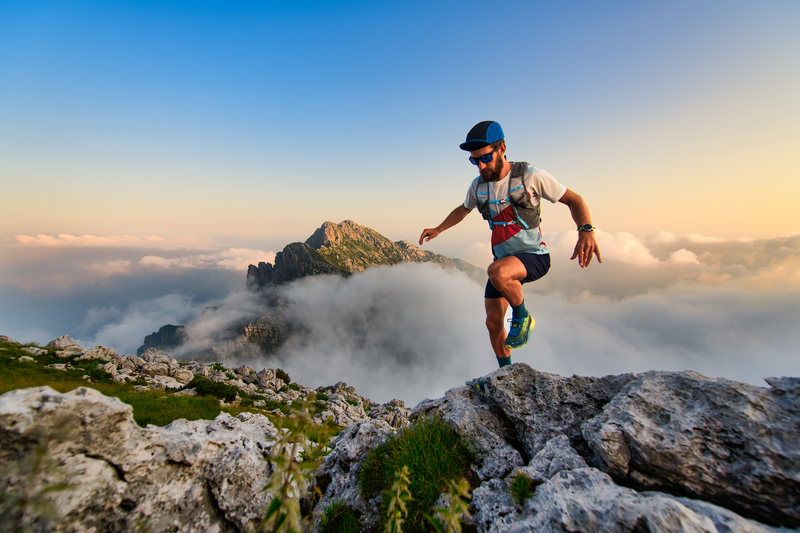
95% of researchers rate our articles as excellent or good
Learn more about the work of our research integrity team to safeguard the quality of each article we publish.
Find out more
ORIGINAL RESEARCH article
Front. Public Health , 13 November 2024
Sec. Public Mental Health
Volume 12 - 2024 | https://doi.org/10.3389/fpubh.2024.1439058
Purpose: This study aimed to determine the impact of job fitness on depression and depressive symptoms in Korean middle-aged and older population.
Methods: We collected data from the Korean Longitudinal Study of Aging (KLoSA), and performed a longitudinal analysis on 3,185 individuals with jobs at baseline. The dependent variable, depressive symptoms was measured by CES-D10 score, and the main variable of interest, job fitness was classified into nine groups according to job satisfaction and education level. To determine whether the estimate of depressive symptoms over the past week and depression changed over time, we applied the Generalized Estimating Equation (GEE) model.
Results: In the fully adjusted model, the impact of job fitness on depression and depressive symptoms was statistically significant. Compared to those with a suitable job level and a medium educational level, those with a large gap between job and educational levels were more likely to develop depression and depressive symptoms. It was also found that satisfaction with job content was a crucial factor affecting depression in the middle-aged and older adults.
Conclusion: Our findings demonstrated that job fitness significantly influenced depression, even after accounting for the effect of job satisfaction caused by a mismatch in job-education fitness. Since work demands and responsibility are difficult to quantify or qualify, job-education fitness could serve as a valuable tool to predict the extent of depression deterioration in individuals.
Depression or major depressive disorder (MDD), is a disease characterized by mood and mentality disorders associated with a negative impact on behavior, loss of interest, and emotional and physical problems which can lead to the degradation of an individual’s activity once enjoyed and ability to function at work and at home (1). Besides, depressive symptoms are positively correlated with the occurrence of suicide (2, 3) and chronic diseases (4, 5) and in terms of health scores, it is worse than in patients with chronic diseases (6).
The increase in depression occurrence is a global leading cause of disability and a great contributor to the global burden of disease (7). Many MDD occur after repetitive and persistent depressive symptoms, and indicators of the CESD scale include the experience of depressive symptoms (8). This, along with anxiety disorders, is an important catalyst for in not only depression but also schizophrenia, mental disorders, diabetes, and suicide (9). Depression is caused by biochemical, genetic, individual, and environmental factors as well as stress or changes in daily life and socioeconomic (SES) levels (10). Among various studies, it was found that low education levels, recent negative life events, and loneliness affected the overall life span and income; while, child abuse, and unhealthy aspects including pain, BMI and number of chronic diseases seemed more strongly associated with depression and depressive syndrome in older ages (11, 12). It was also known that physical activity and exercise volume have a significant impact on depressive symptoms and mental health (13, 14). In addition, financial dependence and work environment were the most important factors involved in the onset of depressive syndrome (12).
Based on several studies on depression as above, there is a lot of various view on depression. Many studies also focused on the impact of relationship between occupation and mental health such as job stress (15), depression and depressive symptoms in specific occupations (16–18), and working conditions on mood disorder (19). There were also studies focused on the importance of educational level found that the area, the learning content and focus, afterschool activities were all factors affecting not only the individuals’ knowledge but also their personality and mental health (20–22). Freeman et al. (23) also used combined scores of socioeconomic variables to measure their effect on the onset of depression.
