- 1Business School, Northeast Normal University, Changchun, China
- 2College of Electronic Science and Engineering, Jilin University, Changchun, China
Objective: This study explored the impact of prevention and control measures taken by Chinese universities on college students’ academic performance during the COVID-19 pandemic.
Participants: The sample includes 1,009 senior students, 1,140 junior and sophomore students, and 1,198 freshman students studying at a top university in China from 2012 to 2023.
Methods: Analysis of variance was used to analyze historical data, and a learning ability warning system based on probabilistic neural networks was further proposed.
Results: There was a significant difference in student academic performance from 2019 to 2022 compared to historical data. Prevention and control measures such as school closures and online teaching have affected the academic performance of college students.
Conclusion: COVID-19 has a negative impact on college students’ learning ability. It is necessary to establish a learning ability warning system to assist university management departments in formulating relevant policies to restore the learning ability of college students.
1 Introduction
The outbreak of COVID-19 has had a profound impact on people’s production and life. Economic recession and mobility restrictions have had a negative impact on the physical and mental health of most people (1–4). The epidemic has caused great psychological impact on people, exacerbating psychological problems such as obsessive-compulsive disorder, anxiety, and depression (5). As a special group, college students are usually managed in a centralized manner, with food, accommodation, and transportation all within the scope of the school. During the epidemic, universities have taken a series of prevention and control measures (6), such as school closures (7), closed management of dormitories (8), home quarantine (9) and online teaching (10). These measures have led to adverse psychological stress reactions among different populations, including that of college students, in terms of emotions, academic performance, and other aspects (11–14). The impact of epidemic management measures taken by Chinese universities during the epidemic on student academic performance is a question worthy of in-depth research.
Social cognitive theory holds that learning is a process of continuous interaction between humans and their environment, with personal, behavioral, and environmental factors being the determining factors of human learning (15). A good learning environment can ensure students’ self-discipline, communication and interaction, as well as classroom and after-school learning (16). The lockdown measures taken to prevent the spread of the epidemic have suddenly changed the learning environment of students, greatly altering their psychological state and behavioral patterns. The Austrian government’s ban on people using public places has greatly affected the daily lives and overall wellbeing of Austrians (17). Norwegian universities have almost closed all campus activities and adopted online and digital teaching to prevent the spread of the virus (18). The lockdown measures in the UK have led to a deterioration in the mental health of young people, with a survey showing that 30.3% of people had poor mental health and 10.8% had engaged in self harm (11). The epidemic prevention measures in Africa have led to a serious deterioration of the mental health status of more young people. The report showed that 59.1% of the population was anxiety, 57.5% was worry, 51.5% was depressed, and 22.3% was angry (13). After COVID-19, the level of depression among college students has significantly increased (19). The fatigue caused by prolonged online teaching had a significant impact on academic performance. The level of fatigue was negatively correlated with academic performance, with higher levels of fatigue leading to poorer academic performance (20). Early detection of mental health problems among college students can prevent a decline in their learning ability, and implementing relevant measures can help them recover their learning ability. How to establish a mental health assessment system to identify potential mental health problems early is a worthwhile research topic.
Social cognitive theory holds that learning is a process of continuous interaction between humans and their environment, with personal, behavioral, and environmental factors being the determining factors of human learning (15). A good learning environment can ensure students’ self-discipline, communication and interaction, as well as classroom and after-school learning (16). The lockdown measures taken to prevent the spread of the epidemic have suddenly changed the learning environment of students, greatly altering their psychological state and behavioral patterns. The Austrian government’s ban on people using public places has greatly affected the daily lives and overall wellbeing of Austrians (17). Norwegian universities have almost closed all campus activities and adopted online and digital teaching to prevent the spread of the virus (18). The lockdown measures in the UK have led to a deterioration in the mental health of young people, with a survey showing that 30.3% of people have poor mental health and 10.8% have engaged in self harm (11). The epidemic prevention measures in Africa have led to a serious deterioration of the mental health status of more young people. The report shows that 59.1% of the population presents anxiety, 57.5% presents worry, 51.5% is depressed, and 22.3% is angry (13). After COVID-19, the level of depression among college students has significantly increased (19). The fatigue caused by prolonged online teaching has a significant impact on academic performance. The level of fatigue is negatively correlated with academic performance, indicating that the higher the level of fatigue, the worse the academic performance (20). Early detection of mental health problems among college students can prevent a decline in their learning ability, and implementing relevant measures can help them recover their learning ability. How to establish a mental health assessment system to identify potential mental health problems early is a worthwhile research topic.
Psychological health assessment helps identify potential mental health issues, and evaluation methods include standardized psychological assessment tools (21), statistical analysis (2, 22), model analysis (1, 4, 23–30), and robot learning (31–40). Kettler et al. developed an assessment scale to measure the progress of graduate students in psychology courses in terms of abilities. The content of this scale is based on the Health Service Psychology Accreditation Standards of the American Psychological Association (APA), and uses the Rutgers University Psychology Student Evaluation Tool (RIESP) to analyze the progress of graduate students in terms of abilities (21). However, using statistical analysis of mental health is the simplest and most direct method, and existing evaluation tools cannot meet the needs of mental health analysis in all scenarios. In order to analyze the impact of different factors on mental health, statistical variables were introduced into the evaluation process. Van Der Horst et al. used specified correlation values to reflect the insecurity, anxiety, and pain of spinal surgery patients (22). Mead et al. used a single sample t-test to verify that the measurement of happiness in the current sample based on the Warwick Edinburgh Mental Health Scale significantly decreased (2). The statistical analysis method can provide qualitative analysis basis for mental health from a variable perspective based on the characteristics of statistics.
