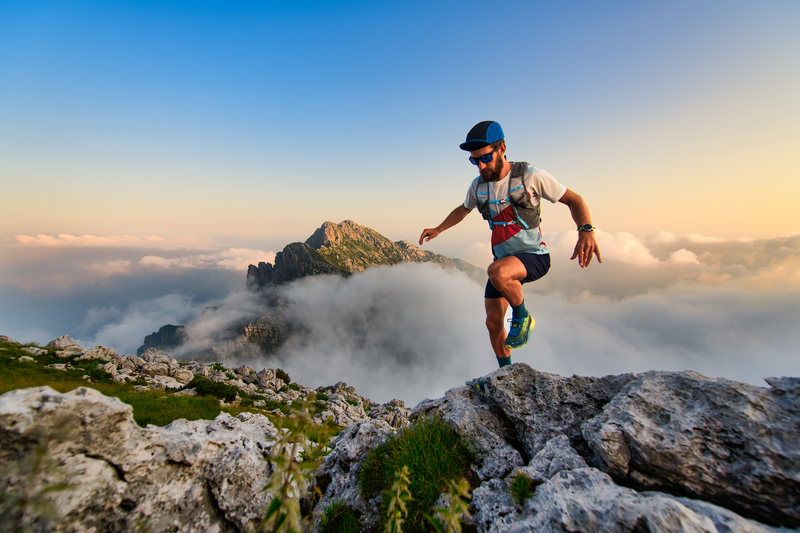
94% of researchers rate our articles as excellent or good
Learn more about the work of our research integrity team to safeguard the quality of each article we publish.
Find out more
ORIGINAL RESEARCH article
Front. Public Health , 12 September 2024
Sec. Infectious Diseases: Epidemiology and Prevention
Volume 12 - 2024 | https://doi.org/10.3389/fpubh.2024.1444176
This article is part of the Research Topic Outbreak Investigations of Nosocomial Infections View all 13 articles
This retrospective study used 10 machine learning algorithms to predict the risk of healthcare-associated infections (HAIs) in patients admitted to intensive care units (ICUs). A total of 2,517 patients treated in the ICU of a tertiary hospital in China from January 2019 to December 2023 were included, of whom 455 (18.1%) developed an HAI. Data on 32 potential risk factors for infection were considered, of which 18 factors that were statistically significant on single-factor analysis were used to develop a machine learning prediction model using the synthetic minority oversampling technique (SMOTE). The main HAIs were respiratory tract infections (28.7%) and ventilator-associated pneumonia (25.0%), and were predominantly caused by gram-negative bacteria (78.8%). The CatBoost model showed good predictive performance (area under the curve: 0.944, and sensitivity 0.872). The 10 most important predictors of HAIs in this model were the Penetration Aspiration Scale score, Braden score, high total bilirubin level, female, high white blood cell count, Caprini Risk Score, Nutritional Risk Screening 2002 score, low eosinophil count, medium white blood cell count, and the Glasgow Coma Scale score. The CatBoost model accurately predicted the occurrence of HAIs and could be used in clinical practice.
The prevalence of healthcare-associated infections (HAIs) in intensive care units (ICUs) remains high owing to the use of invasive procedures (1), prolonged antibiotic use, and critical patient conditions, having a negative impact on quality of life and healthcare costs (2–5). Early identification and quantification of the risk of HAIs, coupled with timely interventions, are critical for reducing the incidence of HAIs and improving patient outcomes (6). Machine learning (ML) technology has emerged as a useful tool among healthcare professionals for predicting prognosis and making treatment decisions. Use of ML applications in ICU research has shown a 22.2% improvement in predicting complications (7). Although existing research often relies on hospitalization data for infection prediction, early initiation of infection control measures is important to improving patient outcomes (8–10). This study aimed to use indicators collected within 24 h of admission to the ICU using ML technology to develop infection prediction models with an earlier predictive window. Notably, the enhanced predictability of ML models, albeit at the expense of interpretability, makes it a reliable tool for predicting specific medical conditions.
Previous studies have used ML techniques to refine risk prediction in the healthcare context. Within ICUs, the Simplified Acute Physiology Score (SAPS) II has shown moderate accuracy for predicting HAIs and 7-day mortality (11). However, combining SAPS II with additional patient characteristics using support vector machine algorithms increased the predictive accuracy for both outcomes (12). In the neonatal ICU, a generalized mixed-effects regression tree with random intercept (GMERT-RI) model identified key predictors of HAIs, highlighting the importance of healthcare-associated factors in addition to neonate-specific factors (13). These findings highlight the potential of ML models to refine risk prognostication in critical care environments and facilitate targeted interventions to improve patient outcomes.
Early identification of HAI risk, enables early intervention; however, relatively few studies have focused on predicting HAIs using data collected within 24 h of ICU admission. This retrospective study used data from patients admitted to the ICU, focusing on the clinical characteristics of patients who developed infections during hospitalization. Multiple ML techniques were used to develop infection prediction models, leading to the development of a comprehensive model for training. These models identified significant predictors and key determinants which can be used the help healthcare professionals in the early identification of infection risk among patients admitted to ICUs, facilitating timely intervention.
