- 1Department of Cultivation Key Laboratory of Metabolic Cardiovascular Diseases Research, Fenyang Hospital Affiliated to Shanxi Medical University, Fenyang, Shanxi, China
- 2Department of Cardiovascular Medicine, Fenyang Hospital Affiliated to Shanxi Medical University, Fenyang, Shanxi, China
- 3Fenyang College of Shanxi Medical University, Fenyang, Shanxi, China
Introduction: Previous studies have demonstrated that both smoking and coronary heart disease (CHD) are linked to red cell distribution width (RDW). However, the role of the RDW in the association between smoking and CHD remains unclear. This study investigates the role of RDW in the association between smoking and coronary heart disease.
Methods: Data from 13,080 adults aged 40–80 years were analyzed from the National Health and Nutrition Examination Survey (NHANES) conducted between 2006 and 2017. Statistical methods included regression analysis, restricted cubic spline curve (RCS), subgroup analysis, and mediation analysis.
Results: Results showed higher RDW levels in participants with smoking and/or CHD than in those without. Smoking status was positively associated with RDW and CHD even after adjusting for potential confounders. A nonlinear relationship between RDW and CHD was observed (P for nonlinear <0.001). Subgroup analysis revealed that sex influenced the relationship between smoking and CHD (p = 0.0284). Mediation analysis showed that increased RDW levels mediated the association between smoking status and CHD (PM = 2.1959%, ACME = 0.000694, 95% CI = 0.000262–0.001259, p < 0.001).
Discussion: Our research showed that smoking, RDW, and CHD are interrelated, with RDW playing as a mediator, offering a novel perspective for the prevention and management of CHD.
1 Introduction
Cardiovascular diseases (CVD) pose a significant global threat to human health, with increasing prevalence and mortality rates annually (1). Among these, coronary heart disease (CHD), encompassing conditions such as angina pectoris, myocardial infarction, ischemic cardiomyopathy, and progressive coronary artery disease, stands as the leading cause of death and a significant contributor to disability within CVD (2–4). Therefore, identifying effective and controllable clinical indicators to predict or prevent the onset of CHD is crucial.
Smoking is consistently recognized as a primary modifiable behavioral risk factor for CHD (5, 6). It increases the risk of coronary atherosclerosis, thereby exacerbating the risk and clinical manifestations of CHD. Studies have found that quitting smoking and reducing cigarette consumption can mitigate the risk of stroke and myocardial infarction, with earlier cessation being more beneficial for survival (7). Despite widespread awareness of its health hazards, smoking prevalence remains high in most countries (8), presenting a significant public health challenge.
Red blood cell distribution width (RDW) serves as a critical blood marker in the pathogenesis of CHD (9, 10). RDW measures the variability and heterogeneity of red blood cells in the circulation and forms an integral part of the standard complete blood count (CBC) (11). Elevated RDW levels are associated with impaired erythropoiesis and heightened red blood cell degradation, leading to compromised blood circulation and oxygen delivery, ultimately contributing to adverse outcomes. Previous studies have shown that RDW can effectively predict the incidence and mortality of various cardiovascular diseases, with higher RDW levels closely associated with the onset and progression of CHD (12–14).
Several studies suggest that smoking is linked to increased RDW levels. Smokers tend to exhibit higher average RDW values compared to nonsmokers, with RDW significantly positively correlated with both the daily quantity of cigarettes smoked and the duration of smoking (15). For instance, one study found evidence that elevated RDW could be attributed to the oxidative stress and inflammation caused by smoking, which disrupts erythropoiesis and promotes red blood cell turnover (16).
However, few studies have investigated whether RDW mediates the association between smoking and CHD. This study addressed this gap in the literature by analyzing data from a public database to explore whether RDW mediates the relationship between smoking and CHD. Specifically, we hypothesized that smoking increases RDW levels, which in turn elevates the risk of CHD. By elucidating the mediating role of RDW, this research aimed to provide new insights into the clinical management of CHD and highlight the importance of RDW as a potential target for intervention in smokers.
2 Materials and methods
2.1 Study population
This study conducted a cross-sectional analysis using survey data from the National Health and Nutrition Examination Survey (NHANES) collected between 2006 and 2017. Ethical approval for this survey was granted by the Ethics Committee of the Center for Disease Control and Prevention (CDC), and the NHANES study protocol was authorized by the Research Ethics Review Board of the National Center for Health Statistics in accordance with the revised Declaration of Helsinki. Informed consent was obtained from all participants. Detailed information on datasets, documentation, and protocols can be accessed freely on the NHANES website.1 Data privacy was maintained through strict adherence to confidentiality protocols to ensure that participant information was de-identified.
Given that age is a significant risk factor for cardiovascular disease, particularly among individuals aged over 40 years. This age group is a primary focus for related research and prevention efforts (17, 18). Due to the complex health statuses often observed in individuals aged over 80 years attributed to the coexistence of multiple diseases (19, 20), this cross-sectional analysis was confined to individuals aged 40–79 years (n = 17,389). From this initial cohort, 4,309 individuals were excluded due to missing data on RDW (n = 1,290), smoking status (n = 11), CHD (n = 70), or other covariates such as demographic variables, body mass index (BMI), alcohol use, diabetes, hypertension, hyperlipidemia (n = 2,938). Ultimately, the final sample comprised 13,080 participants. A flowchart depicting participant selection is provided in Figure 1.
2.2 Exposure: smoking
Trained interviewers conducted smoking-related questions during the NHANES Mobile Examination Center interviews using a computer-assisted personal interview system. Based on their self-reported responses to the question, “Have you smoked at least 100 cigarettes in your lifetime?” participants were categorized into two groups: “nonsmokers” (those who answered “No”) and “smokers.” (those who answered “Yes”).
