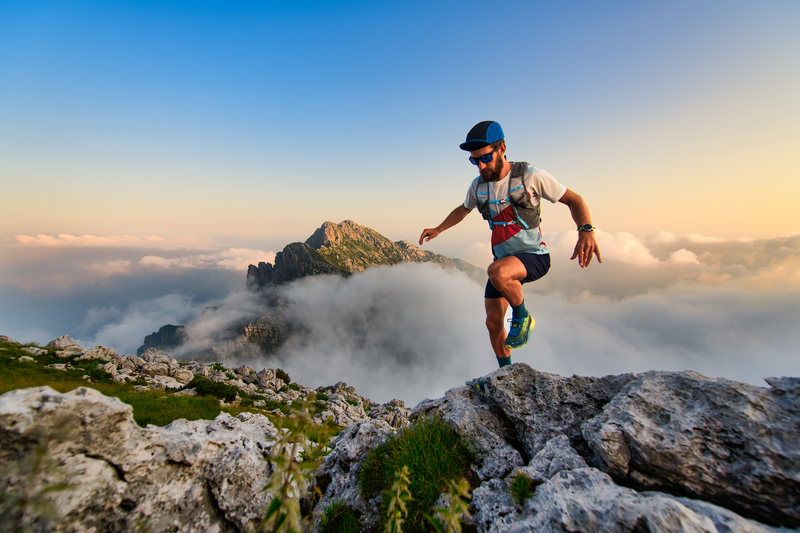
94% of researchers rate our articles as excellent or good
Learn more about the work of our research integrity team to safeguard the quality of each article we publish.
Find out more
ORIGINAL RESEARCH article
Front. Public Health , 19 November 2024
Sec. Aging and Public Health
Volume 12 - 2024 | https://doi.org/10.3389/fpubh.2024.1435329
This article is part of the Research Topic AI-Driven Healthcare Delivery, Ageism, and Implications for Older Adults: Emerging Trends and Challenges in Public Health View all 6 articles
Background: Chatbots are increasingly integrated into the lives of older adults to assist with health and wellness tasks. This study aimed to understand the factors that enhance older adults’ acceptance of chatbots in healthcare delivery.
Methods: This study proposed an extended Unified Theory of Acceptance and Use of Technology model (UTAUT), including aging factors of perceived physical condition, self-actualization needs, and technology anxiety. The model was tested by PLS (Partial Least Squares) with data collected from 428 Chinese citizens aged 60 and above.
Results: The results reveal that performance expectancy, effort expectancy, and social influence significantly affected older adults’ behavioral intention to use chatbots. The facilitating conditions, self-actualization needs, and perceived physical condition significantly affected the actual use behavior of chatbots by older adults, whereas technology anxiety did not. Furthermore, the influence of effort expectancy and social influence on behavioral intention were moderated by experience.
Conclusion: The behavioral intentions of older adults with low experience are more strongly influenced by social influences and effort expectancy. Furthermore, healthcare providers, designers, and policymakers should emphasize the impact of facilitating conditions, self-actualization needs, and perceived physical conditions on chatbot applications among older adults.
Chatbots employ artificial intelligence (AI) and natural language processing (NLP) technologies to simulate human conversations, comprehend questions, and provide automated responses to inquiries (1). They have existed in commonly used smart devices such as smartphones, tablets, and smart speakers (2). Nowadays, they are extending into wearable technologies (3), including head wearables (4) and smartwatches (5), as well as multi-modal technologies (6, 7) and meta-universes (8). There is a growing demand for chatbots in the healthcare sector, with the objective of serving both patients and healthcare professionals. For instance, chatbots have become valuable tools for accessing real-time health information online (9). Chatbots can assist patients in determining whether their symptoms are transient or require further medical attention, offer health-enhancing advice, and encourage disease prevention measures (10). ChatGPT, a new generation of chatbots powered by advances in large-scale language modeling, can be utilized for various consultation, diagnosis, and educational tasks (11). When presented with typical “curbside consult” questions, patient presentations, or summaries of laboratory test results, GPT-4 generally provides functional responses that are useful for healthcare professionals in addressing patients’ concerns (12).
In light of the growing capabilities and accessibility of chatbots, their deployment in the healthcare sector offers a promising avenue for addressing specific needs across diverse demographic groups. One particularly significant group is older adults, who are facing increasing health challenges as they age. According to the United Nations Department of Economic and Social Affairs, the global population aged 65 and over was 761 million in 2021 and is projected to reach 1.6 billion by 2050 (United Nations, 2019). Furthermore, this aging population is contributing to an increase in the prevalence of chronic diseases globally, which is placing a significant economic burden on healthcare systems and society at large (13). Research shows that chatbots contribute to a better quality of life and overall health for older adults, thereby alleviating pressure on the healthcare system through their diverse functionality (14, 15). For example, chatbots can engage in activities such as singing, telling jokes, and conversations with older adults to help prevent dementia (16). By mimicking friendly conversations and asking one question at a time, chatbots facilitate personalized healthcare for older adults (17). In addition, their straightforward interactions encourage physical and mental well-being in older adults. A voice-based healthcare information chatbot, for instance, can assist in overcoming vision and dexterity barriers, enhancing medication awareness and adherence among older adults (18). The user-friendly chatbot interface also provides empathetic companionship for depressed patients over 65, potentially improving their social connectedness and reducing loneliness (19, 20).
Despite the benefits, older adults are often overlooked during the design and development stages of new technologies (21). This oversight can lead to usage challenges, as older adults may struggle with new systems and mistakenly attribute their difficulties to personal shortcomings rather than design flaws (22). Consequently, the older adult demographic often shows a more cautious adoption rate and a slower pace in becoming actively involved (23). The Unified Theory of Acceptance and Use of Technology (UTAUT) framework, which is derived from information technology, has been extensively used to examine the adoption behaviors of older adults. The UTAUT model has identified several factors that significantly influence older adults’ intentions to use medical apps (24), smartphones (25), online shopping (26), and social communication technologies (27). Previous research on the acceptance of chatbots has concentrated on the relationship between trust (28), anthropomorphic design (29), personal innovativeness (30), social self-efficacy (31), and use behavior in various domains, including higher education, finance, and digital marketing. Studies have also investigated the adoption of chatbots in a non-domain-specific context during the COVID-19 pandemic among Romanians (32) and identified key factors influencing the adoption of chatbots in healthcare literacy among students and younger adults (33). However, models used for younger users may not apply to older adults, who are the predominant users of healthcare services (34). This discrepancy arises because these groups value different aspects of technology (35). The specific factors that increase the adoption of chatbots in healthcare delivery among older adults remain unknown.
The promotion of chatbots in healthcare delivery for older adults can facilitate access to medical guidance and the management of their health, thereby reducing the burden and pressure on the social healthcare system caused by an aging population. Consequently, understanding the factors that influence older adults’ acceptance of chatbots in healthcare delivery is crucial. To this end, this study designs a modified UTAUT model that incorporates the aging characteristics of older adults, including perceived physical condition, self-actualization needs, and technology anxiety. The model is then subjected to empirical tests. The research questions are as follows: (1) What factors determine older adults’ behavioral intentions to use chatbots? (2) How do these factors facilitate or hinder older adults’ use of chatbots? (3) How does the moderating effect of experience influence older adults’ adoption? This study is expected to contribute to the existing literature on the subject by extending and empirically testing the UTAUT model with additional variables and providing valuable guidance to healthcare providers, designers, and policymakers.
Venkatesh and Davis combed through Technology Acceptance Model (36), Innovation Diffusion Theory (37), Theory of Reasoned Action (38, 39), Motivational Model (40), Theory of Planning Behavior (41), Combined TAM and TPB (42), Model of PC Utilization (43), and Social Cognitive Theory (44). Next, they integrated the above eight models to propose the Unified Theory of Acceptance and Use of Technology (UTAUT). This new model was able to explain 70% of the variance in usage intention, demonstrating a significant improvement over the original eight models and their extensions (45). It provides a helpful tool for understanding the drivers of acceptance (46). The model contains four main influences: performance expectancy, effort expectancy, social influences, and facilitating conditions. Gender, age, experience, and voluntariness are the moderators (47). Combining these constructs, UTAUT provides a comprehensive framework for understanding technology acceptance and use. The UTAUT model has been empirically tested in terms of the acceptance and use of chatbots in higher education (30), online health communities (48), academia (49), online shopping (50), and financial services (28). The choice of this model is explained by the fact that it has a higher predictive power and is more mature than the alternatives (33). The model has been widely used in research and practice to guide the design and implementation of technology interventions. By understanding these factors, this research can gain insights into what motivates older adults to adopt chatbots in healthcare and identify potential barriers to adoption. The UTAUT model is shown in Figure 1.
The UTAUT suggests that as the younger cohort matures, gender differences in how each perceives information technology may disappear (45). In addition, the behavior of the older adults in this study was voluntary to use chatbots in healthcare delivery. Therefore, the moderating variables of gender, age, and voluntariness were deleted. Experience has been presented as an important moderating variable in the UTAUT model (46). The effect of effort expectancy, social influences, and facilitating conditions tends to be more vital for those with lower experience levels (51). Older users with experience tend to be more independent and more likely to judge based on their perceptions (23). In a qualitative study of ChatGPT, experience was found to moderate the effects of factors in UTAUT on ChatGPT use (52). Therefore, we include the complete UTAUT as the baseline model and examine the moderating effect of experience in this quantitative study.
