- 1RAND Corporation, Boston, MA, United States
- 2Maine Medical Center, Portland, ME, United States
- 3Tufts University School of Medicine, Boston, MA, United States
- 4RAND Corporation, Arlington, VA, United States
- 5RAND Corporation, Santa Monica, CA, United States
- 6RAND Corporation, Pittsburgh, PA, United States
Background: Prior to the development of COVID-19 vaccines, policymakers instituted various non-pharmaceutical interventions (NPIs) to limit transmission. Prior studies have attempted to examine the extent to which these NPIs achieved their goals of containment, suppression, or mitigation of disease transmission. Existing evidence syntheses have found that numerous factors limit comparability across studies, and the evidence on NPI effectiveness during COVID-19 pandemic remains sparse and inconsistent. This study documents the magnitude and variation in NPI effectiveness in reducing COVID-19 transmission (i.e., reduction in effective reproduction rate [Reff] and daily contact rate) in Italy, the United States, the United Kingdom, and China.
Methods: Our rapid review and narrative synthesis of existing research identified 126 studies meeting our screening criteria. We selected four contexts with >5 articles to facilitate a meaningful synthesis. This step yielded an analytic sample of 61 articles that used data from China, Italy, the United Kingdom, and the United States.
Results: We found wide variation and substantial uncertainty around the effectiveness of NPIs at reducing disease transmission. Studies of a single intervention or NPIs that are the least stringent had estimated Reff reductions in the 10–50% range; those that examined so-called “lockdowns” were associated with greater Reff reductions that ranged from 40 to 90%, with many in the 70–80% range. While many studies reported on multiple NPIs, only six of the 61 studies explicitly used the framing of “stringency” or “mild versus strict” or “tiers” of NPIs, concepts that are highly relevant for decisionmakers.
Conclusion: Existing evidence suggests that NPIs reduce COVID-19 transmission by 40 to 90 percent. This paper documents the extent of the variation in NPI effectiveness estimates and highlights challenges presented by a lack of standardization in modeling approaches. Further research on NPI effectiveness at different stringency levels is needed to inform policy responses to future pandemics.
1 Introduction
In the early months of the COVID-19 pandemic, while clinical trials of candidate vaccines were underway, policymakers instituted a wide range of non-pharmaceutical interventions (NPIs), which are defined as strategies intended to limit transmission until a vaccine and other pharmaceutical countermeasures are available to the public (1). These NPIs included various forms of social/physical distancing; such as shelter-in-place orders (often called “lockdowns”), school closures, bans on mass gatherings, mandatory indoor masking, testing requirements, contact tracing, and quarantine (1, 2).
These measures, while implemented for the protection of public health, were costly and disruptive to society (3). Evidence on the extent to which these NPIs “worked,” (i.e., achieved their public health goals of containment, suppression, or mitigation of disease transmission) (4), was urgently needed. Although policymakers and researchers often prefer to rely on evidence from randomized trials, challenges to estimating the effects of NPIs on clinical outcomes abound. These include endogeneity (i.e., policymakers introduce NPIs dynamically in response to COVID-19 case data), lack of consistency (i.e., individual NPIs can be combined in numerous ways and implemented with different levels of stringency and adherence), and the impossibility of randomly assigning individuals to most interventions other than masks. As a result, estimating the impact of NPIs on clinical outcomes is often infeasible and, at best, is done after the estimate is no longer decision-relevant. Given the challenges of conducting randomized studies to answer this critical question, researchers typically used observational data (e.g., historical or geographical) and quasi-experimental methods such as interrupted time-series analysis, and infectious disease modeling (1) to estimate the impact of NPIs on outcomes such as COVID-19 transmission, cases, or deaths. Notable exceptions are two pragmatic randomized studies of the effectiveness of masking. One cluster-randomized study, conducted in Bangladesh, found that “mask wearing averaged 13.3% in villages where no interventions took place but increased to 42.3% in villages” where free masks were distributed in conjunction with promotion efforts, and in villages randomized to mask-wearing interventions, symptomatic seroprevalence was reduced by about 35%; the other study, from Denmark, randomized at the individual level, and results were inconclusive regarding whether an individual recommendation to wear a mask was associated with infection (5, 6).
Following an explosion of studies examining NPI effectiveness, there have been recent efforts to synthesize existing studies of the impact of NPIs on COVID-19 outcomes (1), including a series of rigorous evidence reviews focused on specific NPI types (e.g., masks and face coverings, social distancing and “lockdowns;” test, trace, and isolate) (7–12). These evidence reviews identified substantial variation in COVID-19 modeling approaches and concluded that this variation limits comparability across different studies. An accompanying series of case studies on Hong Kong, New Zealand, and South Korea demonstrated that it was possible to control transmission with early, stringent NPI implementation but that as naturally- or vaccine-induced immunity increased, all three countries relaxed their interventions and moved from suppression to mitigation of transmission (13). While these case studies yield important lessons for policymakers, they cannot be directly generalized to other contexts. Thus, public health practitioners and policymakers at all levels, from national to local, continue to make decisions based on data from contexts that do not reflect their specific situation or produce widely varying estimates of NPI effectiveness.
Furthermore, studies that use absolute observed outcomes such as avoidance of cases, hospitalizations, or deaths as their primary outcomes are common. In fact, they represent one-third of studies from the first year of the pandemic (14) despite these outcomes being biased by factors such as testing availability, inpatient capacity and healthcare infrastructure, and variation in case reporting and categorization of deaths associated with COVID-19. Infectious disease modelers continue to have limited and inconsistent evidence to draw on when parameterizing their models. To avoid model misinterpretation, experts have called for assessing effectiveness of NPIs using epidemiological parameters that measure relative changes in person-to-person transmission at a population level (4, 14).
Focusing on two key limitations of existing research on NPI effectiveness: imprecise definitions of health outcomes and limited attention to local contextual factors when drawing conclusions about these measures, this study builds on prior evidence syntheses. Specifically, we document the variation in NPI effects on COVID-19 transmission measures in four selected geographies: China, Italy, the United States, and the United Kingdom. Despite the challenges of synthesizing existing evidence, we offer suggested next steps for modelers and policymakers.
2 Methods
2.1 Search strategy
To guide our search strategy, we focused on the two main epidemiological measures of disease transmission: time-varying effective reproduction number (often denoted as Re or Reff) and contact rate, typically denoted as b or β (beta).
The study team formulated database search strategies for PubMed (National Institute of Health/National Library of Medicine) and Web of Science Core Collection (Clarivate). Within the Web of Science Core Collection, the search was limited to the following databases: Science Citation Index Expanded (SCI-EXPANDED), Social Sciences Citation Index (SSCI), and Emerging Sources Citation Index (ESCI). This was to decrease the number of unwanted publication types, such as conference proceedings and book chapters, and results from unrelated research areas, such as Arts & Humanities. The following limits were applied to each database search: Articles in peer-reviewed literature, available in English language, and published between November 2019–June 2023.
The primary search was conducted in PubMed and was then translated for Web of Science. The research question was divided into 6 main concepts (search strings) that were combined using Boolean operators: COVID-19, Non-Pharmaceutical Interventions (NPIs), Transmission Prevention and Precautions, Infectious Contacts, Basic Reproduction Number, and Study Type. The Basic Reproduction Number search string was created to increase the yield of relevant studies that estimated the effect of NPIs on quantities versus studies that were estimating the interventions on the number of COVID-19 cases and/or deaths. Study Type was included to narrow the scope of results to the desired type of publications using the PubMed-provided “Article Type” Filters, relevant Medical Subject Headings (MeSH) terms, and the field tag for Title/Abstract ([tiab]).
The searches were conducted on August 2, 2023. The search results from both databases were saved as RIS files and then imported into an EndNote library for de-duplication and to assign details to the records, including database source and the search date. Appendix A contains details on all search strategies.
2.2 Article screening procedures
In the first stage of screening, we reviewed article titles and abstracts for inclusion. The study team excluded articles that met the following criteria: (1) not about COVID-19; (2) not in English; (3) not a report of primary research (e.g., commentaries, letters to the editor); (4) not about NPIs (e.g., only about vaccination); (5) outcomes were not our epidemiological parameters of interest (e.g., only included estimates of cases, deaths). After the initial title and abstract screening, articles were reviewed in full by one reviewer, then approximately 50% underwent a second review by LJF to yield a sample of 151 articles (Figure 1).