2.3 Outcomes: CHD
The outcomes of this study were derived from self-reported diagnoses in a CVD-related questionnaire. Participants who reported a diagnosis of CHD, MI, or angina were included in the CHD group for our research (21).
2.4 Laboratory measures
Blood samples were collected at the NHANES Mobile Examination Center. RDW (%) was measured using the Beckman Coulter MAXM instrument from 2007 to 2012 and the Beckman Coulter DXH 800 instrument from 2013 to 2016, combined with an automatic dilution and mixing device for sample processing.
2.5 Covariates
The choice of covariates was based on established risk factors for CHD and their potential to confound the relationship between smoking, RDW, and CHD. Age was included as a continuous variable. Race (non-Hispanic white, Mexican American, non-Hispanic black, other Hispanic, or other race/multiracial), education level (less than high school, high school or GED, or more than high school), marital status (married/living with partner, widowed/divorced/separated, or never married), poverty-income ratio (PIR) (low, middle, high), BMI(normal weight, over-weight, obese), alcohol consumption (current, former, never), diabetes status (yes, no), hypertension status (yes, no), and hyperlipidemia status(yes, no) were considered as categorical variables.
Income levels were categorized into three tiers based on the ratio of family income to poverty (PIR): low (<1.30), middle (1.30–3.49), and high (≥ 3.50) (22). BMI was calculated using weight (kg) and height (kg/m2) and categorized into the following classes: Normal weight (< 25), Overweight (23–28), and Obese (≥ 30) (29). Alcohol status was categorized as never drinkers (lifetime alcohol <12 drinks), former drinkers (lifetime alcohol ≥12 drinks but last year alcohol <12 drinks), or current drinkers (lifetime alcohol ≥12 drinks and last year alcohol ≥12 drinks) (30). Diabetes mellitus (DM) was defined as hemoglobinA1c ≥6.5%, fasting plasma glucose ≥7.0 mmol/L, a self-reported physician diagnosis, or current use of antidiabetic medication or insulin (23). Hypertension was defined as systolic blood pressure (SBP) ≥140 mmHg, diastolic blood pressure (DBP) ≥90 mmHg, a self-reported physician diagnosis, or current use of antihypertensive medication (24). Hyperlipidemia was defined as triglycerides (TG) ≥150 mg/dL, total cholesterol (TC) ≥200 mg/dL, high-density lipoprotein cholesterol (HDL-C) <40 mg/dL, low-density lipoprotein cholesterol (LDL-C) ≥130 mg/dL, a self-reported physician diagnosis, or current use of lipid-lowering medication (25, 26).
2.6 Statistical analysis
Continuous variables following a normal distribution were presented as mean ± standard deviation (SD), while non-normally distributed continuous variables were presented as median and interquartile range (IQR). Categorical variables were reported as proportions and percentages. Differences between groups were assessed using one-way ANOVA for normally distributed data, the Kruskal-Wallis test for non-normally distributed data, and the chi-square test for categorical variables.
Multivariate logistic regression was used to evaluate the relationships among smoking, RDW, and CHD, while multivariate linear regression analysis assessed the relationship between smoking status and RDW. Two models were developed for the analysis: Model 1 was unadjusted for confounders, representing a univariate analysis. Model 2 was adjusted for age, sex, race, education, PIR, BMI, diabetes, hypertension, and hyperlipidemia.
RCS based on logistic regression models was used to visualize the relationship between RDW and CHD according to Model 2, with knots placed at the 10th, 50th, and 90th percentiles of RDW. Threshold levels for each section were determined using likelihood ratio tests and bootstrap resampling. A segmented regression model was further applied to examine the relationship between RDW and CHD.
Stratified subgroup and interaction analyses were conducted using multivariate logistic regression with full adjustment for categorical variables.
Finally, mediation analysis was performed to examine whether RDW mediated the relationship between the exposure variable (smoking) and outcome (CHD). The analysis used 1,000 bootstrap samples to display the size of the indirect path effect, the proportion of the mediation effect, and the p-value of the mediation effect in the results.
All statistical analyses were performed using statistical software (R version 4.3.3). Descriptive statistics were obtained using the dplyr package, RCS analysis using the plotRCS package, segmented regression analyses using the segmented package, forest plots using the forestplot package, mediation analysis using the mediation package, bootstrap resampling methods using the boot package, and graph plots using the ggplot2 package. A p-value less than 0.05 was considered statistically significant in all analyses.
3 Results
3.1 Participant characteristics
A total of 13,080 participants were enrolled in this study, of whom 49.23% were smokers, and 8.99% were diagnosed with CHD. Participants (n = 13,080) were divided into four groups: “smoke with CHD” (n = 768), “smoke without CHD” (n = 5,672), “CHD without smoke” (n = 408), and “without smoke and CHD” (n = 6,232). None of the continuous variables in our data were normally distributed. As shown in Table 1, the median age of participants was 57 years, and 49.2% were male. The racial distribution was as follows: 14.8% Mexican American, 44.1% non-Hispanic white, 21.3% non-Hispanic black, and 19.8% other. Educational levels were 26.1% less than high school, 22.6% high school or GED, and 51.3% higher than high school. Marital status distribution was: 63.9% married or living with a partner, 27.6% widowed, divorced, or separated, and 8.5% never married. BMI categories were 23.8% normal weight, 34.4% overweight, and 41.8% obese. PIR distribution was 29.9% low, 36.0% medium, and 34.1% high. Drinking status was 70.9% current, 14.9% former, and 14.3% never. The prevalence rates of diabetes, hypertension, and hyperlipidemia were 23.9, 53.5, and 78.6%, respectively.