Based on the knowledge available regarding job and education as single factors, we decided to analyze the effect of job education fitness on major depressive disorder. Job fitness refers to the degree of equilibrium between job and worker’s characteristics such as personality, knowledge, skills, and job performance (24). Currently, the health care industry focuses more on the job, work environment’ stress and specific health risk than job fitness. However, in the economic sector, the mismatch in job fitness is identified as a potential threat to the fourth industrial revolution and as a factor affecting workers’ wages, performance, productivity, and job satisfaction (25, 26). Job fitness should also be considered important in the health and medical fields because factors involved in the onset of depression, such as job satisfaction (27), wages (28), and job stress (15) can occur individually or together when work demands and personal attributes, such as education level, are not aligned. There are various forms of job fitness such as a genuine and apparent mismatch (29), real and formal mismatch (30), educational and skill mismatch (31).
In this study, we decided to study job-education mismatches because of two reasons. First, individuals tend to have better (or worse) jobs depending on their educational level (32), which can be measured through subjective responses comparing job level to educational level. Furthermore, depending on the position, workplace, and personal characteristics of the CEO, the job requirements can vary. Because job requirements cannot be quantified or qualified, disequilibrium between these factors has a negative impact on stress, satisfaction and so on. Second, generally, the standard of education depends on each country and is defined by a graduation system. For this reason, we think many countries can apply this mismatch of job-education by replacing the variables according to the country’s education system. In this study, the job satisfaction, which is correlated with fitness, is included as covariates in the analysis in order to identify the pure effect of job-education fitness on depression. In addition, we attempted to control as much as possible potential confounding factors that could affect depressive symptoms. Through these considerations, comparison between the job and the actual education level are utilized to present academic grounds on what job fitness means for depression and depressive symptoms by utilizing a large panel data.
We used the Korean Longitudinal Study of Aging (KLoSA) data to identify and analyze the effect of job fitness in relation to educational level on depression. The first wave of KLoSA occurred in 2006 and contained participants’ data for community-dwelling Koreans who were 45 years old and older. Since the first wave of KLoSA in 2006, follow up interviews were held every 2 years until 2018. Participants were selected randomly using a multistage, stratified probability sampling design based on the sampling frame. A sampling frame was set up by geographical areas and housing types across Korea to create a nationally representative sample. The data are composed of eight categories: population, family, health status, employment, income, assets, subjective expectations, and quality of life. This original KLoSA study population included South Korean adults 45 years or older who lived in 15 large administrative areas. In the first baseline survey, 10,254 individuals dispatched in 6,171 households (1.7 individuals per household) were surveyed using the computer-assisted personal interviewing method. In 2008, the second survey followed up with 8,688 subjects, representing 86.6% of the original panel. In 2010, the third survey followed up with 7,920 subjects, representing 81.7% of the original panel. In 2012, the fourth survey followed up with 7,486 subjects, representing 80.1% of the original panel. In 2014, the fifth survey followed up with 7,029 subjects, representing 79.2% of the original panel and 920 new subjects included as a new panel. The size of the total sample was 7,949 subjects and represented 80.4% of the sample maintenance rate. In 2016, the sixth survey followed up with 7,490 subjects (including 872 new participants of the fifth), representing 79.6% of the sample maintenance rate. Finally, the seventh survey followed up with 6,940 subjects (including 804 new participants of the fifth), representing 78.8% of the sample maintenance rate. Due to data limitation, we excluded the first baseline survey which lacks the CES-D10 score variable.
Therefore, our study used data from six surveys of KLoSA (2nd to 7th). To identify and analyze the impact of job fitness and educational level on depression, unemployed and economically inactive research participants and unpaid family volunteers were excluded from the study. Participants who had no information about variables or non-response or rejection of responses were also excluded from the study. The general characteristics of the subjects were analyzed using the second survey data from the KLoSA and resulted in the inclusion of a total of 3,185 participants, 2,138 male and 1,047 female workers.
The actual educational level of the subjects was categorized into three groups depending on their graduation level: “Low” for middle school or lower, “Medium” for high school, and “High” for college or higher.
In order to identify the subjective conception of current job levels according to the level of education, five-step measures were used according to the question, “How do you think the level of your occupation is compared to your level of education?”: (1) Very low; (2) Low; (3) Suitable; (4) High; (5) Very high. In this study, we classified responses into three categories: low (very low and low), Suitable, and high (high and very high).
To determine the job fitness, we intended to evaluate the difference between the current job level (relative to educational level) and the actual educational level. The difference between the two variables was classified as follows: Current Job level (compared to Educational level) – Actual Educational Level: Low-Low (≤middle school), Low-medium (high school), Low-High (≥college), Suitable-Low (≤middle school), Suitable-Medium (high school), Suitable-High (≥college), High-Low (≤middle school), High-Medium (high school), and High-High (≥college).