In addition, older adults’ adoption of technology is not purely technical but rather complex with multiple aspects (53). Aging is a distinguishing factor among older adults; therefore, older adults may have different adoption behaviors than younger users. Some prior research on technology acceptance behavior in older adults has developed older adult-specific trait structures to better understand older adults’ unique characteristics. Due to physical or psychological limitations, such as the gradual loss of sensorial capabilities of vision and hearing (54), older adults resist adopting new technologies. In addition, increasing the self-actualization needs of older adults can narrow the digital divide in technology-related opportunities (55). Some research found that technology anxiety is negatively associated with the perception and evaluation of smart health wearable devices and further affects the intention to use (56). The existing literature has examined the significant influences of aging factors on older adults’ adoption of medical software (57), online shopping (58), smart homes (54), and telemedicine (59).
Venkatesh proposed that studies can adjust the original model by considering the user’s cognitive situation and incorporating new variables to expand and optimize it continuously (46). Therefore, we retained the original model of UTAUT and experience as moderating variables and included the aging characteristics of older adults as new variables in the research framework. The perceived physical condition, self-actualization needs, and technology anxiety are incorporated. The framework is shown in Figure 2, and the hypotheses are developed in the next section.
Performance expectancy refers to the degree to which an individual expects technology to improve task performance (45). People use technology if they believe it will help them perform their job effectively (36). This study refers to the degree to which older adults believe that using chatbots in healthcare activities will help them perform their tasks more effectively and efficiently. Prior studies on chatbots suggest that performance expectancy is essential in predicting users’ behavioral intentions in various contexts (60). Camilleri and Menon et al. demonstrated that performance expectancy has a positive impact on the intention to use chatbots in education and the workplace (61). Similarly, Li et al. empirically revealed that the more performance expectancy, the more likely chatbots would be adopted in mental healthcare (62). However, the impact of performance expectancy among older adults’ behavioral intention to adopt chatbots in healthcare remains unknown. Therefore, the following hypothesis is postulated:
H1: Performance expectancy positively influences older adults’ behavioral intention to use chatbots.
Effort expectancy refers to the degree of ease associated with using the technology (45). This study refers to the level of chatbot ease of use for older adults in healthcare delivery. Effort expectancy is a significant positive antecedent of chatbot use behavior (63, 64). Previous literature has documented the positive impact of effort expectancy on behavioral intention (65). For example, Mogaj et al. (2021) found that effort expectancy played a crucial role in adopting and using chatbots in the banking sector (66). Furthermore, in technology acceptance among older adults, effort expectancy has been identified as an essential factor that directly and positively influences older adults’ use of socially assistive robots (67), online shopping (26), digital health technologies (68), and mHealth (62). For older adults, chatbots that are easier to learn and operate are more likely to be adopted. Therefore, the following hypothesis is postulated:
H2: Effort expectancy positively influences older adults’ behavioral intention to use chatbots.
Social influence refers to how an individual perceives that others believe they should use a particular technology (45). The impact of social factors and the opinions of others on an individual’s technology adoption becomes significant when influential individuals or groups in a social network recommend a technology (69). This study refers to the degree to which older adults are influenced by the opinions of surrounding groups, such as friends, family, peers, and healthcare professionals, when choosing or using chatbots for healthcare services. Osta et al. found that social influence strongly impacted users’ intention to adopt AI conversational agents (chatbots) in the healthcare industry. Menon and Shilpa demonstrated that positive social influence can increase the user’s perception of the usefulness and ease of use of chatbots and reduce the barriers to adoption. Information and support others provide in the older adult’s immediate environment can significantly increase intentions to accept new technology (70). Therefore, the following hypothesis is proposed:
H3: Social influence positively influences older adults’ behavioral intention to use chatbots.
Venkatesh suggests that facilitating conditions are objective conditions in which individuals need to use information technology or products (45). This study refers to the degree to which older adults perceive that organizational and technological infrastructure exists to support using chatbots in healthcare. The significance of facilitating conditions has been highlighted in numerous studies regarding financial services (71), wearable devices (56), and telehealth (72). For chatbots, having laptops, smartphones, and the necessary knowledge and experts available for assistance increases the use behavior of chatbots in online health communities. When users receive more facilitating conditions to use chatbots, they will increase their intentions to use them (48). However, specifically for older adults, Yang et al. (2022) found that when older adults receive a smartphone training program, they are equipped with the resources and knowledge to use their smartphones (73). This resulted in no significant impact of facilitating conditions on the older adults’ use behavior of smartphones. Participants in this study were not systematically trained, and willingness to use chatbots in healthcare may increase when older adults perceive that the necessary organizational and technical support is available. Therefore, the following hypothesis is proposed:
H4: Facilitating conditions positively influence older adults’ use behavior of chatbots.
Aging involves biophysical and psychosocial changes that can increase challenges for older adults and potentially decrease their intention to use innovative technology. This study refers to the degree to which older adults feel that their physical condition limits their use of chatbots. Some studies have shown a negative correlation between the perceived physical condition and behavior (74). Chen et al. indicated that perceived physical condition can act as an internal control or inhibitory condition that affects the use of wearable devices by older adults (75). Similarly, Edelstein et al. argued that the underlying diseases induced by personal physiological conditions cause older adults more trouble using wearable devices (76). This trouble negatively impacted the adoption of the older adults’ use behavior of technology. For effective engagement with chatbots in healthcare, users need to have acceptable health conditions, including visual, auditory, and cognitive abilities. Disabilities like hearing or speech impairments may hinder effective interaction with technology among older adults (54). This study focuses on older adults aged 60 and above, who often face deteriorating health conditions that could impact their views on chatbot utility. Therefore, the following hypothesis is proposed:
H5: The perceived physical condition has a negative effect on older adults’ use behavior of chatbots.
The Self-actualization needs are at the highest level in Maslow’s hierarchy theory and represent an individual’s optimal potential and self-actualization (77). This study refers to the degree to which older adults perceive that their self-actualization needs are met through access to chatbots in healthcare. Previous research indicates that high self-actualization needs can facilitate the adoption of new technologies. Balakrishnan et al. further established a positive correlation between self-actualization needs and technology acceptance. In addition, previous studies found that self-actualization positively relates to older adults’ adoption of e-government services (78) and wearable health technology (79). Older adults will be more willing to use it if technology allows them to feel a sense of accomplishment, excitement, and happiness (80). The hypotheses about chatbots deserve further research. Therefore, the following hypothesis is proposed:
H6: Self-actualization needs positively influence older adults’ use behavior of chatbots.
Technology anxiety is an aging factor associated with behavioral intention (81). It is a frustrating, emotional response to feeling worried or uneasy about accepting technology. Existing research has found that older adults experience technology-related anxiety, resistance, fear, and even technophobia when using digital devices or systems (82). In this study, technology anxiety refers to the extent to which older people feel intimidated or uncomfortable when they want to use chatbots. Studies by Hsieh et al. demonstrated that technology anxiety negatively impacts perceived ease of use and perceived benefits. This anxiety stems from a perceived lack of competence and can lead to rejection of technology. In the case of older adults, unfamiliarity and lack of confidence in technology use can reduce their expectations of performance and increase anticipated effort, thus impeding the acceptance and utilization of intelligent wearables (56). Consistent with these arguments, the following hypothesis is proposed:
H7: Technology anxiety has a negative effect on older adults’ use behavior of chatbots.
Many theories related to technology acceptance, such as TAM and UTAUT, proposed the direct impact of the technology use intention on the actual behavior use (59, 83). For instance, Budhathoki et al. (2023) employed an adapted UTAUT framework to investigate university students’ adoption intention of ChatGPT in two higher education contexts– the UK and Nepal, suggesting that intention significantly influences actual usage. Chen et al. (2023) demonstrated that older adults intentions for wearable devices significantly impact their actual usage behavior. Therefore, we proposed a similar effect on older adults’ intention to adopt chatbots.
H8: Behavioral intention directly influences older adults’ actual use behavior of chatbots.
Users with different experience levels perceive effort expectancy, social influences, and facilitating conditions differently (45, 46). Current literature found that experience moderated the effects of these factors (57). Venkatesh et al. (2003) found that experience with technology can diminish the impact of perceived ease of use and perceived usefulness on adopting new technologies. This indicates that experienced users are more likely to make decisions about technology adoption based on their goals and needs rather than on external factors such as social influence or ease of use. Older users with high experience tend to be more independent and weaken the influences of effort expectancy, social influences, and facilitating conditions. A qualitative study showed that experience can moderate the impact of various factors on the use of ChatGPT, and individuals with more technology experience may find ChatGPT more user-friendly and helpful (52). Therefore, we proposed a similar effect in this quantitative study.
H9a: The influence of effort expectancy on behavioral intention will be moderated by experience.
H9b: The influence of social influence on behavioral intention will be moderated by experience.
H9c: The influence of facilitating conditions on use behavioral will be moderated by experience.