Compared to the “without smoke and CHD” group, the “smoke with CHD” group tended to be slightly older, more likely to be female, more likely to be non-Hispanic white, have a lower PIR, and more likely to have DM, hypertension, hyperlipidemia, as well as higher RDW levels. Additionally, the median RDW level was 13.1%. There were significant differences in RDW levels among the groups: “smoke with CHD,” “smoke without CHD,” “CHD without smoke,” and “without smoke and CHD” (RDW: 13.50 [12.88, 14.40] vs. 13.10 [12.50, 13.90] vs. 13.30 [12.80, 14.10] vs. 13.10 [12.50, 13.80], p < 0.001).
3.2 Association analysis
The correlations of smoking with RDW and CHD in both crude and adjusted models are summarized in Table 2. Following adjustment for potential confounders (age, sex, race, education, PIR, BMI, alcohol consumption, DM, hypertension, and hyperlipidemia), smoking was found to be significantly associated with CHD (OR = 1.56, 95% confidence interval[CI] = 1.36–1.80, p < 0.001). Moreover, smoking was associated with RDW (OR = 0.08, 95%CI = 0.03–0.13, p = 0.001).
3.3 Restricted cubic spline curve fitting and segmented regression analyses
Figure 2 depicts the relationship between RDW and CHD using restricted cubic spline curves. Subfigures A and B represent the crude and adjusted models, respectively. Both models showed a nonlinear relationship between RDW and CHD (P for nonlinear <0.001 for the crude model and p = 0.005 for the adjusted model).
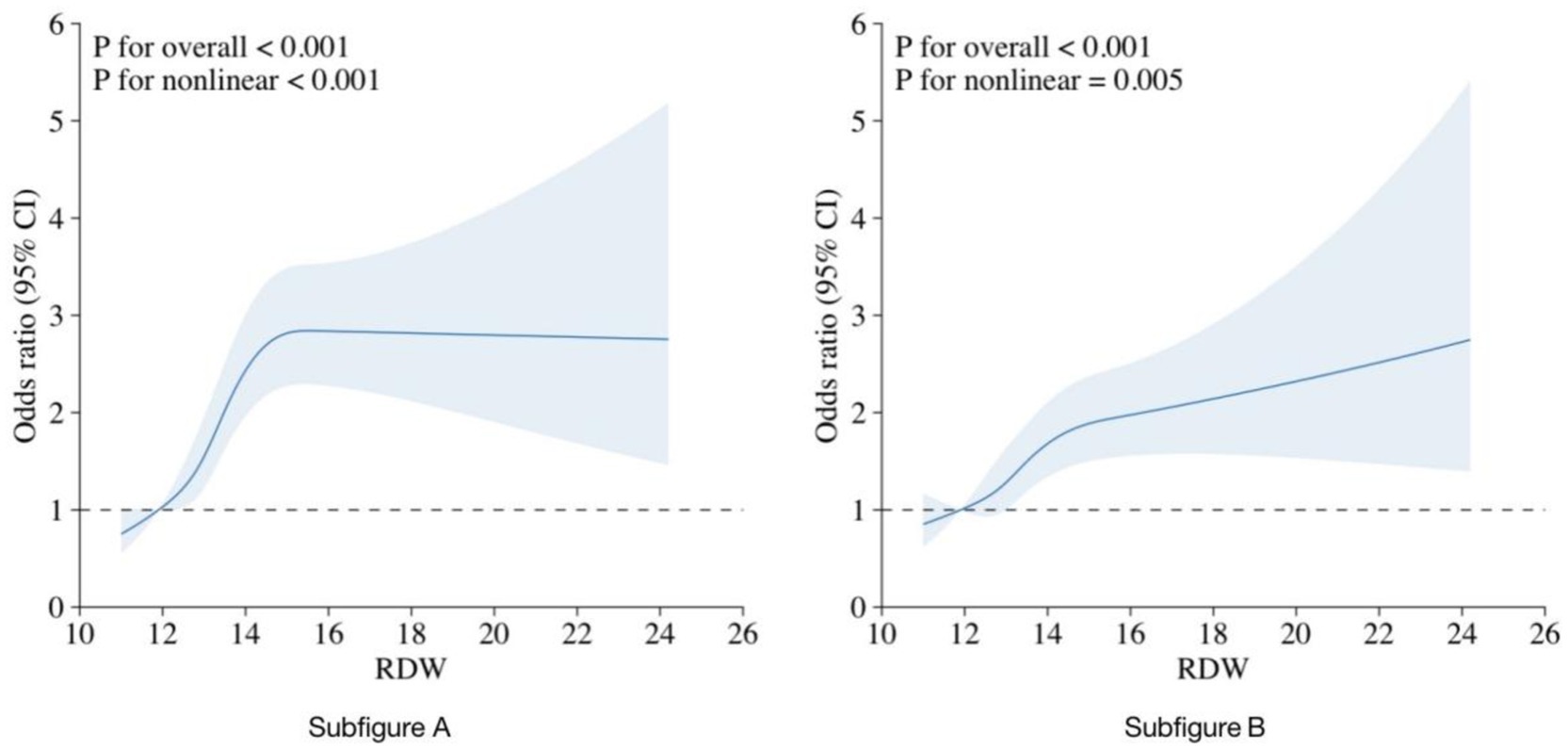
Figure 2. RCS curve fit between RDW and CHD. Notes: Solid lines represent smooth curve fits between the variables. The bands represent the 95%CI from the fit. (A) No adjustment for covariates. (B) Adjusted for age, sex, race, education, PIR, BMI, alcohol consumption, DM, hypertension, and hyperlipidemia.