The occurrence of depressive symptoms was assessed for 7 days before the interview. Responder were asked about their level of depressive symptoms over the past week. This was classified as follows: (1) Less than a day; (2) 1–2 days; (3) 3–4 days; (4) 5–7 days. In this study, we classified responses into two categories: No (less than a day), Yes (1–7 days).
In this study, we applied the Modified Center for Epidemiologic Studies Depression Scale (CES-D) to measure depressive symptomatology (8). The CES-D scale remains one of the most widely used tools in the field of psychiatric epidemiology (33, 34). According to KLoSA’s data, 10 questions out of 20 in CES-D were selected for subjects of the 2nd to 4th wave according to the Andresen form (35). After the 5th wave, data were obtained according to the Boston form (36). The CES-D 10 scores were summed up according to the Korean version of CES-D. According to KLoSA, and the preceding study, the depression symptoms were measured with the total score of CES-D10 (37–39). Modified CES-D scale is total 10 points and we set 4points to the cutoff value of depression.
The covariates of this study are listed based on previous literature as follows (40–42): satisfaction with the job content, gender, age, marital status, self-rated health, number of chronic diseases, smoking status, drinking status, regular physical activity, and the modified CES-D10 form.: “satisfaction with the job content” was measured by a question that “I am satisfied with my job” and it was classified as follows: very satisfied, satisfied, not satisfied and not satisfied at all. “Gender” was categorized into two groups: male, female. “Age” was categorized into four groups: 45–54, 55–64, 65–74, and ≥ 75 (75 or older). “Marital status” categorized participants as married, separated, divorced, and ‘single’ (never married). For “Self-rated health,” participants were asked to rate their health status on a five point Likert scale (1 corresponding to very good and 5 to very bad). In this study, we categorized “Self-rated health” into three groups: good (very good & good), moderate (normal), bad (bad & very bad). The “Number of chronic diseases” is a covariate that refers to the number of chronic illnesses in participants such as high blood pressure, diabetes, cancer, chronic pulmonary disease, liver disease, cardiovascular disease, cerebrovascular disease, mental illnesses, and arthritis. Subjects were categorized into three groups: 0, 1–2, ≥3 (three or more). “Smoking status” referred to nonsmoker who never smoked, former smoker, or smoker and “Drinking status” to drinker, former drinker, and nondrinker. “Regular physical activity” was defined by regular exercise (more than once a week) and participants were categorized as yes or no.
In this study, we performed the chi-square test, the Generalized Estimating Equation (GEE) model, and a clustered model. For the GEE model, we used the proc genmod with link: log and logit, distribution: normal and binary to determine whether the estimate of depressive symptoms over the past week and depression changed over time. The regression coefficient was used to estimate both the change in depression symptoms and independent variables. Depression and the depressive symptoms over the past week where the outcomes in all GEE models. Statistical analysis was performed on a total of five periods each considered as a separate situation regardless of the participants’ number in the study itself. Covariates of interest from all subjects were added to the model to determine their effects on reporting depression. Satisfaction with one’s job is an important covariate, and the pattern of the association between current job suitability and depressive symptoms may vary accordingly, so a subgroup analysis by job satisfaction was performed to confirm this. All statistical analyses were performed using the SAS statistical software package, version 9.4 (SAS Institute, Inc., Cary, NC, United States). All statistical tests were two-tailed with a significance of p < 0.05.
The baseline general characteristics of participants are shown in Table 1. Of the total 3,185 subjects, 1,121 people had depression. The group with the most depression, was found for the group with a high degree of job level and a low degree of educational level (≤middle school). The baseline prevalence of depressive symptoms over the past week was 26.9% (857 participants) among all participants. We also observed a pattern with the job content satisfaction, the lower the satisfaction, the higher depression rate and the percentage of subjects with depressive symptoms in the past week. The depression rate was 14.8% for the most satisfied group and 51.7% for the most dissatisfied group. The proportion of participants with depressive symptoms in the past week was 9.1% (eight participants) in the most satisfied group.
In the fully adjusted model (Table 2), the association between current job fitness and depression was statistically significant, with the following odds ratio (OR) predicting increased depression: OR = 3.421 (p < 0.0001) for individuals with a high job level and a high educational level, OR = 2.194 (p = 0.008) for individuals with a high job level and a low educational level, and OR = 1.696 (p < 0.0001) for individuals with a low job level and a low educational level as compared to the group with a suitable job level and a medium educational level. We also found that the decrease in the degree of satisfaction was associated with an increase the OR of depression. Participants classified as “not satisfied at all” in job content obtained the OR of 3.029 (p < 0.0001) compared to individuals with very satisfying job content (Table 2).