All of the scales were adapted and modified from previous studies. The main constructs of the UTAUT model (performance expectancy, effort expectancy, social influence, facilitating conditions, behavioral intention, and use behavior) were adopted from measurement constructs developed in related studies (45, 46). The perceived physical condition, self-actualization needs, and technology anxiety were each adapted from studies related to technology acceptance among older adults (74, 81, 84). Details on the questionnaire used and definitions of the constructs are shown in Table 1. All items were measured using the 5-point Likert scale, ranging from “strongly disagree” to “strongly agree.”
Moreover, this study contains three demographic information items: education, age, and gender. Education and age were scaled on a 5-point scale and coded as ordinal and interval variables. Consistent with previous research, gender was coded as a 0/1 dummy variable where 0 represented women (46). Experience was measured by the item, “How many months have you been using chatbots in healthcare delivery?” The scale options were: less than 2, 2–4, 4–6, 6–8, 8–10, and over 10 months. In this case, more than half a year of use is defined as a high-experience group, and less than half a year is defined as a low-experience group. The questionnaire was translated into Chinese to ensure that respondents were able to empathize with the content of the instrument and provide appropriate, meaningful responses (85). In addition, we conducted a pilot test with 98 respondents who were not included in the main survey. It showed that Cronbach’s alpha of all questionnaire items exceeded 0.7, proving that the questionnaire was reliable and usable (86).
Due to the lack of a reliable list of older adults with experience using chatbots to access healthcare services and their addresses, we used a convenience sampling method (87). Convenience sampling is a non-random sampling method that is cost-effective compared to other sampling methods and has been commonly used in technology acceptance studies (88). Based on information gathered from the convenient sample, an appropriate reference can be made for the population concerned (89). The survey was conducted on Questionnaire Star (Chinese online survey software) between December 2023 and April 2024. Electronic questionnaires were distributed among older adults in hospital outpatient clinics and wards and several healthcare services WeChat chat groups with a high concentration of older adults. Participants can start filling out the response questionnaire by scanning a QR code online or offline, or by clicking on a web link. In addition, snowball sampling was used in the data collection process to ensure that both the quantity and quality of responses were adequate. Participants were encouraged to share the link with eligible friends or family members. All participants were provided with a consent letter and an information sheet explaining the purpose of the study. To avoid the overclaim usage of the respondents, they were given flexible time to complete the questionnaire (90). Participants were also informed that they had the right to withdraw from participation during the study period, that their anonymity would be guaranteed, and that the results would only be reported in a summary format. To avoid potential bias, no gifts or incentives were given to the participants and all participated voluntarily. Furthermore, before the start of the survey, we gave an example of an interaction with a chatbot providing healthcare services (Figure 3). It was able to respond to users’ healthcare questions, give instant condition analysis, and recommend healthcare information.
Before the survey, two screening questions were used to ensure the accuracy of the data. The World Health Organization defines older adults as 60 years and above (91), we thus include this criterion. Specifically, we asked participants if they were 60 and above (yes/no). In addition, to reduce hypothetical answer bias among participants who had never used a chatbot in healthcare, we asked participants if they had ever used a chatbot in healthcare (yes/no). Participants who answered “yes” to both questions were considered eligible for this study. Participants who answered “no” were filtered out and did not continue with the survey.
Five hundred eligible questionnaires were collected. These questionnaires were subjected to three screening procedures (92). First, 11 respondents provided incomplete questionnaires and we removed them from the study. Second, the questionnaire contained a reverse-coded item, and respondents who provided non-reverse responses were considered to have responded carelessly, resulting in their questionnaire being excluded. Third, questionnaires that chose too many of the same option or showed some regularity in filling in the answers were also excluded. After rigorous screening, 72 invalid questionnaires were excluded, refer to Figure 4. Finally, 428 valid questionnaires were selected. According to previous research recommendations, the sample size should be 15–20 observations for each variable for generalisability purposes (93), and the total number of variables for the present study was 10, including moderating variables. Therefore, the sample size was considered adequate (>200).
Figure 5 presents the respondents’ demographic profile, demonstrating that the university education majority is 44.1% and the high school education is 28.9%. Regarding age, 60–69 is the largest group, accounting for 49.3%. Regarding gender, males make up the majority at 55.4%, and female participants comprise 44.6%. Regarding the duration of use, 2–4 months is the largest group, accounting for 31.6%. The sample covered multiple administrative regions in China, with 69.4% in the province-level regions, 20.8% in the prefecture-level regions, and 9.8% in the county-level and below regions.
This study tested the hypothesized model using the partial least squares structural equation modeling (PLS-SEM), which is more common in information systems research. Unlike covariance-based structural equation modeling (CB-SEM), PLS-SEM employs a component-based analysis (94). PLS-SEM can maintain robust results in the face of small sample sizes and can maximize predictive validity (95). PLS-SEM explores multiple indicators that influence the dependent variable, explaining that a single PLS regression is particularly effective for data analysis (96). PLS-SEM is well suited for analyzing complex relationships between structures because it provides path coefficients and explains variance (97).
Data analysis consisted of three steps. First, a confirmatory factor analysis was conducted to determine the quality of the measurement model by assessing the reliability and validity of the construct. Second, blindfolding was used to test the robustness of the model, and bootstrapping was used to test the significance of the main effects. Third, bootstrap multigroup analysis and two-way ANOVA were used to determine the significance of the moderating effect.
The high collinearity among indicators can produce unstable estimates and make it difficult to distinguish the distinct effects of individual manifest variables on the construct (98). Collinearity assessment involves computing each item’s variance inflation factor (VIF) by running a multiple regression of each indicator in the measurement model of the formatively measured construct on all other items of the same construct. As a rule of thumb, VIF values above 5 indicate collinearity among the indicators (99). In this study, all VIF values were less than 3, indicating that collinearity will not adversely affect future data analysis (refer to Table 2).
Table 3 presents the results of indicator reliability, internal consistency reliability, and convergent validity measures. The rules of thumb for model evaluation are as follows: factor loadings should be 0.60 or higher to indicate good indicator reliability (100); composite reliability (CR) and Cronbach’s α should be above 0.70 to indicate adequate internal consistency reliability (101); and the average variance extracted (AVE) for each construct should be 0.50 or higher to demonstrate adequate convergent validity, meaning the construct explains more than 50% of the variance in the items (102). In this study, all factor loadings exceeded the 0.60 threshold. The Cronbach’s alphas ranged from 0.724 to 0.913, and the minimum composite reliability was 0.86, both above the 0.70 threshold. Additionally, all AVE values exceeded the 0.50 threshold. These results indicate that the constructs exhibit good indicator reliability, internal consistency reliability, and convergent validity.
The correlations of the latent variables were measured by the AVE of each variable, as shown in Table 4. According to Fornell and Larcker, if the square root of the AVE is greater than the correlations among the constructs, the discriminant standards could be met (103). In this study, the square root of the AVE (shown diagonally in bold) exceeded the inter-construct correlations, indicating an appropriate level of discriminant validity. Therefore, all reliability and validity requirements were satisfied.
The structural model evaluation for PLS-SEM requires the use of the explainable variance of the endogenous structure (R2) to describe the extent to which the independent variables of the current model explain the variation in the dependent variable; the calculation of Goodness of Fit (GoF) to describe the quality of the complete measurement model about the quality of the entire structural model; the Stone-Geisser’s Q2 to describe the predictive relevance of the model (94). R2 thresholds of 0.75, 0.50, and 0.25 are considered substantial, moderate, and weak, respectively (95). According to Wetzel et al., the cut-off values of GoF for small, medium, and large effect sizes are 0.10, 0.25, and 0.36, respectively. The Q2 values larger than zero indicate that the exogenous constructs have predictive relevance for the endogenous construct under consideration (104). In this study, the predictive power of behavioral intention (R2 = 0.523) and the use behavior (R2 = 0.614) was moderate, indicating that the model can explain a moderate degree of variance. The GoF value was calculated to be 0.510, which exceeded the 0.36 benchmark, indicating that the model has sufficient goodness of fit. The Q2 values of behavioral intention was 0.376 and use behavior was 0.437, indicating that the model has satisfactory predictive relevance for all the endogenous constructs. For a detailed list of R2, communality, and GoF, Q2, refer to Table 5.
Hypotheses were tested by examining the magnitude of the standardized parameter estimates between the constructs and the corresponding t-values indicating the level of significance. When the t-value is greater than 1.96 and the p-value is smaller than 0.05, it is recommended to accept a hypothesis (105). Path analysis results are shown in Table 6. Bootstrapping was employed to evaluate the significance of the paths. In this study, technology anxiety had a non-significant effect on older adults’ behavioral intention to use chatbots (t = 0.552, p > 0.05), thus hypothesis H7 was not supported. The rest of the hypotheses were supported: Performance expectancy positively and significantly affected older adults’ behavioral intention (t = 7.144, p < 0.001), supporting H1. Effort expectancy positively and significantly affected older adults’ behavioral intention (t = 4.729, p < 0.001), supporting H2. Social influence positively and significantly affected the behavioral intention (t = 5.881, p < 0.001), supporting H3. The significance level of the facilitation conditions (t = 7.309, p < 0.001) was strongest in the UTAUT, which positively and significantly affected older adults’ use behavior, supporting H4. Overall, all four of the original hypotheses in UTAUT were supported. Furthermore, in the aging characteristics, perceived physical condition (t = 3.626, p < 0.001) and self-actualization needs (t = 5.276, p < 0.001) were positively associated with the usage behavior, supporting H5 and H6, respectively. Lastly, results also supported H8, which hypothesized that the behavioral intention is positively connected with the use behavior of chatbots (t = 8.051, p < 0.001).