A threshold effect analysis on the adjusted model identified an RDW cutoff point of 14.5% as the best fit. The risk of CHD rapidly increased with increasing RDW up to this cutoff point and then rose more steadily. The relationship between RDW and CHD remained significant both before and after the cutoff point (p < 0.001). As shown in Table 3, when RDW was <14.5%, a 1 SD increase in RDW was associated with an OR of 1.29 (95%CI = 1.17–1.43) for CHD, while there was no significant difference between RDW and CHD when RDW was ≥14.5% (OR = 1.03, 95% CI = 0.91–1.18, p = 0.711).
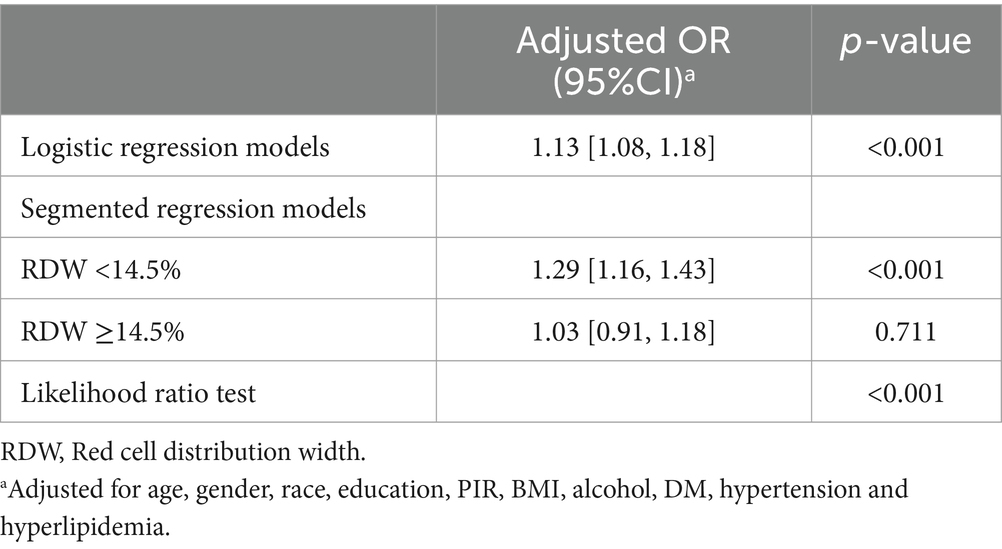
Table 3. Threshold effect analyzes of association between RDW and CHD using segmented regression models.
3.4 Subgroup analysis
Further subgroup analysis was conducted using the adjusted model to study potential variations in the relationship between smoking and CHD among different populations. The results, shown in Figure 3, indicate a significant positive correlation between smoking and CHD incidence in most subgroups, including sex, race, education, marital status, PIR, BMI, alcohol consumption, diabetes, hypertension, and hyperlipidemia (p < 0.001). Exceptions were observed in Mexican Americans (p = 0.11), other races (p = 0.149), and high-PIR populations (p = 0.143). No significant evidence was found regarding the effects of the interactions between smoking and important covariates on the risk of CHD (all p ≥ 0.05). Additionally, a significant interaction between sex subgroups in the relationship between smoking and CHD was observed (p = 0.0284).
3.5 The mediating role of RDW
As shown in Table 4 and Figure 4, RDW significantly mediated the association between smoking and CHD (proportion mediated [PM] = 2.1959%, average causal mediation effect [ACME] = 0.000694, 95%CI = 0.000262–0.001259, p < 0.001). When participants were divided by sex, RDW significantly mediated the relationship between smoking and CHD in males after adjusting for all covariates (PM = 3.4235%, ACME = 0.000950, 95%CI = 0.000193–0.001916, p = 0.012). However, the mediating effect of RDW was not significant in females(PM = 0.7556%, ACME = 0.000256, 95%CI = −0.000115–0.000707, p = 0.178). Interestingly, when females were further divided based on their responses to the question, “At what age did you have your last menstrual period?,” RDW significantly mediated the relationship between smoking and CHD in postmenopausal women (PM = 1.8241%, ACME = 0.000732, 95%CI = 0.000159–0.001616, p = 0.014), but not in premenopausal women (PM = −0.2854%, ACME = −0.000041, 95%CI = −0.000607–0.000495, p = 0.898). The results were smoothed using generalized additive and linear models.
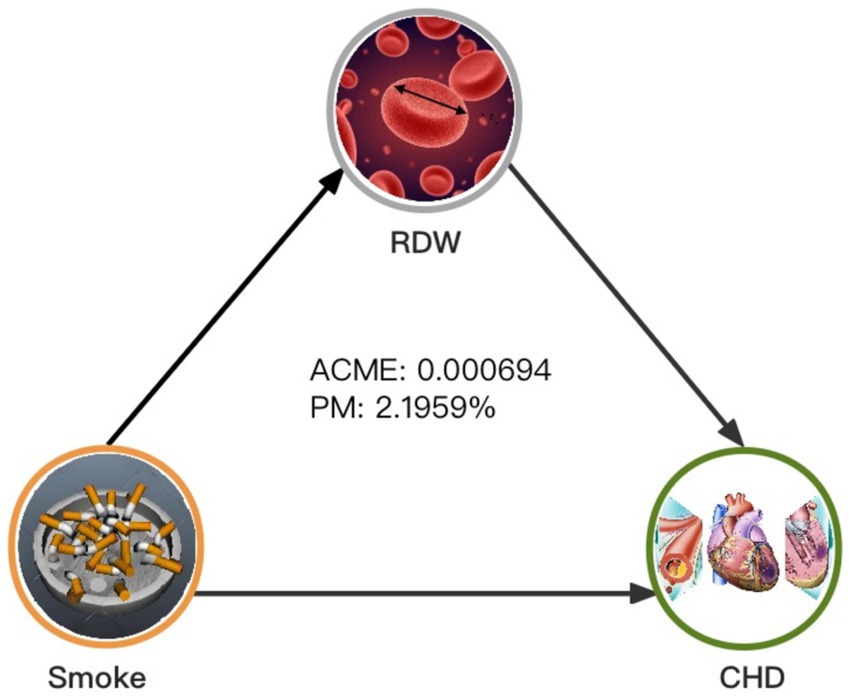
Figure 4. Mediated analysis model path diagram. Notes: Smoking, CHD and RDW are defined as the independent, dependent, and mediating variables, respectively. ACME indicates average causal mediation effects (indirect effect), and PM indicates proportion-mediated effects (e.g., proportion of the total effect due to the mediator). The ACME and average direct effect coefficients are displayed along with PM.