In the fully adjusted model (Table 3), the association between current job fitness and depressive symptoms measured for a week was statistically significant. The following odds ratio (OR) predicted increased depressive symptoms: OR = 3.493 (p < 0.0001) for individuals with a high job level and a low educational level, OR = 2.261 for individuals with a high job level and a high educational level and OR = 1.515 (p < 0.0001) for individuals with a low job level and a low educational level as compared to the group of individuals with a suitable job level and a medium educational level. We also found that the decrease in the degree of satisfaction was associated with an increase in the odds ratio of depressive symptoms. Participants in the group classified as “not satisfied at all” in job content obtained an OR value of 2.537 (p < 0.0001) compared to the group with very satisfying job content (Table 3).
As shown in Table 4, in subgroup satisfied with their job, the association between job fitness and depression and depressive symptoms over past week was not statistically significant for the individuals with a high job level and high education level (OR = 1.933, p = 0.478, OR = 3.438, p = 0.186) and for the individuals with a low job level and a high educational level (OR = 0.744, p = 0.088, OR = 0.901, p = 0.566).
Table 4. The adjusted impact of job suitability on depression/depressive symptom in the past week by satisfaction with current work contents.
In this study, we identified the relevance between job fitness and depression by including the job level and the actual level of education of participants over six periods of KLoSA’s panel data (survey 2 in 2008 to 7 in 2018). According to our study, analyses of CES-D10 scores and depressive symptoms showed that low, high job levels, compared to a suitable job level, showed a negative impact on two indicators of depression. And the both showed a higher degree of depression at a High job level. We found that the probability of experiencing depressive symptoms increased as the job content became less satisfactory. Many similar study (26, 43, 44) suggest that job or education has a meaningful effect on depression from a socioeconomic perspective. In this study, we analyzed the association between job fitness (compared to educational level) and depression. In contrast to prior studies, we suggested that job-education fitness was one of the factors of depression. And our preliminary data showed that job-education fitness affect depression and that series of depression problems can occur when individuals think their job level is inappropriate with their level of education. This study supports previous research with additional evidence for the impact of job and education level on depression. This means that research with additional evidence for the impact of job and educational level on depression. This means that research analyzing depression must be considered through multi-dimensioned variables. Based on the results of Nordin et al. (45), an income penalty may result from a mismatch between the level of job and education. Additionally, Lee et al. (46) stated that income and depression were highly related. They showed that individuals with low income regardless of sex were more likely to develop Major Depressive Disorders (MDD) and that low income can serve as a significant risk factor of MDD. This is supported by our data showing how the gap between job and education levels affected depression in mismatched groups. According to these studies, job and educational levels may directly or indirectly affect depression because of income penalty, low income and so on. The design of the study did not allow us to find any difference between age-specified groups. Participants included in the surveys were all middle-aged or older individuals still engaged in economic activities. Our data were consistent with other study (11) showing that older people are more likely affected by socioeconomic factors including job-fitness.
Many studies investigating the effect of job stress on depression have demonstrated its adverse effect on mental health (15) in all areas regardless of certain ages, professions, nationalities, etc. (47–49). The Center for Disease Control and Prevention in the United States defined job stress as a physical and emotional adverse reaction that occurs when the requirements of work do not match capabilities, resources or requirements of the worker, and emphasized the working conditions and the personal characteristics of the worker. In this study, the results that can able to influence depression based on differences in the educational level of individuals and the job level required if individuals at work, we provided data to support our hypothesis regarding the impact of existing “Job Stress,” individual’s ability and characteristics on the occurrence of depression.
Similarly to the study of Lee et al. (50), the CES-D score, depression level, and proportion of depressive symptoms were consistent with the high values obtained for the group of subjects with a high job level. The CES-D score obtained for the high job level groups was getting higher as the educational level increased. This is consistent with the study (51) stating that people with higher educational levels felt more depressed according to their CES-D score. The implication of this study was consistent with our results: job-education is an important factor to assess the risk for depression, not just the job or level of education alone.
Lifestyle factors, including physical activity, sleep, and diet, can have a significant impact on depressive symptoms. Regular exercise helps alleviate these symptoms by boosting levels of endorphins, serotonin, and dopamine—neurotransmitters that foster a sense of well-being (52, 53). Sufficient sleep is also essential. Poor sleep quality or inadequate sleep can worsen depression by disrupting the balance of mood-related neurotransmitters and heightening inflammation, including markers like c-reactive protein and systemic inflammation (54, 55). Adopting consistent sleep routines and good sleep hygiene can bolster emotional resilience and lower the risk of depressive episodes. Diet is equally crucial. A well-balanced diet, rich in fruits, vegetables, whole grains, and lean proteins, provides vital nutrients that support brain function (56). Accordingly, future study will need to consider lifestyle as these factors can collectively have a profound impact on mental health, and optimizing them may be key strategy in managing and preventing depression.
