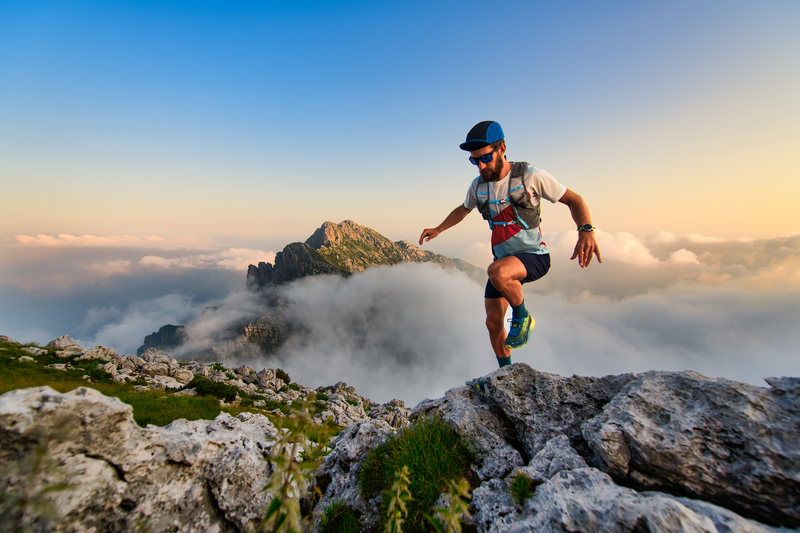
94% of researchers rate our articles as excellent or good
Learn more about the work of our research integrity team to safeguard the quality of each article we publish.
Find out more
MINI REVIEW article
Front. Public Health , 23 October 2024
Sec. Environmental Health and Exposome
Volume 12 - 2024 | https://doi.org/10.3389/fpubh.2024.1467121
The key role of our microbiome in influencing our health status, and its relationship with our environment and lifestyle or health behaviors, have been shown in the last decades. Therefore, the human microbiome has the potential to act as a biomarker or indicator of health or exposure to health risks in the general population, if information on the microbiome can be collected in population-based health surveys or cohorts. It could then be associated with epidemiological participant data such as demographic, clinical or exposure profiles. However, to our knowledge, microbiome sampling has not yet been included as biological evidence of health or exposure to health risks in large population-based studies representative of the general population. In this mini-review, we first highlight some practical considerations for microbiome sampling and analysis that need to be considered in the context of a population study. We then present some examples of topics where the microbiome could be included as biological evidence in population-based health studies for the benefit of public health, and how this could be developed in the future. In doing so, we aim to highlight the benefits of having microbiome data available at the level of the general population, combined with epidemiological data from health surveys, and hence how microbiological data could be used in the future to assess human health. We also stress the challenges that remain to be overcome to allow the use of this microbiome data in order to improve proactive public health policies.
In recent years, several large studies have been carried out sequencing thousands of human genomes (1, 2). However, it is now known that humans are holobionts, or meta-organisms, living in the presence of thousands of microbes on different parts of the body, such as the gut, vagina, skin, mouth and nose (3, 4), constituting a second genome (5). These organisms have a symbiotic interaction with our bodies and play an important role in our health from birth, for example by programming our immune system, providing nutrients for cells and preventing colonization by pathogens (6). Over the past decade, alterations in the microbiome have been associated with the occurrence of a plethora of diseases and conditions, including diabetes, obesity, inflammatory bowel disease, autism and mental health (7, 8). Microbiota transplantation is now even being used as a validated treatment for recurrent Clostridium difficile infections (9). However, the causal effects of the microbiome on health outcomes, or vice versa, are still not fully documented or understood (10–12). Studies have shown that the microbiome, which is even thought to be initiated in the prenatal state (13), is also shaped by exposures throughout our lives (i.e., our exposome). Our microbial diversity begins to expand after the introduction of solid foods and then continues to vary according to health behavior (14, 15), including the diet, stress or activity levels (16), antibiotic use (17), but also our environment (18, 19), including exposure to pollution and environmental contaminants (20, 21) and the biodiversity in the surrounding landscape (22). Infection by a pathogen (23) or non-communicable diseases have also been associated with changes in the microbiome, e.g., metabolic syndrome (24), cancer (25) or pulmonary damage after pollution exposure (26). In addition, the microbiome could predict the severity of the infection, as reported for COVID-19 (27–29). The microbiome has also been shown to indicate how individuals respond to certain drugs, e.g., how cancer patients respond to chemotherapy (30). These recent data suggest that microbiome analysis, when combined with epidemiological data, has the potential to provide valuable information for assessing population health. Furthermore, as the microbiome is influenced by the exposome, the microbiome could also be used to assess environmental exposures. Therefore, combining the determination of the microbiome with population-based epidemiological data (e.g., from national health examination surveys, food consumption surveys or national cohorts) would make it possible to obtain information on the general health status of the population as well as the impact of environmental and health behavior parameters (exposome) on this health or to identify population groups at risk for specific diseases or health problems through biological sampling (Figure 1) (31, 32). Hence, through further research combining epidemiological and microbiome evaluations, microbiome data has the potential to be used as biomarker, i.e., a kind of proxy or measurable indicator of health and exposure determinants in the general population. This would provide relevant authorities with important insights to drive a proactive public health policy (Figure 1).
Figure 1. The pivotal place of the microbiome in population-based health studies. Biological data from non-invasive microbiome (gut, oral, nasal, skin…) testing performed at population-scale, can be associated with information from health, epidemiological surveys and human biomonitoring, with information on environmental and health behavior parameters (exposome, e.g., diet, pollution, stress….) affecting the population through life as well as the prevalence or development of health conditions (e.g., body composition, allergies, diseases, mental health…). After (bio)informatic and statistic processing, this population-level data could be used to drive proactive public health policy by giving information on the health status of the population (and therefore monitor the impact of new policies to improve health) or to identify population groups at risk for specific diseases or health problems and by allowing information-based policy-making on the environment and monitoring of the mitigation and adaptation actions put in place.