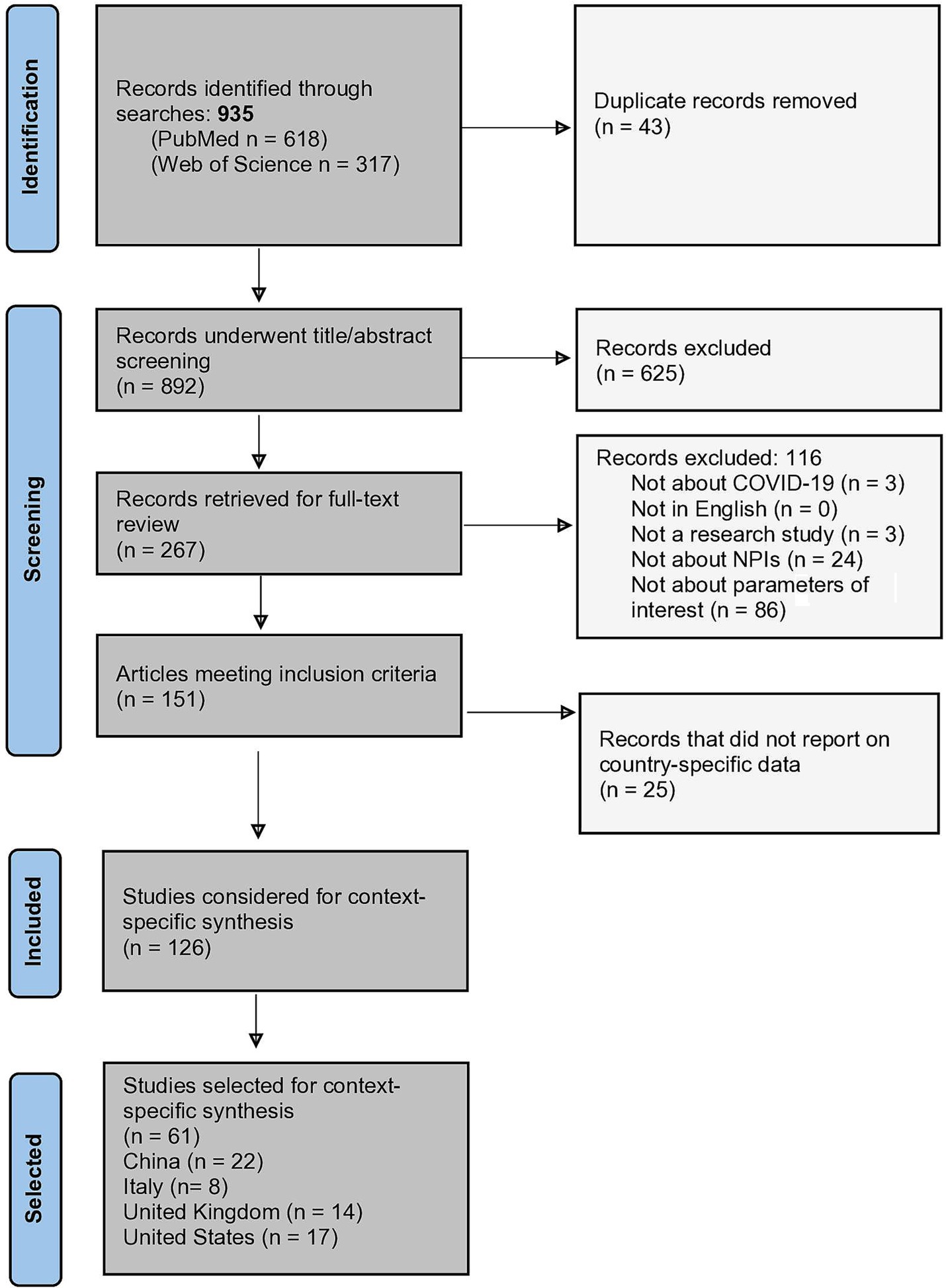
Figure 1. PRISMA flowchart (24).
2.3 Data abstraction
Using a structured Excel form, the study team abstracted standardized information from each article, following an adapted version of the PICOTS framework (16). As articles rarely reported on the populations of interest, the comparators are assumed to be the counterfactual scenario in which NPIs were not implemented, and the outcomes of interest were pre-specified as an inclusion criterion, the remaining relevant elements of PICOTS were (1) intervention(s; NPIs); (2) time frame of the study; and (3) setting in which the research was conducted.
2.4 Selection of contexts
Studies were categorized by country or countries of focus. Hereafter, we use the term “contexts” to indicate either a single country or a group of politically affiliated countries (e.g., the United Kingdom) that are often reported on as a unit. An additional 25 articles that did not present context-specific data were excluded at this step given the study’s emphasis on synthesizing evidence within a specific context, which yielded a sample of 126 included articles (Figure 1). Finally, the study team selected four contexts with enough articles to facilitate a meaningful synthesis, defined as >5 articles: China, Italy, the United Kingdom, and the United States.
2.5 Synthesis of findings
To synthesize this literature, we completed a descriptive summary of the articles that used data from each selected country or context. We attempted to synthesize findings by looking for patterns within and across each country/context. However, this was not possible due to the diversity of methodologic approaches and modeling assumptions and lack of consistency about the populations examined, the interventions themselves, and other dimensions of PICOTS.
2.6 Compiling summary information on COVID-19 disease burden and policy responses
Once our four contexts were selected, we conducted a targeted literature review and web-based scan for country-specific COVID-19 policies in Italy, the United States, the United Kingdom, and China, as well as data on COVID-19 cases and deaths by the end of 2020 (17). Using publicly available data from the University of Oxford’s COVID-19 Government Response Tracker (18), we calculated the median score on the Health and Containment Index (HCI) for each of the four countries for the year 2020. We synthesized this information to provide context for the findings from the literature search.
3 Results
Of the 127 studies included in the review, our final analytic sample consisted of 61 articles, 22 using data from China; 8 using data from Italy; 17 using data from the United States, and 14 using data from the United Kingdom.
3.1 Italy
Italy was the first European country to report a case of COVID-19, which occurred on January 31, 2020 (19). It was also one of the most severely impacted, with 2,141,201 confirmed cases and 74,985 deaths by the end of 2020 (20), corresponding to about 1,247 deaths per million (17). Shortly after Italy’s first COVID-19 case was detected, policymakers instituted a national stay-at-home policy during the months of March and April 2020 (21, 22). This policy was in place during the first wave (February–May 2020). Then, in response to an increase in incidence that signaled the beginning of Italy’s second wave, which lasted from October to December 2020, the government instituted a three-color classification system on November 4 that guided regional approaches to NPIs. Italy’s median HCI score for 2020 was 67, slightly higher than the United States and well above the 75th percentile cutoff for all countries (Figure 2).
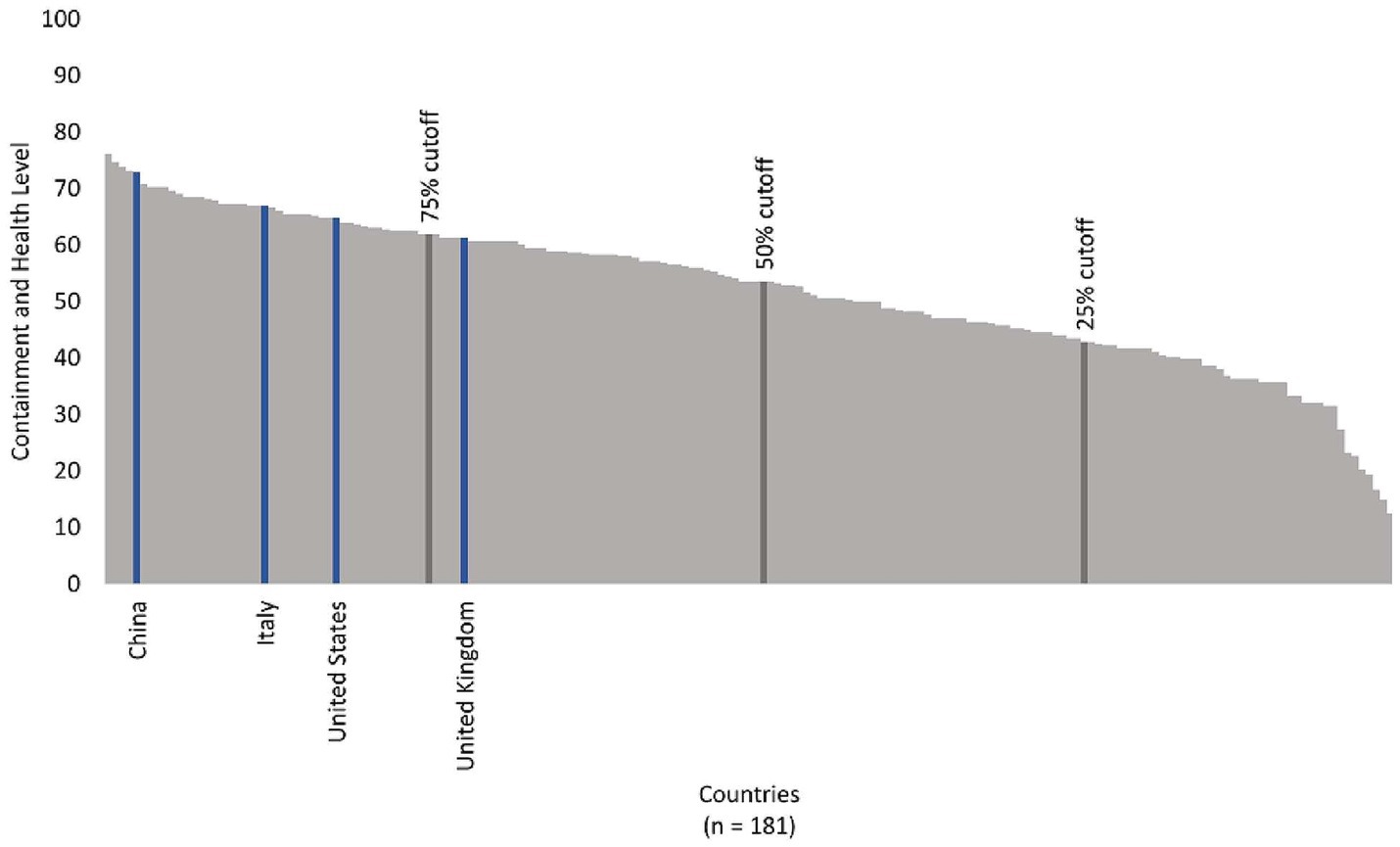
Figure 2. Median country containment and health levels, 2020, for countries with available data, indicating the four selected contexts for this analysis in relation to the 75th percentile cutoff.