Model analysis methods can meet the qualitative analysis of mental health from multiple information and perspectives. Rosas Fuentes et al. designed an explanatory model for depression and suicidal ideation based on irrational beliefs of psychological students using multivariate analysis (23). Huang et al. used decision tree models and Kendall correlation analysis to establish a psychological problem prediction model based on objective behavioral data of college students (24). Yao constructed and tested various health indicators of the two factor liquidity model, verifying the applicability of the psychological health two factor liquidity model in the student population (25). Antaramian used a two factor mental health model to analyze the subjective wellbeing and clinical symptom levels of college students, and examined group differences in student engagement and academic performance (26). Tejada Gallardo et al. used network analysis to explore the restructuring of the relationship between school based mental health indicators after MPPI (27). Kim et al. used the Contrastive Avoidance Model (CAM) to verify that concerns increase negative impacts and decrease positive impacts (28). Ye et al. constructed a sustainable happiness model based on emotion action experience outcome using the PERMA model, which showed that positive emotions have a positive impact on engagement and achievement (29). Uzir et al. analyzed the psychological distress of small business owners: depression, anxiety, and stress (DASS-21 scale) using Partial Least Squares Structural Equation Modeling (PLS-SEM). The research results indicate that during the outbreak of COVID-19, DASS-21 parameters and fear of business losses can affect psychological distress (30). Stanculescu et al. examined the psychometric characteristics of the Romanian version of the COVID-19 Fear Scale (FCV-19S) based on classical testing theory and the graded response model, emphasizing the negative correlation between resilience, happiness, and fear in the context of the COVID-19 pandemic (4). Fazia et al. focused on the adverse effects of social isolation on mental health and wellbeing, and used a linear mixed effects model to test the beneficial effects of Integrated Medication (IM) on happiness, perceived stress, and state anxiety (1). Model analysis can address qualitative analysis issues related to specific mental health. However, the multiple parameters of mental health indicators are usually mutual inductance and non-linear, so using model methods cannot further analyze mental health issues in depth.
The prediction of mental health is a non-linear problem, and machine learning can solve complex causal relationships. Wang et al. proposed a multi-layer attention convolutional neural network (ACNN) for text sentiment analysis based on hierarchical CNN. The CNN validated the proposed model’s good positive psychological classification performance in experiments through online physical education teaching resources (31). Yang et al. conducted a detailed analysis and research on the mental health status of college students using data mining clustering methods, and designed an anomaly mining algorithm based on clustering to quickly discover health problems in abnormal data (32). Collins et al. used machine learning to predict individual responses to network-based PPI. Tests have shown that machine learning can be used to predict changes in results of web-based PPI, and it has important clinical significance for matching individuals with PPI based on personal characteristics (33). Sun et al. have developed a long-term and ubiquitous interpretable psychological computing model based on prior knowledge and multimodal information fusion. This model achieves advanced accuracy in basic and complex emotion detection on the proposed mental health database, effectively solving the scientific and accuracy related problems in long-term recognition and prediction of complex mental health states (34). Pan et al. established an emotion classification model to annotate 105,536 epidemic comments on the official Weibo account of People’s Daily within 86 days (positive and negative). The test results showed that the accuracy of the model reached 88%, and the AUC value was greater than 0.9 (3). Li established a DNN based network model for predicting the mental health of enterprise employees. The key influencing factors in mental health assessment are used as input vectors for DNN, and the DNN network model is used to analyze which are the main factors affecting the mental health of enterprise employees. After sample training, the error between the predicted and measured values of DNN was only 3.55%, achieving the expected effect (35). Yao proposed a psychological state analysis model for college students based on BP neural network to analyze the impact of COVID-19 on the mental health of college students. Based on the behavior and psychological characteristics of college students, relevant survey results were obtained through event monitoring, early warning, and daily performance, and a relationship model between the psychological state of college students and the epidemic was constructed based on BP neural network (36). Luo proposed a fuzzy comprehensive evaluation based on BP algorithm and a mental health prediction system for impoverished college students. Using the International Mental Health Scale SCL-90 and considering the mental health status of impoverished college students, an optimized BP algorithm was selected to establish a mental health prediction model, which was implemented and compared with other models to demonstrate its superiority (37). Liu combined psychological knowledge and machine learning methods to propose a psychological crisis warning algorithm based on educational data, which predicts the duration and intensity of emotions by calculating stress events and emotional decay (38). Lin et al. established a CNN based prediction model to predict the tendencies of public emotions and mental health. The proposed method can accurately determine the emotional tendencies of online users (39). Wang et al. constructed a multi task learning model based on pre trained models to detect depression and identify academic performance distortions (40). Machine learning has become a powerful tool for studying mental health. Machine learning can be used to analyze various potential factors, such as school closures, dormitory closures, and online teaching, and their impact on students’ academic performance and mental health. Therefore, this article studies the construction of a college student academic performance warning system based on machine learning methods, which helps schools understand students’ mental health levels from the perspective of their academic performance.
The anxiety caused by the pandemic had a negative impact on students’ academic performance (41). A cross-sectional study was conducted on college students in Anhui Province, China from May to June 2022, and the results showed that academic stress had a significant impact on depression among college students in the context of COVID-19 (p < 0.01); College students who have not served as student cadres were more affected by academic pressure (p < 0.05); The attitude of college students toward COVID-19 significantly affected depression (p < 0.01) (42). A longitudinal study was conducted on Chinese university students from October 2019 to October 2020, and the results showed a significant decline in critical thinking skills and personality from before to after the lockdown. After the lockdown, perceived academic achievements and critical thinking exhibited greater variability. In addition, those who were more susceptible to emotional distress during the pandemic performed poorly in learning outcomes after lockdown (43). The above research indicates that the pandemic has had a negative impact on the academic performance of college students. The cross-sectional and longitudinal studies on Chinese university students support this conclusion, but the longitudinal study only lasted for 2 years, which is insufficient time.
In order to compensate for the short duration of longitudinal research, the data is analyzed in this paper spans from 2012 to 2023. In order to study the impact of the COVID-19 pandemic on the academic performance of college students, this paper first used the ANOVA method to conduct a longitudinal analysis of the final results, to analyze the impact of the epidemic on college students’ academic performance. Secondly, a learning achievement warning system based on probabilistic neural networks was proposed. The data comes from a certain university in China and is the final grades of three professional courses in a certain engineering major, covering the period from 2012 to 2023. The analysis results of this study support the conclusions of (42) and (43). This study provide a reference method for the management of psychological crises among college students, and have a guiding role in the formulation of policies for schools to respond to sudden public health emergencies.
