- 1Department of Law, Economics and Social Sciences, Magna Graecia University, Catanzaro, Italy
- 2Department of Economics, Statistics and Finance, University of Calabria, Cosenza, Italy
- 3Department of Clinical and Experimental Medicine, Magna Graecia University, Catanzaro, Italy
- 4Scuola Superiore Sant’Anna, Institute of Management, Pisa, Italy
Introduction and objective: The number of literature reviews examining the use of social media in detecting emerging infectious diseases has recently experienced an unprecedented growth. Yet, a higher-level integration of the evidence is still lacking. This study aimed to synthesize existing systematic literature reviews published on this topic, offering an overview that can help policymakers and public health authorities to select appropriate policies and guidelines.
Methods: We conducted an umbrella review: a review of systematic reviews published between 2011 and 2023 following the PRISMA statement guidelines. The review protocol was registered in the PROSPERO database (CRD42021254568). As part of the search strategy, three database searches were conducted, specifically in PubMed, Web of Science, and Google Scholar. The quality of the included reviews was determined using A Measurement Tool to Assess Systematic Reviews 2.
Results: Synthesis included 32 systematic reviews and 3,704 primary studies that investigated how the social media listening could improve the healthcare system’s efficiency in terms of a timely response to treat epidemic situations. Most of the included systematic reviews concluded showing positive outcomes when using social media data for infectious disease surveillance.
Conclusion: Systematic reviews showed the important role of social media in predicting and detecting disease outbreaks, potentially reducing morbidity and mortality through swift public health action. The policy interventions strongly benefit from the continued use of online data in public health surveillance systems because they can help in recognizing important patterns for disease surveillance and significantly improve the disease prediction abilities of the traditional surveillance systems.
Systematic Review Registration:
1 Introduction
In recent years, governmental responses to emerging infectious diseases (EIDs)—including severe acute respiratory syndrome (SARS) in 2003 (1, 2), H1N1 influenza in 2009, and the Ebola outbreak in 2014-2016—have proven insufficient, with health security measures often falling short.
As a result, the risks of epidemics were frequently underestimated, and funding for preventive interventions was inadequate. This under-preparation was evident during the early stages of the COVID-19 pandemic, with shortages of critical supplies like masks and respirators.
The COVID-19 pandemic underscored the necessity of developing early warning systems able to detect potential outbreaks through advanced data analysis. These systems are essential to prevent healthcare facilities from being overwhelmed in emergencies. One promising approach to addressing this challenge is the digitalization of health services. The use of social media (SM) platforms and digital tools by institutions during pandemics facilitates the rapid and widespread dissemination of information and the real-time tracking of disease outbreaks.
The emphasis is on the timeliness and immediacy required from the “first alert” health monitoring systems, and on effective “now casting” actions to counteract the rapidly developing of pre-pandemic events (3). Although the COVID-19 pandemic increased interest in health monitoring systems, digital epidemiology, which focuses on monitoring online and SM activities for public health purposes, has been explored since the early 2010s. Its primary goal is to capture health-related patterns and forecast disease outbreaks (3).
For instance, Al-Garadi et al. (3) conducted a systematic review in 2016, analyzing empirical studies from 2004 to 2015 on the use of SM data for infectious disease surveillance. The review highlighted the potential of platforms like Twitter and Facebook to track diseases such as influenza, Ebola, and Zika. The findings showed that SM data could predict pandemic trends earlier than official sources, despite challenges related to data limitations, algorithms, and privacy.
However, several limitations remain. SM platforms can be biased toward specific countries or regions, i.e., Twitter or Facebook do not represent countries such as China and Russia, or India. Instead, other SM will work only in given countries (i.e., Baidoo in China). Moreover, tracking and monitoring contacts, critical in disease prevention, can be hindered by privacy restrictions. Social interaction graphs based on SM data are often incomplete, limiting the ability to track real contact networks with the risk of losing important information for disease tracking. Additionally, misinformation, fake news, and echo chambers discussing diseases and outbreaks can distort health signals in SM discussions and posts. Researchers need to develop methods to mitigate these limitations by statistically correcting population biases and creating frameworks to counteract misinformation. This could involve boosting official information posts and highlighting credible sources with the support of SM service providers.
Despite these challenges, previous studies have demonstrated the potential of SM listening to reduce both morbidity and mortality by enabling swifter public health responses. According to the According to the World Health Organization (WHO), 51% of outbreak information related to EIDs between 2001 and 2011 was initially sourced from SM platforms (4).
Facebook, Twitter, Instagram, and Reddit are among the most widely used SM platforms globally (2–5). Users spend significant amounts of time online, sharing vast amounts of information. These data provide valuable insights into community health, offering a low-cost means to observe large populations (4, 6) and study health conditions at scale (3, 7). SM data can also help geo-localize potential chains of contagion (8). For example, Dong et al. (5) used spatial–temporal analysis of SM data in 2016 to track geographical information related to disease outbreaks such as flu and hay fever, demonstrating that SM data can predict disease spread.
As such, SM serves as a complementary tool to traditional surveillance systems, capable of detecting latent pre-pandemic phenomena and potentially anticipating successive waves of ongoing epidemics.
Recognizing this potential, the European Center for Disease Prevention and Control (ECDC) launched “Epitweetr” in October 2020, an open-source software for processing Twitter data to monitor epidemic events.
Further evidence supporting the importance of digital tools in detecting epidemic signals comes from a study by Kogan et al. (9). This research demonstrated how platforms like Google searches and Twitter can capture early signals of potential public health threats (10).
In this framework, the rapid increase in empirical studies into the use of SM in detecting EID has been paralleled with a comparable increase in systematic literature reviews on this topic.
However, no comprehensive summary has yet been provided to consolidate these findings and offer general conclusions that could guide healthcare systems in understanding the advantages and limitations of using SM data for infectious disease surveillance. To address this gap, we conducted an umbrella review—a synthesis of existing systematic literature reviews (11). This study compiles the findings of previous systematic reviews on this topic, offering policymakers and public health authorities an overview to inform policy decisions and guidelines. The structure of the paper is as follows: the next section describes the methods used in the review, followed by the results in Section 3. Discussions, conclusions, and policy implications are presented in Section 4.
2 Materials and methods
The review protocol was registered in the Prospective Register of Systematic Reviews (PROSPERO ID: CRD42021254568). The Preferred Reporting Items for Overviews of Reviews (PRIOR) (12) and the methodological considerations when using existing systematic reviews (13) guided the method and the reporting of findings (The complete PRISMA Checklist is provided in Supplementary Table 1).