In this paper, we discuss various practical considerations and benefits of including the microbiome in population-based health studies. We present the potential samples that can be collected to analyze the human microbiomes and how this sampling can be standardized for large-scale public health studies. We describe the different technologies now available to analyze the microbiome of these samples and how this analysis will affect the information that can be obtained from the study. We then discuss how this approach can be implemented in population-based surveys and cohorts, using examples of studies that could benefit from the addition of the study of the microorganisms that live in and on us. Finally, we highlight the opportunities and challenges of including the microbiome in such population-based health studies.
The purpose of the research will determine the type of biological sample. The skin or vaginal microbiome may not answer the same biological questions as the microbiome from the nose, mouth or gut. Therefore, the specific microbiome community to be studied must be carefully chosen. Gut and oral microbiomes are very diverse and have been most studied in relation to diseases and environmental exposures. They are therefore promising options for use in population-based health studies. The collection of feces (i.e., stool) to study the gut microbiome is non-invasive, allows self-sampling and has therefore been documented in large-scale studies (33, 34). The oral cavity has been described as the second largest human environment after the gut in terms of diversity and abundance of microorganisms (35). Samples of the oral microbiome are also easy to collect and preserve, although the exact sample type or location (saliva, tongue swab, biofilm at tooth surface, subgingival plaque…) may give different results (36). Saliva is the easiest to collect by the participants without external intervention and offers the possibility to also investigate the human genome or epigenome (37). Finally, nasal or skin swabs have been shown to be relevant when investigating environmental parameters such as air pollution or greenness and biodiversity levels of the surrounding landscape (38). The lung microbiome can also be of interest when studying respiratory diseases and exposure to air pollution (39–41), although the sampling collection methods are commonly invasive. The collection of sputum is the least invasive sampling of the lung microbiome, although the microbiome obtained is inheritably contaminated by the oral microbiome, and therefore does not represent the ‘pure’ respiratory microbiome. Furthermore, healthy participants may not have sufficient phlegm, which can hinder sampling (42, 43). Once the sample type has been selected, the sampling method (e.g., self-sampling or interviewer-led) needs to be determined. To conduct large-scale studies, self-sampling, preferably without the need for the presence of a health practitioner, as well as the existence of a standardized sampling protocol, will be crucial to limit bias, as has been extensively reviewed (44, 45). This standardization includes the collection [e.g., for nasal swabs, the depth (46)], the timing [e.g., first feces of the day (47)] as the microbiome is affected by circadian changes (48), the collection tubes and the transport and storage conditions (44, 45). Importantly, in Europe, in the context of the General Data Protection Regulation (GDPR), each donor must be (pseudo-)anonymized and the samples must be carefully stored in a biobank (49, 50) and linked to the donor consent. Moreover, although studies have reported relationships between the microbiome and human genome markers, making a combined collection interesting (51), this may raise some ethical issues. Notwithstanding, for GDPR reasons, it is common practice to remove all human genetic data from publicly stored microbiome data to avoid re-identification of the donor.
Until recently, microorganisms have mainly been studied in isolation after being cultured on plates. This is a tedious task for complex environments and communities, and not all organisms living in the human microbiomes can be cultured (52), including viruses. In recent decades, new culture-independent methods have been developed that allow a better understanding of the diversity of the human microbiomes in a single test. These methods are based on sequencing the nucleic acids (mostly DNA) extracted from the microbiome sample. The quality, abundance and unbiased representation of the microbes in the genetic material extract is important to consider for standardization and data comparison (53).
The most widely used method of microbiome analysis is metabarcoding or sequencing of the 16S rRNA subunit gene, a highly conserved gene shared by bacteria and archaea, but presenting hypervariable regions that allow one bacterium to be distinguished from another. Two methods can be used, based on a selection of hypervariable regions of the gene (V1 to V9, of which V3-V4 are the most frequently selected), which may differ in resolution, or based on the sequencing of the entire gene. However, when only regions of the gene are sequenced, it has been reported that this differentiation is limited to accurately determine the genus level for most species (54, 55), whereas the species or strain level may be required to determine accurate biomarkers from the microbiome (56). The sequencing of the entire gene may improve species level identification but still only allows taxonomic classification and not functional analysis (55). Importantly, the use of different hypervariable regions leads to different results (44, 57), which may also bias conclusions from meta-analyses (58). Moreover, this method does not report the presence of fungi (which could be analyzed by the ITS region), eukaryotes (which could be analyzed using the 18S rRNA subunit), but also viruses, which are not targeted by any metabarcoding method (59).
An alternative method that has been developed is shotgun metagenomics or the sequencing of all nucleic acids in the sample, which theoretically allows the entire genome of all the species in the sample to be obtained, including non-bacterial species (60). This includes the information on the different taxa present at the strain level (61), but also allows the functional analysis through the genes present in each genome. In particular, the presence of antimicrobial resistance genes can also be analyzed which is of particular importance for public health. Recently, a team of scientists even defined statistically relevant associations of the donor body mass index at SNP (single-nucleotide polymorphism) level, which is only possible when the entire genome of each microorganism in the sample is sequenced (56). As this method is still expensive, it could be used in a public health setting for pioneering studies to define markers to look for, but may not be feasible for a whole cohort or population, depending on the budget available. This method also allows access to the sequence of the genetic material from the donor as well, which could be of interest for some studies targeting the human DNA, making it more cost-effective. If biomarkers are defined at species, gene or SNP level after sequencing, more targeted methods can be used to test for them (62), providing a much faster and less expensive alternative. However, target-based methods could not be used when the microbiome change of interest is a variation in diversity, richness or evenness, requiring a view of the entire microbiome at once.