2 Academic performance issues among college students in the context of the epidemic
2.1 Psychological environment of college students against the background of the epidemic
Through a survey of students’ mental health and personality traits, it was found that the mental health problems of college students mainly focus on five factors: obsessive-compulsive symptoms, emotional imbalance, learning pressure, maladaptation, interpersonal tension and sensitivity. The COVID-19 pandemic has had a great impact on the psychology of college students, exacerbating psychological problems such as obsessive-compulsive disorder, anxiety, depression, interpersonal sensitivity, and also bringing a series of academic performance problems (44).
Firstly, the risk of COVID-19 infection has caused anxiety among college students, with students wearing masks, maintaining social distance, reducing social interactions, and even staying indoors (45). Some college students were unable to study and live normally, resulting in excessive stress reactions. Secondly, during the epidemic, universities had taken strict epidemic prevention measures, but the epidemic continued to spread. College students were passive and weak, feeling powerless and helpless. Finally, the pandemic lasting for 3 years has led to ineffective relief of anxiety, causing college students to experience persistent psychological states such as low mood, pessimism, and helplessness, and their anxiety turns into depression (46, 47). In addition, COVID-19 caused fear among college students, leading them to be afraid to go out, study in the classroom, and interact with others (48). Long term excessive psychological problems such as anxiety, depression, fear, and helplessness among college students not only harmed their physical and mental health, but also affected their academic performance and have a significant negative impact on education and teaching (49, 50).
2.2 Learning environment for college students against the background of the epidemic
In order to prevent the COVID-19 epidemic, universities have strengthened centralized management and adopted closed management methods for them, such as closed management of dormitories (8), campus restrictions (7), cafeteria restrictions on dine in, etc. These measures have intensified the fear of the epidemic among college students and further reduced their social interactions.
The sudden closed management has changed the learning and living environment of college students, breaking their study and living habits. The closed management model of the school restricted students’ learning space, resulting in them only being able to study and live in the dormitory. Faced with academic pressure, students were unable to alleviate it through methods such as jogging, swimming, watching movies, and listening to music (51). These measures had led to college students being irritable, impulsive, and emotionally unstable, resulting in adverse psychological stress reactions in cognitive aspects (12, 14, 41).
2.3 Teaching methods for college students in the context of the epidemic
In order to stop the spread of COVID-19 to the campus and ensure the safety of teachers and students, the Ministry of Education issued the Guidance of the Leading Group Office of the Ministry of Education in Response to the novel coronavirus Pneumonia Epidemic on Doing a Good Job in the Online Teaching Organization and Management of Ordinary Colleges and Universities during the Period of Epidemic Prevention and Control, calling on all schools around the country to carry out the work of “suspending classes and schools.” It is required to strictly implement the requirements of the technical scheme for the prevention and control of COVID-19 in colleges and universities, primary and secondary schools, and kindergartens issued by the National Health Commission and the Ministry of Education, implement the plan efficiently, and win the first opportunity with speed. According to the requirements of the Ministry of Education, universities have introduced a series of online teaching measures, allowing teachers and students to quickly immerse themselves in unprecedented online teaching models.
Online teaching has changed the learning habits of college students, advocating for self-directed and conscious learning. Under the epidemic prevention and air defense, online teaching in universities included two methods: online teaching at home and online teaching on campus. Home based online teaching adopted a method of home isolation, completing online teaching at home. Compared to traditional classroom teaching, this teaching method lacks a learning atmosphere and requires students to follow the teacher’s teaching process independently, complete knowledge learning in the classroom, and review after class. Online teaching on campus adopts the method of school isolation, and online teaching is completed in the dormitory. Compared to online teaching from home, this teaching method has more stringent restrictions on students. The learning space of students is limited to a smaller space. Classroom knowledge learning and post class review were all completed in the dormitory. Online teaching has brought certain impacts to students, and most students were unable to adapt to the online teaching mode, resulting in certain psychological health problems, including irritability, anxiety, and other emotions (52).
The learning patterns cannot be easily changed, and students’ learning and living habits cannot be easily changed. In response to COVID-19 prevention and control, universities have introduced some policies that have changed the learning and teaching environment of college students, affected their mental health, and had a certain negative impact on their cognition (53).
3 The impact of COVID-19 pandemic on academic performance of college students
3.1 Data collection
The data comes from a top Chinese university, spanning from 2012 to 2023. These data are the final grades of three major courses for students majoring in engineering at the university. This paper replaces a certain university with the symbol HU, and three engineering courses with the symbols G1, G2, and G3. In the teaching and training plan, G1 is offered for senior students, G2 is offered for junior and sophomore students, and G3 is offered for freshman students. Normally, the number of students for G1, G2, and G3 courses is approximately 100 per year. The statistical data of the students participated in G1, G2, and G3 courses are listed in Table 1, and the statistical data for G1, G2, and G3 courses are listed in Table 2. Note: 1. Due to the adjustment of the teaching syllabus in a certain year, there had been a decrease in the number of elective students for G1 course. 2. In the spring semester of 2013–2014, the teacher of the G2 course went abroad for further studies, so the grades for G2 are missing in Table 2. This is considering that fluctuations in student performance may be influenced by different teachers.
G1, G2, and G3 were courses in the same major’s training plan, and their teaching targets were the same year students, but they were implemented in different academic years. In order to ensure comparability of students in each course (G1, G2, G3) at baseline, this study used randomization methods for analysis of variance and significant differences in academic performance. The specific method is as follows: for each course sample, repeat 100 times using Monte Carlo random sampling, and randomly select 100 samples each time. SPSS software was used to average 100 datasets.
3.2 Analysis of variance
One-factor analysis of variance was used to examine the differences in academic performance among college students, according to the year of study of the course. The statistical analysis software is SPSS, and the significance level of One-factor ANOVA is set at 0.05.