In our study, the following limitations should be considered when interpreting the data. First, our data were obtained from KLoSA database which is a self-reported survey. This means that questions about related to CESD score, depressive symptoms, job level, educational level, and job content satisfaction may be affected by subjective responses, false consciousness, and recall bias. This self-reported information about health and symptoms is however critical because it complements objective measures (57). Second, the individual’s ability and characteristics are likely to relate to job level, educational level, and depression. This measure could lead to a potential exaggeration due to a possible failure to include this feature. Third, the education level has grown rapidly in Korea since the Enforcement of the Education Act in 1952. The results of this study may differ in a population composed of highly educated middle-aged and older adults living in OECD countries (58). However, the gap between job level and level of education is still expected to exist, not through the level of education itself about job fitness compared to educational level. Fourth, GEE operates under the assumption that the effect of predictors remains consistent over time, although this may not always hold true. As a result, it may not fully capture associations that fluctuate over time. Finally, although we used longitudinal data, the results may have reflected reverse causality and bidirectional relations in the association between job fitness and depression.
The strength of this study relies on the inclusion of two variables of socioeconomic factors, rather than a simple variable analysis. Unlike previous studies showing that job and education affect depression as independent variables, this study included two factors of socioeconomic level as multi-dimensional variables. Our analysis of the relevance between depression and multiple factors consisted of job level and educational level showed a greater impact on subjects with lower or higher job fitness. Furthermore, we found additional evidence regarding job suitability and job satisfaction and how they were also affected using calibrated variables. Finally, our solid results were obtained considering repeatable targets in the follow-up survey data representative of the Korean population.
In conclusion, unlike previous studies using simple measurements, this study identified the relationship between depression and the gap between job and educational level. This study provides important evidence with great implications for health management and suggests the need to properly monitor the mental health of subjects whose job fitness is unsuitable.
Publicly available datasets were analyzed in this study. This data can be found here: the dataset analyzed in the present study is publicly accessible. Available online at: https://survey.keis.or.kr/klosa/klosa04.jsp.
This study was based on publicly available datasets. Ethical review and approval was not required for the study, in accordance with the local legislation and institutional requirements.
IY: Conceptualization, Investigation, Validation, Visualization, Writing – original draft. S-HJ: Conceptualization, Methodology, Software, Writing – original draft. DC: Data curation, Methodology, Validation, Writing – review & editing. J-HK: Investigation, Project administration, Supervision, Validation, Visualization, Writing – review & editing. JM: Investigation, Methodology, Project administration, Supervision, Validation, Visualization, Writing – review & editing.
The author(s) declare that financial support was received for the research, authorship, and/or publication of this article. This study was supported by Gachon University, Gil Medical Center (grant number FRD2021-19).
The authors declare that the research was conducted in the absence of any commercial or financial relationships that could be construed as a potential conflict of interest.
All claims expressed in this article are solely those of the authors and do not necessarily represent those of their affiliated organizations, or those of the publisher, the editors and the reviewers. Any product that may be evaluated in this article, or claim that may be made by its manufacturer, is not guaranteed or endorsed by the publisher.
1. Vahia, VN . Diagnostic and statistical manual of mental disorders 5: a quick glance. Indian J Psychiatry. (2013) 55:220–3. doi: 10.4103/0019-5545.117131
2. Simon, GE, and Von Korff, M. Suicide mortality among patients treated for depression in an insured population. Am J Epidemiol. (1998) 147:155–60. doi: 10.1093/oxfordjournals.aje.a009428
3. Bostwick, JM, and Pankratz, VS. Affective disorders and suicide risk: a reexamination. Am J Psychiatry. (2000) 157:1925–32. doi: 10.1176/appi.ajp.157.12.1925
4. de Jonge, P, and Rosmalen, JG. Comment on: Knol MJ, Twisk JWR, Beekman ATF, Heine RJ, Snoek FJ, Pouwer F. (2006) depression as a risk factor for the onset of type 2 diabetes mellitus. A meta-analysis. Diabetologia. (2006) 49:2797–8. doi: 10.1007/s00125-006-0389-y