Finally, promising alternative methods are being developed. For example, optical mapping, which is based on the examination of fluorocoding labels on the DNA with a high-resolution microscope to visualize structural variations within a microbial genome, allows species identification at a lower cost and in a shorter time frame (63). However, it does not provide information on the gene content. Specific genetic markers can be detected at a lower cost without the need for shotgun sequencing using hybridization capture sequencing (64), but the gene cannot be linked to the genome and species harboring it. Depending on the scientific question to be answered, multiple microbiome characterization methods could be coupled together to provide a complete view of the samples and limit the bias of each individual method (65). Alternatively, RNA sequencing using metatranscriptomics may also be of interest to study RNA viruses, which have also been reported to be present in microbiomes (66). It is important to note that the handling of the sample, storage, DNA extraction, library preparation, sequencing and data analysis all introduce bias. Therefore, regardless of the method chosen, in addition to including appropriate controls during the analysis (45), the data must be correctly stored in a database with accurate annotation and metadata to allow further analysis and comparison between different studies or approaches (67, 68). Furthermore, as reviewed elsewhere (45), microbiome analysis requires appropriate bioinformatics and biostatistics.
As discussed in the introduction, non-population-based studies have revealed various associations between the microbiome and various aspects of health (as reflected in Table 1), and between environmental exposures and microbial composition, diversity, and function (Table 1), although the biomarkers that have been described are not yet validated at the whole population level. Conducting microbiome studies at the population level offers additional advantages, including broader representation (general population), longitudinal analysis (temporal patterns, causal relationships, i.e., cohorts), large-scale data (higher reliability), and translational impact (actionable insights). Once biomarkers can be clearly defined, by integrating the biological measures from the microbiome data that can be correlated with health disturbance, with the data typically collected during population-based health studies (questionnaires, chemical exposure), we could assess the population’s health status and the impact of environmental and health behavior parameters on that health (Figure 1). This has the potential to improve our understanding of disease etiology, risk factors, and prevention strategies, ultimately contributing to improved population health outcomes through information-based policy making (Figure 1; Table 1).
Table 1. Examples of scientific publications focused on various exposures, including microbiome samples of smaller populations and their main observations, as biological evidence obtained from the microbiome, that could be implemented in future population-based health studies in support of policy when biomarkers are clearly defined.
To date, several hundred thousand microbial genomes have been reported in the human microbiomes, originating from over 5,000 different species (74), of which an estimated 50% is still undefined (75). In addition, a high degree of inter-individual variation has been observed (76). Therefore, the first step to enable population-based microbiome studies is to obtain an image of the general population’s microbiome by sampling the general public, without specific focus on certain (known) diseases, and thus representing a more or less “healthy” microbiome. This makes it possible to identify and study the diversity of species present in the microbiome of the country. Such projects are currently underway in several countries or regions (34, 77, 78) These projects are all based on convenience sampling with no random selection of participants from the “healthy” population (i.e., participation in these studies was at participant’s own initiative) and are therefore not representative of the general, national population. These samplings need to be conducted randomly at all levels of the population that is studied to cover not only different genders, ages and diets but also the diversity of socioeconomic status, ethnicities, childhood conditions or social behaviors (79). Indeed, most studies have tested a particularly high proportion of highly educated males (79), while it has previously been shown that the lack of representation of certain minority groups within the subjects tested can bias the interpretation of the results when applied to a more diverse group (80), as has been the case with the human genome (81). Including microbiome sampling in national health studies would allow to obtain information which is more representative of the general population, although some bias may remain due to non-response of some invited participants. Ensuring the representation of minorities among the participants could be attempted by oversampling of usually underrepresented groups, replacing non-respondents with people belonging to the same category, offering incentives (e.g., vouchers) or by adapting communication strategies (e.g., adapted study material, multiple languages, simplified languages, pre-testing of the communication material in a minority group) in order to ensure acceptability for specific minority groups that usually show a lower positive response to studies (82, 83). In addition, downstream analytical methods (e.g., weighting) can be used to aim for results as representative as possible.
In previous studies (Table 1) alterations in the microbiomes have been associated with several parameters that could be measured during population-based health studies, such as diet, mental health or exposure to pollution. Population-based health studies cover demographic, clinical and/or exposure data. When combined with microbiome analysis, it would allow to gather biological evidence for a variety of factors in the population. Sampling of the microbiome could be included in ongoing population-based health studies such as food consumption surveys, human biomonitoring studies related to environmental chemical exposure (measurement of chemical concentrations in human samples), mental health assessments or projects related to climate change and environmental biodiversity alterations. Associations of this type of data with the microbiome have previously been reported for smaller population groups (Table 1). However, although it is generally assumed that microbiome shifts are observed in association with various lifestyle or health parameters, the causality link is often not established (10–12), and different studies may report contradicting biomarkers. The study of larger groups at different time points may address this issue (11, 84), as suggested by collaborations with national population-based health studies.
Through population-based health surveys, microbiome data could be associated with donor characteristics such as smoking status, region of residence and hence pollution data, diet or hygiene (Figure 1). Artificial intelligence, particularly machine learning and deep learning approaches, has been described as an interesting new tool for predicting health or exposure status (75, 85), but the population size needs to be sufficient to draw robust conclusions (86). Such associations could provide insights for public health policy and guidelines recommendations (Figure 1; Table 1). However, spurious associations (confounding effects) may be obtained if insufficient donor characteristics are collected. It is therefore important to collect sufficient donor information to limit this effect. Gender, age and body mass index have been shown to be important to include, as well as other specific factors depending on the type of microbiome being studied (87). Nevertheless, as unobserved data can also lead to these spurious associations, specific analysis methods that are available in the field of causal inference should be used to try to account for this.
National public health institutes can also sometimes have access to population cohorts for their studies. These are representative groups of people who are followed up year after year for environmental and health-related aspects. This allows longitudinal studies to be conducted on a random panel of the population. Longitudinal studies of cohorts have already been conducted to understand the evolution of the microbiome over time, particularly in the first years of life (3, 88, 89). Studying the microbiome of cohorts over time would also make it possible to uncover, at individual level (since the healthy microbiome varies greatly from person to person), but on a large scale, the dynamics related to the development and progression of diseases, the impact of pre-and post-exposure to pollution, the association with climate change as well as to observe at the biological and physiological level the impact of the implementation of new public health policies aimed at mitigating and adapting to certain adverse health and/or environmental impacts (e.g., air pollution, chemical exposure, loss of biodiversity).