4 Discussion
4.1 Overview
This study aimed to investigate the association between smoking and CHD in middle-aged and older adult people aged 40–80 in the United States using data from NHANES (2006–2017) and to determine whether RDW partly mediates this association.
4.2 Association and mechanisms
Consistent with previous studies (27), our study reaffirmed the positive correlation between smoking and CHD. Even after adjusting for potential confounders, smoking remained a significant risk factor for CHD, emphasizing the urgent need to control smoking to alleviate the burden of cardiovascular diseases.
We also found a correlation between smoking and higher RDW levels, with smokers having higher RDW levels than nonsmokers. We speculated on possible mechanisms underlying the elevation of RDW in smokers. First, smoking has been linked to elevated levels of hs-CRP and other inflammatory markers such as interleukin-6, soluble tumor necrosis factor-alpha, VCAM-1, ICAM-1, and E-selectin (28). Higher RDW values may reflect underlying inflammatory states (12), and significant associations have been observed between CRP levels and RDW (31). Therefore, the increase in RDW may result from ineffective red blood cell production due to chronic inflammation in smokers, leading to the entry of immature red blood cells into circulation and elevated RDW levels. Oxidative stress may be another pathological mechanism linking RDW and smoking. Previous studies have shown an association between smoking and high oxidative stress (32), which can cause red blood cell hemolysis (33). In cases of hemolysis, the reticulocyte count may increase to compensate for the loss of red blood cells, leading to an increase in RDW (34).
Our study revealed a nonlinear relationship between RDW and CHD, with CHD risk increasing significantly up to an RDW cutoff value of 14.5%. Beyond this cutoff value, additional increases in RDW had no substantial effects on CHD risk. The correlation between RDW and inflammatory markers suggests that the association between RDW and CHD may be related to inflammatory reactions. Lappé et al. (35) also reported an association between RDW and CRP levels in patients with coronary artery disease. The study by Ainiwaer et al. (36), which analyzed data from the National Health and Nutrition Examination Survey (1999–2020), further supports these findings. Their study found a significant association between RDW and RDW-to-platelet count ratio with cardiovascular disease (CVD) among US adults, which aligns with our results. They suggest that RDW and related hematological parameters may play a crucial role in cardiovascular health, particularly in populations with a high prevalence of smoking. The present study strengthens the argument that RDW may serve as an important marker for CVD risk and further highlights its potential clinical relevance. These findings indicate that RDW may be a valuable biomarker for identifying individuals at high risk of CHD. These findings are a reminder of the importance of early assessment of RDW levels.
Finally, mediation analysis indicated that RDW partially mediated the relationship between smoking and CHD in middle-aged and older adult individuals, accounting for approximately 2.2% of the total effect. Although the proportion of mediation seems small, it underscores the importance of RDW as a biological pathway through which smoking influences CHD risk. Our subgroup analysis showed a significant effect of sex on the relationship between smoking and CHD. Stratified mediation analysis revealed that the mediating effect of RDW was significant in males but not in females. Further categorization of females based on menopausal status showed a significant mediating effect of RDW in postmenopausal females. This difference suggests that hormonal changes associated with menopause may affect the relationship between smoking, RDW, and CHD. Previous studies have shown that estrogen levels influence biological processes related to atherosclerosis (37). In an atherosclerosis mouse model, estrogen can reduce atherosclerotic plaque burden (38), decrease the upregulation of endothelial cell adhesion molecules induced by cytokines, and reduce interleukin-6 expression, thereby inhibiting inflammation and reducing CHD incidence. Estrogen can also regulate cholesterol reverse transport, lower LDL-C and HDL-C levels, and prevent excessive lipid uptake by macrophages (39). Estrogen also increases endothelial nitric oxide production (40, 41), dilating blood vessels and improving endothelial cell function (42). Additionally, Meng et al. (43) reported that estrogen can reduce vascular endothelial cell pyroptosis through estrogen receptor alpha-mediated activation of autophagy, thus ameliorating atherosclerosis. However, after menopause, ovarian function declines, leading to decreased estrogen levels. When estrogen levels decrease, the efficiency of inflammation inhibition is lower, lipid status is worse than that in premenopausal women, and vascular dilation efficiency is lower. This may exacerbate the impact of smoking on hematological parameters and enhance the mediating role of RDW in this subgroup.