The analysis results of G1, G2, and G3 are shown in Tables 3–6. Table 2 shows the analysis of variance results for G1, G2, and G3. Table 4 shows that there is a significant difference in G1 scores in the 2020–2021 academic year compared to other academic years. Figure 1 shows that the average grade of G1 in the 2020–2021 academic year is the lowest in history. Table 5 shows that there is a significant difference in the final grades of G2 in academic years 2019–2020, 2020–2021, and 2021–2022 compared to the final grades of other academic years. Table 5 shows that the average score of G2 in these three academic years has reached the lowest level in history. Table 6 shows that there is no significant difference in G3 scores for different academic years (Figure 2).
4 Construction of the learning ability warning system based on probabilistic neural networks
Probabilistic Neural Network (PNN) is a pattern classification neural network model based on statistical principles. This section constructs a learning ability classification system based on probabilistic neural networks to analyze whether there are abnormalities in the learning ability level of college students. This paper divides the learning ability of college students into three levels: normal, degraded, and improved, represented by . The structure of the designed probabilistic neural network is shown in Figure 3, which includes five layers: input layer, feature layer, pattern layer, summation layer, and output layer. The input layer receives the grades, and is set as . Let , then represents the grades of 100 students. Feature layer extracts feature data from the grades, and is set as . Let , then represents the maximum, minimum, mean, and standard deviation, respectively. The main function of the pattern layer is to weight and sum the signals output by the feature layer, and send them to the next layer through an activation function. In this paper, the activation function is taken as a Gaussian function. The pattern layer is set as , where represents the number of neurons in the pattern layer, and is the same as the number of training samples. The sum layer is set as , where represents the number of neurons in the sum layer, and is the same as the class value of the training sample. In this system, , then . Finally, the output layer outputs the classification of the sample according to the maximum probability criterion. The definition of is shown in Table 7.
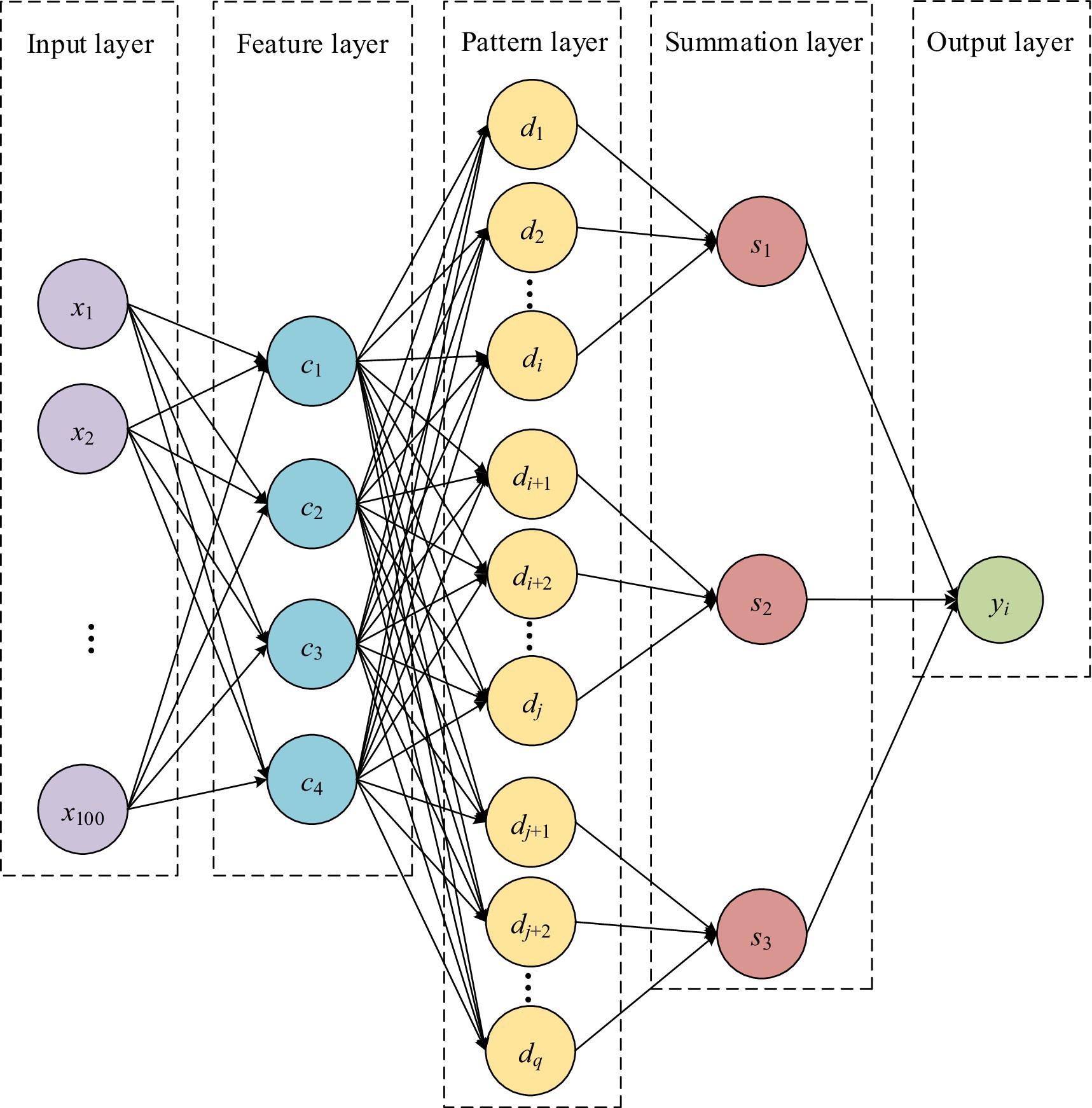
Figure 3. Structure diagram of the learning ability classification system based on probabilistic neural network.
The probabilistic neural network for learning ability classification was trained using the final grades of G1, G2, and G3 courses as training data. Due to the different levels of difficulty and teachers in G1, G2, and G3, PNN were trained for each of them, and the training process was the same. During the period of 2012–2023, a total of 1,009 students participated in the course and obtained final grades. These data were used as samples to train probabilistic neural networks. Randomly select 100 out of 1,009 sample data as one sample. Extract 100 times to obtain 100 samples. The ratio of training to testing sets is 7:3. The training results of PNN for G1 are shown in Figure 4A, and the test results are shown in Figure 4B. The PNN was used to classify the ability of college students to learn G1, and the classification results are shown in Table 8.
