jaime PC. Cobertura da avaliação do estado nutricional no Sistema de Vigilância Alimentar e Nutricional brasileiro: 2008 a 2013. Cadernos de Saúde Pública. (2017) 33:1–14. doi: 10.1590/0102-311x00161516
28. Palmeira PA, Bem-Lignani J, Salles-Costa R. Acesso aos benefícios e programas governamentais e insegurança alimentar nas áreas rurais e urbanas do Nordeste brasileiro. Ciência Saúde Coletiva. (2022) 27:2583–95. doi: 10.1590/1413-81232022277.21592021
29. Rodrigues RM, Souza AM, Bezerra IN, Pereira RA, Yokoo EM, Sichieri R. Most consumed foods in Brazil: evolution between 2008–2009 and 2017–2018. Revista de Saúde Pública. (2021) 55:1–10. doi: 10.11606/s1518-8787.2021055003406
30. Costa DVDP, Lopes MS, Mendonça RD, Malta DC, Freitas PP, Lopes ACS. Diferenças no consumo alimentar nas áreas urbanas e rurais do Brasil: Pesquisa Nacional de Saúde. Cien Saude Colet. (2021) 26:3805–13. doi: 10.1590/1413-81232021269.2.26752019
31. Gombi-Vaca MF, Martinez-Steele E, Andrade GC, Louzada MLDC, Levy RB. Association between ultra-processed food and snacking behavior in Brazil. Eur J Nutr. (2024) 63:1177–86. doi: 10.1007/s00394-024-03340-y
32. Instituto Brasileiro de Geografia e Estatística. Pesquisa de Orçamentos Familiares 2002-2003. Aquisição alimentar domiciliar per capita: Brasil e Grandes Regiões. Rio de Janeiro: IBGE (2004).
33. Instituto Brasileiro de Geografia e Estatística. Pesquisa de orçamentos familiares 2008-2009: análise do consumo alimentar pessoal no Brasil. Rio de Janeiro: IBGE (2011). Available at: https://biblioteca.ibge.gov.br/index.php/biblioteca-catalogo?id=250063&view=detalhes (accessed March 2, 2024).
34. Instituto Brasileiro de Geografia e Estatística. Pesquisa de Orçamentos Familiares 2017-2018: Análise da Segurança Alimentar no Brasil. Rio de Janeiro: IBGE (2020). Available at: https://biblioteca.ibge.gov.br/visualizacao/livros/liv101749.pdf (accessed April 25, 2023).
35. Nilson EA, Ferrari G, Louzada MLC, Levy RB, Monteiro CA, Rezende LF. Premature deaths attributable to the consumption of ultraprocessed foods in Brazil. Am J Prev Med. (2023) 64:129–36. doi: 10.1016/j.amepre.2022.08.013
36. Sociedade Brasileira de Diabetes. Diretrizes da sociedade brasileira de diabetes 2019–2020. São Paulo: Clannad (2020).
37. Hall KD, Ayuketah A, Brychta R, Cai H, Cassimatis T, Chen KY, et al. Ultra-processed diets cause excess calorie intake and weight gain: an inpatient randomized controlled trial of ad libitum food intake. Cell Metab. (2019) 30:67–77.e3. doi: 10.1016/j.cmet.2019.05.008
38. Kirkpatrick SI. Enhancing the comparability of research on ultraprocessed foods. J Nutr. (2023) 153:1–2. doi: 10.1016/j.tjnut.2022.12.020
39. Jaime PC, Stopa SR, Oliveira TP, Vieira ML, Szwarcwald CL, Malta DC. Prevalência e distribuição sociodemográfica de marcadores de alimentação saudável, Pesquisa Nacional de Saúde, Brasil 2013. Epidemiol Serv Saúde. (2015) 24:267–76. doi: 10.5123/S1679-49742015000200009
40. Silveira FC, Susin LRO, Meucci RD. Marcadores de consumo alimentar em mulheres da zona rural de Rio Grande, Rio Grande do Sul, 2017. Epidemiologia e Serviços de Saúde. (2020) 29:1–15. doi: 10.5123/S1679-49742020000100023
41. Lourenço BH, Guedes BDM, Santos TSS. Sisvan food intake markers: structure and measurement invariance in Brazil. Revista de Saúde Pública. (2023) 57:1–9. doi: 10.11606/s1518-8787.2023057004896
42. Brasil. Ministério da Saúde. Secretaria de Atenção à Saúde. Departamento de Atenção Básica. Política Nacional de Alimentação e Nutrição. Brasília: Ministério da Saúde. (2013). Available at: https://bvsms.saude.gov.br/bvs/publicacoes/politica_nacional_alimentacao_nutricao.pdf (accessed January 3, 2024).
Keywords: Food and Nutrition Monitoring, health information systems, cohort studies, nutritional status, obesity
Citation: Barros AQS, Aguiar IWO, Adriano LS, Carioca AAF, Marchioni DML and Sampaio HAC (2024) Food consumption markers and sociodemographic factors associated with obesity among adult women registered in the Food and Nutrition Monitoring System (SISVAN) in Brazil: a cohort study, 2015–2019. Front. Public Health 12:1467339. doi: 10.3389/fpubh.2024.1467339
Received: 19 July 2024; Accepted: 08 October 2024;
Published: 22 October 2024.
Edited by:
Juliana Souza Oliveira, Federal University of Pernambuco, BrazilReviewed by:
Rodrigo Vianna, Federal University of Paraíba, BrazilCopyright © 2024 Barros, Aguiar, Adriano, Carioca, Marchioni and Sampaio. This is an open-access article distributed under the terms of the Creative Commons Attribution License (CC BY). The use, distribution or reproduction in other forums is permitted, provided the original author(s) and the copyright owner(s) are credited and that the original publication in this journal is cited, in accordance with accepted academic practice. No use, distribution or reproduction is permitted which does not comply with these terms.
*Correspondence: Helena Alves de Carvalho Sampaio, ZHIuaGFyZDJAZ21haWwuY29t
Disclaimer: All claims expressed in this article are solely those of the authors and do not necessarily represent those of their affiliated organizations, or those of the publisher, the editors and the reviewers. Any product that may be evaluated in this article or claim that may be made by its manufacturer is not guaranteed or endorsed by the publisher.
Research integrity at Frontiers
Learn more about the work of our research integrity team to safeguard the quality of each article we publish.