After testing for main effects, we used bootstrap multigroup analysis to determine the significance of differences between the high-experience and low-experience groups. The result is significant at the 5% probability of error level if the p-value is smaller than 0.05 or larger than 0.95 for a certain difference of group-specific path coefficients (106). In this study, the differences in p-values are significant for effort expectancy (p < 0.05) and social influence (p < 0.05), as shown in Table 7. Therefore, H9a and H9b are supported. This suggests that the influence of social influence and effort expectancy on behavioral intention were moderated by experience. In addition, the effects of facilitating conditions on use behavior were significant in both the high-experience and low-experience groups. However, their difference in p-value was not significant. Therefore, hypothesis H9c is not supported. This suggests that the influence of facilitating conditions on use behavior was not moderated by experience and facilitating conditions are important drivers for both the high-experience and low-experience groups.
The two-way ANOVA was used to compare the differences between the two groups further (107). The mean score splits the antecedents (effort expectancy, social influence, and facilitating conditions) into low and high groups. According to Hsu et al., the data were normally distributed with no significant outliers, making the mean split more robust. The results also showed that the influence of effort expectancy [F(1,424) = 11.568, p < 0.001] and social influence [F(1,424) = 34.131, p < 0.001] on behavioral intention were moderated by experience, which is plotted in Figure 6 respectively. The slope of the high-experience group is flatter than that of the low-experience group. Therefore, older adults with high experience are less affected by social influence and effort expectancy. Furthermore, the experience did not moderate the influence of facilitating conditions on use behavior [F(1,424) =1.87, p > 0.05].
This study extends UTAUT by considering external variables to explore factors influencing older adults’ adoption of chatbots in healthcare delivery. The proposed model can explain 61.4% of the variance in use behavior (R2 = 0.614) and 52.3% in behavioral intention (R2 = 0.523). Seven of eight hypotheses were supported, including the four factors of the UTAUT and two aging factors. This study demonstrates that UTAUT theory can serve as a valid theoretical lens for exploring the factors influencing older adults’ intention to use chatbots in healthcare delivery. Furthermore, the study demonstrates the impact of experience differences on older adults’ behavioral intention to use chatbots in healthcare delivery. The theory and practical implications of the study’s findings were discussed.
In healthcare delivery, exploring the acceptance of chatbots has been the focus of a small number of empirical research. Nadarzynski et al. incorporated semi-structured interviews to explore the acceptability of chatbot systems for healthcare. Moldt et al. investigated the acceptance of medical students toward chatbots (108). Nevertheless, these survey responses were mainly drawn from students and internet users who are relatively experienced with digital technologies, particularly a young and educated cohort (21). The existing literature needs to look into the acceptance of older adults, the primary healthcare service users. (109). Consequently, this study has significant theoretical contributions to older adults’ acceptance of chatbots in healthcare delivery. This study developed and validated an expanded UTAUT model, including aging factors of perceived physical condition, self-actualization needs, and technology anxiety, to reveal the main factors that affect older adults’ intention and behavior to adopt chatbots in healthcare delivery. Furthermore, the study adds to the growing literature on experience and technology acceptance, with experience identified as moderate chatbot usage among older adults. The study presents the following insights.
First, the results suggest that performance expectancy and effort expectancy significantly influenced older adults’ behavioral intention to use chatbots in healthcare delivery. Consistent with previous studies, older adults want to avoid complex features and put in less effort and learning costs than younger people (110). This finding supports prior research. The ease of use and perceived usefulness are essential factors in technology adoption (63). Older users are more likely to adopt and integrate chatbots into their daily routines if the chatbot technology is easy to use and does not require much effort (52). Furthermore, social influence was a significant predictor of chatbot adoption among older adults in healthcare services. Consistent with the qualitative research on chatbots, older users may rely on recommendations from their peers (66). As older people are often not experts on many health-related issues, they are influenced by significant others in their social groups, such as their children, friends, and family members’ doctors (111). It is worth further noticing that facilitating conditions were the most important predictor of use behavior, consistent with previous studies (71). It is critical to have technical equipment and descriptive information to support older adults using chatbots in healthcare delivery.
Secondly, the results suggest that experience can moderate the impact of effort expectancy and social influence on older adults’ behavioral intention. Consistent with previous studies (52), low-experience users are more likely to put in less effort and learning costs, care more about technical support, and are more likely to listen to people. In contrast, older users with higher experience levels are less likely to be influenced by social media influence (23). This may be due to their greater reliance on their judgment and experience and their more nuanced understanding of the limitations and capabilities of the tool (57). Notably, the effect of facilitation conditions was stable and unmoderated. It further confirms that facilitation condition is an essential determinant in chatbot adoption for both older adults with high and low experience levels.
Finally, concerning the aging factors, the results suggest that the perceived physical condition is an obstacle to adults’ use behavior. Previous studies have found no negative correlation between the perceived physical condition and adoption due to the participants being physically healthy, and perceived physical condition by itself does not naturally lead to a better intention to adopt (54, 112). However, the participants of this study are older adults aged 60 years and above. Older adults’ hearing, vision, and cognitive abilities decline, so they become less sensitive to sound and take longer to find the right words to express themselves, hindering chatbot usage behavior. Furthermore, this study confirms that self-actualization needs are essential indicators of older adults’ use behavior. Previous studies have shown consistent results (113). Increasing the self-actualization needs of older adults can narrow the digital divide in technology-related opportunities and support (55). However, contrary to the assumption, technology anxiety did not impact adults’ use behavior. Previous studies have paid more attention to assistive technologies and health information technology, such as telehealth (50), wearable devices (69), and mobile health apps (114). Unlike these technologies, which require an operational threshold, chatbots use natural language interactions to deliver healthcare, reduce medication errors, and analyze health conditions (115, 116). The technology anxiety induced by chatbots could be alleviated due to natural, intuitive, and simple interactions (114).
This study provides practical insights for designing and implementing more aging-inclusive chatbots in healthcare. The use of chatbots in the field of older adults is still emerging, with a lack of specifically designed options for older users (109). The study suggests that designers should focus on enhancing the performance expectancy and effort expectancy of chatbots to promote older adults’ adoption of chatbots within the healthcare sector. For instance, many older adults cannot look at a picture and understand the meaning in front of minimalist icons designed for younger people (117). Thus, designers can address differences in icon comprehension related to participant age and design principles by adding text labels (118). In addition, the task-oriented interaction design is effective for less experienced older adults because it reduces the cost of effort and cognitive load for older adults (119). Meeting the needs of older adults through tailored and targeted approaches is essential, which could reduce the effort required for them to seek information independently, thereby increasing their use of chatbots in healthcare services. For instance, developers can use the geographic location of older adults to push nearby hospitals to them (120), automatically recommending relevant medical services based on personalized information (121, 122).
Moreover, policymakers should focus mainly on facilitating conditions and social influence and tailor their implementation strategies to ensure maximum reach and adoption of the chatbots (52). Policymakers should emphasize the provision and construction of infrastructure and expand more equipment for older adults (123). In addition, chatbot education and training programs in healthcare should be structured so that older adults receive the guidance they need at the right level of experience. For example, older adults with lower levels of experience are more susceptible to the positive effects of social influences, so training can be structured through creating interest and learning groups by focusing on groups with similar learning experiences (25). Intergenerational cooperation programs can also be developed to allow older adults to receive support from their children.
Finally, the perceived physical condition is a primary barrier to technology acceptance among older adults, and healthy conditions serve as a precondition for older adults’ adoption of chatbots (75). The multimodal interactions of chatbots can compensate to some extent for sensory or cognitive impairments and mitigate the negative impact of the perceived physical condition on older adults. For example, voice input commands can address, to some extent, the problem of finger inflexibility in older adults (124). The depression and Alzheimer’s screening tool Alexa requires only a yes or no answer to the questions to complete the assessment (125). Refer older adults for medical advice and health management services by uploading a picture of a disease condition or a medical report (126, 127). Therefore, healthcare providers can integrate chatbots into existing systems to provide multimodal interaction capabilities. Specifically, chatbots can be compatible with assistive devices such as hearing aids and vital signs monitors (128), providing voice input (129) and screen-reading capabilities (130), thereby countering the barriers posed by declining physical condition and increasing the use of chatbots among older adults.
Despite the theoretical and practical contributions of this study, there are limitations. First, while convenience sampling allows us to collect data from different regions of China, this approach may need to be more represented. Future studies should consider more systematic sampling methods, such as stratified random sampling or whole cluster sampling, to improve the representability of the sample. Second, most of the samples collected for this study were from urban areas in China. This may be because older adults in urban areas are more likely to have experience with chatbot healthcare technology than those in rural areas. For the findings to have potential for generalization, future surveys should consider a better representation of the population across all samples of demographic variables. The proportion of rural older adults can be increased by providing them with equipment, technical support, and training for the first use of chatbots. Finally, technology adoption among older adults is complex and multifaceted. Future research should consider the impact of different influencing factors, such as investigating the perceived privacy risks and perceived psychological risks of older adults in accepting and adopting chatbots.
