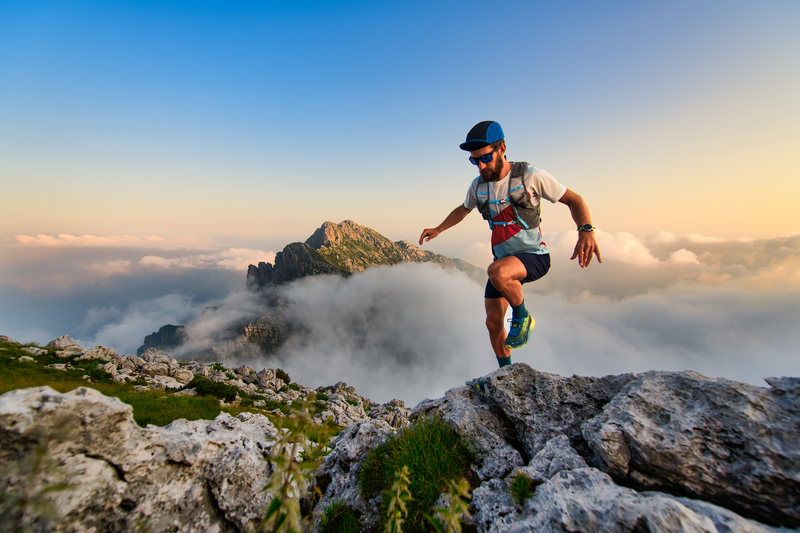
95% of researchers rate our articles as excellent or good
Learn more about the work of our research integrity team to safeguard the quality of each article we publish.
Find out more
ORIGINAL RESEARCH article
Front. Public Health , 16 September 2024
Sec. Aging and Public Health
Volume 12 - 2024 | https://doi.org/10.3389/fpubh.2024.1454847
This article is part of the Research Topic International Perspectives on Older Adult Social Isolation and Loneliness View all 54 articles
Background: Social interactions are essential to social connectedness among older adults. While many scales have been developed to measure various aspects of social connectedness, most are narrow in scope, which may not be optimally encompassing, practical, or relevant for use with older adults across clinical and community settings. Efforts are needed to create more sensitive scales that can identify “upstream risk,” which may facilitate timey referral and/or intervention.
Objective: The purposes of this study were to: (1) develop and validate a brief scale to measure threats to social connectedness among older adults in the context of their social interactions; and (2) offer practical scoring and implementation recommendations for utilization in research and practice contexts.
Methods: A sequential process was used to develop the initial instrument used in this study, which was then methodologically reduced to create a brief 13-item scale. Relevant, existing scales and measures were identified and compiled, which were then critically assessed by a combination of research and practice experts to optimize the pool of relevant items that assess threats to social connectedness while reducing potential redundancies. Then, a national sample of 4,082 older adults ages 60 years and older completed a web-based questionnaire containing the initial 36 items about social connection. Several data analysis methods were applied to assess the underlying dimensionality of the data and construct measures of different factors related to risk, including item response theory (IRT) modeling, clustering techniques, and structural equation modeling (SEM).
Results: IRT modeling reduced the initial 36 items to create the 13-item Upstream Social Interaction Risk Scale (U-SIRS-13) with strong model fit. The dimensionality assessment using different clustering algorithms supported a 2-factor solution to classify risk. The SEM predicting highest risk items fit exceptionally well (RMSEA = 0.048; CFI = 0.954). For the 13-item scale, theta scores generated from IRT were strongly correlated with the summed count of items binarily identifying risk (r = 0.896, p < 0.001), thus supporting the use of practical scoring techniques for research and practice (Cronbach’s alpha = 0.80).
Conclusion: The U-SIRS-13 is a multidimensional scale with strong face, content, and construct validity. Findings support its practical utility to identify threats to social connectedness among older adults posed by limited physical opportunities for social interactions and lacking emotional fulfillment from social interactions.
Social interactions are essential to social connectedness among older adults. Social connectedness refers the structural (e.g., network size and composition, partner status), functional (e.g., perceived social support, loneliness), and quality (e.g., relationship quality or strain) aspects of an individual’s social relationships (1, 2). Taken together, social connectedness can be thought as an umbrella term to encompass social isolation (objectively having limited contact with others) and loneliness (subjective feeling of being alone) (2, 3). An estimated 25% of older adults are thought to be socially isolated (3) and over 40% are thought to be lonely (2, 4, 5), which can be a staggering figure considering these concepts are interrelated but do not necessarily overlap completely (2, 3). Older adults who are socially connected have a reduced risk of all-cause mortality (6, 7), but those experiencing social isolation and loneliness are at an increased risk of diminished physical (e.g., heart disease, stroke) (8, 9) and mental health (e.g., depression, anxiety) (10), cognitive impairment (11, 12), and risky behaviors (e.g., substance use, sedentary behavior) (13–15). As such, it is imperative that we identify threats to social connectedness early through routine screening across community sectors and facilitate meaningful social interactions among and between older adults.
Many validated scales have been developed to measure various aspects of social connectedness (3, 16). These scales are frequently used in the research context and are increasingly used to identify baseline risk (often for service recommendations or referrals) and evaluate the effectiveness of clinical and community-based interventions (3, 17). Each of these scales were purposively developed to measure a specific aspect or trait of social connectedness; therefore, they may be seen as assessing only one component within a larger set of interrelated risks (18). This can create operationalization-related issues and implications for social care because, while social disconnectedness is a multi-faceted problem, the singular outcome selected for use is frequently treated as the sole indicator of how an older adult perceives their overall social wellbeing. As such, in research and practice, administering a combination of multiple scales may be necessary to provide a more comprehensive view of risk or threats to social connectedness, which may introduce undue data collection burdens.