Finally, other types of epidemiological data collection for microbiomes could be envisaged, beyond population surveys or cohorts. Indeed, human biomarkers can be studied at the population level in non-human samples such as sewage (90). For example, the gut microbiome detected in wastewater has previously been linked to obesity rates in the population (91). Taking advantage of the revised European Wastewater Treatment Directive (91/271/EEC), which requires the establishment of national wastewater-based surveillance by 2026, analyzing the sewage also through the lens of the microbiome it contains, would be an interesting, anonymized and non-invasive way to gather large-scale information at the population level and should be further investigated for its association with various exposures or health problems.
The human microbiome has been studied for over a century and new high-throughput technologies have recently allowed to unravel an impact of environmental factors and medical conditions on these microbial communities but also hint to their influence on our health and well-being. However, such studies are not yet commonly conducted at the population level. Therefore, the integration of this biological data into health information systems has the potential to first define a representative microbiome of the general (“healthy”) population, and then, based on further research, to establish microbiome-based biomarkers that can be associated with exposures, health or population groups at risk. However, the integration of microbiome data into population-based health studies is complex and there are challenges to be overcome for the success of such large-scale studies, some of which have already been mentioned above such as standardization and the inclusion of minority groups. Another challenge is the high cost of advanced analytical methods. Although sequencing of the entire genetic material of a microbiome (shotgun metagenomics) would be ideal for higher accuracy and comparability of the taxonomic classification and even SNP-level information, metabarcoding is more cost-effective for a general idea of the species present in a sample. However, once biomarkers can be confirmed at the population level, some studies may only need to analyze a subset of species or genes using less advanced methods, further reducing costs. Proper data management will also be critical. Microbiome information will need to be collected anonymously in databases, along with the relevant additional donor characteristics. This should be harmonized within and between studies to avoid bias or errors in downstream analysis and interpretation. Subsequently, (bio) informatics tools will need to be developed, possibly using artificial intelligence-based methods to mine the data, to produce informed reports based on biological data, human biomonitoring, the exposome (including health behavior parameters) and population health to guide new policies. As discussed elsewhere (45), this will also require training of public health stakeholders in the interpretation of microbiome-based data.
Particularly at a time when various mitigation and adaptation actions measures are being implemented to reverse or cope with some of the detrimental anthropogenic environmental impacts of the past, microbiome data would eventually provide competent authorities with informed data on the whole population to monitor and evaluate the impact of these implemented actions. Therefore, including microbiome characterization in population-based studies is meaningful to further our knowledge of how microbiological data could be used in the future to assess health outcomes and environmental exposures.
FB: Writing – review & editing, Writing – original draft, Visualization, Methodology, Conceptualization. NB: Writing – review & editing, Methodology. JH: Writing – review & editing, Methodology. NR: Writing – review & editing, Methodology. SDK: Writing – review & editing, Writing – original draft, Visualization, Supervision, Project administration, Methodology, Funding acquisition, Conceptualization.
The author(s) declare that financial support was received for the research, authorship, and/or publication of this article. The research was funded by Sciensano, Belgium through contract METAMORPHOSE.
We acknowledge the members of the METAMORPHOSE project team. We would like to thank the reviewers for taking the time and effort necessary to review the manuscript. We sincerely appreciate all valuable comments and suggestions, which helped us to improve the quality of the manuscript.
The authors declare that the research was conducted in the absence of any commercial or financial relationships that could be construed as a potential conflict of interest.
All claims expressed in this article are solely those of the authors and do not necessarily represent those of their affiliated organizations, or those of the publisher, the editors and the reviewers. Any product that may be evaluated in this article, or claim that may be made by its manufacturer, is not guaranteed or endorsed by the publisher.