This study extends the original UTAUT model with new variables from the perspective of older adult characteristics. It demonstrates that UTAUT theory can serve as a valid theoretical lens for understanding older adults’ acceptance of chatbots in healthcare delivery. The results highlight that facilitating conditions, perceived physical conditions, and self-actualization needs play important roles in older adults’ use behavior. Furthermore, this study tests the moderating effect of experience on technology adoption among older adults. Older adults with lower experience levels were more concerned about ease of use and social support. Understanding the factors that motivate older adults to use chatbots in healthcare delivery can offer valuable guidance for developing inclusive policies and designing age-friendly chatbots in healthcare delivery.
The original contributions presented in the study are included in the article/Supplementary material, further inquiries can be directed to the corresponding author.
The study ethics procedures were executed according to the 1964 Helsinki Declaration and its later amendments or comparable ethical standards and do not require ethical external approval. This exemption was because the data was anonymous and there is no way for readers to be able to identify the participants. There are no name lists that correspond to the respondents of the questionnaire. All subjects were informed about the research and all participants included in the study provided informed consent. All respondents were volunteers and were assured that their responses would remain anonymous, their confidentiality would be maintained, and their answers would be used only for research purposes.
SY: Writing – review & editing. TC: Writing – original draft.
The author(s) declare that no financial support was received for the research, authorship, and/or publication of this article.
The authors are grateful for the support of the Joint Research Program of Nanjing Forestry University, Jiangsu Co-Innovation Center of Efficient Processing and Utilization of Forest Resources, College of Furnishings and Industrial Design (Nanjing Forestry University, Nanjing 210037, China) and Major Project to Promote the Implementation of the 14th Five-Year Plan for the Integrated Development of the Yangtze River Delta - Public Service Platform for Social Assistance in the Yangtze River Delta (Project Code: 2201-320000-04-04-685162).
The authors declare that the research was conducted in the absence of any commercial or financial relationships that could be construed as a potential conflict of interest.
All claims expressed in this article are solely those of the authors and do not necessarily represent those of their affiliated organizations, or those of the publisher, the editors and the reviewers. Any product that may be evaluated in this article, or claim that may be made by its manufacturer, is not guaranteed or endorsed by the publisher.
The Supplementary material for this article can be found online at: https://www.frontiersin.org/articles/10.3389/fpubh.2024.1435329/full#supplementary-material
1. Jeon, J-B, Lee, S, and Choi, SY. A systematic review of research on speech-recognition Chatbots for language learning: implications for future directions in the era of large language models. Interact Learn Environ. (2023) 32:4613–31. doi: 10.1080/10494820.2023.2204343
2. Hall, CS, Fottrell, E, Wilkinson, S, and Byass, P. Assessing the impact of Mhealth interventions in low- and middle-income countries - what has been shown to work? Glob Health Action. (2014) 7:1–12. doi: 10.3402/gha.v7.25606
3. Li, J, Xie, L, Chen, Z, Shi, L, Chen, R, Ren, Y, et al. An Aiot-based assistance system for visually impaired people. Electronics. (2023) 12:760. doi: 10.3390/electronics12183760
4. Huang, C, Zhong, H, Prakash, P, Shi, D, Yuan, X, and Pan, M. Eve said yes: Airbone authentication for head-wearable smart voice assistant. Arxiv. (2023) 2309:15203. doi: 10.48550/arXiv.2309.15203
5. Buddi, SS, Sarawgi, UO, Heeramun, T, Sawnhey, K, Yanosik, E, Rathinam, S, et al. Efficient multimodal neural networks for trigger-less voice assistants. Arxiv. (2023). doi: 10.48550/arXiv.2305.12063
6. Baek, S, Kim, J, Lee, J, and Lee, M. Implementation of a virtual assistant system based on deep multi-modal data integration. J Signal Processing Systems for Signal Image and Video Technol. (2023) 96:179–89. doi: 10.1007/s11265-022-01829-5
7. Acosta, JN, Falcone, GJ, Rajpurkar, P, and Topol, EJ. Multimodal Biomedical Ai. Nat Med. (2022) 28:1773–84. doi: 10.1038/s41591-022-01981-2
8. Ho, J-Y, Ju, G, Hong, S, An, J, and Lee, CC. Factors influencing customer satisfaction with Ar shopping assistant applications in E-commerce: An empirical analysis utilizing text-mining techniques. Aslib J Inf Manag. (2023). doi: 10.1108/ajim-03-2023-0089
9. Harrington, CN, and Egede, L, editors. “Trust, comfort and relatability: understanding Black older adults’ perceptions of Chatbot Design for Health Information Seeking.” CHI conference on Human Factors in Computing Systems (CHI); 23-28; Hamburg, GERMANY (2023).
10. Softic, A, Husic, JB, Softic, A, and Barakovic, S, editors. “Health Chatbot: design, implementation, acceptance and usage motivation.” 20th international symposium on INFOTEH-JAHORINA (INFOTEH); (2021) 2020. East Sarajevo, BOSNIA & HERCEG.
11. Ayers, JW, Poliak, A, Dredze, M, Leas, EC, Zhu, Z, Kelley, JB, et al. Comparing physician and artificial intelligence Chatbot responses to patient questions posted to a public social media forum. JAMA Intern Med. (2023) 183:589–96. doi: 10.1001/jamainternmed.2023.1838
12. Lee, P, Bubeck, S, and Petro, J. Benefits, limits, and risks of Gpt-4 as an Ai Chatbot for medicine. N Engl J Med. (2023) 388:1233–9. doi: 10.1056/NEJMsr2214184
13. Boutayeb, A, and Boutayeb, S. The burden of non communicable diseases in developing countries. Int J Equity Health. (2005) 4:2. doi: 10.1186/1475-9276-4-2
14. Corbett, CF, Bowers, DC, Combs, EM, Parmer, M, Jones, K, Patel, K, et al. Using virtual home assistants to address vulnerable adults’ complex care needs: An exploratory feasibility study. J Gerontol Nurs. (2023) 49:33–40. doi: 10.3928/00989134-20230512-05
15. Kim, S, and Choudhury, A. Exploring older adults’ perception and use of smart speaker-based voice assistants: a longitudinal study. Comput Hum Behav. (2021) 124:106914. doi: 10.1016/j.chb.2021.106914
16. Liu, WD, Chuang, KY, and Chen, KY, editors. “The design and implementation of a Chatbot’s character for elderly care.” International Conference on System Science and Engineering (ICSSE); 28–30; Taipei, TAIWAN (2018).
17. Miura, C, Chen, S, Saiki, S, Nakamura, M, and Yasuda, K. Assisting personalized healthcare of elderly people: developing a rule-based virtual caregiver system using Mobile Chatbot. Sensors (Basel). (2022) 22:829. doi: 10.3390/s22103829
18. Gudala, M, Ross, MET, Mogalla, S, Lyons, M, Ramaswamy, P, and Roberts, K. Benefits of, barriers to, and needs for an artificial intelligence-powered medication information voice Chatbot for older adults: interview study with geriatrics experts. JMIR aging. (2022) 5:e32169. doi: 10.2196/32169
19. Corbett, CF, Wright, PJ, Jones, K, and Parmer, M. Voice-activated virtual home assistant use and social isolation and loneliness among older adults: Mini review. Front Public Health. (2021) 9:742012. doi: 10.3389/fpubh.2021.742012
20. Chou, Y-H, Lin, C, Lee, S-H, Lee, Y-F, and Cheng, L-C. User-friendly Chatbot to mitigate the psychological stress of older adults during the Covid-19 pandemic: development and usability study. JMIR Form Res. (2024) 8:e49462. doi: 10.2196/49462
21. Hardt, JH, and Hollis-Sawyer, L. Older adults seeking healthcare information on the internet. Educ Gerontol. (2007) 33:561–72. doi: 10.1080/03601270701364628
22. Wilczewski, H, Soni, H, Ivanova, J, Ong, T, Barrera, JF, Bunnell, BE, et al. Older adults’ experience with virtual conversational agents for health data collection. Front Digital Health. (2023) 5:5. doi: 10.3389/fdgth.2023.1125926
23. Liu, N, Pu, Q, Shi, Y, Zhang, S, and Qiu, L. Older adults’ interaction with intelligent virtual assistants: the role of information modality and feedback. Int J Human–Computer Interaction. (2022) 39:1162–83. doi: 10.1080/10447318.2022.2074667
24. Ma, Y, and Luo, M. Older People’s intention to use medical apps during the Covid-19 pandemic in China: An application of the unified theory of acceptance and use of technology (Utaut) model and the Technology of Acceptance Model (Tam). Ageing Soc. (2022) 44:1515–32. doi: 10.1017/s0144686x22000423
25. Yang, C-C, Liu, C, and Wang, Y-S. The acceptance and use of smartphones among older adults: differences in Utaut determinants before and after training. Library Hi Tech. (2023) 41:1357–75. doi: 10.1108/lht-12-2021-0432
26. Soh, PY, Heng, HB, Selvachandran, G, Le Quynh, A, Hoang Thi Minh, C, Le Hoang, S, et al. Perception, acceptance and willingness of older adults in Malaysia towards online shopping: a study using the Utaut and Irt models. J Ambient Intell Humaniz Comput. (2020). doi: 10.1007/s12652-020-01718-4
27. Bixter, MT, Blocker, KA, Mitzner, TL, Prakash, A, and Rogers, WA. Understanding the use and non-use of social communication technologies by older adults: a qualitative test and extension of the Utaut model. Gerontechnology: Int J fundamental aspects of technol serve the ageing society. (2019) 18:70–88. doi: 10.4017/gt.2019.18.2.002.00
28. Kim, JW, Jo, HI, and Lee, BG. The study on the factors influencing on the behavioral intention of Chatbot Service for the Financial Sector: focusing on the Utaut model. J Digital Contents Society. (2019) 20:41–50. doi: 10.9728/dcs.2019.20.1.41
29. Pawlik, VP, editor. “Design matters! How visual gendered anthropomorphic design cues moderate the determinants of the behavioral intention towards using Chatbots.” 5th international workshop on Chatbot research and design (CONVERSATIONS); (2021), 2022. Univ Oslo, ELECTR NETWORK.