The scales commonly used in research and practice have helped the field define and quantify the prevalence of risk within the older adult population (3, 18). However, many of these scales may not be optimally encompassing, practical, or relevant for use with older adults across clinical and community settings. These scales are valuable to identify dimensions of risk, but when used independently, they may not adequately capture the complexity of all existing risk or guide intervention opportunities. Based on their generation of ample evidence, these scales provide a solid foundation on which to create new, contextually appropriate scales to assess risk among older adults. Further, given the overlap of concepts and items across existing scales, it may be practical to examine how these scales work together to identify risk and if underlying concepts can be captured with a single compilation of measures. Building upon the legacy and strength of existing scales, especially pervasive and commonly use scales such as the UCLA Loneliness Scale (19–21), it may be possible to create a more encompassing and sensitive scale that can identify “upstream risk” and detect the maximum amount of threat to social connectedness. Intentionally using more generous risk scoring algorithms may enable researchers and practitioners to identify older adults upstream (i.e., with emerging threats to social connection), which may allow for timely referral and/or intervention. Therefore, the purposes of this study were to: (1) develop and validate a brief scale to measure threats to social connectedness among older adults in the context of their social interactions; and (2) offer practical scoring recommendations for utilization in research and practice settings. Social interactions were conceptualized in terms of the physical opportunities to interact with others (e.g., having a social network, ability to find companionship, going to gathering places) and the emotional fulfillment resulting from those interactions (e.g., satisfaction, content, longing). In this context, limited physical opportunities to interact and/or emotional fulfillment from interactions may place an older adult at risk for social disconnectedness. The availably of a brief, sensitive, and easy-to-administer scale that more broadly assesses threats to social connectedness can complement the inventory of existing measures and assist researchers and practitioners to efficiently identify multi-faceted risk among older adults.
A sequential process was used to develop the initial instrument used in this study, which was then methodologically reduced to create a brief 13-item scale. The overall intent was to identify a wide range of existing, validated scales and items capable documenting the complexity of social connectedness as an encompassing concept, then employ a series of statistical analyses to identify the most parsimonious set of items to measure threats to social connectedness. The goal of developing a parsimonious brief measure from this process was to increase practical administration in research and practice settings.
First, 13 validated scales were identified from the published literature based their relevance to various social connectedness aspects, presence in the published literature, and use in research and practice with older adults. These scales included the Berkman-Syme Social Network Index (22), Brief Sense of Community Scale (23), Campaign to End Loneliness Measurement Tool (24), Connect2Affect Assessment (25), Connor-Davidson Resilience Scale (26), Cornwell Perceived Isolation Scale (27), de Jong Gierveld Loneliness Scale (28), Duke Social Support Index (29), Life Space Questionnaire (30), Lubben Social Network Scale (31), Patient Health Questionnaire-2 (32), Steptoe Social Isolation Index (33), and Revised UCLA Loneliness Scale (19, 20). During this process, the goal was to identify as many relevant scales and measures as possible that could be associated with social interactions among older adults.
Second, these scales were critically reviewed for content and overlapping concepts to optimize the potential universe of relevant items to assess threats to social connectedness while reducing potential redundancies. This process was undertaken by a combination of research and practice experts (n = 3), who ranked each item within each identified measure in terms of its relevance to social connectedness. When consensus was not reached about whether to include an item, the experts elected to retain the item to be more inclusive at this stage.
Third, an expanded panel of experts (n = 19) was engaged to review the collection of identified items, evaluate their appropriateness, recommend items to fill concept gaps, and/or offer alternative wording and response choices for presented items. Experts were selected from within the researchers’ professional network based on their content expertise and experience engaging older adults in screening and service delivery. This expanded expert panel included a diversified group of clinicians, professionals, and community members. More specifically, experts represented the disciplines of public health, gerontology, medicine, nursing, social work, psychology, physical therapy, and health education. Further, experts included representatives of Area Agencies on Aging, community health workers, caregivers of older adults, and community-dwelling older adults. This process was not a formal consensus-building effort in that experts were not convened together or asked to agree upon the initial instrument to be tested. Rather, experts were asked to provide their feedback individually (i.e., in written or verbal format), and their responses were shared with other experts when appropriate.
Recommendations were assessed, accepted, and incorporated into the initial instrument. A total of 36 items were included to assess threats to social connectedness, which were included as part of a larger survey of older adults. Finally, the instrument was piloted by older adults (n = 5) who provided feedback on the items in terms of comprehension, readability, and appropriateness.
Table 1 presents the initial 36 items used to assess threats to social connectedness along with their source(s) of origin and response categories. The compiled items originated from 12 sources. All items were close-ended and used one of five different sets of response categories (e.g., yes/no; none/one/two or more; none of the time/some of the time/often). To capture the maximum amount of reported risk and create more uniformity across items, responses for each item were recoded to a binary state that indicates “risk” and “no risk.” This was accomplished by scoring response choices indicative of the absence of risk as 0 and all other response choices as 1 (see Table 1). Recoding each item accounted for differing response categories, while retaining the sensitivity of the original item format (e.g., an affirmation of an item to any degree was taken as an affirmation) and allowed for the detection of upstream risk.
This study used a cross-sectional, internet-delivered questionnaire to collect data from older adults ages 60 years and older. Participants were recruited nationwide through a Qualtrics Internet Panel (34) from June 2019 to September 2019. Given the potential sampling bias introduced by convenience sampling, quota sampling parameters were employed to ensure diversity among participants in terms of age, sex, race, and geography (35). A total of 4,101 older adults completed the survey, of which 19 were omitted for missing data. The resulting analytic sample included 4,082 participants from all 50 states and two U.S. territories. All survey procedures were approved by the Texas A&M University Institutional Review Board (IRB2019-0375).