1. Auton, A, Brooks, LD, Durbin, RM, Garrison, EP, Kang, HM, Korbel, JO, et al. A global reference for human genetic variation. Nature. (2015) 526:68–74. doi: 10.1038/nature15393
2. Saunders, G, Baudis, M, Becker, R, Beltran, S, Béroud, C, Birney, E, et al. Leveraging European infrastructures to access 1 million human genomes by 2022. Nat Rev Genet. (2019) 20:693–701. doi: 10.1038/s41576-019-0156-9
3. Biagi, E, Candela, M, Fairweather-Tait, S, Franceschi, C, and Brigidi, P. Ageing of the human metaorganism: the microbial counterpart. Age. (2012) 34:247–67. doi: 10.1007/s11357-011-9217-5
4. Turnbaugh, PJ, Ley, RE, Hamady, M, Fraser-liggett, C, Knight, R, and Gordon, JI. The human microbiome project: exploring the microbial part of ourselves in a changing world. Nature. (2007) 449:804–10. doi: 10.1038/nature06244
5. Grice, EA, and Segre, JA. The human microbiome: our second genome. Annu Rev Genom Hum Genet. (2012) 13:151–70. doi: 10.1146/annurev-genom-090711-163814
6. Ogunrinola, GA, Oyewale, JO, Oshamika, OO, and Olasehinde, GI. The human microbiome and its impacts on health. Int J Microbiol. (2020) 2020:1–7. doi: 10.1155/2020/8045646
7. El-Sayed, A, Aleya, L, and Kamel, M. Microbiota’s role in health and diseases. Environ Sci Pollut Res. (2021) 28:36967–83. doi: 10.1007/s11356-021-14593-z
8. Durack, J, and Lynch, SV. The gut microbiome: relationships with disease and opportunities for therapy. J Exp Med. (2019) 216:20–40. doi: 10.1084/jem.20180448
9. Rohlke, F, and Stollman, N. Fecal microbiota transplantation in relapsing Clostridium difficile infection. Ther Adv Gastroenterol. (2012) 5:403–20. doi: 10.1177/1756283X12453637
10. Walter, J, Armet, AM, Finlay, BB, and Shanahan, F. Establishing or exaggerating causality for the Gut microbiome: lessons from human microbiota-associated rodents. Cell. (2020) 180:221–32. doi: 10.1016/j.cell.2019.12.025
11. Hooks, KB, and O’Malley, MA. Dysbiosis and its discontents. MBio. (2017) 8:e01492–17. doi: 10.1128/mBio.01492-17
12. Lynch, KE, Parke, EC, and O’Malley, MA. How causal are microbiomes? A comparison with the Helicobacter pylori explanation of ulcers. Biol Philos. (2019) 34:62. doi: 10.1007/s10539-019-9702-2
13. Walker, RW, Clemente, JC, Peter, I, and Loos, RJF. The prenatal gut microbiome: are we colonized with bacteria in utero? Pediatr Obes. (2017) 12:3–17. doi: 10.1111/ijpo.12217
14. Chen, J, Siliceo, SL, Ni, Y, Nielsen, HB, Xu, A, and Panagiotou, G. Identification of robust and generalizable biomarkers for microbiome-based stratification in lifestyle interventions. Microbiome. (2023) 11:178. doi: 10.1186/s40168-023-01604-z
15. Manor, O, Dai, CL, Kornilov, SA, Smith, B, Price, ND, Lovejoy, JC, et al. Health and disease markers correlate with gut microbiome composition across thousands of people. Nat Commun. (2020) 11:5206. doi: 10.1038/s41467-020-18871-1
16. Hantsoo, L, and Zemel, BS. Stress gets into the belly: early life stress and the gut microbiome. Behav Brain Res. (2021) 414:113474. doi: 10.1016/j.bbr.2021.113474
17. Patangia, DV, Anthony Ryan, C, Dempsey, E, Paul Ross, R, and Stanton, C. Impact of antibiotics on the human microbiome and consequences for host health. MicrobiologyOpen. (2022) 11:e1260. doi: 10.1002/mbo3.1260
18. Hanson, BM, and Weinstock, GM. The importance of the microbiome in epidemiologic research. Ann Epidemiol. (2016) 26:301–5. doi: 10.1016/j.annepidem.2016.03.008
19. Chong-Neto, HJ, D’amato, G, and Rosário Filho, NA. Impact of the environment on the microbiome. J Pediatr. (2022) 98:S32–7. doi: 10.1016/j.jped.2021.10.001
20. Thompson, KN, Oulhote, Y, Weihe, P, Wilkinson, JE, Ma, S, Zhong, H, et al. Effects of lifetime exposures to environmental contaminants on the adult Gut microbiome. Environ Sci Technol. (2022) 56:16985–95. doi: 10.1021/acs.est.2c03185
21. Ozdemir, C, Kucuksezer, UC, Ogulur, I, Pat, Y, Yazici, D, Ardicli, S, et al. Lifestyle changes and industrialization in the development of allergic diseases. Curr Allergy Asthma Rep. (2024) 24:331–45. doi: 10.1007/s11882-024-01149-7
22. Hanski, I, von Hertzen, L, Fyhrquist, N, Koskinen, K, Torppa, K, Laatikainen, T, et al. Environmental biodiversity, human microbiota, and allergy are interrelated. Proc Natl Acad Sci USA. (2012) 109:8334–9. doi: 10.1073/pnas.1205624109
23. Wang, B, Zhang, L, Wang, Y, Dai, T, Qin, Z, Zhou, F, et al. Alterations in microbiota of patients with COVID-19: potential mechanisms and therapeutic interventions. Sig Transduct Target Ther. (2022) 7:143. doi: 10.1038/s41392-022-00986-0
24. Metwaly, A, Reitmeier, S, and Haller, D. Microbiome risk profiles as biomarkers for inflammatory and metabolic disorders. Nat Rev Gastroenterol Hepatol. (2022) 19:383–97. doi: 10.1038/s41575-022-00581-2
25. Zwezerijnen-Jiwa, FH, Sivov, H, Paizs, P, Zafeiropoulou, K, and Kinross, J. A systematic review of microbiome-derived biomarkers for early colorectal cancer detection. Neoplasia. (2023) 36:100868. doi: 10.1016/j.neo.2022.100868
26. Zhang, J, Cheng, H, di Narzo, A, Zhu, Y, Xie, S, Shao, X, et al. Profiling microbiota from multiple sites in the respiratory tract to identify a biomarker for PM 2.5 nitrate exposure-induced pulmonary damages. Environ Sci Technol. (2023) 57:7346–57. doi: 10.1021/acs.est.2c08807