During the period of 2012–2023, a total of 1,140 students participated in the course and obtained final grades. These data were used as samples to train probabilistic neural networks. Randomly select 100 out of 1,140 sample data as one sample. Extract 100 times to obtain 100 samples. The ratio of training to testing sets is 7:3. The training results of PNN for G2 are shown in 0 (a), and the test results are shown in 0 (b). The PNN was used to classify the ability of college students to learn G2, and the classification results are shown in Table 8.
During the period of 2012–2023, a total of 1,198 students participated in the course and obtained final grades. These data were used as samples to train probabilistic neural networks. Randomly select 100 out of 1,198 sample data as one sample. Extract 100 times to obtain 100 samples. The ratio of training to testing sets is 7:3. The training results of PNN for G3 are shown in 0 (a), and the test results are shown in 0 (b). The PNN was used to classify the ability of college students to learn G3, and the classification results are shown in Table 8.
5 Discussion
This study investigated the impact of the COVID-19 pandemic on the academic performance of college students. The research sample includes the final grades of three courses for freshmen, sophomores, juniors, and seniors, with a time span of 11 calendar years from 2012 to 2023. The data can reflect the learning ability of students under the background of no epidemic and COVID-19 epidemic (Figures 5, 6).
The researchers noticed that COVID-19 would affect the academic performance of college students (54–57). During the COVID-19 pandemic, the increase in workload for college students, concerns about successfully completing their studies, changes in teaching methods, and the shift toward remote learning have all caused physical and psychological pressure (55). Compared with before the epidemic, academic fatigue, learning engagement, and education satisfaction have all changed, and student happiness and education satisfaction related to learning have decreased by 8% (56). The closure of university campuses and other social isolation measures have had a significant impact on students’ learning environment. Isolation measures have prevented students from participating in regular social activities such as group discussions, quizzes, and brief breaks during lectures, leading to psychological and academic issues (57). In a closed environment, individuals with low psychological resilience may be more emotionally sensitive, and the overall severity of confinement is negatively correlated with depression (58). During the COVID-19 pandemic, most students experienced moderate to high levels of stress related to COVID-19. In addition, some senior students believed that online teaching was a poor choice compared to traditional learning, and their satisfaction with online teaching was relatively low (52). This study provides further evidence for the adverse effects of epidemic prevention measures taken by universities on their academic performance. In our study, the academic performance of second and third grade students was significantly affected by the pandemic. The long-term implementation of epidemic prevention measures (at least 2 years) has greatly affected the mental health level of sophomore, junior, and senior students, leading to a decline in learning ability, which is consistent with (52).
Compared with sophomore, junior, and senior students, the learning ability of freshmen is almost unaffected by the epidemic, which is consistent with (52). This may be due to their new learning environment and unfamiliarity with traditional teaching practices of teachers. The epidemic prevention measures taken by universities, such as campus closures, dormitory closures, and online teaching, have a relatively short implementation time (less than 1 year) for freshmen, resulting in a relatively small impact on their mental health.
In our research, the impact of the epidemic on students’ learning ability comes from objective final grade data. Our research findings confirm that the COVID-19 pandemic has had a negative impact on the learning ability of university students. This conclusion is consistent with the subjective data obtained from the questionnaire survey (59, 60). This is conducive to establishing a learning ability warning system and providing assistance for the formulation of relevant policies. The study also indicates that the designed learning ability warning system based on probabilistic neural networks can predict the learning ability of college students.
This study objectively analyzed the impact of the COVID-19 pandemic on students’ academic performance based on historical data. A student learning ability warning system was constructed using student grades as input variables for the warning system. Compared to the questionnaire survey, the data sample selected in this study has objectivity. Questionnaire survey analysis can reflect the subjective feelings of different groups of people, including college students, toward the COVID-19 pandemic, such as psychological stress, low mood, depression, and anxiety. The questionnaire survey of online teaching can provide feedback information on college students’ satisfaction with online courses, online teaching styles, etc., for research and investigation. However, cross-sectional studies such as questionnaire surveys designed for the COVID-19 pandemic have hindered the inference of causal relationships. The data for this study is from 2012 to 2023, and longitudinal data is beneficial for inferring causal relationships. In addition, objective data analysis is essential for understanding the impact of the COVID-19 pandemic on overall learning success, mental health, and new teaching methods. This study complements this work by objectively analyzing the impact of COVID-19 on college students’ academic performance and building a learning ability early warning system to predict students’ learning situation.
But this study also has limitations. Firstly, our research sample lacks information on the mental and physical condition of participants, which makes it impossible to analyze the causes of certain special situations. Secondly, the sample for this study is only from engineering students, and further research should include students from different majors and universities to analyze the differences between different majors and regions. Finally, this warning system does not include subjective variables such as stress, anxiety, and sleep quality, which should be considered in subsequent research.
The results of this study indicate that sudden changes in teaching methods have affected the learning ability of college students, leading to a decline in their academic performance. The warning system constructed by the research institute provides managers with the current learning status of students based on historical data of their grades, avoiding the problems of long questionnaire survey cycles and poor real-time performance. This provides a feasible method for early detection of changes in students’ learning abilities.
6 Conclusion
The epidemic prevention and control measures in universities have effectively controlled the spread of COVID-19 (61). Although college students have well endured the demands of school closures, closed management of dormitories and online teaching, their mental health has been affected to varying degrees. In order to prevent the decline of learning ability among college students, a learning ability warning system should be established to detect problems as early as possible and implement relevant measures to help college students recover their learning ability.
Data availability statement
The raw data supporting the conclusions of this article will be made available by the authors without undue reservation.
Author contributions
ZR: Conceptualization, Investigation, Methodology, Supervision, Writing – original draft, Writing – review & editing. QD: Conceptualization, Data curation, Methodology, Writing – review & editing.