2.1 Research question/objective
Our investigation is driven by the following research question: What we know from the systematic reviews on the role of SM as early warnings tools of an epidemic outbreak?
Although there were no restrictions on specific SM platforms, this study focused on major platforms like Facebook, Sina Weibo, Instagram, Reddit, YouTube, and Twitter. We considered those diseases that for their potential epidemic exhibited the greatest public health risk: COVID-19, Crimean-Congo hemorrhagic fever, Ebola virus disease, Marburg virus disease, Lassa fever, Middle East respiratory syndrome coronavirus (MERS-CoV), SARS, Nipah and henipaviral diseases, Rift Valley fever, Zika (14). The study also examined the H1N1 influenza. While it was not declared a pandemic until 2010, it closely resembles the COVID-19 emergency in terms of epidemiology, clinical behavior, and the need for robust health policy interventions.
2.2 Search strategy and eligibility criteria
We developed a search strategy using similar reviews (15–17). From March 2023 to 1 October 2023, we systematically searched studies using Web of Science and PubMed databases. We chose these databases for their extensive journal coverage in health sciences. Keywords for the query were manually selected from relevant studies for a comprehensive list of cited papers and publishing trends. Specifically, the search strategy combined terms for individual EIDs with terms of health and SM, and terms relative to a range of systematic review types (Table 1).
The search of the database has been extended to the title, keywords, and abstracts (topics range). Only the keywords “review” or “systematic review” were restricted to the title. Only studies published in English were included, due to resource limitations for translating non-English publications. Gray literature was identified using the Google Scholar search engine, with results examined up to the 20th page.
Two investigators independently reviewed potentially eligible articles. The inclusion criteria focused on systematic reviews that investigated: (a) the use of SM for early detection of infectious disease outbreaks, (b) the role of SM as a source of information for improving healthcare system efficiency, and (c) the contribution of SM in tracking and monitoring infectious disease outbreaks and preparing timely interventions. Excluded studies were those that: (a) were not systematic reviews, (b) did not focus on SM’s role in detecting or predicting EIDs, or (c) solely described early warning systems without involving SM. Based on these criteria, two authors screened the complete list of titles generated by the search procedure, creating a preliminary classification list. The articles went through abstract review and the full text was obtained for potentially relevant ones.
Then, two other authors searched the references of the selected articles to identify further relevant studies. Discrepancies in paper inclusion were resolved through discussions until a consensus was reached.
2.3 Data extraction
Data extraction was performed independently by all authors, with the selected papers divided equally among them. Data sheets were used to collect data on the following elements: reference, title, journal, publication year, number of citations, declaration of conflict of interest and funding (items included for quality assessment of systematic reviews), aim/research questions, databases and dates, type of EIDs, type of social network, total studies included, key findings/recommendations on the potential utility of SM in predicting infectious diseases outbreaks, and their potential limitations (Table 2). Missing data were filled in, when possible, by mail correspondence with the study authors. Results were presented narratively. A narrative synthesis is often employed in systematic reviews when a meta-analysis is impractical due to the diversity of studies included, such as variations in methods, populations, interventions, or outcomes In addition, narrative synthesis offers flexibility in recognizing and discussing shared themes, patterns, and trends within the studies, resulting in a more comprehensive grasp of the literature that may not be apparent through quantitative analysis alone (18, 19).
2.4 Quality assessment and risk of bias across studies
The quality of the included systematic reviews was assessed using the “A Measurement Tool to Assess systematic Reviews 2” (AMSTAR2) (20). AMSTAR2 comprises 16 elements that address the reviews’ design (i.e., a priori), data extraction, details of the literature search, inclusion of gray literature, characteristics, methods, the scientific quality of included studies, publication bias, and acknowledgement of conflict of interest(s). Each area in AMSTAR2 is investigated using “yes,” “partial yes,” or “no.” The quality assessment was conducted by two independent reviewers with a high agreement and then checked by a third one. To assign a final score to the selected studies, each systematic review received, respectively, a final rating of 1 for “yes,” 0,5 for “partial yes” and 0 for “no.” We assigned a score equal to 0 whether an item was not reported, not specified or unclear. For “yes” and “partial yes” the AMSTAR2 checklist provides specific elements (sub-items) which a systematic review should include. For items 2 and 4, specific sub-items were highlighted, and the score was calculated by dividing the main item score by the total number of sub-items. The only change introduced in the AMSTAR2 checklist concerns the item 16: a “Partial Yes” section was introduced to account for studies that reported either conflicts of interest, funding sources, or both. Then, AMSTAR2 results were summarized into an overall score out of 16.
The quality assessment revealed that most reviews met the essential AMSTAR2 criteria, including clear research questions, comprehensive search strategies, and well-defined inclusion and exclusion criteria.
However, some concerns were raised about the lack of standardized risk-of-bias assessments in certain reviews, which could affect the reliability of the synthesized evidence. The overall quality of the included reviews ranged from moderate to high, with only a few scoring lower in terms of methodological rigor.
Detailed results of the AMSTAR2 assessment for each review are provided in Supplementary material 2 (pages 13–21).
3 Results
3.1 Flow diagram
The initial search yielded 1,663 articles (Figure 1). After reviewing titles and abstracts, 86 studies were selected, which were further narrowed down to 32 review articles after full-text evaluation. The excluded studies and the reasons for exclusion are listed in Supplementary Table 2.