The study participants were patients admitted to the ICU of a tertiary grade A comprehensive hospital in China between January 2019 and December 2023, with a total of 3,173 patients. We retrospectively analyzed the patients’ demographic characteristics, blood test results, nursing scores, and invasive clinical procedures within 24 h of admission. The data on patient demographics and invasive clinical procedures were extracted from the infection information monitoring system of Xinglin Hospital, blood markers were extracted from the Ruimei laboratory management system, and nursing scores were extracted from the intensive care information system.
The inclusion criteria were: 1. ICU admissions between January 2019 and December 2023; 2. Hospital stays exceeding 48 h; 3. The data within 24 h of ICU admission; 4. Infection-related data including occurrence, site, and pathogens throughout the hospitalization period. According to the “HAIs Diagnosis Criteria (Trial)” (14) in China, HAIs that occur in the hospital without a clear incubation period are classified as HAIs if they manifest 48 h or more after admission. Consequently, the 48-h timeframe serves as a critical criterion for identifying hospital infections. This study focused on patients who were admitted to the ICU for more than 48 h, in adherence to this guideline.
The exclusion criteria were: 1. Exclusion of colonization, community-acquired infections, and contamination in infection diagnoses (N = 21); 2. Exclusion of patients with disputed infection diagnoses (N = 5); 3. Exclusion of patients not discharged by December 31, 2023 (N = 25); 4. Data incompleteness within 24 h of ICU admission exceeds 5.0% (N = 23). Infection diagnosis adhered to the “HAIs Diagnosis Criteria (Trial)” (14) standard, considering the detection of the same pathogen at the same site in the same patient during hospitalization as one infection.
Following screening, the study encompassed a total of 2,517 cases, including 455 instances of HAIs.
The data included 32 factors though to be relevant to predicting the infection risk among ICU patients. The patient characteristics included gender, age, and diabetes status. Blood test results included white blood cell (WBC) count, neutrophil count, monocyte count, lymphocyte count, eosinophil count, basophil count, red blood cell (RBC) count, hemoglobin level, RBC distribution width, hematocrit, mean corpuscular hemoglobin, mean corpuscular hemoglobin concentration, mean corpuscular volume, platelet count, C-reactive protein level, glucose level, albumin level, and total bilirubin level. The nursing scores consisted of the Braden score, Nutritional Risk Screening (NRS) 2002 score, Glasgow Coma Scale (GCS) score, Critical Care Pain Observation Tool (CPOT), Penetration Aspiration Scale (PAS), enteral feeding tolerance, Caprini Risk Score (CRS), and unplanned extubation assessment (2, 15, 16). Invasive procedures included mechanical ventilation, intravascular catheterization, and urinary catheterization. The value of each indicator was determined based on the first test or assessment conducted within 24 h of ICU admission. The primary outcome was the occurrence of HAIs among patients admitted to the ICU.
To respect and protect the legitimate rights and interests of the subjects, this research has been approved by the Ethics Committee of Qingdao Municipal Hospital (approval no: 2024-KY-020). The individual patient consent requirement is waived because the project does not affect clinical treatment of patients, poses no greater than minimal risk, and all protected health-sensitive information has been removed from the limited dataset used in this study.
In compliance with the specifications outlined in the WS/T 640—2018 Clinical Microbiology Examination: Sample Collection and Transportation (17), clinical specimens were collected for culture from patients with suspected HAIs. Bacteria were identified using fully automated microbial identification and drug sensitivity analysis systems such as VITEK 2 (bioMérieux, Marcy-L’Étoile, France) or matrix-assisted laser desorption ionization time-of-flight mass spectrometry (MALDI-TOF MS; Bruker Daltonics, GmbH, Bremen, Germany). In patients with more than one bacterial strain isolated from various specimen types during their ICU admission, only the first pathogen identified was included in the analysis.
During the data processing phase, the researchers performed data anonymization to ensure that evaluators could not access background information related to the sample results. The data for Neutrophil Count, glucose levels, albumin levels, total bilirubin levels, and C-Reactive Protein have some missing values; however, the missing data for each is below 5.0%. The researchers filled in these missing values using the mean of the respective columns. This study used chi-square tests and t-tests to assess the statistical significance of differences in categorical and continuous variables, respectively, to identify predictors of infection in patients with and without HAIs (18, 19), to mitigate the risk of overfitting and enhance the interpretability of the model. As hypothesis testing using chi-square tests and t-tests only assessed the statistical significance of differences between groups, and did not necessarily identify the best predictors, variable selection algorithms and cross-validation techniques were used for variable selection (20). Multiple methodologies were used to construct a prediction model that was both accurate and robust.