4.3 Strengths and limitations of the study
This study is the first to identify the relationship between smoking and CHD mediated by RDW in middle-aged and older adult populations, with a large sample size and diverse ethnic population, enhancing the generalizability of the results. Multiple statistical techniques were used, including regression, restricted cubic spline curve fitting, and mediation analyses, to rigorously examine the relationships among smoking, RDW, and CHD. However, this study has several limitations. First, its cross-sectional design prevents the establishment of causality between smoking, RDW, and CHD, longitudinal studies are needed to confirm the mediating role of RDW and explore underlying mechanisms. Necessitating further longitudinal studies. Second, self-reported methods were used to assess smoking status and CHD, potentially introducing recall and reporting biases. Future studies could incorporate biomarkers (such as nicotine or metabolites in the blood) to validate self-reported data and beter ensure their accuracy. Finally, the RDW was measured at a single time point, failing to capture dynamic changes. Future research should incorporate longitudinal RDW measurements to assess its dynamic relationship with smoking and CHD risk. The findings also need to be confirmed by additional studies on CHD patients with larger a sample size.
4.4 Future research and clinical implications
Future research should focus on longitudinal studies to confirm the mediating role of RDW and elucidate the biological mechanisms underlying the relationship between RDW, smoking, and CHD. Exploring the potential clinical applications of RDW as a biomarker for CHD risk is crucial. Public health interventions aimed at reducing smoking and CHD risk should consider incorporating RDW measurements to identify individuals at higher risk. Tailored interventions based on RDW levels could improve preventive strategies and outcomes for populations at risk of CHD.
5 Conclusion
Our study highlights an association between RDW, smoking status, and CHD. These findings underscore the importance of RDW as a biomarker linking smoking to an elevated risk of CHD, with a particular emphasis on considering sex hormones in studies involving RDW, smoking, and CHD. Further longitudinal studies are imperative to elucidate whether elevated RDW contributes to the development of optimal intervention strategies tailored to different populations.
Data availability statement
The datasets presented in this study can be found in online repositories. The names of the repository/repositories and accession number(s) can be found in the article/supplementary material.
Ethics statement
The studies involving humans were approved by Center for Disease Control and Prevention (CDC). The studies were conducted in accordance with the local legislation and institutional requirements. Written informed consent for participation in this study was provided by the participants’ legal guardians/next of kin.
Author contributions
MM: Data curation, Funding acquisition, Project administration, Supervision, Validation, Writing – original draft, Writing – review & editing. YW: Formal analysis, Methodology, Software, Validation, Visualization, Writing – original draft, Writing – review & editing. XH: Conceptualization, Investigation, Resources, Writing – review & editing. MZ: Investigation, Resources, Writing – review & editing. YH: Investigation, Resources, Writing – review & editing. RG: Conceptualization, Funding acquisition, Investigation, Writing – review & editing. SL: Investigation, Resources, Writing – review & editing.
Funding
The author(s) declare that financial support was received for the research, authorship, and/or publication of this article. This study was supported by the Lvliang Key Laboratory of Cardiovascular Disease (2020ZDSYS16) and the Cultivation Key Laboratory of Metabolic Cardiovascular Diseases Research (TSGJ001) and the Lvliang introduced high-level scientific and technological talents key research and development projects (2019115).
Acknowledgments
We thank all the investigators and members of staff who participated in this study for their important contributions, and Editage (www.editage.cn) for English language editing.
Conflict of interest
The authors declare that the research was conducted in the absence of any commercial or financial relationships that could be construed as a potential conflict of interest.
Publisher’s note
All claims expressed in this article are solely those of the authors and do not necessarily represent those of their affiliated organizations, or those of the publisher, the editors and the reviewers. Any product that may be evaluated in this article, or claim that may be made by its manufacturer, is not guaranteed or endorsed by the publisher.
Footnotes
References
1. Virani, SS, Alonso, A, Benjamin, EJ, Bittencourt, MS, and Callaway, CWAmerican Heart Association Council on Epidemiology and Prevention Statistics Committee and Stroke Statistics Subcommittee. Heart disease and stroke Statistics-2020 update: a report from the American Heart Association. Circulation. (2020) 141:e139–596. doi: 10.1161/CIR.0000000000000757
2. Roth, GA, Mensah, GA, Johnson, CO, and Addolorato, G. Global burden of cardiovascular diseases and risk factors, 1990–2019: update from the GBD 2019 study. J Am Coll Cardiol. (2020) 76:2982–3021. doi: 10.1016/j.jacc.2020.11.010