Data were analyzed using R in an exploratory fashion to generate a brief and practical scale to identify threats to social connectedness (i.e., risk for social disconnectedness) among older adults. Several methods were applied to assess the underlying dimensionality of the data and construct measures of different factors related to risk (i.e., limited opportunity for social interactions and lacking emotional fulfillment from social interactions). First, after assessing relevant data assumptions (e.g., local independence, monotonicity, item variance), a unidimensional item response theory (IRT) model was fit to confirm the item’s relationship with the measure of risk and correlate participant scores (i.e., θs) on this measure. IRT guided item reduction from the initial 36 items to the resulting 13-item scale. Second, clustering techniques were used to assess the number of subgroups present in the sample based on participants’ response patterns to the instrument items. Third, a structural equation model (SEM) was constructed to confirm the relationship among the latent variables on this risk dimension measured by the 13-item scale.
Items were recoded to reflect a binary solution (risk/no risk). Two (2PL) and three (3PL) parameter logistic models (36, 37) were fit to both the initial 36 items and 13-item versions of the scales in which the primary dimension was assumed to be risk. S-χ2 was used to detect items that did not fit well within each model, respectively. Several items were flagged as ‘poorly’ performing items in that they did not discriminate among participants at any point on the primary dimension. These items were discarded through the iterative process of pruning. A one parameter logistic model (i.e., 1PL or Rasch Model) (38) was also fit, which does not allow for discrimination, to confirm the theory that the two parameter logistic model best fit these data. The item response model was recalibrated on the subset of items selected for inclusion into the 13-item scale on the basis of the original item parameters and domain knowledge of the researchers. Results from recalibrating item and participant parameters for the 13-item scale closely mirrored results for the initial 36-item set, suggesting minimal impact on measuring the primary dimension when using the 13-item subset of items. All IRT models were fit using the MIRT Package in R (39).
An unsupervised neural network approach was used to explore the underlying dimensionality of the participants responding to the 13-item scale. Four different clustering algorithms were compared utilizing a k-fold method of selecting criterion. A “leave one out” k-fold methodology allows assessment of individual item importance to the stability of the clustering solutions. Furthermore, it allows multiple solution sets to be compared in a data-driven manner to assess a level of agreement on the underlying number of sub-groups present in the data. Partitions around medioids (PAM) (40, 41) was ultimately selected as the best option to cluster the data based on validation metrics (42–45). A two-cluster solution representing low-and high-risk participants was chosen by validating the cluster membership with comparisons of proportions of endorsement for items representing either low or high risk.
A structural equation modeling framework was utilized to confirm the relationship among the latent factors governing the responses in the 13-item scale. Based on participant response patterns, three groups of items emerged, which were used as latent factors in the SEM. Clustering results gave evidence for items that were related to each other in response pattern among the participants, as well as being conceptually related. The three factors that emerged were related to the physical opportunity of the older adult to interact with others (i.e., Factor 2), their emotional fulfillment from these interactions (or lack thereof) (i.e., Factor 3), and general feelings of disconnectedness (i.e., Factor 1) captured by select broad items from the UCLA Loneliness Scale. The structure of the model was implemented to support the hypothesis that physical opportunities for interaction and the emotional fulfillment of interactions would predict how older adults perceived their general disconnectedness. As such, Factor 1 was treated as endogenous and regressed onto the two exogenous factors (i.e., Factors 2 and 3). While allowing the exogenous factors to correlate to emulate the potential interrelation between these factors in real-world conditions, no other individual item variances or error variances were allowed to correlate in the final model.
Table 2 presents sample characteristics of the 4,082 older adults who participated in this study. On average, participants were age 69.58 (±5.24), and the majority was female (58.5%), non-Hispanic (84.7%), White or Caucasian (73.5%), and lived with a spouse or partner (57.1%). Approximately 47% of participants had a college degree or more, with 35.6% reporting some college or technical school education and 17.6% reporting a high school education or less. On average, participants reported 3.29 (±2.56) chronic conditions, with the most frequently reported conditions being hypertension (53.4%), high cholesterol (48.0%), arthritis or rheumatic disease (31.7%), chronic pain (23.0%), and diabetes (20.4%).
Two and three parameter logistic IRT models were calibrated for the initial 36 items, and again for the 13-item screener in part on some results from the dimensionality assessment above alongside the long-form calibration. The items included were determined to cover a good nomeopathic span (46) regarding an older adult’s risk for limited social interactions in terms of physical opportunities to interact with others and the emotional fulfillment from social interactions.
As seen in Table 3, the overall model fit was better for the 13-item compared to the initial 36 items. The 2PL model was selected because the discrimination values for each of the items allowed for the selection of items that were relevant along the entirety of the trait continuum. The 1PL model was tested for its parsimonious nature, but it was not expected to fit as well as a 2PL model due to the discrimination parameter. Lastly, a 3PL variant was tested to account for random guessing, but the increase in parameterization did not outweigh the lack of statistical and conceptual fit to the task. This is most evident by the improvement of fit observed for each of the transitions from the 1PL to 2PL models illustrated in Table 3. In theory, there are items that ‘discriminate’ more on different components of the trait spectrum, and if this assumption is to be considered true, an increase in fit should be observed (as it is here). The drop of AIC/BIC and − 2 x LL for each model stops with the addition of the third parameter for each item. The guessing parameter does not afford any sizable increase in fit, and as such, the most appropriate form of a model for these data is the 2PL model (47, 48).
As seen in Table 4, the M2 model fit statistic (49) indicates that the 13-item scale of the test fits better than the initial 36 item for every model metric. More specifically, the relative fit for the 13-item scale improves over the initial 36 items as illustrated by the confidence interval for the RMSEA not containing 0.05 and the CFI larger than 0.95. It can be concluded that the 13-item variant reproduces data under the model more consistently, if not better than the 36-item scale, likely due to a loss of complexity by reducing ‘noise’ from modeling items that are not as strongly related to the trait of interest.
Individual item fit was calculated using a S-χ2 statistic (50, 51). This goodness-of-fit test shows how well the expected score during parameter estimation conforms with the observed score at different places on the trait continuum. Table 5 illustrates how individual item fit improved in many cases when reducing the scale from 36 to 13 items. In other cases, fit remained relative the same.