Data processing and analysis were performed using Python pandas, and Python Scikit-learn was used for model construction. OneHotEncoder was used to transform categorical variables into one-hot encoding (21), and StandardScaler was used to standardize numerical variables and enhance model performance and convergence speed (22). This study used ColumnTransformer to combine two distinct variable-processing methods in ML. The dataset is divided into training and testing sets with an 80–20 split. This means 80.0% of the data is used for training the model, and 20.0% is reserved for testing the model. This study used 10 commonly used machine learning models: Random Forest (23), eXtreme Gradient Boosting (XGBoost) (24), Multilayer Perceptron (MLP), Adaptive Boosting (AdaBoost), Logistic Regression (25), Light Gradient Boosting Machine (LightGBM) (26), Decision Tree (27), Naive Bayes, Neural Networks (28), and Categorical Boosting (CatBoost) (29). The least absolute shrinkage and selection operator (LASSO) was used for variable selection (23). The predictive performance of each model was assessed using the area under the receiver operating characteristic (ROC) curve (AUC), sensitivity, specificity, and Youden’s index (30, 31). In this study, the optimal thresholds for the best model were determined using Youden’s J statistic in the test dataset. Additionally, all model output results were generated by an automated system, which further minimized the potential for human intervention, thereby enhancing the reliability of the evaluation results. The code has been shared on GitHub, please visit https://github.com/aarontroy/HAIs-prediction-model.git.
A total of 2,517 patients, including 1,544 male and 973 female patients with a mean age of 66.191 ± 19.067 years, met the eligibility criteria and were included in the analysis. Of the patients, 706 had been diagnosed with diabetes. In this cohort, 455 patients (18.1%, 309 male and 146 female patients; mean age 70.160 ± 18.403 years) developed HAIs (Table 1). Comparison of the characteristics of patients with and without HAIs using chi-square tests and t-tests, identified 18 statistically significant variables including gender, WBC count, RBC count, lymphocyte count, hemoglobin level, total bilirubin level, RBC distribution width, hematocrit, mean corpuscular hemoglobin concentration, mean corpuscular volume, eosinophil count, age, Braden score, NRS 2002 score, GCS score, PAS, enteral feeding tolerance, and the CRS.
Of the patients included in the analysis, 585 HAIs were identified in 455 patients, including 91 patients who experienced multiple episodes of HAI. HAIs were identified at 14 different sites. The top 5 infected sites were lower respiratory tract (28.7%, 168 cases), ventilator-associated pneumonia (25.0%, 146 cases), Bacteremia (19.3%, 113 cases), Catheter-associated urinary tract infection (9.6%, 56 cases) and Central line-associated bloodstream infection (5.5%, 32 cases) (Table 2).
Among the 455 patients with HAIs, 396 bacterial pathogens were grown on culture, an 87.0% detection rate. A total of 640 strains were identified, comprising 504 (78.8%) gram-negative bacteria, 94 (14.7%) gram-positive bacteria, and 42 (6.6%) fungi. Of the patients, 217 had a single pathogen identified, and 179 were infected with multiple pathogens. The top five pathogens identified were Acinetobacter baumannii (25.5%, 163 cases), Klebsiella pneumoniae (17.0%, 109 cases), Pseudomonas aeruginosa (14.1%, 90 cases), Enterobacter cloacae (5.2%, 33 cases), and Escherichia coli (4.8%, 31 cases) (Table 3).
The synthetic minority oversampling technique (SMOTE) algorithm was used to inflate the number of patients with HAIs from 445 to 2062, to align the size of the HAI cohort with that of the non- HAI cohort, thereby effectively mitigating class imbalance. This study categorized the model predictions into two groups based on whether oversampling was conducted, thus facilitating a comparative evaluation of the predictive performance.
The LASSO algorithm is a classic linear regression technique that is widely used for variable selection and dimensionality reduction. Compared with traditional linear regression methods, LASSO is capable of automatically selecting variables that have a marked effect on the target variable while preserving predictive accuracy (23). Consequently, this leads to a reduction in model complexity and an enhancement in generalization performance. In the analysis without SMOTE oversampling, the LASSO algorithm excluded the following variables: C-reactive protein, mean corpuscular hemoglobin concentration, GCS score, CPOT score, gastrointestinal tolerance to nutrition, and CRS. Conversely, in the analysis with SMOTE oversampling, the LASSO algorithm excluded gender, CRS, unplanned extubation assessment, CPOT score, diabetes, aspiration/asphyxia score, GCS score, and gastrointestinal tolerance to nutrition.
Typically, AUC is used as the primary metric for evaluating model performance owing to its comprehensive consideration of both true-positive and false-positive rates (Figure 1). According to the data provided, the Random Forest model demonstrated the best performance in terms of the AUC (0.958). However, given the 18.1% incidence of infection in the study hospital, sensitivity was considered the most important metric of model performance. Notably, the CatBoost model outperformed the others in terms of sensitivity (0.872) at detecting HAIs. In this context, the CatBoost model was considered the best choice (Table 4).
Figure 1. (A) The receiver-operating characteristic curves for ten ML models without undergoing SMOTE oversampling processing. (B) The ten-fold cross-validation results of the CatBoost model without SMOTE oversampling. (C) The receiver-operating characteristic curves for ten ML models with undergoing SMOTE oversampling processing. (D) The ten-fold cross-validation results of the CatBoost model with SMOTE oversampling.
Table 4. Evaluation metrics computation of machine learning models without or with undergoing SMOTE oversampling.