We identified eight studies using data from Italy that met our inclusion criteria, six of which exclusively reported data from Italy and two of which were studies of multiple countries that included case data from Italy (Table 1). Two used primary data collected through surveys. The studies reflected interventions, time frame, and setting in the following manner:
• Intervention(s): Four studies examined the effects of social distancing or “lockdowns” on Reff (23–26); and four studies examined multiple NPIs in combination (27–30).
• Time frame: Nearly all studies used February or March 2020 as the start of the study time frame, and five examined data from only a few months (e.g., February to March 2020; or February to May 2020). Two of the eight studies’ data extended into 2021 (28, 30).
• Setting: Studies were primarily conducted at the national level in Italy, with the exceptions of one that focused on Milan (30) and one on Lombardy (24).
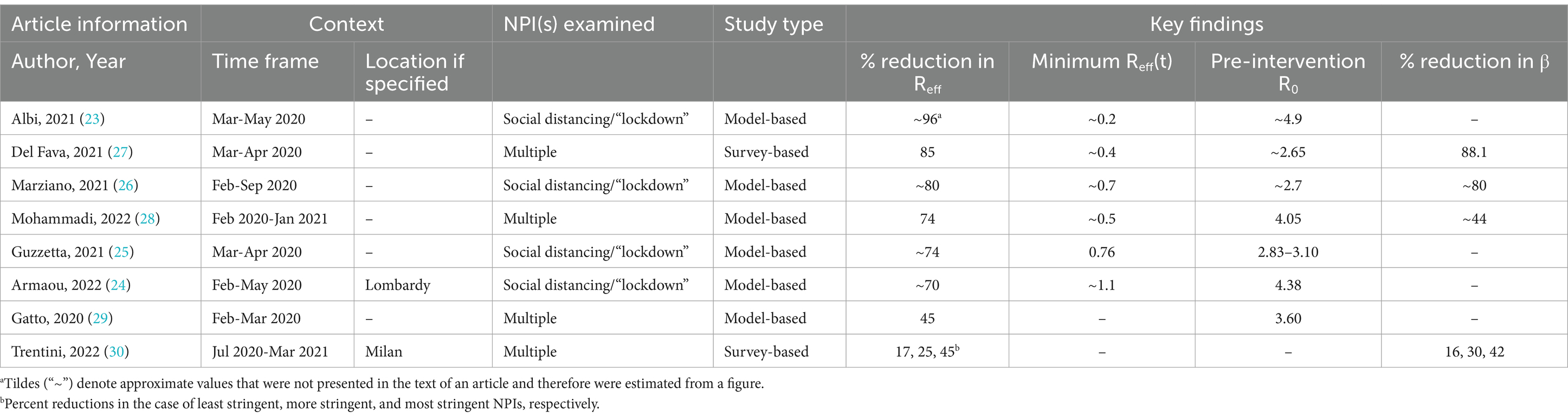
Table 1. Summary of studies of NPI effects on transmission parameters using data from Italy, from largest to smallest percentage reduction in effective reproduction number (N = 8).
Among the eight studies that reported changes in Reff, the estimated percentage reduction in this parameter typically ranged from about 45% (29) to nearly 96 percent (23), with several studies reporting a percent reduction in the 70–80% range. One of these eight studies reported three different percentage reductions in Reff by level of NPI stringency, estimating a 44.7% reduction only with the most stringent set of interventions. When interventions were implemented with medium stringency, the authors estimated a 25.1% reduction in Reff and that percentage was only 17.1% with the least stringent set of NPIs (30) (Table 1).
Four of the eight studies reported percentage reductions in daily contact rates, ranging from about 16 to 88%. One of those studies, (the same analysis that reported different reductions in Reff for three levels of NPI stringency), also reported three different reductions in daily contact rates which were again lower than other studies from Italy, finding that they decreased by 16 with the least stringent suite of NPIs, 30% with an intermediate stringency level, and 42% with the most stringent set (30).
3.2 United States
The first COVID-19 case in the United States was confirmed on January 20, 2020, (31) and by the end of 2020, there were 20,271,441 cases and 362,570 deaths (20), corresponding to about 1,057 deaths per million (17). Like Italy, the United States aimed for suppression of viral transmission (32). States implemented stay-at-home orders, mask mandates, inter-state travel restrictions, and other policies to contain the spread of COVID-19 during March and April 2020. After states began to reopen in May and June 2020, the country experienced a second wave of cases, then saw a third wave in October 2020. The United States’ median HCI score in 2020 was 65 (Figure 2).We identified 17 studies that met our inclusion criteria and used data from the United States, only two of which collected primary data from surveys or passive monitoring (Table 2).
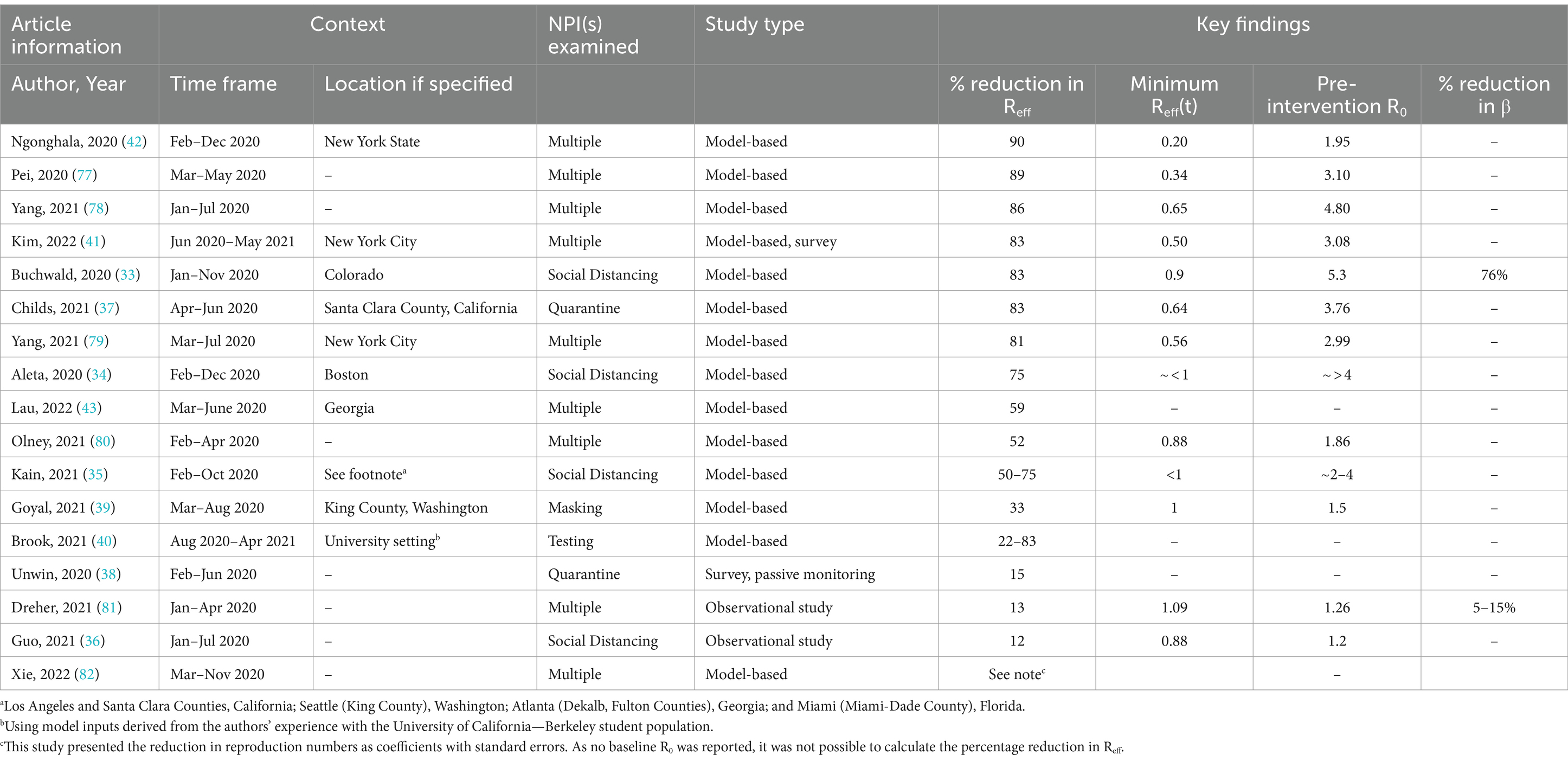
Table 2. Summary of studies of NPI effects on transmission parameters using data from the United States, from largest to smallest percentage reduction in effective reproduction number.
These studies varied by intervention(s) examined, time frame of the study, and setting(s) in which they were conducted, as follows:
• Intervention(s): Nine studies looked at multiple NPIs simultaneously, four studies examined the impact of social distancing on Reff (33–36), two studies focused on quarantine (37, 38), one study focused on the effects of masking (39), and one study explored the impact of testing (40).
• Time frame: Most of the studies used data collected during the first wave of the COVID-19 pandemic in the United States, with 15 of the study time frames beginning in the first 4 months of 2020, most ending in the late summer/early fall 2020, and only two extending into 2021 (40, 41).
• Setting: Three used data from a single state (33, 42, 43), five used data from a single county or city (34, 37, 39, 41), and one study was conducted in a university setting (40). The others used national-level data, included data from multiple cities or counties (35), or did not specify a setting.
These studies using data from the United States found widely varying estimates of our two key outcomes of interest, Reff and daily contact rate. The estimated percentage reduction in Reff ranged from 12% (36) in a study evaluating social distancing to approximately 90% (42) in an analysis of multiple NPIs in combination (Table 2). Overall, studies that found a percent decrease in Reff that was less than 50% tended to be studies of a single NPI such as masking or testing, while those that found greater reductions tended to be studies of multiple NPIs in combination. Otherwise, the variation in assumptions, modeling approaches, and data sources precludes further comparisons of the key findings.