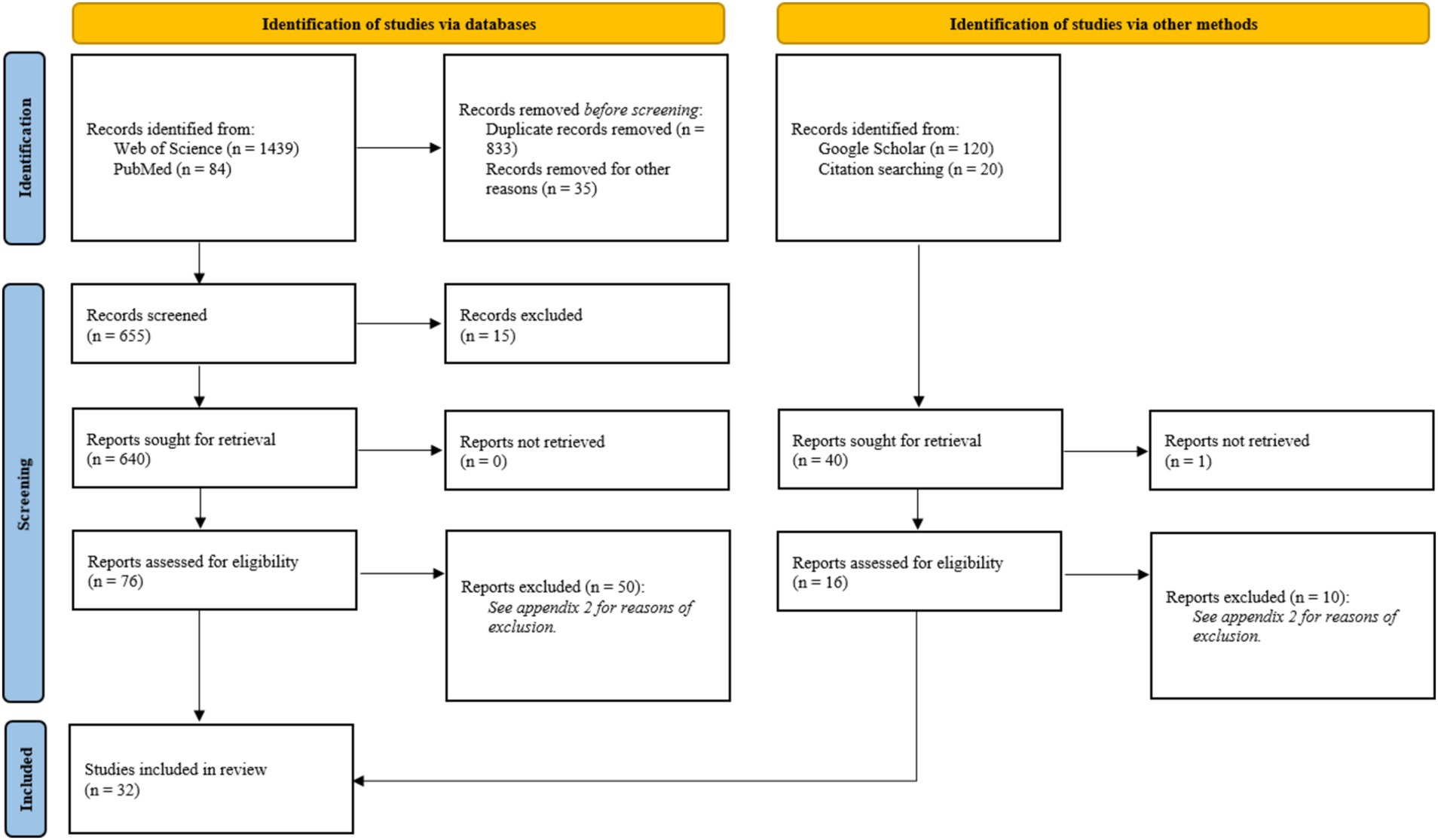
Figure 1. Prisma 2020 flow diagram from Page et al. (52). For more information, visit: http://www.prisma-statement.org/.
3.2 Temporal extension and journals
As digital epidemiology emerged in the 21st century, no selected articles in this study were published before 2011. From 2011 to 2015, 7 systematic reviews were included. In 2016, three papers were found, followed by three reviews each in 2017 and 2018. One paper was published in 2019, five in 2020, and four in 2021. Finally, three reviews were published in both 2022 and 2023. Figure 2 presents a vertical histogram showing the journals with the highest publication frequency.
3.3 Key findings in using social media for disease surveillance
Starting from the systematic reviews, this study aimed to explore how SM can improve the early detection of infectious disease outbreaks.
The review studies’ results (see Table 2) can be summarized as follows:
• SM is effective in supporting public health efforts in identifying EIDs and target populations for interventions. Agrawal and Gupta (21), for example, confirm the potentiality of the SM data for the clinicians, public health practitioners, and lay people that seek health information for the detection of EIDs. Also Gianfredi et al. (22), consider data streams as promising tools for predicting the spread of infectious agents, especially when information and parameters related to the infection rates are scarcely known or not available.
• This emphasizes the advantages of integrating SM analyses into traditional disease surveillance and outbreak management practices (23–29): although social networks will probably never replace traditional surveillance systems, the integration can offer complementary data, improving the disease prediction ability of traditional syndromic surveillance systems (30–33). However, integration with traditional data requires advanced algorithms and computational linguistics methods (3).
• SM can be used for geolocalizing potential chains of contagion. Luan and Law (34) showed that SM can enhance investigations for timely collection of geo-referenced health data, aiding large-scale public health surveillance. Carroll et al. (35) concluded that social networks could be useful in identifying the source case and predicting which individuals are more likely to become infected and further infect others (35). Also Dalili Shoaei and Dastani (36), confirmed that the information drawn from SM platforms can provide useful demographic and geographic details on the characteristics of people who are at potential risk of being infected (36). In this context, Twitter platform is the most used social network (45, 49).
• SM listening can be used as a communication tool available for public policy makers, especially in the initial stages of the infection spread. Eckert et al. (37), for example, demonstrated the successful use of SM by government agencies to share accurate information and debunk misinformation, particularly during the preparation, onset, and containment phases. However, Fung et al. (38) noted that it may occasionally fail, as seen during the Ebola virus disease emergency response.
• There are some limitations in using a social network as a surveillance instrument. These are related to:
a. misinformation phenomena (33, 39–41);
b. lack of an ethical framework (25, 30, 32);
c. lack of internationally recognized policies regarding privacy protection (30–32, 42–45);
d. risk of dissemination of conspiracy theories, pseudo-scientific claims and misleading information, particularly during the initial phase of the pandemic (22, 36);
e. extraction - given the heterogeneous data characteristics - of unstructured data from SM (27, 30, 46, 47);
f. biases in terms of minor accuracy, i.e., false-positive events introduced through geographic or cultural variability in language and reporting when compared with reliable traditional surveillance methods (28).
3.4 The role of the social media in detecting each disease: the circle packing visualization and a network analysis
In this paragraph, we investigate the studies selected in our systematic review, starting from the following research question: Is it possible to explore the role and thus the importance of a specific SM to deal with a disease? To visualize the importance of each disease and SM tools in tracking and monitoring the disease, we use a circlepack plot (Figure 3): a tool that uses nested circles proportional to the number of citations of, respectively, each disease (container circles) and each SM (inner circles) citing the disease.