We conducted ten-fold cross-validation using the CatBoost classifier, training the model using preprocessed data (X_processed). In each fold of cross-validation, data splitting was performed using the StratifiedKFold method with 10 folds (n_splits = 10), ensuring data shuffling (shuffle = True) and maintaining consistency by setting a random seed (random_state = 42). Subsequently, the model was fitted to each training set (train) and the probability values were predicted using the corresponding test set (test). The mean AUC was 0.947 ± 0.008. These results highlight the excellent predictive performance and stability of the CatBoost model after SMOTE oversampling. In this study, the optimal thresholds for the CatBoost model were determined using Youden’s J statistic in the test dataset. For the NO_SMOTE group, the optimal threshold was calculated to be 0.558. This threshold allows the model to achieve the best balance between sensitivity and specificity. In the SMOTE group, which addresses class imbalance issues, the optimal threshold was found to be 0.581. This higher threshold helps to further enhance the model’s performance on imbalanced datasets.
A model was developed to identify factors contributing to HAIs in ICU patients using the CatBoost method. Eighteen independent variables identified through single-factor analysis were used as inputs, and the occurrence of HAIs events was the dependent (outcome) variable. The SHapley Additive exPlanation (SHAP) values were used as a technique to elucidate model predictions, to assist with understanding of individual variable contributions to model predictions. The SHAP values of each selected variable was visualized using violin plots, to reveal the distribution of the impact of variable on the model output. The width of the violin shape denotes the distribution of the SHAP values for the variable; a broader shape signifies a wider range of effects and potentially more diverse effects of the selected predictor variables. In the CatBoost model, the top 10 predictors of HAIs and their SHAP values were PAS (0.470), Braden score (0.470), high total bilirubin level (0.465), female (0.414), high WBC count (0.387), CRS (0.345), NRS 2002 score (0.321), low eosinophil count (0.318), medium WBC count (0.284), and GCS score (0.269) (Figure 2).
This study devised an algorithmic model for predicting the occurrence of HAIs in patients admitted to the ICU. CatBoost was used as the ML model and trained on the full dataset containing 32 variables and a dataset containing 18 selected variables. Subsequent enhancement of model performance was achieved by preprocessing the data using SMOTE oversampling. By using the SHAP method to interpret the findings, this study attained interpretability and transparency in the HAI outcomes predicted using ML at both the population and individual level. The use of ML to predict the occurrence of HAIs has potential application for early alerts and preemptive strategies, facilitating personalized treatment regimens and resource distribution, streamlining the clinical decision-making process and management protocols, and promoting advancements in the quality and safety of medical care.
Machine learning has gradually been applied to clinical sample analysis to handle variable data related to patient infection status (6). AI and ML technologies are expected to develop hospital infection monitoring algorithms to identify risk factors, improve patient risk stratification, track transmission pathways, and achieve real-time infection detection. Electronic health data plays a critical role in this process and is increasingly accessible. Advanced data management systems support real-time decision-making, aiding in automated hospital infection monitoring (32, 33). In the ICU, ML-supported clinical decision research focuses on monitoring, diagnosing, early identification of clinical events, outcome prediction, and prognosis assessment, to assist doctors, researchers, and policymakers in making treatment decisions. Currently, numerous ML models are used to predict risks of VAP, CLABSI, SSI, and hospital-acquired MDR pathogens, with particular emphasis on predicting sepsis and septic shock (6). In hospital infection risk prediction, clinical oversight is crucial to prevent applying machine learning systems to new data that differs from the training data (34), thus minimizing discrepancies between prediction results and actual clinical assessments (35).
The incidence rate of HAIs in ICUs is generally high, which may lead to a prolonged hospital stay and increased disease burden for patients. In this study, the incidence of infection in the ICU was 18.1%. Other studies have reported an incidence of HAIs in ICUs ranging from 8.0 to 60.0% (2, 36). The incidence of HAIs related to invasive procedures ranges from 25.0 to 50.0%, with patients in the ICU having a risk of HAIs 5 to 10 times higher than that in patients in general wards (37). Urinary tract infections related to catheterization in the ICU can increase the mortality rate by approximately 10.0% and prolong the length of hospital stay by an average of 10 days (38). Among the pathogens detected in this study, gram-negative bacteria were predominant, accounting for 78.8% of the cases. This finding is slightly higher than the 75.3% reported by Wang et al. (2) and substantially higher than the 67.4% reported by Li et al. (3) and the 57.4% reported by Cabrera-Tejada et al. (39). HAIs present a significant challenge in the management of ICU patients, often resulting in serious complications. HAIs can significantly affect patient outcomes, leading to increased complexity of treatment, prolonged hospital stays, an increased risk of bacterial resistance, increased mortality rates, an increased risk of disability, and higher healthcare costs (4, 6). In a study conducted by Cabrera-Tejada et al. (4), of patients admitted to the ICU and undergoing invasive mechanical ventilation for over 48 h, the mean length of hospital stay increased by 13.6 days, with an additional treatment cost of 20,965 Euros in patients with HAIs. These findings highlight the substantial burden HAIs pose on healthcare systems and patient outcomes, and the importance of effective prevention and management strategies in ICU setting (4).