5. Voinov, B, Richie, WD, and Bailey, RK. Depression and chronic diseases: it is time for a synergistic mental health and primary care approach. Prim Care Companion CNS Disord. (2013) 15:26226. doi: 10.4088/PCC.12r01468
6. Moussavi, S, Chatterji, S, Verdes, E, Tandon, A, Patel, V, and Ustun, B. Depression, chronic diseases, and decrements in health: results from the world health surveys. Lancet. (2007) 370:851–8. doi: 10.1016/S0140-6736(07)61415-9
7. You, D, Hug, L, Ejdemyr, S, Idele, P, Hogan, D, Mathers, C, et al. United Nations inter-agency Group for Child Mortality E. Global, regional, and national levels and trends in under-5 mortality between 1990 and 2015, with scenario-based projections to 2030: a systematic analysis by the UN inter-agency Group for Child Mortality Estimation. Lancet. (2015) 386:2275–86. doi: 10.1016/S0140-6736(15)00120-8
8. Radloff, LS . The CES-D scale: a self-report depression scale for research in the general population. Appl Psychol Meas. (1977) 1:385–401. doi: 10.1177/014662167700100306
9. Buckley, PF, Miller, BJ, Lehrer, DS, and Castle, DJ. Psychiatric comorbidities and schizophrenia. Schizophr Bull. (2009) 35:383–402. doi: 10.1093/schbul/sbn135
11. Schaakxs, R, Comijs, HC, van der Mast, RC, Schoevers, RA, Beekman, ATF, and Penninx, B. Risk factors for depression: differential across age? Am J Geriatr Psychiatry. (2017) 25:966–77. doi: 10.1016/j.jagp.2017.04.004
12. Eaton, WW, Muntaner, C, Bovasso, G, and Smith, C. Socioeconomic status and depressive syndrome: the role of inter-and intra-generational mobility, government assistance, and work environment. J Health Soc Behav. (2001) 42:277–94. doi: 10.2307/3090215
13. Torabi, F, and Ahmadi, R. The effect of exercise volume on depressive-related behaviors and Levels of brain-derived neurotrophic factor and serum testosterone levels. Int J Sport Stud Health. (2024) 7:56–61. doi: 10.61838/kman.intjssh.7.3.8
14. Taheri, M . Enhancing cognitive abilities and delaying cognitive decline in the elderly through tailored exercise programs. Health Nexus. (2023) 1:67–77. doi: 10.61838/kman.hn.1.4.8
15. Iacovides, A, Fountoulakis, KN, Kaprinis, S, and Kaprinis, G. The relationship between job stress, burnout and clinical depression. J Affect Disord. (2003) 75:209–21. doi: 10.1016/s0165-0327(02)00101-5
16. Kawakami, N, Haratani, T, and Araki, S. Effects of perceived job stress on depressive symptoms in blue-collar workers of an electrical factory in Japan. Scand J Work Environ Health. (1992) 18:195–200. doi: 10.5271/sjweh.1588
17. Gallery, ME, Whitley, TW, Klonis, LK, Anzinger, RK, and Revicki, DA. A study of occupational stress and depression among emergency physicians. Ann Emerg Med. (1992) 21:58–64. doi: 10.1016/s0196-0644(05)82238-3
18. Ferguson, K, and Hall, D. Predicting teacher anxiety, depression, and job satisfaction. J Teach Learn. (2012) 8:27–42. doi: 10.22329/jtl.v8i1.2896
19. Woo, JM, and Postolache, TT. The impact of work environment on mood disorders and suicide: evidence and implications. Int J Disabil Hum Dev. (2008) 7:185–200. doi: 10.1515/ijdhd.2008.7.2.185
20. Dahmann, S, and Anger, S. The impact of education on personality: evidence from a German high school reform. IZA Discuss Paper. (2014) 8139:1–51. doi: 10.2139/ssrn.2432423
21. Xiu-Juan, Q, Li-Na, W, and Yu-Ying, D. A survey of anxiety/depress in infertile women. Matern Child Health Care Chin. (2008) 2:228–230.
22. Bjelland, I, Krokstad, S, Mykletun, A, Dahl, AA, Tell, GS, and Tambs, K. Does a higher educational level protect against anxiety and depression? The HUNT study. Soc Sci Med. (2008) 66:1334–45. doi: 10.1016/j.socscimed.2007.12.019
23. Freeman, A, Tyrovolas, S, Koyanagi, A, Chatterji, S, Leonardi, M, Ayuso-Mateos, JL, et al. The role of socio-economic status in depression: results from the COURAGE (aging survey in Europe). BMC Public Health. (2016) 16:1098. doi: 10.1186/s12889-016-3638-0
24. Edwards, JR . Person-job fit: a conceptual integration, literature review, and methodological critique. Int Rev Ind Organ Psychol. (1991) 6:283–357.
25. Kim, S-J, and Choi, SO. The effects of job mismatch on pay, job satisfaction, and performance. J Open Innov: Technol Mark Complex. (2018) 4:1–13. doi: 10.1186/s40852-018-0085-4
27. Faragher, EB, Cass, M, and Cooper, CL. The relationship between job satisfaction and health: a meta-analysis. Occup Environ Med. (2005) 62:105–12. doi: 10.1136/oem.2002.006734
28. Platt, J, Prins, S, Bates, L, and Keyes, K. Unequal depression for equal work? How the wage gap explains gendered disparities in mood disorders. Soc Sci Med. (2016) 149:1–8. doi: 10.1016/j.socscimed.2015.11.056
29. Chevalier, A . Measuring over-education. Economica. (2003) 70:509–31. doi: 10.1111/1468-0335.t01-1-00296
30. Green, F, and Zhu, Y. Overqualification, job dissatisfaction, and increasing dispersion in the returns to graduate education. Oxf Econ Pap. (2010) 62:740–63. doi: 10.1093/oep/gpq002
31. Allen, J, and Velden, RD. Educational mismatches versus skill mismatches: effects on wages, job satisfaction, and on-the-job search. Oxf Econ Pap. (2001) 53:434–52. doi: 10.1093/oep/53.3.434
32. Verhofstadt, E, and Omey, E. The impact of education on job satisfaction in the first job. Book Work Paper. (2003) 169:16.