Further evidence to support the fit of the model comes from the use of the participant parameters. When the binary responses from 13-item scale were summed to create a total score (range from 0 to 13) and correlated with the θs parameter generated from the IRT process, a strong and significant correlation was observed (r = 0.896, p < 0.001). This indicates that the summed score of binary responses can serve as a statistical proxy for an older adult, which lends itself to more practical use in research and practice settings. Additional information about practical scoring of the 13-item scale is provided elsewhere in this manuscript.
Reliability in the transition from the initial 36 items to the 13-item scale was assessed. The initial Cronbach’s alpha for the initial 36 items was 0.81. This reliability metric was reassessed after the item selection process was employed following the results of the IRT models dimensionality assessment, and SEM model. This transition revealed favorable results for a 13-item scale was seen as the Cronbach’s alpha for the 13-item scale was 0.80, which indicates no significant loss in reliability in the sample of 4,082 individuals after omitting 23 items. To assess any potential subgroup biases with the 13-item scale, reliability coefficients were calculated for variable categories by sex, ethnicity, race, education, living with partner, and reporting multi-morbidity. Each respective calculation yielded Cronbach’s alpha coefficients equal to or greater than 0.78, which indicates consistently strong internal reliability for subgroups within the current sample.
Three different measures were chosen to evaluate the number of clusters in the solution (i.e., older adults based on their responses to the 13 items). Connectiveness, similarity, and compactness were selected to compare clustering methodologies. Measures of connectivity and silhouette width should be minimized, and the Dunn index should be maximized (42). As such, it appears that the optimal number of clusters is 2. The Dunn index uses the ratios of the smallest distance between observations not in the same cluster to the largest intra-cluster distance; therefore, it has a bias towards a larger number of clusters.
The stability measures assessed the change in distance metrics used across clustering techniques when one column is removed from the dataset and the clustering technique is re-ran in a k-fold fashion. Therefore, the stability measure through this leave-one-out procedure should be able to detect if there are any clustering methods that are sensitive to a particular item. Average proportion of non-overlap (APN); average distance of means (ADM); and average distance (AD) between cases in the same clusters should all be minimized.
The separation of the clusters is one of the most important metrics to consider when the goal is to classify individuals in an applied setting. Plotting the component scores, as seen in Figure 1, illustrates that we can expect clear separation on the first dimension and subtle separation on the second vertical dimension for the clusters.
A discriminant clustering algorithm was applied to the data to ‘trim’ fringe cases by selecting a discriminant function, which maximizes the differences between these cases (52, 53). Figure 2 illustrates the results of these analyses and shows that when attempting to maximize differences between clusters with a discriminant function, more separation can be seen on the vertical dimension. It should be noted that the primary discriminant coordinate still categorized the older adults primarily despite clear vertical separation of the clusters.
A structural equation modeling framework was leveraged against the data for the 13-item scale and fit using three latent factors (see Figure 3). Identification of items for each factors utilized results from the dimensionality assessment and IRT model parameters in conjunction with the intended ecological application of each item. The three items in Factor 1 (i.e., ‘I lack companionship’; ‘I feel isolated from others’; and ‘I feel like no one really knows me well’) were considered the target of the prediction because they were deemed to capture general feelings of disconnectedness and they were the strongest items indicative of risk. Meanwhile, the remaining items represent two distinct ways in which this outcome can occur, namely a physical opportunity component (Factor 2) and an emotional fulfillment component (Factor 3).
The model fits exceptionally well (RMSEA = 0.048; CFI = 0.954) (54, 55). The structural regression component favored the contribution of the Factor 3 items over the Factor 2 items (Coefficients of 1.348/0.832, respectively). However, the physical opportunity component significantly predicted the Factor 1 items. All coefficients were positive, indicating that higher scores on the individual items were directly related to an increase in the Factor 1 risk probability (i.e., general feelings of social disconnectedness). Covariance between Factor 2 and Factor 3 were permitted, which were only roughly related with a true correlation of 0.59. This suggests that the physical opportunity of an older adult to interact with others does not directly infer that the emotional fulfillment from of those interactions is positive.
This study reports the process used to develop and validate the Upstream Social Interaction Risk Scale (U-SIRS), a 13-item scale to assess threats to social connectedness among older adults in terms of their social interactions. This scale is novel in that it was created from a modified composite of seven existing scales used to measure various aspects of social connectedness, and it aims to measure “upstream risk” by scoring scale items binarily to identify the maximum amount of risk. This scale is practical in that it emphasizes general threats to social connectedness, as well as elements associated with physical opportunities to socially interact and the emotional fulfillment from such interactions (or the lack thereof), which may enable a more actionable approach to connecting older adults to services, resources, and programs. The potential utility of this scale in clinical and community settings is vast because it may help identify a wider scope of risk using a single measure and may provide sites with a practical alternative to existing scales, which can alleviate the screening and data collection burden associated with using multiple scales simultaneously.
The process to develop this scale was two-fold in that it engaged a diverse set of clinicians, professionals, and community members to help define the possible universe of questions to measure threats to social connectedness. Through this process, these experts prioritized and omitted items that seemed overlapping and of limited practical value to identify risk. They suggested language changes to the items and response choices, which helped the items’ readability, comprehension, and feasibility for use in real-world circumstances. Further, the items were refined to be phrased in a more positive or uplifting manner in attempt to avoid evoking negative feelings simply from completing the items. Interestingly, when attempting to define the universe of possible items, no single existing scale was included in its entirety and most items were altered in terms of their phraseology or response choices. This process resulted in 36 items with strong face validity as a starting point for further testing and scale refinement.
After collecting data from over 4,000 older adults ages 60 years and older nationwide, the item response theory (IRT) process analyzed the initial 36 items to quantify the latent trait of this general risk or threat to social connectedness. Through this process, a subset of 13 items were identified as contributing most to the overall latent trait of risk and that the other 23 items did not provide much information to the latent trait. Further, the Cronbach’s alpha for the initial 36 items and the reduced set of 13 items were comparable and above 0.80, indicating strong internal consistency reliability. Taken together, the reduced 13-item scale was identified as the strongest set of items to measure this latent trait of risk.