Refining unbalanced datasets can enhance the accuracy of classification models used in scientific research. Cleaning unbalanced datasets can enhance the accuracy of classification models (40). The study revealed that compared with random under-sampling and oversampling techniques, predictions using the clustered under-sampling method led to more precise predictions of mortality rates (40). There are three common methods for addressing imbalanced ML data: data-level, algorithm-level, and hybrid approaches (41). In data-level methods, researchers modify the training dataset to suit the classifier algorithms, such as oversampling or under-sampling. Algorithm-level methods involve adjusting existing learners to reduce the bias toward the majority of the population, such as using cost-sensitive methods (41). This study aimed to predict HAIs by applying various balancing methods to the baseline data of ICU patients collected within 24 h of admission. In medical datasets, the records in the minority class are often more crucial than those in the control class. Therefore, addressing imbalanced data is essential to enhance the HAI identification rate. In addition to using SMOTE for oversampling, this study incorporated the LASSO operator for variable selection to reduce model complexity and enhance generalization capabilities.
The optimal model for predicting HAIs risk varies depending on the research context. Zhang et al. (18) found that the Naive Bayes model performed best in predicting surgical site infections after spinal surgery, with a mean AUC of 0.95, a sensitivity of 0.78, a specificity of 0.88, and an accuracy of 0.87. Cho et al. (42) have developed an ML model to monitor site infections during colon surgery. Their study revealed that neural networks using recursive variable elimination with 29 variables showed the best performance, achieving an AUC of 0.963. Previous studies have identified age, hemoglobin level, WBC count, and neutrophil count as predictors of catheter-related bloodstream infections using the XGBoost model (6), consistent with the findings of this study.
Owing to the increased risk of aspiration in ICU patients, inhalation of oropharyngeal secretions or vomitus can easily contaminate respiratory tract hygiene, leading to an increased risk of lower respiratory tract infections (43). Therefore, in patients with high PAS scores, the head of the bed should be elevated by 30° to 45° to reduce the risk of aspiration (43). In this study, the Braden score was identified as another predictor of HAI occurrence. Ding et al. (44) found that a low Braden score was an independent risk factor for stroke-associated pneumonia (SAP) after spontaneous intracerebral hemorrhage, showing moderate effectiveness in predicting SAP. In a study of chemotherapy-related bacterial infections, Jin et al. (45) found that an NRS 2002 score ≥ 3 was an independent risk factor; therefore, improving nutritional status could reduce the occurrence of bacterial infections. The findings of the studies by Ding et al. and Jin et al. were similar to the results of this study. It is crucial to monitor the scores of patients within 24 h of ICU admission and to intervene effectively if indicated.
This study has some limitations. The data were from a single center; therefore, the generalizability of the results may be limited. Multicenter studies should be conducted to enable a more thorough examination of variations according to region, demographics, and clinical practice. The research subjects for hospital infection monitoring using machine learning methods can be tens of thousands of people, which makes it more convenient for training and testing AI algorithms (46). Moreover, using the predictive model in real-world clinical settings, accompanied by real-time performance monitoring, is essential. Furthermore, strategies need to be devised for seamlessly integrating the model into existing medical information systems to facilitate easy access to and use of the predicted outcomes by clinicians. Further research could include additional variables associated with HAIs, such as demographic information (e.g., height and weight), vital sign (e.g., pulse, respiration, blood pressure, and temperature), and laboratory measurements (e.g., arterial blood gases) (47). The only pre-existing medical condition considered in this study was a history of diabetes. Future research should explore additional pre-existing medical conditions as potential predictors of HAIs.
This study presents the development and analysis of an ML algorithm aimed at predicting the risk of HAIs using data on patients admitted to an ICU collected within 24 h of admission, as predictors. Through a meticulous examination of the ranking of predictors derived from the ML model, this study identified key risk factors associated with HAIs, facilitating the identification of at-risk patients and the formulation of personalized treatment strategies. Future studies should include additional potential predictor variables, multicenter data, and a larger sample size to enhance the accuracy of prediction outcomes.
The raw data supporting the conclusions of this article will be made available by the authors, without undue reservation.
The studies involving humans were approved by Ethics Committee of Qingdao Municipal Hospital (approval no: 2024-KY-020). The studies were conducted in accordance with the local legislation and institutional requirements. The ethics committee/institutional review board waived the requirement of written informed consent for participation from the participants or the participants’ legal guardians/next of kin because this project does not affect clinical treatment of patients, poses no greater than minimal risk, and all protected health-sensitive information has been removed from the limited dataset used in this study.
JW: Investigation, Project administration, Writing – original draft, Writing – review & editing. GW: Conceptualization, Formal analysis, Methodology, Software, Supervision, Visualization, Writing – original draft, Writing – review & editing. YujW: Resources, Supervision, Writing – review & editing. YunW: Resources, Supervision, Writing – review & editing.
The author(s) declare that no financial support was received for the research, authorship, and/or publication of this article.
We would like to thank Editage (www.editage.cn) for English language editing.
The authors declare that the research was conducted in the absence of any commercial or financial relationships that could be construed as a potential conflict of interest.