30. Tian, W, Ge, J, Zhao, Y, and Zheng, X. Ai Chatbots in Chinese higher education: adoption, perception, and influence among graduate students-an integrated analysis utilizing Utaut and Ecm models. Front Psychol. (2024) 15:15. doi: 10.3389/fpsyg.2024.1268549
31. Balakrishnan, J, Abed, SS, and Jones, P. The role of Meta-Utaut factors, perceived anthropomorphism, perceived intelligence, and social self-efficacy in Chatbot-based services? Technol Forecast Soc Chang. (2022) 180:121692. doi: 10.1016/j.techfore.2022.121692
32. Iancu, I, and Iancu, B. Interacting with Chatbots later in life: a technology acceptance perspective in Covid-19 pandemic situation. Front Psychol. (2023) 13:13. doi: 10.3389/fpsyg.2022.1111003
33. Budhathoki, T, Zirar, A, Njoya, ET, and Timsina, A. Chatgpt adoption and anxiety: a cross-country analysis Utilising the unified theory of acceptance and use of technology (Utaut). Stud High Educ. (2024) 49:831–46. doi: 10.1080/03075079.2024.2333937
34. Ponathil, A, Ozkan, F, Bertrand, J, Agnisarman, S, Narasimha, S, Welch, B, et al. An empirical study investigating the user acceptance of a virtual conversational agent Interface for family health history collection among the geriatric population. Health Informatics J. (2020) 26:2946–66. doi: 10.1177/1460458220955104
35. Zhong, R, Ma, M, Zhou, Y, Lin, Q, Li, L, and Zhang, N. User acceptance of smart home voice assistant: a comparison among younger, middle-aged, and older adults. Univ Access Inf Soc. (2024) 23:275–292. doi: 10.1007/s10209-022-00936-1
36. Davis, FD. Perceived usefulness, perceived ease of use, and user acceptance of information technology. MIS Q. (1989) 13:319–40. doi: 10.2307/249008
37. Rogers, EM. Diffusion of innovations: modifications of a model for telecommunications. Heidelberg: Springer Berlin Heidelberg. (1995) 6:151.
38. Fishbein, M, and Ajzen, I. Belief, attitude, intention, and behavior: An introduction to theory and research. Contemp Sociol. (1977) 6:244. doi: 10.2307/2065853
39. Sheppard, BH, Hartwick, J, and Warshaw, PR. The theory of reasoned action: a Meta-analysis of past research with recommendations for modifications and future research. J Consum Res. (1988) 15:325–43. doi: 10.1086/209170
40. Vallerand, RJ, and Zanna, M. P. Toward a hierarchical model of intrinsic and extrinsic motivation In: Advances in experimental social psychology, vol. 29. San Diego, CA, US: Academic Press (1997). 271–360.
41. Ajzen, I. The theory of planned behavior. Organ Behav Hum Decis Process. (1991) 50:179–211. doi: 10.1016/0749-5978(91)90020-T
42. Taylor, S, and Todd, P. Assessing it usage: the role of prior experience. MIS Q. (1995) 19:561–70. doi: 10.2307/249633
43. Thompson, RL, Higgins, CA, and Howell, JM. Personal computing: toward a conceptual model of utilization. MIS Q. (1991) 15:125–43. doi: 10.2307/249443
44. Bandura, A. National Inst of Mental Health. Social foundations of thought and action. Prentice-Hall, Inc. (1986).
45. Venkatesh, V, Morris, MG, Davis, GB, and Davis, FD. User acceptance of information technology: toward a unified view. MIS Q. (2003) 27:425–78. doi: 10.2307/30036540
46. Venkatesh, V, Thong, JY, and Xu, X. Consumer acceptance and use of information technology: extending the unified theory of acceptance and use of technology. MIS Q. (2012) 36:157–78. doi: 10.2307/41410412
47. Charness, N, and Boot, WR. Aging and information technology use:potential and barriers. Curr Dir Psychol Sci. (2009) 18:253–8. doi: 10.1111/j.1467-8721.2009.01647.x
48. Osta, A, Kokkinaki, AI, and Chedrawi, C, “Online health communities: The impact of Ai conversational agents on users.” European, Mediterranean and middle eastern conference on information systems; (2021).
49. Chen, G, Fan, J, and Azam, M. Exploring artificial intelligence (Ai) Chatbots adoption among research scholars using unified theory of acceptance and use of technology (Utaut). J Librariansh Inf Sci. (2024). doi: 10.1177/09610006241269189
50. Fu, J, Mouakket, S, and Sun, Y. Factors affecting customer readiness to trust Chatbots in an online shopping context. J Glob Inf Manag. (2024) 32:1–22. doi: 10.4018/jgim.347503
51. Czaja, SJ, Charness, N, Fisk, AD, Hertzog, C, Nair, SN, Rogers, WA, et al. Factors predicting the use of technology: findings from the Center for Research and Education on aging and technology enhancement (create). Psychol Aging. (2006) 21:333–52. doi: 10.1037/0882-7974.21.2.333
52. Menon, D, and Shilpa, K. "chatting with Chatgpt": analyzing the factors influencing users’ intention to use the open Ai’s Chatgpt using the Utaut model. Heliyon. (2023) 9:e20962. doi: 10.1016/j.heliyon.2023.e20962
53. Lee, C, and Coughlin, JF. Perspective: older adults’ adoption of technology: An integrated approach to identifying determinants and barriers. J Prod Innov Manag. (2015) 32:747–59. doi: 10.1111/jpim.12176
54. Song, Y, Yang, Y, and Cheng, P. The investigation of adoption of voice-user Interface (Vui) in smart home systems among Chinese older adults. Sensors (Basel). (2022) 22:614. doi: 10.3390/s22041614
55. Fox, G, and Connolly, R. Mobile health technology adoption across generations: narrowing the digital divide. Inf Syst J. (2018) 28:995–1019. doi: 10.1111/isj.12179
56. Jeng, M-Y, Pai, F-Y, and Yeh, T-M. Antecedents for older adults’ intention to use smart health wearable devices-technology anxiety as a moderator. Behav Sci. (2022) 12:114. doi: 10.3390/bs12040114
57. Hsu, CW, and Peng, CC. What drives older adults’ use of Mobile registration apps in Taiwan? An investigation using the extended Utaut model. Inform Health Soc Care. (2022) 47:258–73. doi: 10.1080/17538157.2021.1990299
58. Erjavec, J, and Manfreda, A. Online shopping adoption during Covid-19 and social isolation: extending the Utaut model with herd behavior. J Retail Consum Serv. (2022) 65:102867. doi: 10.1016/j.jretconser.2021.102867
59. Bokolo, AJ. Exploring the adoption of telemedicine and virtual software for Care of Outpatients during and after Covid-19 pandemic. Irish J Med Sci. (2021) 190:1–10. doi: 10.1007/s11845-020-02299-z
60. Connolly, SL, Miller, CJ, Lindsay, JA, and Bauer, MS. A systematic review of providers’ attitudes toward Telemental health via videoconferencing. Clin Psychol Sci Prac. (2020) 27:311. doi: 10.1111/cpsp.12311
61. Camilleri, MA. Factors affecting performance expectancy and intentions to use Chatgpt: using Smartpls to advance an information technology acceptance framework. Technol Forecast Soc Chang. (2024) 201:123247. doi: 10.1016/j.techfore.2024.123247
62. Li, L, Peng, W, and Rheu, MMJ. Factors predicting intentions of adoption and continued use of artificial intelligence Chatbots for mental health: examining the role of Utaut model, stigma, privacy concerns, and artificial intelligence hesitancy. Telemedicine and E-Health. (2024) 30:722–30. doi: 10.1089/tmj.2023.0313
63. Lee, U-K. Accessing the acceptance of the speech recognition based virtual assistant service: applying Utaut model. J Product Res. (2020) 38:111–20. doi: 10.36345/KACST.2020.38.5.013
64. Polyportis, A, and Pahos, N. Understanding students’ adoption of the Chatgpt Chatbot in higher education: the role of anthropomorphism, trust, design novelty and institutional policy. Behav Inform Technol. (2024) 22:1–22. doi: 10.1080/0144929x.2024.2317364
65. Ben Arfi, W, Ben Nasr, I, Kondrateva, G, and Hikkerova, L. The role of Trust in Intention to use the Iot in Ehealth: application of the modified Utaut in a consumer context. Technol Forecast Soc Chang. (2021) 167:120688. doi: 10.1016/j.techfore.2021.120688
66. Mogaji, E, Balakrishnan, J, Nwoba, AC, and Nguyen, NP. Emerging-market consumers’ interactions with banking Chatbots. Telematics Inform. (2021) 65:101711. doi: 10.1016/j.tele.2021.101711