3. Vogel, B, Claessen, BE, Arnold, SV, Chan, D, and Cohen, DJ. ST-segment elevation myocardial infarction. Nat Rev Dis Primers. (2019) 5:39. doi: 10.1038/s41572-019-0090-3
4. Stanhewicz, AE, Wenner, MM, and Stachenfeld, NS. Sex differences in endothelial function important to vascular health and overall cardiovascular disease risk across the lifespan. Am J Physiol Heart Circ Physiol. (2018) 315:H1569–88. doi: 10.1152/ajpheart.00396.2018
5. Gallucci, G, Tartarone, A, Lerose, R, Lalinga, AV, and Capobianco, AM. Cardiovascular risk of smoking and benefits of smoking cessation. J Thorac Dis. (2020) 12:3866–76. doi: 10.21037/jtd.2020.02.47
6. Di Cicco, ME, Ragazzo, V, and Jacinto, T. Mortality in relation to smoking: the British doctors study. Breathe (Sheff). (2016) 12:275–6. doi: 10.1183/20734735.013416
7. Møller, AL, and Andersson, C. Importance of smoking cessation for cardiovascular risk reduction. Eur Heart J. (2021) 42:4154–6. doi: 10.1093/eurheartj/ehab541
8. Collishaw, NE, and Lopez, AD. Prevalence of cigarette smoking in developing countries. Tob Control. (1995) 4:327. doi: 10.1136/tc.4.4.327
9. Cavusoglu, E, Chopra, V, Gupta, A, Battala, VR, Poludasu, S, Eng, C, et al. Relation between red blood cell distribution width (RDW) and all-cause mortality at two years in an unselected population referred for coronary angiography. Int J Cardiol. (2010) 141:141–6. doi: 10.1016/j.ijcard.2008.11.187
10. Uyarel, H, Ergelen, M, Cicek, G, Kaya, MG, Ayhan, E, Turkkan, C, et al. Red cell distribution width as a novel prognostic marker in patients undergoing primary angioplasty for acute myocardial infarction. Coron Artery Dis. (2011) 22:138–44. doi: 10.1097/MCA.0b013e328342c77b
11. Sadaka, F, O’Brien, J, and Prakash, S. Red cell distribution width and outcome in patients with septic shock. J Intensive Care Med. (2013) 28:307–13. doi: 10.1177/0885066612452838
12. Tonelli, M, Sacks, F, Arnold, M, Moye, L, Davis, B, Pfeffer, M, et al. Relation between red blood cell distribution width and cardiovascular event rate in people with coronary disease. Circulation. (2008) 117:163–8. doi: 10.1161/CIRCULATIONAHA.107.727545
13. Zalawadiya, SK, Veeranna, V, Niraj, A, Pradhan, J, and Afonso, L. Red cell distribution width and risk of coronary heart disease events. Am J Cardiol. (2010) 106:988–93. doi: 10.1016/j.amjcard.2010.06.006
14. Patel, KV, Semba, RD, Ferrucci, L, Newman, AB, Fried, LP, Wallace, RB, et al. Red cell distribution width and mortality in older adults: a meta-analysis. J Gerontol A Biol Sci Med Sci. (2010) 65:258–65. doi: 10.1093/gerona/glp163
15. Kurtoğlu, E, Aktürk, E, Korkmaz, H, Sincer, I, Yılmaz, M, Erdem, K, et al. Elevated red blood cell distribution width in healthy smokers. Turk Kardiyol Dern Ars. (2013) 41:199–206. doi: 10.5543/tkda.2013.42375
16. Ahmed, IA, Mohammed, MA, Hassan, HM, and Ali, IA. Relationship between tobacco smoking and hematological indices among Sudanese smokers. J Health Popul Nutr. (2024) 43:5. doi: 10.1186/s41043-023-00493-0
17. Tsao, CW, Aday, AW, Almarzooq, ZI, Anderson, CAM, Arora, P, Avery, CL, et al. Heart disease and stroke Statistics-2023 update: a report from the American Heart Association. Circulation. (2023) 147:e93–e621. doi: 10.1161/CIR.0000000000001123
18. Piepoli, MF, Hoes, AW, Agewall, S, Albus, C, Brotons, C, Catapano, AL, et al. 2016 European guidelines on cardiovascular disease prevention in clinical practice: the sixth joint task force of the European Society of Cardiology and Other Societies on cardiovascular disease prevention in clinical practice (constituted by representatives of 10 societies and by invited experts)developed with the special contribution of the European Association for Cardiovascular Prevention & rehabilitation (EACPR). Eur Heart J. (2016) 37:2315–81. doi: 10.1093/eurheartj/ehw106
19. Marengoni, A, Angleman, S, Melis, R, Mangialasche, F, Karp, A, Garmen, A, et al. Aging with multimorbidity: a systematic review of the literature. Ageing Res Rev. (2011) 10:430–9. doi: 10.1016/j.arr.2011.03.003
20. Barnett, K, Mercer, SW, Norbury, M, Watt, G, Wyke, S, and Guthrie, B. Epidemiology of multimorbidity and implications for health care, research, and medical education: a cross-sectional study. Lancet. (2012) 380:37–43. doi: 10.1016/S0140-6736(12)60240-2
21. Xu, JP, Zeng, RX, He, MH, Lin, SS, Guo, LH, and Zhang, MZ. Associations between serum soluble α-klotho and the prevalence of specific cardiovascular disease. Front Cardiovasc Med. (2022) 9:899307. doi: 10.3389/fcvm.2022.899307
22. Wang, L, Martínez Steele, E, Du, M, Pomeranz, JL, O’Connor, LE, Herrick, KA, et al. Trends in consumption of ultraprocessed foods among US youths aged 2–19 years, 1999–2018. JAMA. (2021) 326:519–30. doi: 10.1001/jama.2021.10238
23. Cosentino, F, Grant, PJ, Aboyans, V, Bailey, CJ, Ceriello, A, Delgado, V, et al. 2019 ESC guidelines on diabetes, prediabetes, and cardiovascular diseases developed in collaboration with the EASD. Eur Heart J. (2020) 41:255–323. doi: 10.1093/eurheartj/ehz486
24. Bakris, G, Ali, W, and Parati, G. ACC/AHA Versus ESC/ESH on Hypertension Guidelines: JACC guideline comparison. J Am Coll Cardiol. (2019) 73:3018–26. doi: 10.1016/j.jacc.2019.03.507
25. Nelson, RH . Hyperlipidemia as a risk factor for cardiovascular disease. Prim Care. (2013) 40:195–211. doi: 10.1016/j.pop.2012.11.003