3.3 United Kingdom
The United Kingdom’s first COVID-19 case was reported on January 30, 2020 (44), and by the end of 2020, there were 2,722,251 cases and 95,706 deaths in this country, corresponding to approximately 1,079 deaths per million (17). While the United Kingdom initially took a mitigation approach (i.e., aiming to minimize the pandemic’s impacts on vulnerable groups to avoid overwhelming the health care system while permitting controlled transmission in low-risk groups) (32), it shifted to a suppression strategy in April 2020 as daily incidence rates increased (32). Public health measures were relaxed in June 2020 but were reinstituted in response to a second wave in September 2020. The United Kingdom’s median HCI score in 2020 was 61, putting it just below the 75th percentile cut point for all countries with available data (Figure 2).
We identified a total of 14 studies using data from the United Kingdom that met our inclusion criteria (Table 3). These studies varied across interventions, time frame, and setting as follows:
• Intervention(s): Eight studies examined multiple containment measures in combination, three studies examined “lockdowns” (45, 46), one evaluated the impact of social distancing (47); one evaluated testing, tracing, quarantine, and isolation (48); and one study examined work-from-home policies (49).
• Time frame: The majority of studies (n = 12) were conducted at the peak of the first wave of COVID-19 in 2020. Three were performed during the nascent stages of the pandemic from February to April 2020, preceding the UK government’s shift to a more stringent suppression strategy in April 2020 (47, 50, 51). Only one study extended into 2021 (46), and another extended to February (52).
• Setting: Ten studies looked at the United Kingdom broadly, while four limited their analysis to data from England only (46, 52, 53).
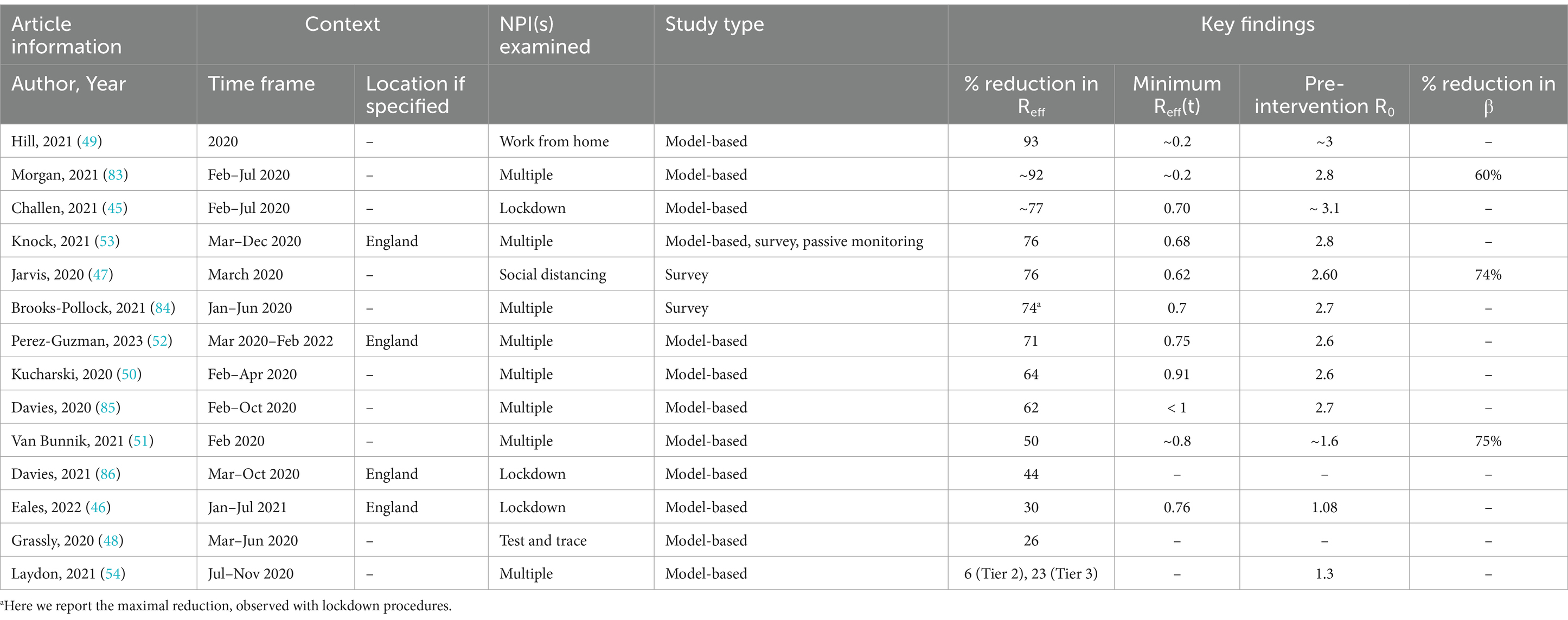
Table 3. Summary of studies of NPI effects on transmission parameters using data from the United Kingdom, from largest to smallest percentage reduction in effective reproduction number.
The reduction in Reff varied substantially across the studies, with estimates ranging from as low as 6% (54) considering tier 2 restrictions to a reduction of approximately 93%, from a simulation study of work from home policies (49) (Table 3). Similar to the studies from the United States, studies from the United Kingdom that reported smaller decreases in Reff typically examined the impact of less stringent measures, either a single NPI or the lowest-tier set of interventions, while those reporting greater Reff reductions generally evaluated more stringent interventions such as “lockdowns” or the combined effect of multiple NPIs.
3.4 China
After Chinese authorities published the sequence of the novel coronavirus, SARS-CoV-2 (55) on January 12, 2020, Chinese health officials instituted one of the strongest policy responses of any country with the goal of completely interrupting transmission, commonly termed a “Zero-COVID” strategy (56). This aggressive containment strategy (32) involved strongly-enforced stay-at-home orders, including through the extensive use of electronic health passes; multiple rounds of mass screening testing for entire cities; contact-tracing involving global positioning system (GPS) technology; and a host of other NPIs (e.g., mask mandates, screening inbound travelers) that were rapidly implemented and sustained throughout the first year of the pandemic (57). By the end of 2020, China had reported 96,972 cases and 4,791 deaths (20), corresponding to 3.2 deaths per million people (17). China’s median HCI score over the course of 2020 was 73, one of the highest of any country with available data, only exceeded by Argentina, Chile, Peru and Oman (Figure 2).
We identified a total of 22 studies using data from China that met our inclusion criteria (Table 4). These studies presented interventions, time frame, and setting in the following manner:
• Intervention(s): Six studies examined social distancing or lockdown measures, one focused on school closure, and the remaining 15 examined the effects of suites of non-pharmaceutical interventions.
• Time frame: Most (N = 16) included studies were centered in 2020. Several used data starting December 2019 (Wuhan-based). Only six study time frames extended beyond 2020 and only one used 2023 data (58).
• Setting: The majority of studies were conducted at the city level. Five analyzed the initial Wuhan outbreak, two focused on Beijing, and others used data from Shanghai (59), and Guangzhou (60). The remainder compared cities across China to one another or used data from Hong Kong.
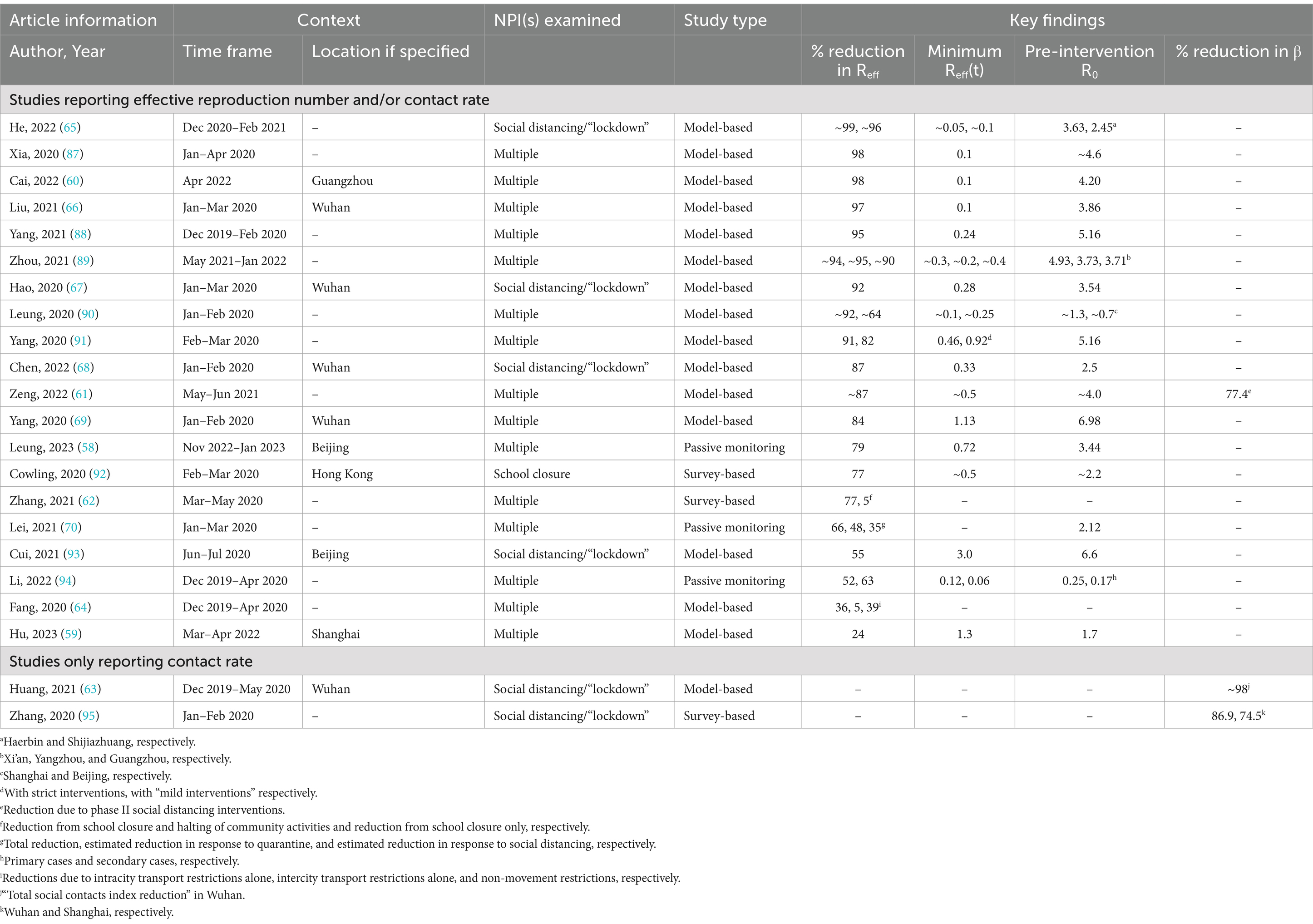
Table 4. Summary of studies of NPI effects on transmission parameters using data from China, from largest to smallest percentage reduction in effective reproduction number (N = 22).