67. Zhang, L. Breaking barriers: a dual-factor model unraveling ageism in socially assistive robot adoption among older Chinese adults. Int J Human-Computer Interaction. (2024) 36:1–17. doi: 10.1080/10447318.2024.2314352
68. Chen, Y, Yuan, J, Shi, L, Zhou, J, Wang, H, Li, C, et al. Understanding the role of technology anxiety in the adoption of digital health technologies (Dhts) by older adults with chronic diseases in Shanghai: An extension of the unified theory of acceptance and use of technology (Utaut) model. Health. (2024) 12:421. doi: 10.3390/healthcare12141421
69. Wu, C, and Lim, GG. Investigating older adults users’ willingness to adopt wearable devices by integrating the technology acceptance model (Utaut2) and the technology readiness index theory. Front Public Health. (2024) 12:12. doi: 10.3389/fpubh.2024.1449594
70. Lu, CC, and Tsai-Lin, TF. Are older adults special in adopting public Ehealth service initiatives? The modified model of Utaut. SAGE Open. (2024) 14:20. doi: 10.1177/21582440241228639
71. Priya, B, and Sharma, V. Exploring users’ adoption intentions of intelligent virtual assistants in financial services: An anthropomorphic perspectives and socio-psychological perspectives. Comput Hum Behav. (2023) 148:107912. doi: 10.1016/j.chb.2023.107912
72. Cimperman, M, Makovec Brenčič, M, and Trkman, P. Analyzing older users’ home telehealth services acceptance behavior—applying an extended Utaut model. Int J Med Inform. (2016) 90:22–31. doi: 10.1016/j.ijmedinf.2016.03.002
73. Yang, C-C, Li, C-L, Yeh, T-F, and Chang, Y-C. Assessing older adults’ intentions to use a smartphone: using the Meta–unified theory of the acceptance and use of technology. Int J Environ Res Public Health. (2022) 19:5403. doi: 10.3390/ijerph19095403
74. Deng, Z, Mo, X, and Liu, S. Comparison of the middle-aged and older users’ adoption of Mobile health Services in China. Int J Med Inform. (2014) 83:210–24. doi: 10.1016/j.ijmedinf.2013.12.002
75. Chen, J, Wang, T, Fang, Z, and Wang, H. Research on elderly users’ intentions to accept wearable devices based on the improved Utaut model. Front Public Health. (2023) 10:10. doi: 10.3389/fpubh.2022.1035398
76. Edelstein, B, and Scandiffio, J. Effectiveness of an assess and restore program in treating older adults with physiological and functional decline: the heart program. Arch Gerontol Geriatr. (2022) 99:104609. doi: 10.1016/j.archger.2021.104609
77. Kaufman, SB. Self-actualizing people in the 21st century: integration with contemporary theory and research on personality and well-being. J Humanist Psychol. (2023) 63:51–83. doi: 10.1177/0022167818809187
78. Phang, CW, Sutanto, J, Kankanhalli, A, Li, Y, Tan, BCY, and Teo, H-H. Senior citizens’ acceptance of information systems: a study in the context of E-government services. IEEE Trans Eng Manag. (2006) 53:555–69. doi: 10.1109/TEM.2006.883710
79. Talukder, MS, Sorwar, G, Bao, YK, Ahmed, JU, and Palash, MAS. Predicting antecedents of wearable healthcare technology acceptance by elderly: a combined Sem-neural network approach. Technol Forecast Soc Chang. (2020) 150:119793. doi: 10.1016/j.techfore.2019.119793
80. Zhou, Y-L, Chen, C-L, Chang, S-J, and Wu, B-S. Home-based intelligent exercise system for Seniors&Rsquo; healthcare: the example of golf croquet. Sports. (2023) 11:207. doi: 10.3390/sports11110207
81. Xue, L, Yen, CC, Chang, L, Chan, HC, Tai, BC, Tan, SB, et al. An exploratory study of ageing Women’s perception on access to health informatics via a Mobile phone-based intervention. Int J Med Inform. (2012) 81:637–48. doi: 10.1016/j.ijmedinf.2012.04.008
82. Kim, H, Freddolino, PP, and Greenhow, C. Older adults’ technology anxiety as a barrier to digital inclusion: a scoping review. Educ Gerontol. (2023) 49:1021–38. doi: 10.1080/03601277.2023.2202080
83. Chung, J, Brakey, HR, Reeder, B, Myers, O, and Demiris, G. Community-dwelling older adults’ acceptance of smartwatches for health and location tracking. Int J Older People Nursing. (2023) 18:e12490. doi: 10.1111/opn.12490
84. Hsieh, P-J. An empirical investigation of patients’ acceptance and resistance toward the health cloud: the dual factor perspective. Comput Hum Behav. (2016) 63:959–69. doi: 10.1016/j.chb.2016.06.029
85. van de Vijver, FJR, and Leung, K. Methods and data analysis for cross-cultural research. 2nd ed. Cambridge: Cambridge University Press (2021).
86. Geisen, E, and Murphy, J. A compendium of web and Mobile survey pretesting methods. Advances in questionnaire design, development, Evaluation and Testing. (2020):287–314. doi: 10.1002/9781119263685.ch12
87. Wei, W, Gong, X, Li, J, Tian, K, and Xing, K. A study on community older People’s willingness to use smart home—an extended technology acceptance model with intergenerational relationships. Front Public Health. (2023) 11:11. doi: 10.3389/fpubh.2023.1139667
88. Mensah, IK, and Khan, MK. Unified theory of acceptance and use of technology (Utaut) model: factors influencing Mobile banking services’ adoption in China. SAGE Open. (2024) 14:21582440241234230. doi: 10.1177/21582440241234230
89. Cornesse, C, Blom, AG, Dutwin, D, Krosnick, JA, De Leeuw, ED, Legleye, S, et al. A review of conceptual approaches and empirical evidence on probability and nonprobability sample survey research. J Survey Statistics and Methodol. (2020) 8:4–36. doi: 10.1093/jssam/smz041
90. Alam, MZ, Hoque, MR, Hu, W, and Barua, Z. Factors influencing the adoption of Mhealth Services in a Developing Country: a patient-centric study. Int J Inf Manag. (2020) 50:128–43. doi: 10.1016/j.ijinfomgt.2019.04.016
91. Rudnicka, E, Napierala, P, Podfigurna, A, Meczekalski, B, Smolarczyk, R, and Grymowicz, M. The World Health Organization (who) approach to healthy ageing. Maturitas. (2020) 139:6–11. doi: 10.1016/j.maturitas.2020.05.018
92. Porter, COLH, Outlaw, R, Gale, JP, and Cho, TS. The use of online panel data in management research: a review and recommendations. J Manag. (2019) 45:319–44. doi: 10.1177/0149206318811569
93. Jackson, DL. Revisiting sample size and number of parameter estimates: some support for the N:Q hypothesis. Struct Equ Model Multidiscip J. (2003) 10:128–41. doi: 10.1207/S15328007SEM1001_6
94. Tenenhaus, M, Vinzi, VE, Chatelin, Y-M, and Lauro, C. Pls path modeling. Computational Statistics & Data Analysis. (2005) 48:159–205. doi: 10.1016/j.csda.2004.03.005
95. Wetzels, M, Odekerken-Schröder, G, and Cv, O. Using Pls path modeling for assessing Hierarchial construct models: guidelines and Impirical illustration. Manag Inf Syst Q. (2009) 33:177–95. doi: 10.2307/20650284
96. Hair, JF Jr, Sarstedt, M, Hopkins, L, and Kuppelwieser, VG. Partial least squares structural equation modeling (Pls-Sem). Eur Bus Rev. (2014) 26:106–21. doi: 10.1108/EBR-10-2013-0128
97. Chin, WW, Marcolin, BL, and Newsted, PR. A partial least squares latent variable modeling approach for measuring interaction effects: results from a Monte Carlo simulation study and an electronic-mail emotion/adoption study. Inf Syst Res. (2003) 14:189–217. doi: 10.1287/isre.14.2.189.16018
98. Barroso, A, González-López, ÓR, Sanguino, R, and Buenadicha-Mateos, M. Analysis and evaluation of the largest 500 family firms’ websites through Pls-Sem technique. Sustain For. (2018) 10:557. doi: 10.3390/su10020557
99. Hair, JF, Ringle, CM, and Sarstedt, M. Pls-Sem: indeed a silver bullet. J Mark Theory Pract. (2014) 19:139–52. doi: 10.2753/mtp1069-6679190202
100. Fornell, C, and Larcker, DF. Structural equation models with unobservable variables and measurement error: algebra and statistics. J Mark Res. (1981) 18:382–8. doi: 10.1177/002224378101800313
101. Thorndike, RM. Book review: psychometric theory. Appl Psychol Meas. (1995) 19:303–5. doi: 10.1177/014662169501900308
102. Hair, JF, Black, WC, Babin, BJ, and Anderson, RE. Multivariate data analysis. (7th Edition). United Kingdom of Great Britain and Northern Ireland, UK: PearsonI. (2009).