33. Naughton, MJ, and Wiklund, I. A critical review of dimension-specific measures of health-related quality of life in cross-cultural research. Qual Life Res. (1993) 2:397–432. doi: 10.1007/BF00422216
34. Shin, J, Park, EC, Lee, SG, Choi, Y, Kim, JH, and Kim, TH. The cross-interaction between global and age-comparative self-rated health on depressive symptoms-considering both the individual and combined effects. BMC Psychiatry. (2016) 16:433. doi: 10.1186/s12888-016-1098-9
35. Andresen, EM, Malmgren, JA, Carter, WB, and Patrick, DL. Screening for depression in well older adults: evaluation of a short form of the CES-D (Center for Epidemiologic Studies Depression Scale). Am J Prev Med. (1994) 10:77–84. doi: 10.1016/S0749-3797(18)30622-6
36. Kohout, FJ, Berkman, LF, Evans, DA, and Cornoni-Huntley, J. Two shorter forms of the CES-D (Center for Epidemiological Studies Depression) depression symptoms index. J Aging Health. (1993) 5:179–93. doi: 10.1177/089826439300500202
37. Irwin, M, Artin, KH, and Oxman, MN. Screening for depression in the older adult: criterion validity of the 10-item Center for Epidemiological Studies Depression Scale (CES-D). Arch Intern Med. (1999) 159:1701–4. doi: 10.1001/archinte.159.15.1701
38. Mohebbi, M, Nguyen, V, JJ, MN, Woods, RL, Nelson, MR, Shah, RC, et al. Psychometric properties of a short form of the Center for Epidemiologic Studies Depression (CES-D-10) scale for screening depressive symptoms in healthy community dwelling older adults. Gen Hosp Psychiatry. (2018) 51:118–25. doi: 10.1016/j.genhosppsych.2017.08.002
39. Bjorgvinsson, T, Kertz, SJ, Bigda-Peyton, JS, McCoy, KL, and Aderka, IM. Psychometric properties of the CES-D-10 in a psychiatric sample. Assessment. (2013) 20:429–36. doi: 10.1177/1073191113481998
40. You, Y, Chen, Y, Liu, R, Zhang, Y, Wang, M, Yang, Z, et al. Inverted U-shaped relationship between sleep duration and phenotypic age in US adults: a population-based study. Sci Rep. (2024) 14:6247. doi: 10.1038/s41598-024-56316-7
41. You, Y, Li, J, Zhang, Y, Li, X, Li, X, and Ma, X. Exploring the potential relationship between short sleep risks and cognitive function from the perspective of inflammatory biomarkers and cellular pathways: insights from population-based and mice studies. CNS Neurosci Ther. (2024) 30:e14783. doi: 10.1111/cns.14783
42. You, Y, Mo, L, Tong, J, Chen, X, and You, Y. The role of education attainment on 24-hour movement behavior in emerging adults: evidence from a population-based study. Front Public Health. (2024) 12:1197150. doi: 10.3389/fpubh.2024.1197150
43. Levels, M, van der Velden, R, and Allen, J. Educational mismatches and skills: new empirical tests of old hypotheses. Oxf Econ Pap. (2014) 66:959–82. doi: 10.1093/oep/gpu024
44. Bauer, TK . Educational mismatch and wages: a panel analysis. Econ Educ Rev. (2002) 21:221–9. doi: 10.1016/S0272-7757(01)00004-8
45. Nordin, M, Persson, I, and Rooth, D-O. Education-occupation mismatch: is there an income penalty? Econ Educ Rev. (2010) 29:1047–59. doi: 10.1016/j.econedurev.2010.05.005
46. Lee, CT, Chiang, YC, Huang, JY, Tantoh, DM, Nfor, ON, Lee, JF, et al. Incidence of major depressive disorder: variation by age and sex in low-income individuals: a population-based 10-year follow-up study. Medicine (Baltimore). (2016) 95:e3110. doi: 10.1097/MD.0000000000003110
47. Wang, LJ, Chen, CK, Hsu, SC, Lee, SY, Wang, CS, and Yeh, WY. Active job, healthy job? Occupational stress and depression among hospital physicians in Taiwan. Ind Health. (2011) 49:173–84. doi: 10.2486/indhealth.ms1209
48. Rydmark, I, Wahlberg, K, Ghatan, PH, Modell, S, Nygren, A, Ingvar, M, et al. Neuroendocrine, cognitive and structural imaging characteristics of women on longterm sickleave with job stress-induced depression. Biol Psychiatry. (2006) 60:867–73. doi: 10.1016/j.biopsych.2006.04.029
49. Clays, E, De Bacquer, D, Leynen, F, Kornitzer, M, Kittel, F, and De Backer, G. Job stress and depression symptoms in middle-aged workers--prospective results from the Belstress study. Scand J Work Environ Health. (2007) 33:252–9. doi: 10.5271/sjweh.1140