27. Lymberopoulos, E, Gentili, GI, Budhdeo, S, and Sharma, N. COVID-19 severity is associated with population-level gut microbiome variations. Front Cell Infect Microbiol. (2022) 12:1–17. doi: 10.3389/fcimb.2022.963338
28. Yeoh, YK, Zuo, T, Lui, GCY, Zhang, F, Liu, Q, Li, AYL, et al. Gut microbiota composition reflects disease severity and dysfunctional immune responses in patients with COVID-19. Gut. (2021) 70:698–706. doi: 10.1136/gutjnl-2020-323020
29. Soffritti, I, D’Accolti, M, Fabbri, C, Passaro, A, Manfredini, R, Zuliani, G, et al. Oral microbiome Dysbiosis is associated with symptoms severity and local immune/inflammatory response in COVID-19 patients: a cross-sectional study. Front Microbiol. (2021) 12:1–15. doi: 10.3389/fmicb.2021.687513
30. Alexander, JL, Wilson, ID, Teare, J, Marchesi, JR, Nicholson, JK, and Kinross, JM. Gut microbiota modulation of chemotherapy efficacy and toxicity. Nat Rev Gastroenterol Hepatol. (2017) 14:356–65. doi: 10.1038/nrgastro.2017.20
31. Kho, ZY, and Lal, SK. The human Gut microbiome – a potential controller of wellness and disease. Front Microbiol. (2018) 9:1–23. doi: 10.3389/fmicb.2018.01835
32. Trinh, P, Zaneveld, JR, Safranek, S, and Rabinowitz, PM. One health relationships between human, animal, and environmental microbiomes: a Mini-review. Front Public Health. (2018) 6:235. doi: 10.3389/fpubh.2018.00235
33. Vandeputte, D, Tito, RY, Vanleeuwen, R, Falony, G, and Raes, J. Practical considerations for large-scale gut microbiome studies. FEMS Microbiol Rev. (2017) 41:S154–67. doi: 10.1093/femsre/fux027
34. Falony, G, Joossens, M, Vieira-Silva, S, Wang, J, Darzi, Y, Faust, K, et al. Population-level analysis of gut microbiome variation. Science. (2016) 352:560–4. doi: 10.1126/science.aad3503
35. Deo, PN, and Deshmukh, R. Oral microbiome: unveiling the fundamentals. J Oral Maxillofac Pathol. (2019) 23:122–8. doi: 10.4103/jomfp.JOMFP_304_18
36. Lu, H, Zou, P, Zhang, Y, Zhang, Q, Chen, Z, and Chen, F. The sampling strategy of oral microbiome. iMeta. (2022) 1:e23. doi: 10.1002/imt2.23
37. Herzig, AF, Velo-Suárez, L, le Folgoc, G, Boland, A, Blanché, H, Olaso, R, et al. Evaluation of saliva as a source of accurate whole-genome and microbiome sequencing data. Genet Epidemiol. (2021) 45:537–48. doi: 10.1002/gepi.22386
38. Gisler, A, Korten, I, de Hoogh, K, Vienneau, D, Frey, U, Decrue, F, et al. Associations of air pollution and greenness with the nasal microbiota of healthy infants: a longitudinal study. Environ Res. (2021) 202:111633. doi: 10.1016/j.envres.2021.111633
39. Adar, SD, Huffnagle, GB, and Curtis, JL. The respiratory microbiome: an underappreciated player in the human response to inhaled pollutants? Ann Epidemiol. (2016) 26:355–9. doi: 10.1016/j.annepidem.2016.03.010
40. Li, R, Li, J, and Zhou, X. Lung microbiome: new insights into the pathogenesis of respiratory diseases. Sig Transduct Target Ther. (2024) 9:19. doi: 10.1038/s41392-023-01722-y
41. Vieceli, T, Tejada, S, Martinez-Reviejo, R, Pumarola, T, Schrenzel, J, Waterer, GW, et al. Impact of air pollution on respiratory microbiome: a narrative review. Intensive Crit Care Nurs. (2023) 74:103336. doi: 10.1016/j.iccn.2022.103336
42. Carney, SM, Clemente, JC, Cox, MJ, Dickson, RP, Huang, YJ, Kitsios, GD, et al. Methods in lung microbiome research. Am J Respir Cell Mol Biol. (2020) 62:283–99. doi: 10.1165/rcmb.2019-0273TR
43. Chang, D, Dela Cruz, CS, and Sharma, L. Challenges in understanding lung microbiome: it is NOT like the gut microbiome. Respirology. (2020) 25:244–5. doi: 10.1111/resp.13759
44. Nearing, JT, Comeau, AM, and Langille, MGI. Identifying biases and their potential solutions in human microbiome studies. Microbiome. (2021) 9:113. doi: 10.1186/s40168-021-01059-0
45. Wilkinson, JE, Franzosa, EA, Everett, C, Li, C, HCMPH researchers and traineesBae, S, et al. A framework for microbiome science in public health. Nat Med. (2021) 27:766–74. doi: 10.1038/s41591-021-01258-0
46. Proctor, DM, and Relman, DA. The landscape ecology and microbiota of the human nose, mouth, and throat. Cell Host Microbe. (2017) 21:421–32. doi: 10.1016/j.chom.2017.03.011
47. Jones, J, Reinke, SN, Ali, A, Palmer, DJ, and Christophersen, CT. Fecal sample collection methods and time of day impact microbiome composition and short chain fatty acid concentrations. Sci Rep. (2021) 11:13964. doi: 10.1038/s41598-021-93031-z
48. Sarkar, A, Kuehl, MN, Alman, AC, and Burkhardt, BR. Linking the oral microbiome and salivary cytokine abundance to circadian oscillations. Sci Rep. (2021) 11:2658–13. doi: 10.1038/s41598-021-81420-3
49. Ryan, MJ, Schloter, M, Berg, G, Kostic, T, Kinkel, LL, Eversole, K, et al. Development of microbiome biobanks – challenges and opportunities. Trends Microbiol. (2021) 29:89–92. doi: 10.1016/j.tim.2020.06.009
50. Chuong, KH, Hwang, DM, Tullis, DE, Waters, VJ, Yau, YCW, Guttman, DS, et al. Navigating social and ethical challenges of biobanking for human microbiome research. BMC Med Ethics. (2017) 18:1. doi: 10.1186/s12910-016-0160-y