Funding
The author(s) declare that financial support was received for the research, authorship, and/or publication of this article. This research was supported by Jilin Province Higher Education Teaching Reform Research Project (No. JLJY202282048802) and Jilin Provincial Department of Education’s 13th Five Year Plan Social Science Project (No. JJKH20147961SK).
Conflict of interest
The authors declare that the research was conducted in the absence of any commercial or financial relationships that could be construed as a potential conflict of interest.
Publisher’s note
All claims expressed in this article are solely those of the authors and do not necessarily represent those of their affiliated organizations, or those of the publisher, the editors and the reviewers. Any product that may be evaluated in this article, or claim that may be made by its manufacturer, is not guaranteed or endorsed by the publisher.
Supplementary material
The Supplementary material for this article can be found online at: https://www.frontiersin.org/articles/10.3389/fpubh.2024.1454406/full#supplementary-material
References
1. Fazia, T, Bubbico, F, Nova, A, Riggi, E, Caimi, G, Calgan, B, et al. Online short-term mindfulness-based intervention during COVID-19 quarantine in Italy: effects on wellbeing, stress, and anxiety. Front Psychol. (2022) 13:914183. doi: 10.3389/fpsyg.2022.914183
2. Mead, JP, Fisher, Z, Tree, JJ, Wong, PTP, and Kemp, AH. Protectors of wellbeing during the COVID-19 pandemic: key roles for gratitude and tragic optimism in a UK-based cohort. Front Psychol. (2021) 12:647951. doi: 10.3389/fpsyg.2021.647951
3. Pan, W, Wang, RJ, Dai, WQ, Huang, G, Hu, C, Pan, WL, et al. China public psychology analysis about COVID-19 under considering Sina Weibo data. Front Psychol. (2021) 12:713597. doi: 10.3389/fpsyg.2021.713597
4. Stanculescu, E. Fear of COVID-19 in Romania: validation of the Romanian version of the fear of COVID-19 scale using graded response model analysis. eCOMMUNITY. (2022) 20:1094–109. doi: 10.1007/s11469-020-00428-4
5. Pérez-Escobar, JA, Carreno, DF, Pérez-Escobar, R, and Eisenbeck, N. Sexual and mental health in healthcare workers during the COVID-19 outbreak: exploring the role of meaning-centered coping. Policy. (2024) 21:1086–99. doi: 10.1007/s13178-024-00963-y
6. Luo, YF, Shen, HY, Yang, SC, and Chen, LC. The relationships among anxiety, subjective well-being, media consumption, and safety-seeking behaviors during the COVID-19 epidemic. IJERPH. (2021) 18:13189. doi: 10.3390/ijerph182413189
7. Rotvold, A, Parker, K, Honrath, K, and Rhee, Y. Sleep and diet patterns of college students during the COVID-19 pandemic lockdowns. J Am Coll Heal. (2024) 72:1692–5. doi: 10.1080/07448481.2022.2089850
8. Sebong, PH, Tjitradinata, C, and Goldman, RE. Promoting COVID-19 prevention strategies in student dormitory setting: a qualitative study. J Am Coll Heal. (2023) 71:1397–406. doi: 10.1080/07448481.2021.1926271
9. Shanahan, ML, Fischer, IC, Rogers, SK, and Rand, KL. Coping with COVID-19: a snapshot of college student mental health, coping, and expectancies during stay-at-home orders. J Am Coll Heal. (2024) 72:451–62. doi: 10.1080/07448481.2022.2039670
10. Hargreaves, A, Loughnane, G, Nguyen, H, and Mothersill, D. Online learning predictors of mental health in third-level students during the COVID-19 pandemic in Ireland. J Am Coll Heal. (2024) 72:1778–84. doi: 10.1080/07448481.2022.2089852
11. Dewa, LH, Crandell, C, Choong, E, Jaques, J, Bottle, A, Kilkenny, C, et al. CCopeY: a mixed-methods coproduced study on the mental health status and coping strategies of young people during COVID-19 UK lockdown. J Adolesc Health Care. (2021) 68:666–75. doi: 10.1016/j.jadohealth.2021.01.009
12. Archibong, V, Usman, IM, Kasozi, KI, Aigbogun, EO Jr, Josiah, I, Monima, AL, et al. Anxiety, anger and depression amongst low-income earners in southwestern Uganda during the COVID-19 Total lockdown. Front Public Health. (2021) 9:590458. doi: 10.3389/fpubh.2021.590458
13. Langsi, R, Osuagwu, UL, Goson, PC, Abu, EK, Mashige, KP, Ekpenyong, B, et al. Prevalence and factors associated with mental and emotional health outcomes among Africans during the COVID-19 lockdown period—a web-based cross-sectional study. IJERPH. (2021) 18:899. doi: 10.3390/ijerph18030899
14. Zeng, YL, Sun, WX, Wang, M, Wei, JX, Sun, GC, and Hu, JS. The association between online class-related anxiety and academic achievement among undergraduates. Soc Behav Personal. (2023) 51:e12462:1–13. doi: 10.2224/sbp.12462
15. Bandura, A. Social cognitive theory of mass communication. Media Psychol. (2001) 3:265–99. doi: 10.1207/S1532785xmep0303_03
16. Zhu, JQ, Zhao, HX, Wang, X, Yang, L, Qin, ZY, and Geng, JC. Effects of online learning on college students in eastern China: a structural equation model. Front Public Health. (2022) 10:853928. doi: 10.3389/fpubh.2022.853928
17. Gugerell, K, and Netsch, S. Reflection on the Austrian newspaper coverage of the role and relevance of urban open-and green-spaces in Vienna during the first COVID-19 lockdown in 2020. Displays. (2020) 56:54–63. doi: 10.1080/02513625.2020.1906051
18. Megías-Robles, A, Gutiérrez-Cobo, MJ, Cabello, R, Gómez-Leal, R, and Fernández-Berrocal, P. A longitudinal study of the influence of concerns about contagion on negative affect during the COVID-19 lockdown in adults: the moderating effect of gender and resilience. J Health Psychol. (2022) 27:1165–75. doi: 10.1177/1359105321990794
19. Morris, JIII, Morris, B, and Briars, A. COVID shelter in place orders and mental health outcomes among college undergraduates. J Am Coll Heal. (2023) 71:2530–7. doi: 10.1080/07448481.2021.1978459
20. Chen, Y, and Qin, XQ. Student fatigue and its impact on teaching effectiveness based on online teaching. Educ Inf Technol. (2024) 29:10177–200. doi: 10.1007/s10639-023-12197-3
21. Kehler, RJ, Forman, SG, Feeney-Kehler, KA, and Haboush, KL. Development and initial validation of the Rutgers instrument for evaluation of students in psychology. Train Educ Prof Psychol. (2018) 12:154–62. doi: 10.1037/tep0000200
22. Van Der Horst, A, Bohlmeijer, ET, Schreurs, KMG, and Kelders, SM. Strength Back – a qualitative study on the co-creation of a positive psychology digital health intervention for spinal surgery patients. Front Psychol. (2023) 14:1117357. doi: 10.3389/fpsyg.2023.1117357
23. Rosas-Fuentes, PD, Valdés-García, KP, Monroy-Velasco, IR, Pérez-Pedraza, BD, and Sánchez-Loyo, LM. Depression, suicide ideation, and irrational beliefs: explanatory models in psychology students. Salud Ment. (2023) 46:61–7. doi: 10.17711/Sm.0185-3325.2023.009
24. Huang, YP, Li, S, Lin, B, Ma, S, Guo, JA, and Wang, CL. Early detection of college students’ psychological problems based on decision tree model. Front Psychol. (2022) 13:946998. doi: 10.3389/fpsyg.2022.946998
25. Yao, SG. Construction of action model of Students’ mental health education system based on positive psychology. Rev Psicol Deporte. (2020) 29:90–102.