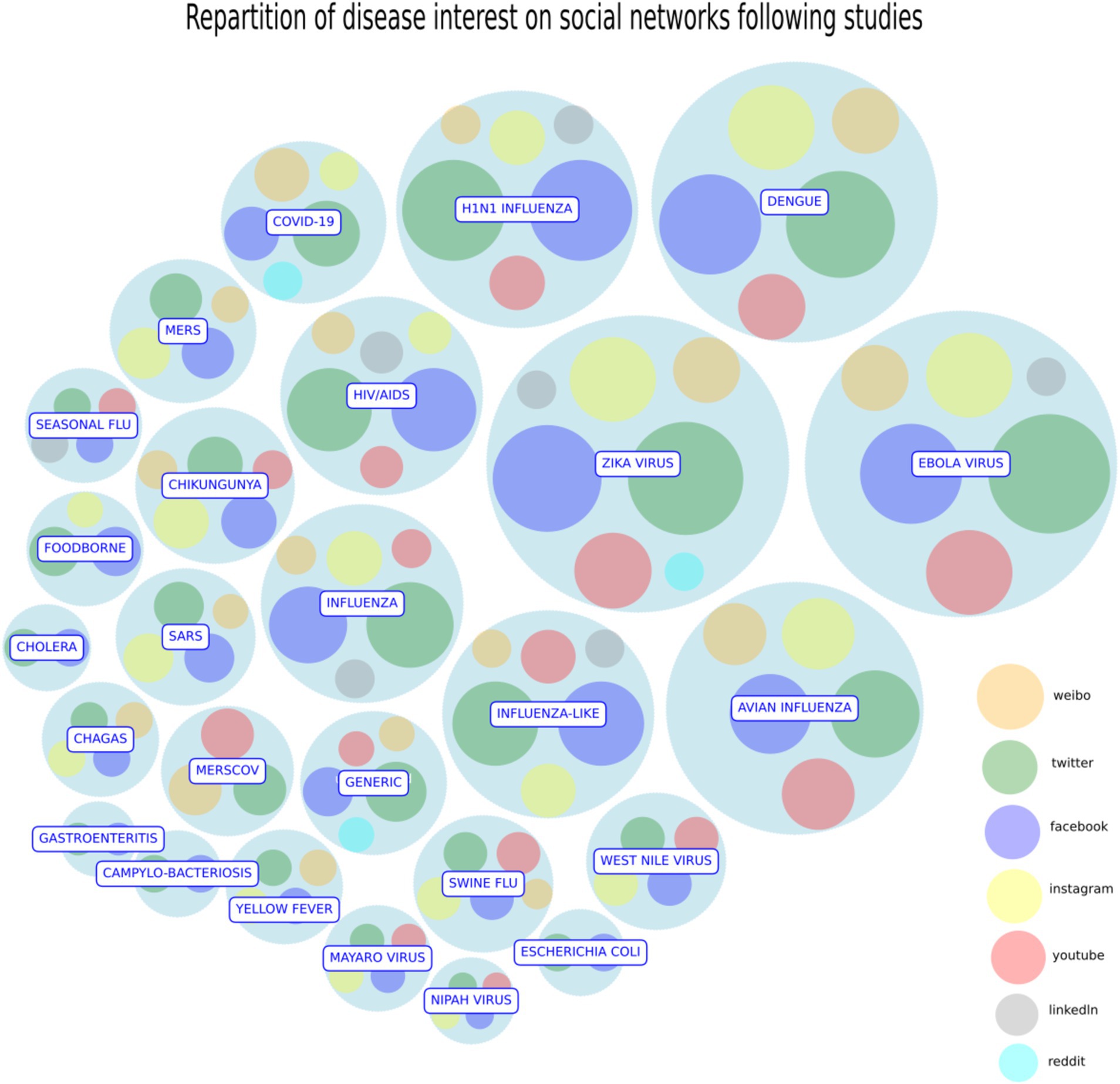
Figure 3. Circlepack plot. The circle pack plot represents the importance of each disease and the SM citing it as nested circles. Containers circles describe each disease proportional to the number of citations in studies, while for each disease the relative importance of each SM is expressed by the radius of their inner circle. Date reference: October 2023. Source: Authors’ elaboration.
In this way, we can both summarize how the literature studies and weights each SM in the analysis of every disease. After conducting the analysis, it was found that the number of studies discussing the COVID-19 pandemic is consistent and equally important as other relevant diseases. However, they have not yet reached the highest position in the ranking list because of a possible “delay effect.” In a future, it is expected that a significant number of papers on COVID-19 will be published. On the other side, Zika, Ebola and the influenza H1N1, Dengue Fever, together with COVID-19 show the largest bubble sizes since they have raised a lot of concern in the public and they were vastly studied by the experts of the field as the first examples of disease communication through the social networks. Twitter, Facebook, Youtube and Instagram are the most relevant social platforms in the circlepack plot. Other social platforms of less importance, like MySpace, show a smaller size bubble: although they pioneered the initial phase of the internet diffusion, they are now extinct. Finally, Weibo and VK have a minor circle size because they target specific markets (Chinese for Weibo and Russian for VK). To complete the analysis, we propose a network representation of the diseases according to the intensity of the social networks’ communication (Figure 4). The basic idea is to represent diseases according to the way SM treat them. Two diseases form a link if they are similar in the treatment they received by SM. This measure represents both citation impact (size of the node) and visual representation of SM response.