51. Sanna, S, Kurilshikov, A, van der Graaf, A, Fu, J, and Zhernakova, A. Challenges and future directions for studying effects of host genetics on the gut microbiome. Nat Genet. (2022) 54:100–6. doi: 10.1038/s41588-021-00983-z
52. Lagier, J-C, Khelaifia, S, Alou, MT, Ndongo, S, Dione, N, Hugon, P, et al. Culture of previously uncultured members of the human gut microbiota by culturomics. Nat Microbiol. (2016) 1:16203. doi: 10.1038/nmicrobiol.2016.203
53. Fiedorová, K, Radvanský, M, Němcová, E, Grombiříková, H, Bosák, J, Černochová, M, et al. The impact of DNA extraction methods on stool bacterial and fungal microbiota community recovery. Front Microbiol. (2019) 10:821. doi: 10.3389/fmicb.2019.00821
54. Winand, R, Bogaerts, B, Hoffman, S, Lefevre, L, Delvoye, M, van Braekel, J, et al. Targeting the 16S rRNA gene for bacterial identification in complex mixed samples: comparative evaluation of second (Illumina) and third (Oxford Nanopore technologies) generation sequencing technologies. Int J Mol Sci. (2019) 21:298. doi: 10.3390/ijms21010298
55. Johnson, JS, Spakowicz, DJ, Hong, B-Y, Petersen, LM, Demkowicz, P, Chen, L, et al. Evaluation of 16S rRNA gene sequencing for species and strain-level microbiome analysis. Nat Commun. (2019) 10:1–11. doi: 10.1038/s41467-019-13036-1
56. Zahavi, L, Lavon, A, Reicher, L, Shoer, S, Godneva, A, Leviatan, S, et al. Bacterial SNPs in the human gut microbiome associate with host BMI. Nat Med. (2023) 29:2785–92. doi: 10.1038/s41591-023-02599-8
57. López-Aladid, R, Fernández-Barat, L, Alcaraz-Serrano, V, Bueno-Freire, L, Vázquez, N, Pastor-Ibáñez, R, et al. Determining the most accurate 16S rRNA hypervariable region for taxonomic identification from respiratory samples. Sci Rep. (2023) 13:3974. doi: 10.1038/s41598-023-30764-z
58. Lozupone, CA, Stombaugh, J, Gonzalez, A, Ackermann, G, Wendel, D, Vázquez-Baeza, Y, et al. Meta-analyses of studies of the human microbiota. Genome Res. (2013) 23:1704–14. doi: 10.1101/gr.151803.112
59. Iliev, ID, and Cadwell, K. Effects of intestinal Fungi and viruses on immune responses and inflammatory bowel diseases. Gastroenterology. (2021) 160:1050–66. doi: 10.1053/j.gastro.2020.06.100
60. Matijašić, M, Meštrović, T, Čipčić Paljetak, H, Perić, M, Barešić, A, and Verbanac, D. Gut microbiota beyond Bacteria—Mycobiome, Virome, Archaeome, and eukaryotic parasites in IBD. IJMS. (2020) 21:2668. doi: 10.3390/ijms21082668
61. Arnold, JW, Roach, J, and Azcarate-Peril, MA. Emerging Technologies for Gut Microbiome Research. Trends Microbiol. (2016) 24:887–901. doi: 10.1016/j.tim.2016.06.008
62. Sezgin, E, Terlemez, G, Bozkurt, B, Bengi, G, Akpinar, H, and Büyüktorun, İ. Quantitative real-time PCR analysis of bacterial biomarkers enable fast and accurate monitoring in inflammatory bowel disease. PeerJ. (2022) 10:e14217. doi: 10.7717/peerj.14217
63. Bouwens, A, Deen, J, Vitale, R, D’Huys, L, Goyvaerts, V, Descloux, A, et al. Identifying microbial species by single-molecule DNA optical mapping and resampling statistics. NAR Genom Bioinformat. (2020) 2:p. lqz007. doi: 10.1093/nargab/lqz007
64. Ferreira, I, Lepuschitz, S, Beisken, S, Fiume, G, Mrazek, K, Frank, BJH, et al. Culture-free detection of antibiotic resistance markers from native patient samples by hybridization capture sequencing. Microorganisms. (2021) 9:1672. doi: 10.3390/microorganisms9081672
65. Walker, AW, and Hoyles, L. Human microbiome myths and misconceptions. Nat Microbiol. (2023) 8:1392–6. doi: 10.1038/s41564-023-01426-7
66. Zheludev, IN, Edgar, RC, Lopez-Galiano, MJ, de la Peña, M, Babaian, A, Bhatt, AS, et al. Viroid-like colonists of human microbiomes. bioRxiv. (2024). doi: 10.1101/2024.01.20.576352
67. Pasolli, E, Schiffer, L, Manghi, P, Renson, A, Obenchain, V, Truong, DT, et al. Accessible, curated metagenomic data through ExperimentHub. Nat Methods. (2017) 14:1023–4. doi: 10.1038/nmeth.4468
68. Huttenhower, C, Finn, RD, and McHardy, AC. Challenges and opportunities in sharing microbiome data and analyses. Nat Microbiol. (2023) 8:1960–70. doi: 10.1038/s41564-023-01484-x
69. David, L, Maurice, C, Carmody, R, et al. Diet rapidly and reproducibly alters the human gut microbiome. Nature. (2014) 505:559–63. doi: 10.1038/nature12820
70. Oliver, A, Chase, AB, Weihe, C, Orchanian, SB, Riedel, SF, Hendrickson, CL, et al. High-fiber, whole-food dietary intervention alters the human gut microbiome but not fecal short-chain fatty acids. mSystems. (2021) 6:e00115–21. doi: 10.1128/mSystems.00115-21
71. Abdelsalam, NA, Ramadan, AT, ElRakaiby, MT, and Aziz, RK. Toxicomicrobiomics: The human microbiome vs. pharmaceutical, dietary, and environmental xenobiotics. Front Pharmacol. (2020) 11:390. doi: 10.3389/fphar.2020.00390
72. Fournier, E, Ratel, J, Denis, S, Leveque, M, Ruiz, P, Mazal, C, et al. Exposure to polyethylene microplastics alters immature gut microbiome in an infant in vitro gut model. J Hazard Mater. (2023) 443:130383. doi: 10.1016/j.jhazmat.2022.130383
73. Radjabzadeh, D, Bosch, JA, Uitterlinden, AG, et al. Gut microbiome-wide association study of depressive symptoms. Nat Commun. (2022) 13:7128. doi: 10.1038/s41467-022-34502-3