These studies reported a wide range of NPI effectiveness. Only three studies examined changes in daily contact rate; percent reduction ranged from 75 to 98 (61–63) (Table 4). All studies aside from three reported percent reduction in the effective reproductive number (Reff) or pre-intervention Reff and minimum Reff from which percent reduction could be extrapolated. Percent reductions ranged from 5%, in studies that only examined inter-city transport restrictions (64) or school closure (62), to 99% (65), in a study of lockdown. However, most studies reported large reductions in Reff, e.g., 11 studies reported percent reductions of 90% or more. Among all cites examined, NPI effectiveness was highest in Wuhan: 84–97% reductions in Reff (63, 66–69) and a 98% reduction in contact rate (63).
4 Discussion
We conducted a rapid review and narrative synthesis of context-specific evidence to document the magnitude and variation of NPI effectiveness during the pre-vaccine phase of the COVID-19 pandemic. Our analysis of recent published studies from four countries found wide variation and substantial uncertainty around the NPI effectiveness estimates, despite narrowing the focus to two epidemiological parameters rather than outcomes such as cases, hospitalizations, and deaths, and despite examining studies from the same local context. In addition, we found that while many studies of the 61 we examined reported on multiple different NPIs, either individually or in combination, only six studies explicitly used the framing of “stringency” or “mild versus strict” or “tiers” of NPIs, which are concepts that are highly relevant for decisionmakers (30, 54, 62, 64, 70).
Existing literature suggests that NPIs are highly effective at reducing disease transmission. However, studies report a wide range of estimated effectiveness, defined in our study as reductions in the time-varying reproduction number, due primarily to variation in NPI definitions, time frames, and settings. Overall, studies of a single intervention or NPIs that are the least stringent estimated Reff reductions in the 10–50% range, while those that examined “lockdowns,” shorthand for the most stringent social distancing measures or stay-at-home orders, were associated with greater Reff reductions that ranged from 40 to 90%, with many clustered around the 70–80% range.
The challenges we encountered align with those reported by Lison et al., who concluded that a lack of standardized practices for intervention assessment, outcome reporting, and modeling frameworks has limited the utility of existing evidence (4). For example, many of the studies that met our inclusion criteria defined social distancing as broad “lock-downs” while others focused more narrowly on a specific type of physical/social distancing measure, such as working from home. Some examined an NPI in (relative) isolation, like masking or testing-and-tracing, while others took a broader view of suites of NPIs that were implemented concurrently. Studies also varied in the population of interest, including students at a university or school, workers who have the ability to work from home, or the general population. Finally, studies assessed interventions across varying time frames. Data from shorter study periods might capture immediate effects of NPIs but miss long-term trends including sustainability of adherence to those NPIs and effects of their de-implementation. On the other hand, longer time frames allow for data on these trends but results might be difficult to interpret given changing contextual factors over time.
Addressing this lack of standardization would enable the global community to share insights on the relative effectiveness of different, but well-defined NPIs (alone and in combination) across countries where effects are expected to be similar, a key recommendation from a previous report (4). Until better, more standardized data are available, modelers seeking to incorporate NPI effectiveness into their pandemic projections should strive to consider scenarios that reflect different combinations of NPIs, speed, and stringency of their implementation, expected public adherence, and timing of de-implementation. They should also ensure that they are clearly communicating the broad uncertainty these factors create for their modeling parameters.
The results of NPI effectiveness we summarize are not causal estimates, and the wide range of effectiveness estimates within and across countries highlights the degree of uncertainty that is an inherent challenge to this complex work. Yet these results are still useful to inform the debate about the benefits, drawbacks, and cost-effectiveness of NPIs for pandemic response. These relatively large effectiveness estimates reflect an unprecedented collective public health effort to reduce disease transmission during a pandemic and have a clear mechanistic rationale (i.e., less contact with other humans reduces person-to-person transmission of a respiratory pathogen). Hence, these estimates suggest societies can mount a collective effort to reduce disease transmission by at least 40%, and a 70–80% reduction in disease transmission is possible. Whether achieving those reductions is worth the adverse consequences to society including quantifiable and non-quantifiable costs, is a separate but critical question. Existing literature shows the answer is nuanced and depends on, pathogen transmissibility and severity, the anticipated time frame for developing vaccines, and the cost of NPIs relative to disease severity (71, 72). Hence, this study contributes to the literature by documenting the plausible range of one of the inputs for cost-effectiveness analyses of various NPI strategies.
Details around NPI implementation, including how quickly the measures are put into place and their degree of stringency, are key pieces of information that would be highly relevant to modelers and to decision-makers considering these NPI measures. In addition, how well the public adheres to the NPIs once they are implemented varies enormously depending on the local context, and societal trust in institutions is a strong predictor of adherence to public health recommendations (73–75). Yet, these details are mostly missing from the existing evidence base and contribute to both the mathematical uncertainty around the effectiveness estimates as well as policymaker uncertainty about how to interpret these data. Two notable exceptions are a study of mostly middle-to-high-income European countries that found that wealth and demographic structure explain country-level variation in “NPI effects” (defined as relative change in avoided new infections) (76) and the one study in our sample of 61 that estimated different reductions in the specific COVID-19 transmission parameters of interest by NPI stringency. Additional studies examining varying degrees of NPI stringency in countries with different demographic compositions and income levels are urgently needed to inform the debate about the effectiveness of NPIs.
Our findings may be subject to the following limitations: we may have missed relevant studies through our search strategy, inadvertently excluded studies that met our inclusion criteria, or misclassified information abstracted from included studies.
In summary, our work demonstrates the extent of the variation in assessments of NPI effectiveness in the early months of the COVID-19 pandemic and highlights the challenges presented by a lack of standardization in modeling approaches. To improve our global scientific preparedness, (to be ready with pre-positioned research infrastructure and protocols in advance of the next public health emergency), we must collectively address this lack of standardization, as well as other limitations articulated by Lison et al. (4). These include insufficient explanations of modeling decisions, unrealistic assumptions that influence model parameterization, and imprecise definitions of interventions and outcomes. These goals will not be simple to achieve, but they are critically important. Otherwise, when we face the next, inevitable pandemic, or a more transmissible or deadly SARS-CoV-2 variant that evades our existing vaccines, infectious disease modelers and researchers will once again produce siloed results that are not comparable within or across local contexts, and decisionmakers will be again faced with making complex policy choices based on limited evidence.
Data availability statement
The original contributions presented in the study are included in the article/Supplementary material and further inquiries can be directed to the corresponding author.
Author contributions
LF: Conceptualization, Writing – original draft, Writing – review & editing. PL: Writing – review & editing, Conceptualization. JL: Data curation, Formal analysis, Writing – review & editing. DR: Data curation, Formal analysis, Writing – review & editing. SK: Data curation, Formal analysis, Writing – review & editing. EL: Writing – review & editing, Data curation, Methodology. HW: Formal analysis, Funding acquisition, Supervision, Writing – review & editing, Conceptualization.
Funding
The author(s) declare that financial support was received for the research, authorship, and/or publication of this article. This work was made possible by generous gifts from supporters of RAND.
Acknowledgments
The authors would like to express their gratitude to Angela O’Mahony for her guidance and leadership throughout this work as well as Leslie Canterbury for her expert assistance with preparing this manuscript for submission.
Conflict of interest
The authors declare that the research was conducted in the absence of any commercial or financial relationships that could be construed as a potential conflict of interest.
Publisher’s note
All claims expressed in this article are solely those of the authors and do not necessarily represent those of their affiliated organizations, or those of the publisher, the editors and the reviewers. Any product that may be evaluated in this article, or claim that may be made by its manufacturer, is not guaranteed or endorsed by the publisher.