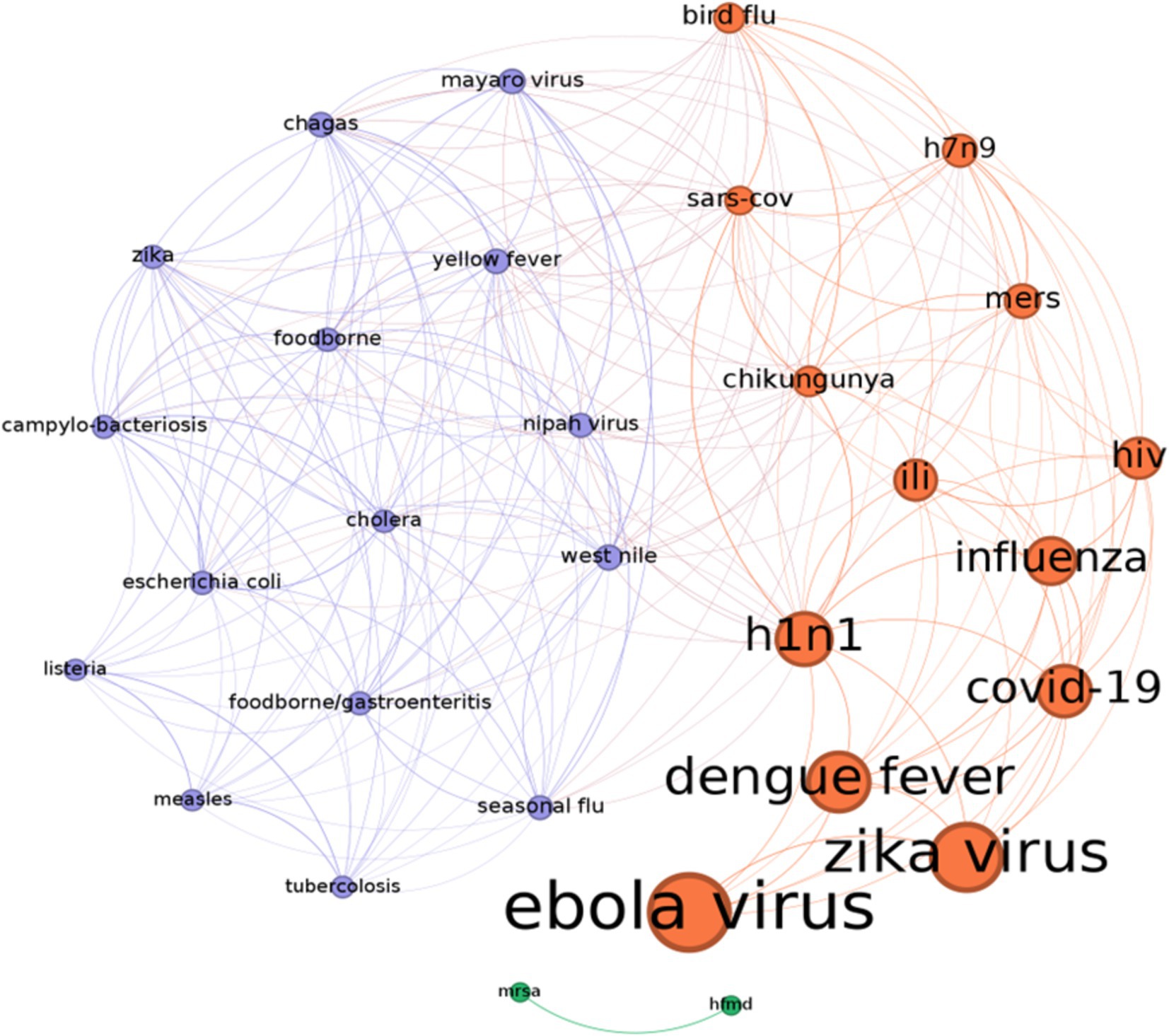
Figure 4. The diseases’ similarity network according to their citations on social networks. In detail, the more two diseases share a similar impact on different social networks the stronger is their link (shown by thickness of the link). The size of nodes is proportional to the number of citations. The color of the nodes defines three communities dividing the diseases in two main groups that received more homogeneous coverage by social networks, and a disconnected sub-network connecting mrsa, and hfmd (hfmd: hand foot and mouth diseases, mrsa: methicillin-resistant staphylococcus aureus). Source: Authors’ elaboration.
The steps of the network formation are the following:
• Each disease is linked to a vector of social networks with vector elements that correspond to a number of studies’ citations per disease;
• The links reflect how many social networks, and corresponding citations, two diseases have in common. If two diseases share a similar presence on social networks, the link becomes stronger. The used similarity metric is the “weighted Jaccard index”.1
• Links are removed below a given threshold (0.3) to maintain only the stronger levels of similarity;
• Nodes were colored using a community detection algorithm (48) that recovers the homogeneous disease by dividing nodes into dense groups.
The number of studies on each disease determines its relevance in the network structure. Ebola received the most attention, followed by Zika, Dengue, and COVID-19.
A simple clustering procedure, known as community detection (48) is then used to divide the nodes in groups/clusters that are homogenous according to how the social networks discuss a particular disease (Figure 3).
According to this representation, the cluster of “flu like” diseases and viruses is identified in orange color, opposed to a cluster of different diseases with cholera, tuberculosis and other gastroenteritis viruses representing a different sample set that is shown in violet. In summary, we recover the importance of COVID-19 through the higher number of citations making it among the most discussed diseases (it is not surprising) in the social networks’ studies. Ebola virus, identified in 1976, remains a deadly disease with high mortality rates capturing a growing attention of the researchers and the discussions on virtually all social networks.
4 Discussion
This umbrella review included 32 systematic reviews and 3,704 primary studies on the use of SM to detect EIDs. While policymakers should be cautious about the limitations of using digital data for health surveillance, most studies highlight positive outcomes. SM plays a key role in policy actions and healthcare management, helping reduce morbidity and mortality. It provides an opportunity to detect early epidemic signals, complementing traditional surveillance systems, which, though specific, often face delays, high costs, and geographical limitations.
Our review has both strengths and limitations.
A major strength is the comprehensive overview of SM’s role in detecting emerging diseases, covering a wide range of diseases and social networks. The systematic approach highlights new insights into SM’s role in health surveillance. For instance, only a few studies, such as Velasco et al. (32), focus on event-based approaches, whereas most rely on continuous SM news streams (filtered by keywords, like disease symptoms such as “cough,” “fever” etc.), which can be misleading and imprecise. Targeting specific events (e.g., outbreak news) could improve robustness and reduce misinformation. Additionally, De Araujo et al. (27) suggest using SM as active tools, connecting experts and practitioners to create geographical networks for verifying health emergencies.
Some limitations should be noted. First, the scope of the review was limited to systematic reviews published up to October 2023, which may have excluded relevant studies published thereafter. This temporal limitation might affect the comprehensiveness of the findings, particularly given the rapidly evolving nature of studies on this topic. Second, non-English papers were excluded, potentially missing relevant studies and excluding evidence from countries with different policy approaches. Third, we did not assess the quality of the primary studies, which was the role of the original systematic reviews. Also, although SM shows potential as a complement to traditional systems, challenges such as misinformation, noise, and privacy concerns remain. The unstructured nature of SM data complicates its integration into existing surveillance systems, requiring further research to address these issues. Finally, we attempted to minimize sample bias through a systematic search for gray literature using Google Scholar; however, we cannot fully exclude it due to our limited search.
It is also important to acknowledge the limitations inherent in many of the systematic reviews included. Many studies used observational or retrospective data, leading to potential biases in data collection and interpretation. Most primary studies relied on self-reported or public SM data, which may be unrepresentative and subject to selection bias. Moreover, methodological heterogeneity made it difficult to compare findings across reviews, with different computational tools, algorithms, and linguistic methods producing inconsistent outcomes. This variability also applied to the types of SM platforms studied, with more popular platforms like Twitter and Facebook receiving more attention, while others potentially relevant to specific populations were overlooked.