26. Antaramian, S. Assessing psychological symptoms and well-being. J Psychoeduc Assess. (2015) 33:419–29. doi: 10.1177/0734282914557727
27. Tejada-Gallardo, C, Blasco-Belled, A, and Alsinet, C. Changes in the network structure of mental health after a multicomponent positive psychology intervention in adolescents: a moderated network analysis. Appl Psychol Health Well Being. (2022) 14:987–1003. doi: 10.1111/aphw.12363
28. Kim, H, and Newman, MG. Worry and rumination enhance a positive emotional contrast based on the framework of the contrast avoidance model. J Anxiety Disord. (2023) 94:102671. doi: 10.1016/j.janxdis.2023.102671
29. Ye, JH, Wu, YT, Wu, YF, Chen, MY, Nong, WGJ, and Lee, YS. A study on the construction and validation of pathways to the sustainable well-being of Chinese vocational students in the post-epidemic era. Curr Psychol. (2024) 43:7511–25. doi: 10.1007/s12144-023-04954-x
30. Uzir, MUH, Bukari, Z, Jerin, I, Hasan, N, Hamid, AA, and Rannayah, T. Impact of COVID‐19 on psychological distress among SME owners in Ghana: partial least square–structural equation modeling (PLS‐SEM) approach. J Community Psychol. (2022) 50:1282–314. doi: 10.1002/jcop.22716
31. Yang, SB, Lin, L, and Zhang, X. Adjustment method of college students’ mental health based on data analysis under the background of positive psychology. Front Psychol. (2022) 13:921621. doi: 10.3389/fpsyg.2022.921621
32. Wang, W, and Hu, J. Extraction of PE online teaching resources with positive psychology based on advanced intelligence algorithm. Front Psychol. (2022) 13:948721. doi: 10.3389/fpsyg.2022.948721
33. Collins, AC, Price, GD, Woodworth, RJ, and Jacobson, NC. Predicting individual response to a web-based positive psychology intervention: a machine learning approach. J Posit Psychol. (2024) 19:675–85. doi: 10.1080/17439760.2023.2254743
34. Sun, X, Song, YZ, and Wang, M. Toward sensing emotions with deep visual analysis: a long-term psychological modeling approach. IEEE Multimedia. (2020) 27:18–27. doi: 10.1109/Mmul.2020.3025161
35. Li, X. Psychological health assessment model of enterprise employees based on DNN technology. Wirel Commun Mob Comput. (2022) 2022:1–9. doi: 10.1155/2022/4824038
36. Yao, SG. Construction of relationship model between college students’ psychological status and epidemic situation based on BP neural network. Comput Intell Neurosci. (2022) 2022:5115432. doi: 10.1155/2022/5115432
37. Luo, L. The practice of psychological well-being education model for poor university students from the perspective of positive psychology. Front Psychol. (2022) 13:951668. doi: 10.3389/fpsyg.2022.951668
38. Liu, LX. The influence of ideological education on students’ mental health during the pandemic: an empirical analysis based on big data and intelligent model. Front Psychol. (2022) 13:940770. doi: 10.3389/fpsyg.2022.940770
39. Lin, HF, and Bu, NQ. A CNN-based framework for predicting public emotion and multi-level behaviors based on network public opinion. Front Psychol. (2022) 13:909439. doi: 10.3389/fpsyg.2022.909439
40. Wang, BC, Zhao, YY, Lu, X, and Qin, B. Cognitive distortion based explainable depression detection and analysis technologies for the adolescent internet users on social media. Front Public Health. (2023) 10:1045777. doi: 10.3389/fpubh.2022.1045777
41. Edo, GI, Onoharigho, FO, Nwosu, LC, Jikah, AN, Marris, DM, Akpoghelie, PO, et al. Adapting to change: exploring the impact of the COVID-19 pandemic on academic performance in African students and the role of virtual learning as a substitute. J Contin High Educ. (2024):1–17. doi: 10.1080/07377363.2024.2381263
42. Chen, BL, Wang, WW, and Yang, SL. The relationship between academic stress and depression among college students during the COVID-19 pandemic: a cross-sectional study from China. BMC Psychiatry. (2024) 24:46. doi: 10.1186/s12888-024-05506-8
43. Lv, XJ, Ma, JJ, Brinthaupt, TM, Zhao, SC, and Ren, XZ. Impacts of university lockdown during the coronavirus pandemic on college students’ academic achievement and critical thinking: a longitudinal study. Front Psychol. (2022) 13:995784. doi: 10.3389/fpsyg.2022.995784
44. Garcia, C, Ayala, JA, Roldan, KD, and Bavarian, N. Exploring Reddit conversations about mental health difficulties among college students during the COVID-19 pandemic. J Am Coll Heal. (2024) 72:2419–25. doi: 10.1080/07448481.2022.2115297
45. Wang, ZE, and Bao, SS. The impact of social distancing measures (quarantine) policy on tertiary education and medical consultations in China during the COVID-19 pandemic. Front Public Health. (2024) 12:1365805. doi: 10.3389/fpubh.2024.1365805
46. Thiria, E, Pellegrini, C, Kase, BE, DeVivo, K, and Steck, SE. Health behavior and anxiety changes during the COVID-19 pandemic among students, faculty, and staff at a US university. J Am Coll Heal. (2024) 72:2180–7. doi: 10.1080/07448481.2022.2104615