Supplementary material
The Supplementary material for this article can be found online at: https://www.frontiersin.org/articles/10.3389/fpubh.2024.1426992/full#supplementary-material
Abbreviations
COVID-19, coronavirus disease 2019; HCI, Health and Containment Index; ESCI, Emerging Sources Citation Index database; MeSH, medical subject heading; NPI, non-pharmaceutical intervention or non-pharmacological intervention; Reff, effective reproduction number; SCI-EXPANDED, Science Citation Index Expanded database; SSCI, Social Sciences Citation Index database.
References
1. Iezadi, S, Gholipour, K, Azami-Aghdash, S, Ghiasi, A, Rezapour, A, Pourasghari, H, et al. Effectiveness of non-pharmaceutical public health interventions against COVID-19: a systematic review and meta-analysis. PLoS One. (2021) 16:e0260371. doi: 10.1371/journal.pone.0260371
2. Centers for Disease Control and Prevention. Nonpharmaceutical interventions (NPIs). (2022). Available from: https://www.cdc.gov/nonpharmaceutical-interventions/index.html (Accessed June 26, 2023).
3. Zhou, L, Yan, W, Li, S, Yang, H, Zhang, X, Lu, W, et al. Cost-effectiveness of interventions for the prevention and control of COVID-19: systematic review of 85 modelling studies. J Glob Health. (2022) 12:12. doi: 10.7189/jogh.12.05022
4. Lison, A, Banholzer, N, Sharma, M, Mindermann, S, Unwin, HJT, Mishra, S, et al. Effectiveness assessment of non-pharmaceutical interventions: lessons learned from the COVID-19 pandemic. Lancet Public Health. (2023) 8:e311–7. doi: 10.1016/S2468-2667(23)00046-4
5. Abaluck, J, Kwong, LH, Styczynski, A, Haque, A, Kabir, MA, Bates-Jefferys, E, et al. Impact of community masking on COVID-19: a cluster-randomized trial in Bangladesh. Science. (2022) 375:eabi9069. doi: 10.1126/science.abi9069
6. Bundgaard, H, Bundgaard, JS, Raaschou-Pedersen, DET, von Buchwald, C, Todsen, T, Norsk, JB, et al. Effectiveness of adding a mask recommendation to other public health measures to prevent SARS-CoV-2 infection in Danish mask wearers: a randomized controlled trial. Ann Intern Med. (2021) 174:335–43. doi: 10.7326/M20-6817
7. Boulos, L, Curran, JA, Gallant, A, Wong, H, Johnson, C, Delahunty-Pike, A, et al. Effectiveness of face masks for reducing transmission of SARS-CoV-2: a rapid systematic review. Philos Trans R Soc A. (2023) 381:20230133. doi: 10.1098/rsta.2023.0133
8. Grépin, KA, Aston, J, and Burns, J. Effectiveness of international border control measures during the COVID-19 pandemic: a narrative synthesis of published systematic reviews. Philos Trans R Soc A. (2023) 381:20230134. doi: 10.1098/rsta.2023.0134
9. Littlecott, H, Herd, C, O'Rourke, J, Chaparro, LT, Keeling, M, James Rubin, G, et al. Effectiveness of testing, contact tracing and isolation interventions among the general population on reducing transmission of SARS-CoV-2: a systematic review. Philos Trans R Soc A. (2023) 381:20230131. doi: 10.1098/rsta.2023.0131
10. Madhusudanan, A, Iddon, C, Cevik, M, Naismith, JH, and Fitzgerald, S. Non-pharmaceutical interventions for COVID-19: a systematic review on environmental control measures. Philos Trans R Soc A. (2023) 381:20230130. doi: 10.1098/rsta.2023.0130
11. Murphy, C, Lim, WW, Mills, C, Wong, JY, Chen, D, Xie, Y, et al. Effectiveness of social distancing measures and lockdowns for reducing transmission of COVID-19 in non-healthcare, community-based settings. PhilosTrans R Soc A. (2023) 381:20230132. doi: 10.1098/rsta.2023.0132
12. Williams, SN, Dienes, K, Jaheed, J, Wardman, JK, and Petts, J. Effectiveness of communications in enhancing adherence to public health behavioural interventions: a COVID-19 evidence review. Philos Trans R Soc A. (2023) 381:20230129. doi: 10.1098/rsta.2023.0129
13. The Royal Society. COVID-19: Examining the effectiveness of non-pharmaceutical interventions. (2023). The Royal Society Publishing.
14. Banholzer, N, Lison, A, Özcelik, D, Stadler, T, Feuerriegel, S, and Vach, W. The methodologies to assess the effectiveness of non-pharmaceutical interventions during COVID-19: a systematic review. Eur J Epidemiol. (2022) 37:1003–24. doi: 10.1007/s10654-022-00908-y
15. Page, MJ, McKenzie, JE, Bossuyt, PM, Boutron, I, Hoffmann, TC, Mulrow, CD, et al. The PRISMA 2020 statement: an updated guideline for reporting systematic reviews. Int J Surg. (2021) 88:105906. doi: 10.1016/j.ijsu.2021.105906
16. Hartmann, KE, Matchar, DB, and Chang, S. Assessing applicability of medical test studies in systematic reviews In: SM Chang, DB Matchar, and GW Smetana, et al., editors. Methods guide for medical test reviews. Rockville, MD: Agency for Healthcare Research and Quality (US) (2012).
17. Our World in Data. Total confirmed COVID-19 deaths per million: how rapidly are they increasing?. 2020:12–31. Available from: https://ourworldindata.org/grapher/covid-deaths-per-million-vs-days-since-exemplar?endpointsOnly=1&time=earliest (Accessed October 25, 2023). (2020).
18. Hale, T, Angrist, N, Goldszmidt, R, Kira, B, Petherick, A, Phillips, T, et al. A global panel database of pandemic policies (Oxford COVID-19 government response tracker). (2023). Available from: https://www.bsg.ox.ac.uk/research/covid-19-government-response-tracker (Accessed October 25, 2023).
19. Severgnini, CR. Coronavirus, primi due casi in Italia. Corriere Della Sera (in Italian). (2020).
20. World Health Organization. Number of COVID-19 cases reported to WHO. (2020). Available from: https://covid19.who.int/region/euro/country/it.
21. Dorrucci, M, Minelli, G, Boros, S, Manno, V, Prati, S, Battaglini, M, et al. Excess mortality in Italy during the COVID-19 pandemic: assessing the differences between the first and the second wave, year 2020. Front Public Health. (2021) 9:9. doi: 10.3389/fpubh.2021.669209
22. Coronavirus: Italy extends emergency measures nationwide. United Kingdom: BBC News (2020). Available at: https://www.bbc.com/news/world-europe-51810673 (Accessed October 25, 2023).
23. Albi, G, Pareschi, L, and Zanella, M. Modelling lockdown measures in epidemic outbreaks using selective socio-economic containment with uncertainty. Math Biosci Eng. (2021) 18:7161–90. doi: 10.3934/mbe.2021355
24. Armaou, A, Katch, B, Russo, L, and Siettos, C. Designing social distancing policies for the COVID-19 pandemic: a probabilistic model predictive control approach. Math Biosci Eng. (2022) 19:8804–32. doi: 10.3934/mbe.2022409
25. Guzzetta, G, Riccardo, F, Marziano, V, Poletti, P, Trentini, F, Bella, A, et al. Impact of a nationwide lockdown on SARS-CoV-2 transmissibility, Italy. Emerg Infect Dis. (2021) 27:267–70. doi: 10.3201/eid2701.202114
26. Marziano, V, Guzzetta, G, Rondinone, BM, Boccuni, F, Riccardo, F, Bella, A, et al. Retrospective analysis of the Italian exit strategy from COVID-19 lockdown. Proc Natl Acad Sci. (2021) 118:e2019617118. doi: 10.1073/pnas.2019617118
27. Del Fava, E, Cimentada, J, Perrotta, D, Grow, A, Rampazzo, F, Gil-Clavel, S, et al. Differential impact of physical distancing strategies on social contacts relevant for the spread of SARS-CoV-2: evidence from a cross-national online survey, march–April 2020. BMJ Open. (2021) 11:e050651. doi: 10.1136/bmjopen-2021-050651