5 Conclusion and implications
We can conclude that the policy interventions strongly benefit from the continued use of online data in public health surveillance systems, in order to improve the disease prediction abilities of the traditional surveillance systems.
The findings emphasize several key policy implications. First, SM analytics are transforming how public health experts monitor diseases by integrating real-time geolocation of health status, allowing early identification of potential pandemics.
Second, for health systems management, the digital innovation based on SM is a tool to promote new models of prevention, diagnosis, treatment and assistance that are sustainable and interconnected with the transformation processes underway (reduction of resources, demographic explosion, aging of the population, affirmation of new health emergencies connected to the phenomenon of globalization).
These transformations are the basis of that new frontier of eHealth which refers to the combined use of electronic communication and technological information analytics in the health sector (49). Finally, the detection of early outbreaks through SM tracking provides a significant timeliness advantage in a variety of EIDs, potentially improving healthcare systems efficiency in terms of a better management of healthcare facilities.
Policy makers and governments may effectively leverage SM information if they implement research projects and specialized platforms. These ones should perform social listening of disease symptoms for early outbreak detection, and starting from social interaction data, help creating specialized networks of professionals (doctors, scientists and others) that will cope with misinformation and will provide firsthand evidence of emerging outbreaks.
Overall, misinformation for the vast public can be counteracted by working together with SM platforms to promote curated content, reducing user exposure to fake news (50, 51). In this context, policymakers can support initiatives targeted to measure the effects of correct information, like for example the user polarization caused by specific fake news on a given topic, does it really decrease after an information campaign?
This study aims to provide helpful suggestions to health policy decision-makers in deciding whether to incorporate new methods into comprehensive programs of surveillance that already contain well established indicator-based surveillance tools. Considering the limitations associated with the use of SM data, we suggest the creation of specially designated working groups able to analyze and correctly make use of the online data, to reduce the potential of SM into spreading rumors, fake news, and misleading information. From this point of view, cost–benefit investigations are needed to examine the advantages of SM data in the long term, guiding future best practices in the field. The creation of an integrated, accessible, and constantly updated data set is the first step toward the creation of both an effective and efficient pandemic surveillance system. It will not only be fed by data from digital platforms, but multiple sources can also be relevant to build a multisectoral database. As possible inputs, it may also include environmental data (i.e., composition of wastewater that may contain traces of the disease pathogens), which, whether properly analyzed, can provide useful information to prevent the emergence of new pathogens.
Data availability statement
The original contributions presented in the study are included in the article/Supplementary material, further inquiries can be directed to the corresponding author.
Author contributions
MG: Data curation, Methodology, Writing – original draft, Writing – review & editing. ML: Writing – original draft, Writing – review & editing. MM: Writing – original draft, Writing – review & editing. MP: Writing – original draft, Writing – review & editing.
Funding
The author(s) declare that financial support was received for the research, authorship, and/or publication of this article. The authors of this paper thank the “Health & Innovation” Research Center of Magna Graecia University of Catanzaro for the financial support received for the publication of this article.
Conflict of interest
The authors declare that the research was conducted in the absence of any commercial or financial relationships that could be construed as a potential conflict of interest.
Publisher’s note
All claims expressed in this article are solely those of the authors and do not necessarily represent those of their affiliated organizations, or those of the publisher, the editors and the reviewers. Any product that may be evaluated in this article, or claim that may be made by its manufacturer, is not guaranteed or endorsed by the publisher.
Supplementary material
The Supplementary material for this article can be found online at: https://www.frontiersin.org/articles/10.3389/fpubh.2024.1435724/full#supplementary-material
Footnotes
References
1. McMullan, LK . Clinical trials in an Ebola outbreak seek to find an evidence-based cure. EBioMedicine. (2020) 52:102614. doi: 10.1016/j.ebiom.2019.102614
2. Alamoodi, AH, Zaidan, BB, Zaidan, AA, Albahri, OS, Mohammed, KI, Malik, R, et al. Sentiment analysis and its applications in fighting COVID-19 and infectious diseases: a systematic review. Expert Syst Appl. (2021) 167:114155. doi: 10.1016/j.eswa.2020.114155
3. Al-Garadi, MA, Khan, MS, Varathan, KD, Mujtaba, G, and Al-Kabsi, AM. Using online social networks to track a pandemic: a systematic review. J Biomed Inform. (2016) 62:1–11. doi: 10.1016/j.jbi.2016.05.005
4. World Health Organisation-SEARO (2011) ‘Developing tools for strategic communication to the media on emerging infectious diseases (EIDs)’, Available at: http://apps.who.int/iris/bitstream/handle/10665/204966/B4803.pdf?sequence=1&isAllowed=y (Accessed: 15 September 2023).
5. Ali, K., Dong, H., Bouguettaya, A., Erradi, A., and Hadjidj, R. (2017) ‘Sentiment analysis as a service: a social media based sentiment analysis framework’, in 2017 IEEE international conference on web services (ICWS), IEEE, pp. 660–667.
6. Choi, S, Lee, J, Kang, MG, Min, H, Chang, YS, and Yoon, S. Large-scale machine learning of media outlets for understanding public reactions to nation-wide viral infection outbreaks. Methods. (2017) 129:50–9. doi: 10.1016/j.ymeth.2017.07.027
7. Centola, D . The spread of behavior in an online social network experiment. Science. (2010) 329:1194–7. doi: 10.1126/science.1185231
8. Lopreite, M, Panzarasa, P, Puliga, M, and Riccaboni, M. Early warnings of COVID-19 outbreaks across Europe from social media. Sci Rep. (2021) 11:2147. doi: 10.1038/s41598-021-81333-1
9. Kogan, NE, Clemente, L, Liautaud, P, Kaashoek, J, Link, NB, Nguyen, AT, et al. An early warning approach to monitor COVID-19 activity with multiple digital traces in near real time. Sci Adv. (2021) 7:eabd6989. doi: 10.1126/sciadv.abd6989
10. Espinosa, L, Wijermans, A, Orchard, F, Höhle, M, Czernichow, T, Coletti, P, et al. Epitweetr: early warning of public health threats using twitter data. Euro Surveill. (2022) 27:2200177. doi: 10.2807/1560-7917.ES.2022.27.39.2200177
11. Parè, G, Trudel, MC, Jaana, M, and Kitsiou, S. Synthesizing information systems knowledge: a typology of literature reviews. Inf Manag. (2015) 52:183–99. doi: 10.1016/j.im.2014.08.008
12. Pollock, M, Fernandes, RM, Pieper, D, Tricco, AC, Gates, M, Gates, A, et al. Preferred reporting items for overviews of reviews (PRIOR): a protocol for development of a reporting guideline for overviews of reviews of healthcare interventions. Syst Rev. (2019) 8:335–9. doi: 10.1186/s13643-019-1252-9
13. Whitlock, EP, Lin, JS, Chou, R, Shekelle, P, and Robinson, KA. Using existing systematic reviews in complex systematic reviews. Ann Intern Med. (2008) 148:776–82. doi: 10.7326/0003-4819-148-10-200805200-00010
14. World Health Organization . Prioritizing diseases for research and development in emergency contexts. Geneva: Switzerland (2021).