All claims expressed in this article are solely those of the authors and do not necessarily represent those of their affiliated organizations, or those of the publisher, the editors and the reviewers. Any product that may be evaluated in this article, or claim that may be made by its manufacturer, is not guaranteed or endorsed by the publisher.
The Supplementary material for this article can be found online at: https://www.frontiersin.org/articles/10.3389/fpubh.2024.1444176/full#supplementary-material
1. Migliara, G, Di Paolo, C, Barbato, D, Baccolini, V, Salerno, C, Nardi, A, et al. Multimodal surveillance of healthcare associated infections in an intensive care unit of a large teaching hospital. Ann Ig. (2019) 31:399–413. doi: 10.7416/ai.2019.2302
2. Wang, Y, Ren, J, Yao, Z, Wang, W, Wang, S, Duan, J, et al. Clinical impact and risk factors of intensive care unit-acquired nosocomial infection: a propensity score-matching study from 2018 to 2020 in a teaching Hospital in China. Infect Drug Resist. (2023) 16:569–79. doi: 10.2147/IDR.S394269
3. Li, RJ, Wu, YL, Huang, K, Hu, XQ, Zhang, JJ, Yang, LQ, et al. A prospective surveillance study of healthcare-associated infections in an intensive care unit from a tertiary care teaching hospital from 2012-2019. Medicine. (2023) 102:e34469. doi: 10.1097/MD.0000000000034469
4. Gastmeier, P, Kola, A, Schwab, F, Behnke, M, and Geffers, C. Etiology of nosocomial infections in intensive care patients in German hospitals: an analysis of trends between 2008 and 2022. Int J Med Microbiol. (2024) 314:151594. doi: 10.1016/j.ijmm.2023.151594
5. Wang, Z, Du, M, Cao, H, Yao, H, Liu, B, Bai, Y, et al. Epidemiology and risk factors of nosocomial infections in a Chinese tertiary-care hospital: a 10-year retrospective case-control study. Infect Dis. (2024) 56:320–9. doi: 10.1080/23744235.2024.2310647
6. Baddal, B, Taner, F, and Ozsahin, DU. Harnessing of artificial intelligence for the diagnosis and prevention of hospital-acquired infections: a systematic review. Diagnostics. (2024) 14:484. doi: 10.3390/diagnostics14050484
7. Biesheuvel, LA, Dongelmans, DA, and Elbers, PWG. Artificial intelligence to advance acute and intensive care medicine. Curr Opin Crit Care. (2024) 30:246–50. doi: 10.1097/MCC.0000000000001150
8. Samadani, A, Wang, T, van Zon, K, and Celi, LA. VAP risk index: early prediction and hospital phenotyping of ventilator-associated pneumonia using machine learning. Artif Intel Med. (2023) 146:102715. doi: 10.1016/j.artmed.2023.102715
9. Frondelius, T, Atkova, I, Miettunen, J, Rello, J, Vesty, G, Chew, HSJ, et al. Early prediction of ventilator-associated pneumonia with machine learning models: a systematic review and meta-analysis of prediction model performance. Eur J Intern Med. (2024) 121:76–87. doi: 10.1016/j.ejim.2023.11.009
10. Gao, T, Nong, Z, Luo, Y, Mo, M, Chen, Z, Yang, Z, et al. Machine learning-based prediction of in-hospital mortality for critically ill patients with sepsis-associated acute kidney injury. Ren Fail. (2024) 46:2316267. doi: 10.1080/0886022X.2024.2316267
11. Barchitta, M, Maugeri, A, Favara, G, Riela, PM, Gallo, G, Mura, I, et al. A machine learning approach to predict healthcare-associated infections at intensive care unit admission: findings from the SPIN-UTI project. J Hosp Infect. (2021) 112:77–86. doi: 10.1016/j.jhin.2021.02.025
12. Barchitta, M, Maugeri, A, Favara, G, Riela, PM, Gallo, G, Mura, I, et al. Early prediction of seven-day mortality in intensive care unit using a machine learning model: results from the SPIN-UTI project. J Clin Med. (2021) 10:992. doi: 10.3390/jcm10050992
13. Beltempo, M, Bresson, G, and Lacroix, G. Using machine learning to predict nosocomial infections and medical accidents in a NICU. Heal Technol. (2023) 13:75–87. doi: 10.1007/s12553-022-00723-1
14. Ministry of Health of the People’s Republic of China. Healthcare-associated infections diagnosis criteria (trial). Nat Med J China. (2001) 5:61–7.