103. Henseler, J, Ringle, CM, and Sinkovics, RR. The use of partial least squares path modeling in international marketing In: RR Sinkovics and PN Ghauri, editors. New challenges to international marketing. US: Emerald Group Publishing Limited (2009). 277–319.
104. Falk, RF, and Miller, NB. A Primer for Soft Modeling. The University of Akron Press: Akron, OH. (1992).
105. Hair, J, and Alamer, A. Partial least squares structural equation modeling (Pls-Sem) in second language and education research: guidelines using an applied example. Res Methods in Applied Linguistics. (2022) 1:100027. doi: 10.1016/j.rmal.2022.100027
106. Cheah, J-H, Amaro, S, and Roldán, JL. Multigroup analysis of more than two groups in Pls-Sem: a review, illustration, and recommendations. J Bus Res. (2023) 156:113539. doi: 10.1016/j.jbusres.2022.113539
107. Baron, RM, and Kenny, DA. The moderator-mediator variable distinction in social psychological research: conceptual, strategic, and statistical considerations. J Pers Soc Psychol. (1986) 51:1173–82. doi: 10.1037/0022-3514.51.6.1173
108. Moldt, JA, Festl-Wietek, T, Mamlouk, AM, Nieselt, K, Fuhl, W, and Herrmann-Werner, A. Chatbots for future docs: exploring medical students’ attitudes and knowledge towards artificial intelligence and medical Chatbots. Med Educ. (2023) 28:659. doi: 10.1080/10872981.2023.2182659
109. Zhang, Q, Wong, AKC, and Bayuo, J. The role of Chatbots in enhancing health Care for Older Adults: a scoping review. J Am Med Dir Assoc. (2024) 25:105108. doi: 10.1016/j.jamda.2024.105108
110. Garcia de Blanes Sebastian, M, Sarmiento Guede, JR, and Antonovica, A. Application and extension of the Utaut2 model for determining behavioral intention factors in use of the artificial intelligence virtual assistants. Front Psychol. (2022) 13:993935. doi: 10.3389/fpsyg.2022.993935
111. Zhou, J, Rau, P-LP, and Salvendy, G. Older adults’ use of smart phones: An investigation of the factors influencing the acceptance of new functions. Behav Inform Technol. (2014) 33:552–60. doi: 10.1080/0144929X.2013.780637
112. Teng, Y-M, Wu, K-S, Wang, W-C, and Chen, L-W. What factors drive consumers’ desire to continue using food delivery apps (Fda) in Taiwan after the Covid-19 pandemic? J Hosp Tour Technol. (2023) 14:878–92. doi: 10.1108/jhtt-09-2022-0259
113. Lin, W, Chen, H-C, and Yueh, H-P. Using different error handling strategies to facilitate older users’ interaction with Chatbots in learning information and communication technologies. Front Psychol. (2021) 12:12. doi: 10.3389/fpsyg.2021.785815
114. Bertolazzi, A, Quaglia, V, and Bongelli, R. Barriers and facilitators to health technology adoption by older adults with chronic diseases: An integrative systematic review. BMC Public Health. (2024) 24:506. doi: 10.1186/s12889-024-18036-5
115. Liu, IV, Liu, FY, Xiao, YT, Huang, YJ, Wu, SM, and Ni, SG. Investigating the key success factors of Chatbot-based positive psychology intervention with retrieval- and generative pre-trained transformer (Gpt)-based Chatbots. Int J Human-Computer Interaction. (2024) 12:1. doi: 10.1080/10447318.2023.2300015
116. Lahat, A, Shachar, E, Avidan, B, Glicksberg, B, and Klang, E. Evaluating the utility of a large language model in answering common patients’ gastrointestinal health-related questions: are we there yet? Diagnostics. (2023) 13:10. doi: 10.3390/diagnostics13111950
117. Chen, R, Huang, J, and Zhou, J. Skeuomorphic or flat icons for an efficient visual search by younger and older adults? Appl Ergon. (2020) 85:103073. doi: 10.1016/j.apergo.2020.103073
118. Song, XY, Lin, ZB, and Tang, RX. Developing icons for older adults: the roles of icon design categories, text labels, and learning strategies. Int J Ind Ergon. (2024) 102:103613. doi: 10.1016/j.ergon.2024.103613
119. Chattaraman, V, Kwon, W-S, Gilbert, JE, and Ross, K. Should Ai-based, conversational digital assistants employ social- or task-oriented interaction style? A task-competency and reciprocity perspective for older adults. Comput Hum Behav. (2019) 90:315–30. doi: 10.1016/j.chb.2018.08.048
120. Boulos, MNK, Peng, GC, and VoPham, T. An overview of Geoai applications in health and healthcare. Int J Health Geogr. (2019) 18:18. doi: 10.1186/s12942-019-0171-2
121. Johnson, KB, Wei, WQ, Weeraratne, D, Frisse, ME, Misulis, K, Rhee, K, et al. Precision medicine, Ai, and the future of personalized health care. Cts-Clinical and Translational Sci. (2021) 14:86–93. doi: 10.1111/cts.12884
122. Aggarwal, A, Tam, CC, Wu, DZ, Li, XM, and Qiao, S. Artificial intelligence-based Chatbots for promoting health behavioral changes: systematic review. J Med Internet Res. (2023) 25:e40789. doi: 10.2196/40789
123. Kakera, Y, Tandukar, B, Chandra, S, and Martin-Hammond, A, “Building community capacity: exploring voice assistants to support older adults in an independent living community.” CHI conference on Human Factors in Computing Systems (CHI); 23–28; Hamburg, GERMANY (2023).
124. Barricelli, BR, Bondioli, A, Fogli, D, Iemmolo, L, and Locoro, A. Creating routines for Iot ecosystems through conversation with smart speakers. Int J Human-Computer Interaction. (2023) 40:6109–27. doi: 10.1080/10447318.2023.2247845
125. Tan, K, Sekhar, K, Wong, J, Holgado, J, Ameer, M, and Vesonder, G, editors. “Alexa eldercare toolbox: a Smarthome solution for the elderly.” 2020 11th IEEE annual ubiquitous computing, Electronics & Mobile Communication Conference (UEMCON); (2020). 28–31.
126. Cadamuro, J, Cabitza, F, Debeljak, Z, De Bruyne, S, Frans, G, Perez, SM, et al. Potentials and pitfalls of Chatgpt and natural-language artificial intelligence models for the understanding of laboratory medicine test results. Europe: An assessment by the European Federation of Clinical Chemistry and Laboratory Medicine (Eflm) working group on artificial intelligence (Wg-Ai). (2023) 61:1158–1166.
127. Tang, X, Hacker, N, Danish, A, and Chen, S. Patient-reported outcome plus platform for remote symptom management, featuring automated triage system. Int J Radiat Oncol Biol Phys. (2021) 111:E98–e99. doi: 10.1016/j.ijrobp.2021.07.489
128. Apuzzo, C, and Burresi, G, editors. “Designing accessible Chatbots for deaf people.” 11th Mediterranean Conference on Embedded Computing (MECO) / 3rd Summer School on Cyber-Physical + Systems and Internet of Things (CPS and IoT); (2022). Budva, MONTENEGRO.
129. Federici, S, de Filippis, ML, Mele, ML, Borsci, S, Bracalenti, M, Gaudino, G, et al. Inside Pandora’s box: a systematic review of the assessment of the perceived quality of Chatbots for people with disabilities or special needs. Disab Rehab Assistive Technol. (2020) 15:832–7. doi: 10.1080/17483107.2020.1775313
130. Tsai, YT, and Lin, W-A. “Design of an Intelligent Cognition Assistant for people with cognitive impairment.” 20th IEEE International Conference on High Performance Computing and Communications (HPCC) / 16th IEEE International Conference on Smart City (SmartCity) / 4th IEEE International Conference on Data Science and Systems (DSS); (2018). Exeter, ENGLAND.
Keywords: Chatbot, older adults, UTAUT, perceived physical condition, self-actualization needs, technology anxiety, healthcare
Citation: Yu S and Chen T (2024) Understanding older adults’ acceptance of Chatbots in healthcare delivery: an extended UTAUT model. Front. Public Health. 12:1435329. doi: 10.3389/fpubh.2024.1435329
Received: 20 May 2024; Accepted: 04 November 2024;
Published: 19 November 2024.
Edited by:
Amit Mittal, Chitkara University, IndiaReviewed by:
James Leonhardt, University of Nevada, Reno, United StatesCopyright © 2024 Yu and Chen. This is an open-access article distributed under the terms of the Creative Commons Attribution License (CC BY). The use, distribution or reproduction in other forums is permitted, provided the original author(s) and the copyright owner(s) are credited and that the original publication in this journal is cited, in accordance with accepted academic practice. No use, distribution or reproduction is permitted which does not comply with these terms.
*Correspondence: Shulan Yu, eXVzbEBuamZ1LmVkdS5jbg==
Disclaimer: All claims expressed in this article are solely those of the authors and do not necessarily represent those of their affiliated organizations, or those of the publisher, the editors and the reviewers. Any product that may be evaluated in this article or claim that may be made by its manufacturer is not guaranteed or endorsed by the publisher.
Research integrity at Frontiers
Learn more about the work of our research integrity team to safeguard the quality of each article we publish.