26. Aggarwal, R, Bhatt, DL, Rodriguez, F, Yeh, RW, and Wadhera, RK. Trends in lipid concentrations and lipid control among US adults, 2007–2018. JAMA. (2022) 328:737–45. doi: 10.1001/jama.2022.12567
27. Siasos, G, Tsigkou, V, Kokkou, E, Oikonomou, E, Vavuranakis, M, Vlachopoulos, C, et al. Smoking and atherosclerosis: mechanisms of disease and new therapeutic approaches. Curr Med Chem. (2014) 21:3936–48. doi: 10.2174/092986732134141015161539
28. Levitzky, YS, Guo, CY, Rong, J, Larson, MG, Walter, RE, Keaney, JF, et al. Relation of smoking status to a panel of inflammatory markers: the Framingham offspring. Atherosclerosis. (2008) 201:217–24. doi: 10.1016/j.atherosclerosis.2007.12.058
29. James, WPT . Obesity: a global public health challenge. Clin Chem. (2018) 64:24–9. doi: 10.1373/clinchem.2017.273052
30. Jiang, M, Tang, X, Wang, P, Yang, L, and Du, R. Association between daily alcohol consumption and serum alpha klotho levels among US adults over 40 years old: a cross-sectional study. BMC Public Health. (2023) 23:1901. doi: 10.1186/s12889-023-16830-1
31. Lippi, G, Targher, G, Montagnana, M, Salvagno, GL, Zoppini, G, and Guidi, GC. Relation between red blood cell distribution width and inflammatory biomarkers in a large cohort of unselected outpatients. Arch Pathol Lab Med. (2009) 133:628–32. doi: 10.5858/133.4.628
32. Carnevali, S, Petruzzelli, S, Longoni, B, Vanacore, R, Barale, R, Cipollini, M, et al. Cigarette smoke extract induces oxidative stress and apoptosis in human lung fibroblasts. Am J Phys Lung Cell Mol Phys. (2003) 284:L955–63. doi: 10.1152/ajplung.00466.2001
33. Straat, M, van Bruggen, R, de Korte, D, and Juffermans, NP. Red blood cell clearance in inflammation. Transfus Med Hemother. (2012) 39:353–60. doi: 10.1159/000342229
34. Mohanty, JG, Nagababu, E, and Rifkind, JM. Red blood cell oxidative stress impairs oxygen delivery and induces red blood cell aging. Front Physiol. (2014) 5:84. doi: 10.3389/fphys.2014.00084
35. Lappé, JM, Horne, BD, Shah, SH, May, HT, Muhlestein, JB, Lappé, DL, et al. Red cell distribution width, C-reactive protein, the complete blood count, and mortality in patients with coronary disease and a normal comparison population. Clin Chim Acta. (2011) 412:2094–9. doi: 10.1016/j.cca.2011.07.018
36. Ainiwaer, A, Kadier, K, Abulizi, A, Hou, WQ, Rehemuding, R, Maimaiti, H, et al. Association of red cell distribution width (RDW) and the RDW to platelet count ratio with cardiovascular disease among US adults: a cross-sectional study based on the National Health and nutrition examination survey 1999-2020. BMJ Open. (2023) 13:e068148. doi: 10.1136/bmjopen-2022-068148
37. Vaura, F, Palmu, J, Aittokallio, J, Kauko, A, and Niiranen, T. Genetic, molecular, and cellular determinants of sex-specific cardiovascular traits. Circ Res. (2022) 130:611–31. doi: 10.1161/CIRCRESAHA.121.319891
38. Hodgin, JB, Krege, JH, Reddick, RL, Korach, KS, Smithies, O, and Maeda, N. Estrogen receptor alpha is a major mediator of 17beta-estradiol’s atheroprotective effects on lesion size in Apoe−/− mice. J Clin Invest. (2001) 107:333–40. doi: 10.1172/JCI11320
39. Fernández-Suárez, ME, Escolà-Gil, JC, Pastor, O, Dávalos, A, Blanco-Vaca, F, Lasunción, MA, et al. Clinically used selective estrogen receptor modulators affect different steps of macrophage-specific reverse cholesterol transport. Sci Rep. (2016) 6:32105. doi: 10.1038/srep32105
40. Schulz, E, Anter, E, Zou, MH, and Keaney, JF. Estradiol-mediated endothelial nitric oxide synthase association with heat shock protein 90 requires adenosine monophosphate-dependent protein kinase. Circulation. (2005) 111:3473–80. doi: 10.1161/CIRCULATIONAHA.105.546812
41. Vanhoutte, PM, Zhao, Y, Xu, A, and Leung, SWS. Thirty years of saying NO: sources, fate, actions, and misfortunes of the endothelium-derived vasodilator mediator. Circ Res. (2016) 119:375–96. doi: 10.1161/CIRCRESAHA.116.306531
42. Darblade, B, Pendaries, C, Krust, A, Dupont, S, Fouque, MJ, Rami, J, et al. Estradiol alters nitric oxide production in the mouse aorta through the alpha-, but not beta-, estrogen receptor. Circ Res. (2002) 90:413–9. doi: 10.1161/hh0402.105096
Keywords: coronary heart disease, smoking, red blood cell distribution width, NHANES, mediation analysis
Citation: Ma M, Wu Y, He X, Zhang M, Han Y, Guo R and Li S (2024) Associations between smoking and coronary heart disease: mediating role of RDW. Front. Public Health. 12:1447303. doi: 10.3389/fpubh.2024.1447303
Edited by:
Linwei Tian, The University of Hong Kong, Hong Kong SAR, ChinaReviewed by:
Behnam Tajik, University of Eastern Finland, FinlandAndrea Abreu, University of São Paulo, Brazil
Copyright © 2024 Ma, Wu, He, Zhang, Han, Guo and Li. This is an open-access article distributed under the terms of the Creative Commons Attribution License (CC BY). The use, distribution or reproduction in other forums is permitted, provided the original author(s) and the copyright owner(s) are credited and that the original publication in this journal is cited, in accordance with accepted academic practice. No use, distribution or reproduction is permitted which does not comply with these terms.
*Correspondence: Mingfeng Ma, bWFtaW5nZmVuZzEwNkBzaW5hLmNvbQ==
†These authors have contributed equally to this work and share first authorship