After reducing the scale from 36 to 13 items, the dimensionality assessment examined potential patterns of participant responses for the 13-item scale. In these analyses, it was determined that the 2PL solution clearly stratified this older adult population into high and low risk groups. This reinforces that these 13 items can be utilized to create separation based on risk levels, which has practical implications for future studies to establish risk-based scoring for the scale (i.e., identifying cut-points for risk thresholds).
Among the most compelling findings of this validation process is that the SEM confirmed that the U-SIRS-13 scale consists of three distinct factors, which include general feelings of disconnectedness (identified by three items from the UCLA Loneliness Scale), physical opportunities for social interaction, and emotional fulfillment from social interactions (or the lack thereof). The SEM model indicates that the distinct physical opportunity and emotional fulfillment sub-scales roughly predict each other (r = 0.59), but both strongly predict the general feelings of social disconnectedness. This reinforces the notion that an older adult may have the physical opportunity to interact with others, but that physical opportunity will not always infer that they perceive emotional fulfillment from such opportunities. The SEM model also shows that each scale item does not function on each scale factor, respectively. For example, the item ‘I lack companionship’ informs only the general feelings of disconnectedness factor and functions solely on this dimension. This particular item does not inform the other two factors, except that it can be predicted by the other items in the scale (i.e., those from the physical opportunity and emotional fulfillment sub-scales). As such, it is important to stress that, despite the U-SIRS-13 comprising three distinct factors, the IRT shows that all 13 items conform to a singular trait, which is also confirmed by the strong internal reliability coefficient for the binarily scored data (i.e., Cronbach’s alpha=0.80). Therefore, it is recommended that the U-SIRS-13 be scored as a continuous count variable of risk rather than scoring each sub-scale independently. However, examining the risk for each item may help practitioners identify threats to structure, function, and/or quality aspects of social connection and make appropriate resources, programs, or services.
An important and practical finding of this study is that the theta score from the IRT strongly correlated (r = 0.896, p < 0.001) with the number of items that participants endorsed as ‘risk’ (i.e., a count variable of binarily-scored items ranging from 0 to 13). Therefore, the results from the theta parameters from IRT model validates the use of total count score in practice. It is recommended that future efforts deploy these items using a uniform set of response choices capable of identifying varying levels of risk for each item. Table 6 presents the recommended U-SIRS-13 items, response choices, and practical scoring for dichotomizing items to identify the maximum amount of risk (i.e., “upstream risk”). After dichotomizing responses for each item, the 13 items should be summed to create a count variable ranging from 0 to 13, with higher values indicating more risk (17, 18). Additional ongoing demonstration studies and evaluation efforts have utilized this practical scoring for the U-SIRS-13 to examine the scale’s internal reliability. As seen in Table 7, the internal reliability of data collected with the practically-scored U-SIRS-13 remains consistently strong (i.e., Cronbach’s alpha ranging from 0.78 to 0.85) for general samples of older adults as well as those purposively recruited for social engagement interventions. Future and ongoing studies will also examine alternative scoring strategies for the U-SIRS-13.
Table 7. Internal reliability of practically-scored U-SIRS-13 in ongoing demonstration studies and evaluation efforts.
This validation study was not without limitation. Despite conducted with a large sample of diverse older adults ages 60 years and older across the United States (n = 4,082), probabilistic sampling was not used to select participants. Therefore, sociodemographics somewhat align with those of the greater older adult population in the United States (e.g., age, sex, ethnicity, living alone), these data were not nationally representative. Further, while Qualtrics panels are strong methods to recruit large samples quickly (35), the internet-based nature of recruitment and data collection may have introduced selection bias in terms of technology access, education level, and affluence (i.e., not reaching those with more potential risk for social disconnectedness). For these reasons, the analytic sample in this study may not be generalizable to the overall older adult population in the United States, especially among those with lower socioeconomic status. Data were self-reported, thus subject to social desirability bias, especially given the stigmatization of loneliness and social disconnection in the United States (56, 57). Despite these potential shortcomings, the strengths of this study include a diverse set of professionals who assisted in the initial item selection, a robust set of statistical analyses to generate and assess the U-SIRS-13, and the emerging evidence of replicability of the practically-scored scale.
Findings from this study highlight the need for additional research efforts to advance the utilization and application of the U-SIRS-13. First, beyond the set of ongoing demonstration studies and evaluation efforts, additional replication studies are needed among diverse samples of older adults. More specifically, studies are needed to examine the appropriateness and reliability of the scale among samples of older adults with varying races and ethnicities, sexual orientations, socioeconomic statuses, impairments (e.g., sensory, mobility, cognitive), disabilities, and other known threats to social disconnectedness (e.g., partner status, living alone, limited transportation, caregiver status, multi-morbidity, fall history, food insecurity). Additional studies should also be performed to identify the utility and statistical integrity of this scale for younger adults. Second, statistical efforts are needed to identify risk-related thresholds and establish cut-points for risk levels. While higher scores on the U-SIRS-13 are indicative of higher risk, the identification of cut-points may help researchers and practitioners utilize the scale to identify high-risk older adults and make informed decisions for programs and services. Third, as another form of validation, efforts are needed to identify the concordance between professional perceptions of social disconnectedness relative to self-reported threats of disconnectedness using the U-SIRS-13 among older adults in clinical and community settings. Such efforts may provide insights into the ability of those serving older adults to recognize various threats to social connection and the relative advantage of administering the U-SIRS-13. Fourth, although the U-SIRS-13 is considered brief, professionals in clinical and community settings may find it lengthy for use during intake and routine assessments. As such, future research should consider validating a reduced set of items indicative of probable risk and requiring further assessment (e.g., akin to administering the 2-item PHQ as a “first step” approach, then administering the full 9-item PHQ if risk on the 2-item PHQ is identified) (58). Fifth, while anticipated to be sensitive to change over time, the U-SIRS-13 should be used in concurrent validity assessments to predict other outcomes as well as an outcome evaluation in relevant interventions to assess its ability to identify baseline risk and improvement post-intervention.