50. Lee, SA, Ju, YJ, Han, KT, Choi, JW, Yoon, HJ, and Park, EC. The association between loss of work ability and depression: a focus on employment status. Int Arch Occup Environ Health. (2017) 90:109–16. doi: 10.1007/s00420-016-1178-7
51. Bracke, P, Pattyn, E, and von dem Knesebeck, O. Overeducation and depressive symptoms: diminishing mental health returns to education. Sociol Health Illn. (2013) 35:1242–59. doi: 10.1111/1467-9566.12039
52. You, Y, Chen, Y, Fang, W, Li, X, Wang, R, Liu, J, et al. The association between sedentary behavior, exercise, and sleep disturbance: a mediation analysis of inflammatory biomarkers. Front Immunol. (2023) 13:1080782. doi: 10.3389/fimmu.2022.1080782
53. You, Y, Chen, Y, Zhang, Y, Zhang, Q, Yu, Y, and Cao, Q. Mitigation role of physical exercise participation in the relationship between blood cadmium and sleep disturbance: a cross-sectional study. BMC Public Health. (2023) 23:1465. doi: 10.1186/s12889-023-16358-4
54. You, Y . Accelerometer-measured physical activity and sedentary behaviour are associated with C-reactive protein in US adults who get insufficient sleep: a threshold and isotemporal substitution effect analysis. J Sports Sci. (2024) 42:527–36. doi: 10.1080/02640414.2024.2348906
55. You, Y, Ablitip, A, Chen, Y, Ding, H, Chen, K, Cui, Y, et al. Saturation effects of the relationship between physical exercise and systemic immune inflammation index in the short-sleep population: a cross-sectional study. BMC Public Health. (2024) 24:1920. doi: 10.1186/s12889-024-19432-7
56. You, Y, Chen, Y, Wei, M, Tang, M, Lu, Y, Zhang, Q, et al. Mediation role of recreational physical activity in the relationship between the dietary intake of live microbes and the systemic immune-inflammation index: a real-world cross-sectional study. Nutrients. (2024) 16:777. doi: 10.3390/nu16060777
57. McGrady, A, Lynch, DJ, Nagel, RW, and Tamburrino, M. Coherence between physician diagnosis and patient self reports of anxiety and depression in primary care. J Nerv Ment Dis. (2010) 198:420–4. doi: 10.1097/NMD.0b013e3181e084ce
Keywords: job fitness, job level, educational level, CES-D, depression, depressive symptoms
Citation: Yun I, Jung S-H, Chon D, Kim J-H and Moon JY (2024) The impact of job fitness on depressive symptoms in Korean middle-aged and older population: a longitudinal study. Front. Public Health. 12:1439058. doi: 10.3389/fpubh.2024.1439058
Received: 27 May 2024; Accepted: 30 October 2024;
Published: 13 November 2024.
Edited by:
Giorgio Di Lorenzo, University of Rome Tor Vergata, ItalyReviewed by:
Morteza Taheri, University of Tehran, IranCopyright © 2024 Yun, Jung, Chon, Kim and Moon. This is an open-access article distributed under the terms of the Creative Commons Attribution License (CC BY). The use, distribution or reproduction in other forums is permitted, provided the original author(s) and the copyright owner(s) are credited and that the original publication in this journal is cited, in accordance with accepted academic practice. No use, distribution or reproduction is permitted which does not comply with these terms.
*Correspondence: Jae-Hyun Kim, amFlaHl1bkBkYW5rb29rLmFjLmty; Jong Youn Moon, bW9vbmp5QGdhY2hvbi5hYy5rcg==
Jae-Hyun Kim, https://orcid.org/0000-0002-3531-489X
Jong Youn Moon, orcid.org/0000-0001-5278-6816
†These authors have contributed equally to this work and share first authorship
‡These authors have contributed equally to this work and share co-corresponding authorship
Disclaimer: All claims expressed in this article are solely those of the authors and do not necessarily represent those of their affiliated organizations, or those of the publisher, the editors and the reviewers. Any product that may be evaluated in this article or claim that may be made by its manufacturer is not guaranteed or endorsed by the publisher.
Research integrity at Frontiers
Learn more about the work of our research integrity team to safeguard the quality of each article we publish.