15. Nittas, V, Gao, M, West, EA, Ballouz, T, Menges, D, Wulf Hanson, S, et al. Long COVID through a public health lens: an umbrella review. Public Health Rev. (2022) 43:1604501. doi: 10.3389/phrs.2022.1604501
16. de Bont, J, Jaganathan, S, Dahlquist, M, Persson, Å, Stafoggia, M, and Ljungman, P. Ambient air pollution and cardiovascular diseases: an umbrella review of systematic reviews and meta-analyses. J Intern Med. (2022) 291:779–800. doi: 10.1111/joim.13467
17. Belbasis, L, Bellou, V, Evangelou, E, Ioannidis, JP, and Tzoulaki, I. Environmental risk factors and multiple sclerosis: an umbrella review of systematic reviews and meta-analyses. Lancet Neurol. (2015) 14:263–73. doi: 10.1016/s1474-4422(14)70267-4
18. Eijkenaar, F, Emmert, M, Scheppach, M, and Schöffski, O. Effects of pay for performance in health care: a systematic review of systematic reviews. Health Policy. (2013) 110:115–30. doi: 10.1016/j.healthpol.2013.01.008
19. Hatmi, ZN . A systematic review of systematic reviews on the COVID-19 pandemic. SN Comprehensive Clin Med. (2021) 3:419–36. doi: 10.1007/s42399-021-00749-y
20. Shea, BJ, Reeves, BC, Wells, G, Thuku, M, Hamel, C, Moran, J, et al. AMSTAR 2: a critical appraisal tool for systematic reviews that include randomised or non-randomised studies of healthcare interventions, or both. BMJ. (2017) 358:j4008. doi: 10.1136/bmj.j4008
21. Agrawal, A, and Gupta, A. The utility of social media during an emerging infectious diseases crisis: a systematic review of literature. J Microbiol Infectious Dis. (2020) 10:188–98. doi: 10.5799/jmid.839415
22. Gianfredi, V, Bragazzi, NL, Nucci, D, Martini, M, Rosselli, R, Minelli, L, et al. Harnessing big data for communicable tropical and sub-tropical disorders: implications from a systematic review of the literature. Front Public Health. (2018) 6:90. doi: 10.3389/fpubh.2018.00090
23. Moorhead, SA, Hazlett, DE, Harrison, L, Carroll, JK, Irwin, A, and Hoving, C. A new dimension of health care: systematic review of the uses, benefits, and limitations of social media for health communication. J Med Internet Res. (2013) 15:e1933. doi: 10.2196/jmir.1933
24. Bernardo, TM, Rajic, A, Young, I, Robiadek, K, Pham, MT, and Funk, JA. Scoping review on search queries and social media for disease surveillance: a chronology of innovation. J Med Internet Res. (2013) 15:e2740. doi: 10.2196/jmir.2740
25. Charles-Smith, LE, Reynolds, TL, Cameron, MA, Conway, M, Lau, EH, Olsen, JM, et al. Using social media for actionable disease surveillance and outbreak management: a systematic literature review. PLoS One. (2015) 10:e0139701. doi: 10.1371/journal.pone.0139701
26. Choi, J, Cho, Y, Shim, E, and Woo, H. Web-based infectious disease surveillance systems and public health perspectives: a systematic review. BMC Public Health. (2016) 16:1238–10. doi: 10.1186/s12889-016-3893-0
27. De Araujo, D.H.M., de Carvalho, E.A., da Motta, C.L.R., da Silva Borges, M.R., Gomes, J.O., and de Carvalho, P.V.R. (2019). ‘Social networks applied to Zika and H1N1 epidemics: a systematic review’, in Proceedings of the 20th congress of the international ergonomics association (IEA 2018) Volume II: Safety and Health, Slips, Trips and Falls 20, Springer International Publishing, pp. 679–692.