This study documented the development and validation of the Upstream Social Interaction Risk Scale (U-SIRS-13), a 13-item scale to document threats to social connection, which was created using a compilation of items from seven previously validated scales. The U-SIRS-13 contains an interrelated set of three distinct sub-scales that measure feelings of general disconnectedness, physical opportunities for social interactions, and emotional fulfillment from social interactions (or lack thereof). Despite the distinct sub-scales, the IRT and SEM support its use as a single scale. The strong correlation between the theta score and summed composite of binarily-scored items supports the practical utilization of the practically-scored U-SIRS-13 in research and practice settings (further supported by emerging replicability in demonstration studies and evaluation efforts). Building upon the legacy of existing scales, especially the UCLA Loneliness Scale, the U-SIRS-13 adds to the existing inventory of measures about social connection (16) with a more encompassing and sensitive scale that can identify “upstream risk” and detect the maximum amount of threat to social connectedness among older adults. Future efforts are needed to examine and identify risk levels and thresholds, which can help those using the U-SIRS-13 to classify older adults’ risk level, determine whether their risk is in terms of physical opportunities to interact or emotional fulfillment from interactions, and refer older adults to appropriate programs and services.
The raw data supporting the conclusions of this article will be made available by the authors, without undue reservation.
The studies involving humans were approved by Texas A&M University Institutional Review Board. The studies were conducted in accordance with the local legislation and institutional requirements. The ethics committee/institutional review board waived the requirement of written informed consent for participation from the participants or the participants’ legal guardians/next of kin because an information sheet was provided to participants before they completed the online questionnaire.
MS: Conceptualization, Data curation, Investigation, Methodology, Project administration, Writing – original draft, Writing – review & editing. MB: Formal analysis, Writing – original draft, Writing – review & editing.
The author(s) declare that no financial support was received for the research, authorship, and/or publication of this article.
The authors thank Leigh Ann Eagle and Sue Lachenmayr of Maintaining Active Citizens and the Maryland Living Well Center of Excellence for their leadership and invaluable innovation during the development of the Upstream Social Interaction Risk Scale (U-SIRS). The authors also thank the AARP Foundation, Administration for Community Living, USAging, Meals on Wheels America, National Council on Aging, and Foundation for Social Connection for their support and national leadership to address the issues of social isolation and loneliness. The authors also thank the many clinicians, professionals, and community members who provided feedback during this scale development process.
The authors declare that the research was conducted in the absence of any commercial or financial relationships that could be construed as a potential conflict of interest.
All claims expressed in this article are solely those of the authors and do not necessarily represent those of their affiliated organizations, or those of the publisher, the editors and the reviewers. Any product that may be evaluated in this article, or claim that may be made by its manufacturer, is not guaranteed or endorsed by the publisher.
1. Holt-Lunstad, J. Why social relationships are important for physical health: a systems approach to understanding and modifying risk and protection. Annu Rev Psychol. (2018) 69:437–58. doi: 10.1146/annurev-psych-122216-011902
2. Office of the U.S. Surgeon General. Our epidemic of loneliness and isolation: The U.S. Surgeon General’s advisory on the healing effects of social connection and community. Washington, DC (2023). Available at: https://www.hhs.gov/sites/default/files/surgeon-general-social-connection-advisory.pdf (accessed May 1, 2024).
3. National Academies of Sciences, Engineering, and Medicine. Social isolation and loneliness in older adults: Opportunities for the health care system. Washington, DC: The National Academies Press (2020).
4. Anderson, GO, and Thayer, CE. Loneliness and social connections: A national survey of adults 45 and older. Washington, DC: AARP Foundation (2018).
5. Hawkley, LC, Kozloski, M, and Wong, J. A profile of social connectedness in older adults. (2017). Available at: https://capitolhillvillage.org/wp-content/uploads/2018/11/A-Profile-of-Social-Connectedness-1.pdf
6. Holt-Lunstad, J, Smith, TB, and Layton, JB. Social relationships and mortality risk: a meta-analytic review. PLoS Med. (2010) 7:e1000316. doi: 10.1371/journal.pmed.1000316
7. Holt-Lunstad, J, Smith, TB, Baker, M, Harris, T, and Stephenson, D. Loneliness and social isolation as risk factors for mortality: a meta-analytic review. Perspect Psychol Sci. (2015) 10:227–37. doi: 10.1177/1745691614568352
8. Perissinotto, CM, Cenzer, IS, and Covinsky, KE. Loneliness in older persons: a predictor of functional decline and death. Arch Intern Med. (2012) 172:1078–83. doi: 10.1001/archinternmed.2012.1993
9. Valtorta, NK, Kanaan, M, Gilbody, S, Ronzi, S, and Hanratty, B. Loneliness and social isolation as risk factors for coronary heart disease and stroke: systematic review and meta-analysis of longitudinal observational studies. Heart. (2016) 102:1009–16. doi: 10.1136/heartjnl-2015-308790
10. Beutel, ME, Klein, EM, Brähler, E, Reiner, I, Jünger, C, Michal, M, et al. Loneliness in the general population: prevalence, determinants and relations to mental health. BMC Psychiatry. (2017) 17:1–7. doi: 10.1186/s12888-017-1262-x
11. Kuiper, JS, Zuidersma, M, Voshaar, RCO, Zuidema, SU, van den Heuvel, ER, Stolk, RP, et al. Social relationships and risk of dementia: a systematic review and meta-analysis of longitudinal cohort studies. Ageing Res Rev. (2015) 22:39–57. doi: 10.1016/j.arr.2015.04.006
12. Penninkilampi, R, Casey, AN, Singh, MF, and Brodaty, H. The association between social engagement, loneliness, and risk of dementia: a systematic review and meta-analysis. J Alzheimers Dis. (2018) 66:1619–33. doi: 10.3233/JAD-180439
13. Schrempft, S, Jackowska, M, Hamer, M, and Steptoe, A. Associations between social isolation, loneliness, and objective physical activity in older men and women. BMC Public Health. (2019) 19:1–10. doi: 10.1186/s12889-019-6424-y
14. Canham, SL, Mauro, PM, Kaufmann, CN, and Sixsmith, A. Association of alcohol use and loneliness frequency among middle-aged and older adult drinkers. J Aging Health. (2016) 28:267–84. doi: 10.1177/0898264315589579
15. Ingram, I, Kelly, PJ, Deane, FP, Baker, AL, Goh, MC, Raftery, DK, et al. Loneliness among people with substance use problems: a narrative systematic review. Drug Alcohol Rev. (2020) 39:447–83. doi: 10.1111/dar.13064
16. Foundation for Social Connection. Social connection measurement tools inventory. (2024). Available at: https://www.social-connection.org/social-connection-measurement-tools-inventory (accessed May 1, 2024).