28. Mohammadi, Z, Cojocaru, MG, and Thommes, EW. Human behaviour, NPI and mobility reduction effects on COVID-19 transmission in different countries of the world. BMC Public Health. (2022) 22:1594. doi: 10.1186/s12889-022-13921-3
29. Gatto, M, Bertuzzo, E, Mari, L, Miccoli, S, Carraro, L, Casagrandi, R, et al. Spread and dynamics of the COVID-19 epidemic in Italy: effects of emergency containment measures. PNAS. (2020) 117:10484–91. doi: 10.1073/pnas.2004978117
30. Trentini, F, Manna, A, Balbo, N, Marziano, V, Guzzetta, G, O’Dell, S, et al. Investigating the relationship between interventions, contact patterns, and SARS-CoV-2 transmissibility. Epidemics. (2022) 40:100601. doi: 10.1016/j.epidem.2022.100601
31. Holshue, ML, DeBolt, C, Lindquist, S, Lofy, KH, Wiesman, J, Bruce, H, et al. First case of 2019 novel coronavirus in the United States. N Engl J Med. (2020) 382:929–36. doi: 10.1056/NEJMoa2001191
32. Wu, S, Neill, R, De Foo, C, Chua, AQ, Jung, A-S, Haldane, V, et al. Aggressive containment, suppression, and mitigation of covid-19: lessons learnt from eight countries. BMJ. (2021) 375:e067508. doi: 10.1136/bmj-2021-067508
33. Buchwald, AG, Bayham, J, Adams, J, Bortz, D, Colborn, K, Zarella, O, et al. Estimating the impact of statewide policies to reduce spread of severe acute respiratory syndrome coronavirus 2 in real time, Colorado, Usa. Emerg Infect Dis. (2021) 27:2312–22. doi: 10.3201/eid2709.204167
34. Aleta, A, Martín-Corral, D, Pastore y Piontti, A, Ajelli, M, Litvinova, M, Chinazzi, M, et al. Modelling the impact of testing, contact tracing and household quarantine on second waves of COVID-19. Nat. Nat Hum Behav. (2020) 4:964–71. doi: 10.1038/s41562-020-0931-9
35. Kain, MP, Childs, ML, Becker, AD, and Mordecai, EA. Chopping the tail: how preventing superspreading can help to maintain COVID-19 control. Epidemics. (2021) 34:100430. doi: 10.1016/j.epidem.2020.100430
36. Guo, C, Chan, SHT, Lin, C, Zeng, Y, Bo, Y, Zhang, Y, et al. Physical distancing implementation, ambient temperature and Covid-19 containment: an observational study in the United States. Sci Total Environ. (2021) 789:147876. doi: 10.1016/j.scitotenv.2021.147876
37. Childs, ML, Kain, MP, Harris, MJ, Kirk, D, Couper, L, Nova, N, et al. The impact of long-term non-pharmaceutical interventions on COVID-19 epidemic dynamics and control: the value and limitations of early models. Proc R Soc B. (1957) 288:20210811. doi: 10.1098/rspb.2021.0811
38. Unwin, HJT, Mishra, S, Bradley, VC, Gandy, A, Mellan, TA, Coupland, H, et al. State-level tracking of COVID-19 in the United States. Nat Commun. (2020) 11:6189. doi: 10.1038/s41467-020-19652-6
39. Goyal, A, Reeves, DB, Thakkar, N, Famulare, M, Cardozo-Ojeda, EF, Mayer, BT, et al. Slight reduction in SARS-CoV-2 exposure viral load due to masking results in a significant reduction in transmission with widespread implementation. Sci Rep. (2021) 11:11838. doi: 10.1038/s41598-021-91338-5
40. Brook, CE, Northrup, GR, Ehrenberg, AJ, Doudna, JA, and Boots, MConsortium IS-C-T. Optimizing COVID-19 control with asymptomatic surveillance testing in a university environment. Epidemics. (2021) 37:100527. doi: 10.1016/j.epidem.2021.100527
41. Kim, H-Y, Bershteyn, A, McGillen, JB, Shaff, J, Sisti, J, Ko, C, et al. Social distancing and mask-wearing could avoid recurrent stay-at-home restrictions during COVID-19 respiratory pandemic in new York City. Sci Rep. (2022) 12:10312. doi: 10.1038/s41598-022-13310-1
42. Ngonghala, CN, Iboi, E, Eikenberry, S, Scotch, M, MacIntyre, CR, Bonds, MH, et al. Mathematical assessment of the impact of non-pharmaceutical interventions on curtailing the 2019 novel coronavirus. Math Biosci. (2020) 325:108364. doi: 10.1016/j.mbs.2020.108364
43. Lau, MS, Liu, C, Siegler, AJ, Sullivan, PS, Waller, LA, Shioda, K, et al. Post-lockdown changes of age-specific susceptibility and its correlation with adherence to social distancing measures. Sci Rep. (2022) 12:4637. doi: 10.1038/s41598-022-08566-6
44. Lillie, PJ, Samson, A, Li, A, Adams, K, Capstick, R, Barlow, GD, et al. Novel coronavirus disease (Covid-19): the first two patients in the UK with person to person transmission. J Inf Secur. (2020) 80:578–606. doi: 10.1016/j.jinf.2020.02.020
45. Challen, R, Tsaneva-Atanasova, K, Pitt, M, Edwards, T, Gompels, L, Lacasa, L, et al. Estimates of regional infectivity of COVID-19 in the United Kingdom following imposition of social distancing measures. Philos Trans R Soc B. (1829) 376:20200280. doi: 10.1098/rstb.2020.0280
46. Eales, O, Wang, H, Haw, D, Ainslie, KE, Walters, CE, Atchison, C, et al. Trends in SARS-CoV-2 infection prevalence during England’s roadmap out of lockdown, January to July 2021. PLoS Comput Biol. (2022) 18:e1010724. doi: 10.1371/journal.pcbi.1010724
47. Jarvis, CI, Van Zandvoort, K, Gimma, A, Prem, K, Klepac, P, Rubin, GJ, et al. Quantifying the impact of physical distance measures on the transmission of COVID-19 in the UK. BMC Med. (2020) 18:1–10. doi: 10.1186/s12916-020-01597-8
48. Grassly, NC, Pons-Salort, M, Parker, EP, White, PJ, Ferguson, NM, Ainslie, K, et al. Comparison of molecular testing strategies for COVID-19 control: a mathematical modelling study. Lancet Infect Dis. (2020) 20:1381–9. doi: 10.1016/S1473-3099(20)30630-7
49. Hill, EM, Atkins, BD, Keeling, MJ, Dyson, L, and Tildesley, MJ. A network modelling approach to assess non-pharmaceutical disease controls in a worker population: an application to SARS-CoV-2. PLoS Comput Biol. (2021) 17:e1009058. doi: 10.1371/journal.pcbi.1009058
50. Kucharski, AJ, Klepac, P, Conlan, AJ, Kissler, SM, Tang, ML, Fry, H, et al. Effectiveness of isolation, testing, contact tracing, and physical distancing on reducing transmission of SARS-CoV-2 in different settings: a mathematical modelling study. Lancet Infect Dis. (2020) 20:1151–60. doi: 10.1016/S1473-3099(20)30457-6
51. Van Bunnik, BA, Morgan, AL, Bessell, PR, Calder-Gerver, G, Zhang, F, Haynes, S, et al. Segmentation and shielding of the most vulnerable members of the population as elements of an exit strategy from COVID-19 lockdown. Philos Trans R Soc B. (1829) 376:20200275. doi: 10.1098/rstb.2020.0275
52. Perez-Guzman, PN, Knock, E, Imai, N, Rawson, T, Elmaci, Y, Alcada, J, et al. Epidemiological drivers of transmissibility and severity of SARS-CoV-2 in England. Nat Commun. (2023) 14:8099. doi: 10.1038/s41467-023-44062-9
53. Knock, ES, Whittles, LK, Lees, JA, Perez-Guzman, PN, Verity, R, FitzJohn, RG, et al. Key epidemiological drivers and impact of interventions in the 2020 SARS-CoV-2 epidemic in England. Sci Transl Med. (2021) 13:eabg4262. doi: 10.1126/scitranslmed.abg4262
54. Laydon, DJ, Mishra, S, Hinsley, WR, Samartsidis, P, Flaxman, S, Gandy, A, et al. Modelling the impact of the tier system on SARS-CoV-2 transmission in the UK between the first and second national lockdowns. BMJ Open. (2021) 11:e050346. doi: 10.1136/bmjopen-2021-050346
55. European Centre for Disease Prevention and Control. Outbreak of acute respiratory syndrome associated with a novel coronavirus, Wuhan, China, vol. 9 (2020). 11 p. The Royal Society Publishing.