47. Vlacha, V, and Perivolaropoulou, P. Changes in dietary, lifestyle habits and mood in college students during the COVID-19 pandemic: a survey distributed across Greek universities. J Am Coll Heal. (2024):1–8. doi: 10.1080/07448481.2023.2299428
48. Baba, Z, Kienle, S, and Edelblute, HB. Using COVID-19 online learning modules to examine concerns of university students returning to in-person learning: a mixed-methods study. J Am Coll Heal. (2024):1–9. doi: 10.1080/07448481.2023.2299407
49. Shrestha, NR, Deason, RG, Cordaro, M, Howard, K, and Haskard-Zolnierek, K. Evaluating the relationship of empathic concern to college students’ responses to the COVID-19 pandemic. J Am Coll Heal. (2023):1–7. doi: 10.1080/07448481.2023.2224432
50. Quattrone, F, Borghini, A, Emdin, M, and Nuti, S. Protecting higher education institutions from COVID-19: insights from an Italian experience. J Am Coll Heal. (2022) 70:1354–5. doi: 10.1080/07448481.2020.1791885
51. Petersen, CB, Krüger, C, Guldager, JD, Algren, MH, Jervelund, SS, and Berg-Beckhoff, G. Are changes in physical activity during COVID-19 associated with mental health among Danish university students? Front Public Health. (2023) 11:1126240. doi: 10.3389/fpubh.2023.1126240
52. Tomic, SD, Tomic, S, Malenkovic, G, Malenkovic, J, Sljivo, A, and Mujicic, E. COVID-19-related stress, fear and online teaching satisfaction among nursing students during the COVID-19 pandemic. Healthcare. (2023) 11:894. doi: 10.3390/healthcare11060894
53. Jang, S, and Lee, H. Changes in Core competencies among Korean university students due to remote learning during the COVID-19 pandemic. Environ Res Public Health. (2021) 18:7476. doi: 10.3390/ijerph18147476
54. Fialho, PMM, Spatafora, F, Kuhne, L, Busse, H, Helmer, SM, Zeeb, H, et al. Perceptions of study conditions and depressive symptoms during the COVID-19 pandemic among university students in Germany: results of the international COVID-19 student well-being study. Front Public Health. (2021) 9:674665. doi: 10.3389/fpubh.2021.674665
55. Birmingham, WC, Wadsworth, LL, Lassetter, JH, Graff, TC, Lauren, E, and Hung, M. COVID-19 lockdown: impact on college students’ lives. J Am Coll Heal. (2023) 71:879–93. doi: 10.1080/07448481.2021.1909041
56. Vollmann, M, Scheepers, RA, Nieboer, AP, and Hilverda, F. Study-related wellbeing, behavior, and attitudes of university students in the Netherlands during emergency remote teaching in the context of COVID-19: a longitudinal study. Front Psychol. (2022) 13:1056983. doi: 10.3389/fpsyg.2022.1056983
57. Strand, LB, Eilertsen, ME, Moksnes, UK, and Andre, B. Nursing students’ experiences on psychosocial learning environment, personal and social challenges and communication in periods of social isolation: a qualitative study. Inquiry. (2024) 61:00469580241227021. doi: 10.1177/00469580241227021
58. de la Rosa, PA, Cowden, RG, de Filippis, R, Jerotic, S, Nahidi, M, Ori, D, et al. Associations of lockdown stringency and duration with Google searches for mental health terms during the COVID-19 pandemic: a nine-country study. J Psychiatr Res. (2022) 150:237–45. doi: 10.1016/j.jpsychires.2022.03.026.
59. Baloch, GM, Sundarasen, S, Chinna, K, Nurunnabi, M, Kamaludin, K, Khoshaim, HB, et al. COVID-19: exploring impacts of the pandemic and lockdown on mental health of Pakistani students. Peerj. (2021) 9:e10612. doi: 10.7717/peerj.10612
60. Prowse, R, Sherratt, F, Abizaid, A, Gabrys, RL, Hellemans, KGC, Patterson, ZR, et al. Coping with the COVID-19 pandemic: examining gender differences in stress and mental health among university students. Psychiatry. (2021) 12:650759. doi: 10.3389/fpsyt.2021.650759
Keywords: academic performance, Chinese college student, COVID-19, learning ability warning system, analysis of variance
Citation: Ren Z and Du Q (2024) The influence of COVID-19 pandemic on college students’ academic performance and the construction of a learning ability warning system. Front. Public Health. 12:1454406. doi: 10.3389/fpubh.2024.1454406
Edited by:
Nelly Lagos San Martín, University of the Bío Bío, ChileReviewed by:
Beatriz Lucia Salvador Bizotto, Unifacvest, BrazilBeatrice Tosti, University of Cassino, Italy
Copyright © 2024 Ren and Du. This is an open-access article distributed under the terms of the Creative Commons Attribution License (CC BY). The use, distribution or reproduction in other forums is permitted, provided the original author(s) and the copyright owner(s) are credited and that the original publication in this journal is cited, in accordance with accepted academic practice. No use, distribution or reproduction is permitted which does not comply with these terms.
*Correspondence: Qiaoling Du, ZHVxbEBqbHUuZWR1LmNu