17. Administration for Community Living & USAging. Topical guide: Six considerations for outcome evaluation of social engagement programs serving older adults and people with disabilities. (2024). Available at: https://committoconnect.org/wp-content/uploads/2024/05/CtoC-Outcome-Evaluation-508-links.pdf (accessed May 1, 2024).
18. Smith, ML, Steinman, LE, and Casey, EA. Combatting social isolation among older adults in a time of physical distancing: the COVID-19 social connectivity paradox. Front Public Health. (2020) 8:403. doi: 10.3389/fpubh.2020.00403
19. Russell, DW. UCLA loneliness scale (version 3): reliability, validity, and factor structure. J Pers Assess. (1996) 66:20–40. doi: 10.1207/s15327752jpa6601_2
20. Russell, D, Peplau, LA, and Cutrona, CE. The revised UCLA loneliness scale: concurrent and discriminant validity evidence. J Pers Soc Psychol. (1980) 39:472–80. doi: 10.1037/0022-3514.39.3.472
21. Banerjee, A, Kaur Chawla, S, and Kohli, N. The 100 top-cited studies on loneliness: a bibliometric analysis. Cureus. (2023) 15:e37246. doi: 10.7759/cureus.37246
22. Berkman, LF, and Syme, SL. Social networks, host resistance, and mortality: a nine-year follow-up study of Alameda County residents. Am J Epidemiol. (1979) 109:186–204. doi: 10.1093/oxfordjournals.aje.a112674
23. Peterson, NA, Speer, PW, and McMillan, D. Validation of a brief sense of community scale: confirmation of the principal theory of sense of community. J Community Psychol. (2008) 36:61–73. doi: 10.1002/jcop.20217
24. Campaign to End Loneliness. Measuring your impact on loneliness in later life. (2019). Available at: https://www.campaigntoendloneliness.org/wp-content/uploads/Loneliness-Measurement-Guidance1-1.pdf. (accessed May 1, 2024)
25. AARP Foundation Connect2Affect. Are you affected by social isolation? (2024). Available online at: https://connect2affect.org/assessment/ (accessed May 1, 2024).
26. Connor, KM, and Davidson, JR. Development of a new resilience scale: the Connor-Davidson resilience scale (CD-RISC). Depress Anxiety. (2003) 18:76–82. doi: 10.1002/da.10113
27. Cornwell, EY, and Waite, LJ. Social disconnectedness, perceived isolation, and health among older adults. J Health Soc Behav. (2009) 50:31–48. doi: 10.1177/002214650905000103
28. de Jong-Gierveld, J, and Tilburg, TV. A 6-item scale for overall, emotional, and social loneliness: confirmatory tests on survey data. Res Aging. (2006) 28:582–98. doi: 10.1177/0164027506289723
29. Koenig, HG, Westlund, RE, George, LK, Hughes, DC, Blazer, DG, and Hybels, C. Abbreviating the Duke social support index for use in chronically ill elderly individuals. Psychosomatics. (1993) 34:61–9. doi: 10.1016/S0033-3182(93)71928-3
30. Stalvey, BT, Owsley, C, Sloane, ME, and Ball, K. The life space questionnaire: a measure of the extent of mobility of older adults. J Appl Gerontol. (1999) 18:460–78. doi: 10.1177/073346489901800404
31. Lubben, J, Blozik, E, Gillmann, G, Iliffe, S, von Renteln Kruse, W, Beck, JC, et al. Performance of an abbreviated version of the Lubben social network scale among three European community-dwelling older adult populations. The Gerontologist. (2006) 46:503–13. doi: 10.1093/geront/46.4.503
32. Li, C, Friedman, B, Conwell, Y, and Fiscella, K. Validity of the patient health questionnaire 2 (PHQ-2) in identifying major depression in older people. J Am Geriatr Soc. (2007) 55:596–602. doi: 10.1111/j.1532-5415.2007.01103.x
33. Steptoe, A, Shankar, A, Demakakos, P, and Wardle, J. Social isolation, loneliness, and all-cause mortality in older men and women. Proc Natl Acad Sci. (2013) 110:5797–801. doi: 10.1073/pnas.1219686110
34. Qualtrics. Online sample. (2024). Available at: https://www.qualtrics.com/research-services/online-sample/ (accessed May 1, 2024).
35. Lehdonvirta, V, Oksanen, A, Räsänen, P, and Blank, G. Social media, web, and panel surveys: using non-probability samples in social and policy research. Policy Internet. (2021) 13:134–55. doi: 10.1002/poi3.238
36. Birnbaum, A. Some latent trait models and their use in inferring an examinee's ability In: FM Lord and MR Novick, editors. Statistical theories of mental test scores. Reading, MA: Addison-Wesley (1968)
37. Harris, D. Comparison of 1-, 2-, and 3-parameter IRT models. Educ Meas Issues Pract. (1989) 8:35–41. doi: 10.1111/j.1745-3992.1989.tb00313.x
38. Rasch, G. Probabilistic models for some intelligence and attainment tests. Copenhagen: Danmarks Paedogogiske Institut. (1960) (Chicago: University of Chicago Press, 1980).