74. Almeida, A, Nayfach, S, Boland, M, Strozzi, F, Beracochea, M, Shi, ZJ, et al. A unified catalog of 204,938 reference genomes from the human gut microbiome. Nat Biotechnol. (2021) 39:105–14. doi: 10.1038/s41587-020-0603-3
75. Zha, Y, Chong, H, Yang, P, and Ning, K. Microbial dark matter: from discovery to applications. Genomics Proteomics Bioinformatics. (2022) 20:867–81. doi: 10.1016/j.gpb.2022.02.007
76. Tierney, BT, Yang, Z, Luber, JM, Beaudin, M, Wibowo, MC, Baek, C, et al. The landscape of genetic content in the Gut and Oral human microbiome. Cell Host Microbe. (2019) 26:283–295.e8. doi: 10.1016/j.chom.2019.07.008
77. McDonald, D, Hyde, E, Debelius, JW, Morton, JT, Gonzalez, A, Ackermann, G, et al. American Gut: an open platform for citizen science microbiome research. mSystems. (2018) 3:e00031–18. doi: 10.1128/mSystems.00031-18
78. Lebeer, S, Ahannach, S, Gehrmann, T, et al. A citizen-science-enabled catalogue of the vaginal microbiome and associated factors. Nat Microbiol. (2023) 8:2183–2195. doi: 10.1038/s41564-023-01500-0
79. Herd, P, Palloni, A, Rey, F, and Dowd, JB. Social and population health science approaches to understand the human microbiome. Nat Hum Behav. (2018) 2:808–15. doi: 10.1038/s41562-018-0452-y
80. Shanahan, F, Ghosh, TS, and O’Toole, PW. Human microbiome variance is underestimated. Curr Opin Microbiol. (2023) 73:102288. doi: 10.1016/j.mib.2023.102288
81. Sirugo, G, Williams, SM, and Tishkoff, SA. The missing diversity in human genetic studies. Cell. (2019) 177:26–31. doi: 10.1016/j.cell.2019.02.048
82. Diaz, V . Encouraging participation of minorities in research studies. Ann Fam Med. (2012) 10:372–3. doi: 10.1370/afm.1426
83. Bonevski, B, Randell, M, Paul, C, Chapman, K, Twyman, L, Bryant, J, et al. Reaching the hard-to-reach: a systematic review of strategies for improving health and medical research with socially disadvantaged groups. BMC Med Res Methodol. (2014) 14:42. doi: 10.1186/1471-2288-14-42
84. Xiao, L, Zhang, F, and Zhao, F. Large-scale microbiome data integration enables robust biomarker identification. Nat Comput Sci. (2022) 2:307–16. doi: 10.1038/s43588-022-00247-8
85. Seneviratne, CJ, Balan, P, Suriyanarayanan, T, Lakshmanan, M, Lee, DY, Rho, M, et al. Oral microbiome-systemic link studies: perspectives on current limitations and future artificial intelligence-based approaches. Crit Rev Microbiol. (2020) 46:288–99. doi: 10.1080/1040841X.2020.1766414
86. Rothschild, D, Leviatan, S, Hanemann, A, Cohen, Y, Weissbrod, O, and Segal, E. An atlas of robust microbiome associations with phenotypic traits based on large-scale cohorts from two continents. PLoS One. (2022) 17:e0265756. doi: 10.1371/journal.pone.0265756
87. Vujkovic-Cvijin, I, Sklar, J, Jiang, L, Natarajan, L, Knight, R, and Belkaid, Y. Host variables confound gut microbiota studies of human disease. Nature. (2020) 587:448–54. doi: 10.1038/s41586-020-2881-9
88. Craig, SJC, Blankenberg, D, Parodi, ACL, Paul, IM, Birch, LL, Savage, JS, et al. Child weight gain trajectories linked to Oral microbiota composition. Sci Rep. (2018) 8:14030–14. doi: 10.1038/s41598-018-31866-9
89. Wernroth, ML, Peura, S, Hedman, AM, Hetty, S, Vicenzi, S, Kennedy, B, et al. Development of gut microbiota during the first 2 years of life. Sci Rep. (2022) 12:1–13. doi: 10.1038/s41598-022-13009-3
90. Gao, Z, Li, P, Lin, H, Lin, W, and Ren, Y. Biomarker selection strategies based on compound stability in wastewater-based epidemiology. Environ Sci Pollut Res. (2023) 30:5516–29. doi: 10.1007/s11356-022-24268-y
Keywords: microbiome, population-based health studies, population, cohort, metagenomics, biomarkers
Citation: Buytaers FE, Berger N, Van der Heyden J, Roosens NHC and De Keersmaecker SCJ (2024) The potential of including the microbiome as biomarker in population-based health studies: methods and benefits. Front. Public Health. 12:1467121. doi: 10.3389/fpubh.2024.1467121
Received: 19 July 2024; Accepted: 09 October 2024;
Published: 23 October 2024.
Edited by:
Atin Adhikari, Georgia Southern University, United StatesReviewed by:
Anindya Chanda, Mycologics LLC, United StatesCopyright © 2024 Buytaers, Berger, Van der Heyden, Roosens and De Keersmaecker. This is an open-access article distributed under the terms of the Creative Commons Attribution License (CC BY). The use, distribution or reproduction in other forums is permitted, provided the original author(s) and the copyright owner(s) are credited and that the original publication in this journal is cited, in accordance with accepted academic practice. No use, distribution or reproduction is permitted which does not comply with these terms.
*Correspondence: Sigrid C. J. De Keersmaecker, Sigrid.DeKeersmaecker@sciensano.be
Disclaimer: All claims expressed in this article are solely those of the authors and do not necessarily represent those of their affiliated organizations, or those of the publisher, the editors and the reviewers. Any product that may be evaluated in this article or claim that may be made by its manufacturer is not guaranteed or endorsed by the publisher.
Research integrity at Frontiers
Learn more about the work of our research integrity team to safeguard the quality of each article we publish.