15. Kumpf, O, Assenheimer, M, Bloos, F, Brauchle, M, Braun, JP, Brinkmann, A, et al. Quality indicators in intensive care medicine for Germany—fourth edition 2022. Ger Med Sci. (2023) 21:Doc10. doi: 10.3205/000324
16. Zhang, Q, Wang, J, Liu, G, and Zhang, W. Artificial intelligence can use physiological parameters to optimize treatment strategies and predict clinical deterioration of sepsis in ICU. Physiol Meas. (2023) 44:15003. doi: 10.1088/1361-6579/acb03b
17. National Health Commission of the People’s Republic of China. Collection and transportation of clinical microbiology test samples. Available at: http://www.nhc.gov.cn/wjw/s9492/201812/f1c15b1b58bc45729f8f9afc164b7805.shtml
18. Zhang, Q, Chen, G, Zhu, Q, Liu, Z, Li, Y, Li, R, et al. Construct validation of machine learning for accurately predicting the risk of postoperative surgical site infection following spine surgery. J Hosp Infect. (2024) 146:232–41. doi: 10.1016/j.jhin.2023.09.024
19. Haskins, IN, Tamer, R, Phillips, SE, Thorson, FC, Kothari, VM, and Perez, AJ. Association of active smoking on 30-day wound events and additional morbidity and mortality following inguinal hernia repair with mesh: an analysis of the ACHQC database. Hernia. (2024) 28:33–42. doi: 10.1007/s10029-023-02886-w
20. Rafalko, N, Webster, JL, Jacob, G, Kutzler, MA, and Goldstein, ND. Generalizability of predictive models for Clostridioides difficile infection, severity and recurrence at an urban safety-net hospital. J Hosp Infect. (2024) 146:10–20. doi: 10.1016/j.jhin.2024.01.001
21. Shrivastava, T, Singh, V, and Agrawal, A. Autism spectrum disorder detection with kNN imputer and machine learning classifiers via questionnaire mode of screening. Health Inform Sci Syst. (2024) 12:18. doi: 10.1007/s13755-024-00277-8
22. Saeed, MH, and Hama, JI. Cardiac disease prediction using AI algorithms with SelectKBest. Med Biol Eng Comput. (2023) 61:3397–408. doi: 10.1007/s11517-023-02918-8
23. Sun, X. Supervised machine learning for exploratory analysis in family research. J Marriage Fam. (2024). doi: 10.1111/jomf.12973
24. Chen, T, and Guestrin, C. XGBoost: a scalable tree boosting system. in: kdd’16: proceedings of the 22nd acm sigkdd international conference on knowledge discovery and data mining. assoc comp machinery; assoc comp machinery sigmod; Assoc Comp Machinery SIGKDD; (2016). p. 785–794.
25. Zhang, Y, Xu, J, Zhang, C, Zhang, X, Yuan, X, Ni, W, et al. Community screening for dementia among older adults in China: a machine learning-based strategy. BMC Public Health. (2024) 24:1206. doi: 10.1186/s12889-024-18692-7
26. Ke, G, Meng, Q, Finley, T, Wang, T, Chen, W, Ma, W, et al. LightGBM: a highly efficient gradient boosting decision tree In: I Guyon, U Luxburg, S Bengio, H Wallach, R Fergus, and S Vishwanathan, et al., editors. Proceedings of the 31st international conference on neural information processing systems (NIPS’17). Red Hook, NY, USA:Curran Associates Inc, (2017) 3149–3157.
27. Musa, F, and Prasad, R. Predicting preeclampsia using principal component analysis and decision tree classifier. Curr Womens Health Rev. (2024) 20:14–15. doi: 10.2174/1573404820666230227120828
28. Monteverde-Suarez, D, Gonzalez-Flores, P, Santos-Solorzano, R, Garcia-Minjares, M, Zavala-Sierra, I, de la Luz, VL, et al. Predicting students’ academic progress and related attributes in first-year medical students: an analysis with artificial neural networks and Naïve Bayes. BMC Med Educ. (2024) 24:74. doi: 10.1186/s12909-023-04918-6
29. Prokhorenkova, L, Gusev, G, Vorobev, A, Dorogush, AV, and Gulin, A. CatBoost: unbiased boosting with categorical features In: S Bengio, H Wallach, H Larochelle, K Grauman, N CesaBianchi, and R Garnett, editors. Proceedings of the 32nd international conference on neural information processing systems (NIPS'18). Red Hook, NY, USA: Curran Associates Inc. (2018) 31:6639–6649.