39. Chalmers, RP. Mirt: a multidimensional item response theory package for the R environment. J Stat Softw. (2012) 48:1–29. doi: 10.18637/jss.v048.i06
40. Reynolds, AP, Richards, G, de la Iglesia, B, and Rayward-Smith, VJ. Clustering rules: a comparison of partitioning and hierarchical clustering algorithms. J Math Model Algorithms. (2006) 5:475–504. doi: 10.1007/s10852-005-9022-1
41. Schubert, E, and Rousseeuw, PJ Faster k-Medoids clustering: improving the PAM, CLARA, and CLARANS algorithms; (2019). Preprint, doi: 10.1007/978-3-030-32047-8_16
42. Datta, S, and Datta, S. Comparisons and validation of statistical clustering techniques for microarray gene expression data. Bioinformatics. (2003) 19:459–66. doi: 10.1093/bioinformatics/btg025
43. Datta, S, and Datta, S. Methods for evaluating clustering algorithms for gene expression data using a reference set of functional classes. BMC Bioinf. (2006) 7:397. Available at: http://www.biomedcentral.com/1471-2105/7/397
44. Handl, J, Knowles, J, and Kell, DB. Computational cluster validation in post-genomic data analysis. Bioinformatics. (2005) 21:3201–12. doi: 10.1093/bioinformatics/bti517
45. Brock, G, Pihur, V, Datta, S, and Datta, S. clValid: an R package for cluster validation. J Stat Softw. (2008) 25:1–22. doi: 10.18637/jss.v025.i04
46. Embretson, SE. Construct validity: construct representation versus nomothetic span. Psychol Bull. (1983) 93:179–97. doi: 10.1037/0033-2909.93.1.179
47. Cai, L, and Hansen, M. Limited-information goodness-of-fit testing of hierarchical item factor models. Br J Math Stat Psychol. (2013) 66:245–76. doi: 10.1111/j.2044-8317.2012.02050.x
48. Cai, L, and Monro, S. A new statistic for evaluating item response theory models for ordinal data. (2014). National Center for research on evaluation, standards, & student testing. Technical Report.
49. Maydeu-Olivares, A, and Joe, H. Limited information goodness-of-fit testing in multidimensional contingency tables. Psychometrika. (2006) 71:713–32. doi: 10.1007/s11336-005-1295-9
50. Orlando, M, and Thissen, D. Likelihood-based item-fit indices for dichotomous item response theory models. Appl Psychol Meas. (2000) 24:50–64. doi: 10.1177/01466216000241003
51. Kang, T, and Chen, TT. An investigation of the performance of the generalized S–X 2 item-fit index for polytomous IRT models (2007). ACT research report series, 2007-1. ACT, Inc. Available at http://eric.ed.gov/?id=ED510479 (accessed May 1, 2024).
52. García-Escudero, LA, Gordaliza, A, Matrán, C, and Mayo-Iscar, A. A general trimming approach to robust cluster analysis. Ann Stat. (2008) 36:1324–45. doi: 10.1214/07-AOS515
53. Fritz, H, Garcia-Escudero, LA, and Mayo-Iscar, A. Tclust: an R package for a trimming approach to cluster analysis. J Stat Softw. (2012) 47:1–26. doi: 10.18637/jss.v047.i12
54. Bentler, PM. Comparative fit indexes in structural models. Psychol Bull. (1990) 107:238–46. doi: 10.1037/0033-2909.107.2.238
55. Mulaik, SA, James, LR, Van-Alstine, J, and Bennett, N. Evaluation of goodness-of-fit indices for structural equation models. Psychol Bull. (1989) 105:430–45. doi: 10.1037/0033-2909.105.3.430
56. Barreto, M, van Breen, J, Victor, C, Hammond, C, Eccles, A, Richins, MT, et al. Exploring the nature and variation of the stigma associated with loneliness. J Soc Pers Relat. (2022) 39:2658–79. doi: 10.1177/02654075221087190
57. Kerr, NA, and Stanley, TB. Revisiting the social stigma of loneliness. Personal Individ Differ. (2021) 171:110482. doi: 10.1016/j.paid.2020.110482
58. American Psychological Association. Patient Health Questionnaire (PHQ-9 & PHQ-2) (2020). Available at: https://www.apa.org/pi/about/publications/caregivers/practice-settings/assessment/tools/patient-health (accessed May 1, 2024).
Keywords: social disconnectedness, loneliness, social isolation, scale validation, older adult
Citation: Smith ML and Barrett ME (2024) Development and validation of the Upstream Social Interaction Risk Scale (U-SIRS-13): a scale to assess threats to social connectedness among older adults. Front. Public Health. 12:1454847. doi: 10.3389/fpubh.2024.1454847
Received: 25 June 2024; Accepted: 02 September 2024;
Published: 16 September 2024.
Edited by:
Hesham Fathy Gadelrab, Mansoura University, EgyptReviewed by:
Nasser N. Hasan, Kuwait University, KuwaitCopyright © 2024 Smith and Barrett. This is an open-access article distributed under the terms of the Creative Commons Attribution License (CC BY). The use, distribution or reproduction in other forums is permitted, provided the original author(s) and the copyright owner(s) are credited and that the original publication in this journal is cited, in accordance with accepted academic practice. No use, distribution or reproduction is permitted which does not comply with these terms.
*Correspondence: Matthew Lee Smith, bWF0dGhldy5zbWl0aEB0YW11LmVkdQ==
Disclaimer: All claims expressed in this article are solely those of the authors and do not necessarily represent those of their affiliated organizations, or those of the publisher, the editors and the reviewers. Any product that may be evaluated in this article or claim that may be made by its manufacturer is not guaranteed or endorsed by the publisher.
Research integrity at Frontiers
Learn more about the work of our research integrity team to safeguard the quality of each article we publish.