28. Barros, JM, Duggan, J, and Rebholz-Schuhmann, D. The application of internet-based sources for public health surveillance (infoveillance): systematic review. J Med Internet Res. (2020) 22:e13680. doi: 10.2196/13680
29. Gunasekeran, DV, Chew, A, Chandrasekar, EK, Rajendram, P, Kandarpa, V, Rajendram, M, et al. The impact and applications of social media platforms for public health responses before and during the COVID-19 pandemic: systematic literature review. J Med Internet Res. (2022) 24:e33680. doi: 10.2196/33680
30. Gupta, A, and Katarya, R. Social media based surveillance systems for healthcare using machine learning: a systematic review. J Biomed Inform. (2020) 108:103500. doi: 10.1016/j.jbi.2020.103500
31. O’Shea, J . Digital disease detection: a systematic review of event-based internet biosurveillance systems. Int J Med Inform. (2017) 101:15–22. doi: 10.1016/j.ijmedinf.2017.01.019
32. Velasco, E, Agheneza, T, Denecke, K, Kirchner, G, and Eckmanns, T. Social media and internet-based data in global systems for public health surveillance: a systematic review. Milbank Q. (2014) 92:7–33. doi: 10.1111/1468-0009.12038
33. Khan, JI, Khan, J, Ali, F, Ullah, F, Bacha, J, and Lee, S. Artificial intelligence and internet of things (AI-IoT) technologies in response to COVID-19 pandemic: a systematic review. IEEE Access. (2022) 10:62613–60. doi: 10.1109/ACCESS.2022.3181605
34. Luan, H, and Law, J. Web GIS-based public health surveillance systems: a systematic review. ISPRS Int J Geo Inf. (2014) 3:481–506. doi: 10.3390/ijgi3020481
35. Carroll, LN, Au, AP, Detwiler, LT, Fu, TC, Painter, IS, and Abernethy, NF. Visualization and analytics tools for infectious disease epidemiology: a systematic review. J Biomed Inform. (2014) 51:287–98. doi: 10.1016/j.jbi.2014.04.006
36. Dalili Shoaei, M, and Dastani, M. The role of twitter during the covid-19 crisis: a systematic literature review. Acta Informatica Pragensia. (2020) 9:154–69. doi: 10.18267/j.aip.138
37. Eckert, S, Sopory, P, Day, A, Wilkins, L, Padgett, D, Novak, J, et al. Health-related disaster communication and social media: mixed-method systematic review. Health Commun. (2018) 33:1389–400. doi: 10.1080/10410236.2017.1351278
38. Fung, ICH, Duke, CH, Finch, KC, Snook, KR, Tseng, PL, Hernandez, AC, et al. Ebola virus disease and social media: a systematic review. Am J Infect Control. (2016) 44:1660–71. doi: 10.1016/j.ajic.2016.05.011
39. Phillips, L, Dowling, C, Shaffer, K, Hodas, N, and Volkova, S. Using social media to predict the future: a systematic literature review. Comput Soc Netw. (2017) 1–55. doi: 10.48550/arXiv.1706.06134
40. Hagg, E, Dahinten, VS, and Currie, LM. The emerging use of social media for health-related purposes in low and middle-income countries: a scoping review. Int J Med Inform. (2018) 115:92–105. doi: 10.1016/j.ijmedinf.2018.04.010
41. Alvarez-Galvez, J, Suarez-Lledo, V, and Rojas-Garcia, A. Determinants of infodemics during disease outbreaks: a systematic review. Front Public Health. (2021) 9:603603. doi: 10.3389/fpubh.2021.603603
42. Chen, J, and Wang, Y. Social media use for health purposes: systematic review. J Med Internet Res. (2021) 23:e17917. doi: 10.2196/17917
43. Golinelli, D, Boetto, E, Carullo, G, Nuzzolese, AG, Landini, MP, and Fantini, MP. Adoption of digital technologies in health care during the COVID-19 pandemic: systematic review of early scientific literature. J Med Internet Res. (2020) 22:e22280. doi: 10.2196/22280
44. Sinnenberg, L, Buttenheim, AM, Padrez, K, Mancheno, C, Ungar, L, and Merchant, RM. Twitter as a tool for health research: a systematic review. Am J Public Health. (2017) 107:e1–8. doi: 10.2105/ajph.2016.303512
45. Takats, C, Kwan, A, Wormer, R, Goldman, D, Jones, HE, Romero, D, et al. Ethical and methodological considerations of twitter data for public health research: systematic review. J Med Internet Res. (2022) 24:e40380. doi: 10.2196/40380
46. Corsi, A, de Souza, FF, Pagani, RN, and Kovaleski, JL. Big data analytics as a tool for fighting pandemics: a systematic review of literature. J Ambient Intell Humaniz Comput. (2021) 12:9163–80. doi: 10.1007/s12652-020-02617-4
47. Guy, S., Ratzki-Leewing, A., Bahati, R., and Gwadry-Sridhar, F. (2012) ‘Social media: a systematic review to understand the evidence and application in infodemiology’, in Electronic healthcare: 4th international conference, eHealth 2011, Málaga, Spain, November 21–23, 2011, revised selected papers 4, Springer Berlin Heidelberg, pp. 1–8.
48. Newman, ME . Modularity and community structure in networks. Proc Natl Acad Sci USA. (2006) 103:8577–82. doi: 10.1073/pnas.0601602103
49. World Health Organization (2005). Fifty-eighth world health assembly. Geneva, Switzerland. Available at: https://apps.who.int/gb/ebwha/pdf_files/WHA58-REC1/english/A58_2005_REC1-en.pdf (Accessed August 3, 2023)
50. Iacus, SM, Porro, G, Salini, S, and Siletti, E. Controlling for selection Bias in social media indicators through official statistics: a proposal. J Off Stat. (2020) 36:315–38. doi: 10.2478/jos-2020-0017
51. Rodrigues, F, Newell, R, Babu, GR, Chatterjee, T, Sandhu, N, and Gupta, L. The social media Infodemic of health-related misinformation and technical solutions. Health Policy and Technol. (2024) 100846:100846. doi: 10.1016/j.hlpt.2024.100846
52. Page, MJ, McKenzie, JE, Bossuyt, PM, Boutron, I, Hoffmann, TC, Mulrow, CD, et al. The PRISMA 2020 statement: an updated guideline for reporting systematic reviews. Int J Surg. (2021) 88:105906. doi: 10.1016/j.ijsu.2021.105906
53. Tsao, SF, Chen, H, Tisseverasinghe, T, Yang, Y, Li, L, and Butt, ZA. What social media told us in the time of COVID-19: a scoping review. Lancet Digital Health. (2021) 3:e175–94. doi: 10.1016/s2589-7500(20)30315-0
54. Campo, JD, Saiz, BC, and Domínguez, MÁC. Use of twitter for health communication: a systematic review. Revista Española de Comunicación en Salud. (2023) 14:95–105. doi: 10.20318/recs.2023.7049
55. Pujante-Otalora, L, Canovas-Segura, B, Campos, M, and Juarez, JM. The use of networks in spatial and temporal computational models for outbreak spread in epidemiology: a systematic review. J Biomed Inform. (2023) 143:104422. doi: 10.1016/j.jbi.2023.104422
Keywords: health policy, emerging infectious diseases, umbrella review, social media, surveillance
Citation: Giancotti M, Lopreite M, Mauro M and Puliga M (2024) Innovating health prevention models in detecting infectious disease outbreaks through social media data: an umbrella review of the evidence. Front. Public Health. 12:1435724. doi: 10.3389/fpubh.2024.1435724
Edited by:
Daniel Rhys Thomas, Public Health Wales NHS Trust, United KingdomReviewed by:
Eustachio Cuscianna, University of Bari Aldo Moro, ItalyMohamed Elhakim, WHO Regional Office for the Eastern Mediterranean, Egypt
Copyright © 2024 Giancotti, Lopreite, Mauro and Puliga. This is an open-access article distributed under the terms of the Creative Commons Attribution License (CC BY). The use, distribution or reproduction in other forums is permitted, provided the original author(s) and the copyright owner(s) are credited and that the original publication in this journal is cited, in accordance with accepted academic practice. No use, distribution or reproduction is permitted which does not comply with these terms.
*Correspondence: Monica Giancotti, bWdpYW5jb3R0aUB1bmljei5pdA==