30. Mungan, I, Bektas, S, Cavus, MA, Sari, S, and Turan, S. The predictive power of SAPS-3 and SOFA scores and their relations with patient outcomes in the surgical intensive care unit. Turkish J Surg. (2019) 35:124–30. doi: 10.5578/turkjsurg.4223
31. Martos-Benitez, FD, Larrondo-Muguercia, H, Leon-Perez, D, Rivero-Lopez, JC, Orama-Requejo, V, and Martinez-Alfonso, JL. Performance of three prognostic models in critically ill patients with cancer: a prospective study. Int J Clin Oncol. (2020) 25:1242–9. doi: 10.1007/s10147-020-01659-0
32. Hong, N, Liu, C, Gao, J, Han, L, Chang, F, Gong, M, et al. State of the art of machine learning–enabled clinical decision support in intensive care units: literature review. JMIR Med Inform. (2022) 10:e28781. doi: 10.2196/28781
33. De Corte, T, Van Hoecke, S, and De Waele, J. Artificial intelligence in infection management in the ICU. Crit Care. (2022) 26:79. doi: 10.1186/s13054-022-03916-2
34. Ferrara, M, Bertozzi, G, Di Fazio, N, Aquila, I, Di Fazio, A, Maiese, A, et al. Risk management and patient safety in the artificial intelligence era: a systematic review. Healthcare. (2024) 12:549. doi: 10.3390/healthcare12050549
35. Zhu, Y, Simon, GJ, Wick, EC, Abe-Jones, Y, Najafi, N, Sheka, A, et al. Applying machine learning across sites: external validation of a surgical site infection detection algorithm. J Am Coll Surg. (2021) 232:963–971e1. e1. doi: 10.1016/j.jamcollsurg.2021.03.026
36. European Centre for Disease Prevention and Control. Healthcare-associated infections. (Accessed May 22, 2024). Available at: https://www.ecdc.europa.eu/en/healthcare-associated-infections
37. Stamm, WE. Catheter-associated urinary tract infections: epidemiology, pathogenesis, and prevention. Am J Med. (1991) 91:65S–71S. doi: 10.1016/0002-9343(91)90345-x
38. Al Nasser, W, El-Saed, A, Al-Jardani, A, Althaqafi, A, Alansari, H, Alsalman, J, et al. Rates of catheter-associated urinary tract infection in tertiary care hospitals in 3 Arabian gulf countries: a 6-year surveillance study. Am J Infect Control. (2016) 44:1589–94. doi: 10.1016/j.ajic.2016.06.030
39. Cabrera-Tejada, GG, Chico-Sánchez, P, Gras-Valentí, P, Jaime-Sánchez, FA, Galiana-Ivars, M, Balboa-Esteve, S, et al. Estimation of additional costs in patients with ventilator-associated pneumonia. Antibiotics. (2024) 13:2. doi: 10.3390/antibiotics13010002
40. Karajizadeh, M, Nasiri, M, Yadollahi, M, Zolfaghari, AH, and Pakdam, A. Mortality prediction from hospital-acquired infections in trauma patients using an unbalanced dataset. Healthc Inform Res. (2020) 26:284–94. doi: 10.4258/hir.2020.26.4.284
41. Krawczyk, B. Learning from imbalanced data: open challenges and future directions. Prog Artif Intell. (2016) 5:221–32. doi: 10.1007/s13748-016-0094-0
42. Cho, SY, Kim, Z, Chung, DR, Cho, BH, Chung, MJ, Kim, JH, et al. Development of machine learning models for the surveillance of colon surgical site infections. J Hosp Infect. (2024) 146:224–31. doi: 10.1016/j.jhin.2023.03.025
43. National Health Commission of the People’s Republic of China. Intensive Care Unit Hospital Infection Prevention and Control Guidelines. Available at: http://www.nhc.gov.cn/wjw/s9496/201701/1f9de66563304061a4fcd7f54a9399fb.shtml
44. Ding, Y, Ji, Z, Liu, Y, and Niu, J. Braden scale for predicting pneumonia after spontaneous intracerebral hemorrhage. Rev Assoc Med Bras. (2022) 68:904–11. doi: 10.1590/1806-9282.20211339
45. Jin, X, Wu, S, and Bai, Y. Risk factors and characteristics of bacterial infection during first-line chemotherapy for metastatic gastric or gastroesophageal junction adenocarcinoma. Support Care Cancer. (2022) 30:2121–9. doi: 10.1007/s00520-021-06557-3
46. Arzilli, G, De Vita, E, Pasquale, M, Carloni, LM, Pellegrini, M, Di Giacomo, M, et al. Innovative techniques for infection control and surveillance in hospital settings and long-term care facilities: a scoping review. Antibiotics. (2024) 13:77. doi: 10.3390/antibiotics13010077
Keywords: machine learning, prediction, risk factors, intensive care, healthcare-associated infections
Citation: Wang J, Wang G, Wang Y and Wang Y (2024) Development and evaluation of a model for predicting the risk of healthcare-associated infections in patients admitted to intensive care units. Front. Public Health. 12:1444176. doi: 10.3389/fpubh.2024.1444176
Received: 05 June 2024; Accepted: 28 August 2024;
Published: 12 September 2024.
Edited by:
Eleonora Cella, University of Central Florida, United StatesReviewed by:
Igor Tona Peres, Pontifical Catholic University of Rio de Janeiro, BrazilCopyright © 2024 Wang, Wang, Wang and Wang. This is an open-access article distributed under the terms of the Creative Commons Attribution License (CC BY). The use, distribution or reproduction in other forums is permitted, provided the original author(s) and the copyright owner(s) are credited and that the original publication in this journal is cited, in accordance with accepted academic practice. No use, distribution or reproduction is permitted which does not comply with these terms.
*Correspondence: Gan Wang, Z2Fud2FuZzIxQG0uZnVkYW4uZWR1LmNu
†These authors have contributed equally to this work
Disclaimer: All claims expressed in this article are solely those of the authors and do not necessarily represent those of their affiliated organizations, or those of the publisher, the editors and the reviewers. Any product that may be evaluated in this article or claim that may be made by its manufacturer is not guaranteed or endorsed by the publisher.
Research integrity at Frontiers
Learn more about the work of our research integrity team to safeguard the quality of each article we publish.