56. Lai, S, Ruktanonchai, NW, Zhou, L, Prosper, O, Luo, W, Floyd, JR, et al. Effect of non-pharmaceutical interventions to contain COVID-19 in China. Nature. (2020) 585:410–3. doi: 10.1038/s41586-020-2293-x
57. Chen, Q, Rodewald, L, Lai, S, and Gao, GF. Rapid and sustained containment of covid-19 is achievable and worthwhile: implications for pandemic response. BMJ. (2021) 375:e066169. doi: 10.1136/BMJ-2021-066169
58. Leung, K, Lau, EH, Wong, CK, Leung, GM, and Wu, JT. Estimating the transmission dynamics of SARS-CoV-2 omicron BF. 7 in Beijing after adjustment of the zero-COVID policy in November–December 2022. Nat Med. (2023) 29:579–82. doi: 10.1038/s41591-023-02212-y
59. Hu, L, Shi, M, Li, M, and Ma, J. The effectiveness of control measures during the 2022 COVID-19 outbreak in Shanghai, China. PLoS One. (2023) 18:e0285937. doi: 10.1371/journal.pone.0285937
60. Cai, W, Yang, Z, Liang, J, Lin, Z, Ma, Y, Chen, C, et al. How fast and how well the omicron epidemic was curtailed. A Guangzhou experience to share. Front Public Health. (2022) 10:979063. doi: 10.3389/fpubh.2022.979063
61. Zeng, Z, Wu, T, Lin, Z, Luo, L, Lin, Z, Guan, W, et al. Containment of SARS-CoV-2 Delta strain in Guangzhou, China by quarantine and social distancing: a modelling study. Sci Rep. (2022) 12:21096. doi: 10.1038/s41598-022-21674-7
62. Zhang, J, Litvinova, M, Liang, Y, Zheng, W, Shi, H, Vespignani, A, et al. The impact of relaxing interventions on human contact patterns and SARS-CoV-2 transmission in China. Sci Adv. (2021) 7:eabe2584. doi: 10.1126/sciadv.abe2584
63. Huang, B, Wang, J, Cai, J, Yao, S, Chan, PKS, Tam, TH, et al. Integrated vaccination and physical distancing interventions to prevent future COVID-19 waves in Chinese cities. Nat Hum Behav. (2021) 5:695–705. doi: 10.1038/s41562-021-01063-2
64. Fang, L-Q, Zhang, H-Y, Zhao, H, Che, T-L, Zhang, A-R, Liu, M-J, et al. Meteorological conditions and nonpharmaceutical interventions jointly determined local transmissibility of COVID-19 in 41 Chinese cities: a retrospective observational study. Lancet Reg Health West Pac. (2020) 2:100020. doi: 10.1016/j.lanwpc.2020.100020
65. He, Y, Chen, Y, Yang, L, Zhou, Y, Ye, R, and Wang, X. The impact of multi-level interventions on the second-wave SARS-CoV-2 transmission in China. PLoS One. (2022) 17:e0274590. doi: 10.1371/journal.pone.0274590
66. Liu, W, Wang, D, Hua, S, Xie, C, Wang, B, Qiu, W, et al. Spatiotemporal analysis of COVID-19 outbreaks in Wuhan, China. Sci Rep. (2021) 11:13648. doi: 10.1038/s41598-021-93020-2
67. Hao, X, Cheng, S, Wu, D, Wu, T, Lin, X, and Wang, C. Reconstruction of the full transmission dynamics of COVID-19 in Wuhan. Nature. (2020) 584:420–4. doi: 10.1038/s41586-020-2554-8
68. Chen, Y, Zhang, H, Wang, J, Li, C, Yi, N, and Wen, Y. Investigation on the control of COVID-19 in Wuhan: number of infections outside hospitals and the reproduction number. Disaster Med Public Health Prep. (2023) 17:e240. doi: 10.1017/dmp.2022.161
69. Yang, T, Liu, Y, Deng, W, Zhao, W, and Deng, J. SARS-Cov-2 trajectory predictions and scenario simulations from a global perspective: a modelling study. Sci Rep. (2020) 10:18319. doi: 10.1038/s41598-020-75332-x
70. Lei, H, Wu, X, Wang, X, Xu, M, Xie, Y, Du, X, et al. Different transmission dynamics of coronavirus disease 2019 (COVID-19) and influenza suggest the relative efficiency of isolation/quarantine and social distancing against COVID-19 in China. Clin Infect Dis. (2021) 73:e4305–11. doi: 10.1093/cid/ciaa1584
71. Nowak, SA, Nascimento de Lima, P, and Vardavas, R. Optimal non-pharmaceutical pandemic response strategies depend critically on time horizons and costs. Sci Rep. (2023) 13:2416. doi: 10.1038/s41598-023-28936-y
72. Nowak, SA, Nascimento De Lima, P, and Vardavas, R. The cost of myopic pandemic response. medRxiv. (2024) 1–14. doi: 10.1101/2024.02.19.24303020
73. Choi, Y, and Fox, AM. Mistrust in public health institutions is a stronger predictor of vaccine hesitancy and uptake than Trust in Trump. Soc Sci Med. (2022) 314:115440. doi: 10.1016/j.socscimed.2022.115440
74. Majid, U, Wasim, A, Truong, J, and Bakshi, S. Public trust in governments, health care providers, and the media during pandemics: a systematic review. J Trust Res. (2021) 11:119–41. doi: 10.1080/21515581.2022.2029742
75. Silver, D, Kim, Y, McNeill, E, Piltch-Loeb, R, Wang, V, and Abramson, D. Association between COVID-19 vaccine hesitancy and trust in the medical profession and public health officials. Prev Med. (2022) 164:107311. doi: 10.1016/j.ypmed.2022.107311
76. Banholzer, N, Feuerriegel, S, and Vach, W. Estimating and explaining cross-country variation in the effectiveness of non-pharmaceutical interventions during COVID-19. Sci Rep. (2022) 12:7526. doi: 10.1038/s41598-022-11362-x
77. Pei, S, Kandula, S, and Shaman, J. Differential effects of intervention timing on COVID-19 spread in the United States. Science. Sci Adv. (2020) 6:eabd6370. doi: 10.1126/sciadv.abd6370
78. Yang, B, Huang, AT, Garcia-Carreras, B, Hart, WE, Staid, A, Hitchings, MD, et al. Effect of specific non-pharmaceutical intervention policies on SARS-CoV-2 transmission in the counties of the United States. Nat Commun. (2021) 12:3560. doi: 10.1038/s41467-021-23865-8
79. Yang, W, Shaff, J, and Shaman, J. Effectiveness of non-pharmaceutical interventions to contain COVID-19: a case study of the 2020 spring pandemic wave in new York City. J R Soc Interface. (2021) 18:20200822. doi: 10.1098/rsif.2020.0822
80. Olney, AM, Smith, J, Sen, S, Thomas, F, and Unwin, HJT. Estimating the effect of social distancing interventions on COVID-19 in the United States. Am J Epidemiol. (2021) 190:1504–9. doi: 10.1093/aje/kwaa293
81. Dreher, N, Spiera, Z, McAuley, FM, Kuohn, L, Durbin, JR, Marayati, NF, et al. Policy interventions, social distancing, and SARS-CoV-2 transmission in the United States: a retrospective state-level analysis. Am J Med Sci. (2021) 361:575–84. doi: 10.1016/j.amjms.2021.01.007
82. Xie, S, Wang, W, Wang, Q, Wang, Y, and Zeng, D. Evaluating effectiveness of public health intervention strategies for mitigating COVID-19 pandemic. Stat Med. (2022) 41:3820–36. doi: 10.1002/sim.9482
83. Morgan, AL, Woolhouse, ME, Medley, GF, and van Bunnik, BA. Optimizing time-limited non-pharmaceutical interventions for COVID-19 outbreak control. Philos Trans R Soc B. (1829) 376:20200282. doi: 10.1098/rstb.2020.0282
84. Brooks-Pollock, E, Read, JM, McLean, AR, Keeling, MJ, and Danon, L. Mapping social distancing measures to the reproduction number for COVID-19. Philos Trans R Soc B. (1829) 376:20200276. doi: 10.1098/rstb.2020.0276
85. Davies, NG, Kucharski, AJ, Eggo, RM, Gimma, A, Edmunds, WJ, Jombart, T, et al. Effects of non-pharmaceutical interventions on COVID-19 cases, deaths, and demand for hospital services in the UK: a modelling study. Lancet Public Health. (2020) 5:e375–85. doi: 10.1016/S2468-2667(20)30133-X
86. Davies, NG, Barnard, RC, Jarvis, CI, Russell, TW, Semple, MG, Jit, M, et al. Association of tiered restrictions and a second lockdown with COVID-19 deaths and hospital admissions in England: a modelling study. Lancet Infect Dis. (2021) 21:482–92. doi: 10.1016/S1473-3099(20)30984-1
87. Xia, F, Xiao, Y, Liu, P, Cheke, RA, and Li, X. Differences in how interventions coupled with effective reproduction numbers account for marked variations in COVID-19 epidemic outcomes. Math Biosci Eng. (2020) 17:5085–98. doi: 10.3934/mbe.2020274
88. Yang, S, Liu, Y, Chen, K, Li, T, Huang, Y, Chen, X, et al. A dynamic model of the coronavirus disease 2019 outbreak to analyze the effectiveness of control measures. Medicine. (2021) 100:e23925. doi: 10.1097/MD.0000000000023925
89. Zhou, W, Bai, Y, and Tang, S. The effectiveness of various control strategies: an insight from a comparison modelling study. J Theor Biol. (2022) 549:111205. doi: 10.1016/j.jtbi.2022.111205
90. Leung, K, Wu, JT, Liu, D, and Leung, GM. First-wave COVID-19 transmissibility and severity in China outside Hubei after control measures, and second-wave scenario planning: a modelling impact assessment. Lancet. (2020) 395:1382–93. doi: 10.1016/S0140-6736(20)30746-7
91. Yang, J, Wang, G, and Zhang, S. Impact of household quarantine on SARS-Cov-2 infection in mainland China: a mean-field modelling approach. Math Biosci Eng. (2020) 17:4500–12. doi: 10.3934/mbe.2020248
92. Cowling, BJ, Ali, ST, Ng, TW, Tsang, TK, Li, JC, Fong, MW, et al. Impact assessment of non-pharmaceutical interventions against coronavirus disease 2019 and influenza in Hong Kong: an observational study. Lancet Public Health. (2020) 5:e279–88. doi: 10.1016/S2468-2667(20)30090-6
93. Cui, X, Zhao, L, Zhou, Y, Lin, X, Ye, R, Ma, K, et al. Transmission dynamics and the effects of non-pharmaceutical interventions in the COVID-19 outbreak resurged in Beijing, China: a descriptive and modelling study. BMJ Open. (2021) 11:e047227. doi: 10.1136/bmjopen-2020-047227
94. Li, F, Li, Y-Y, Liu, M-J, Fang, L-Q, Dean, NE, Wong, GW, et al. Household transmission of SARS-CoV-2 and risk factors for susceptibility and infectivity in Wuhan: a retrospective observational study. Lancet Infect Dis. (2021) 21:617–28. doi: 10.1016/S1473-3099(20)30981-6
Keywords: COVID-19, non-pharmaceutical interventions, effective reproduction number, contact rate, disease transmission, infectious disease modeling
Citation: Faherty LJ, Nascimento de Lima P, Lim JZ, Roberts D, Karr S, Lawson E and Willis HH (2024) Effects of non-pharmaceutical interventions on COVID-19 transmission: rapid review of evidence from Italy, the United States, the United Kingdom, and China. Front. Public Health. 12:1426992. doi: 10.3389/fpubh.2024.1426992
Edited by:
Sarafa Iyaniwura, Los Alamos National Laboratory (DOE), United StatesReviewed by:
Yao Li, Johns Hopkins University, United StatesNicola Luigi Bragazzi, University of Parma, Italy
Copyright © 2024 Faherty, Nascimento de Lima, Lim, Roberts, Karr, Lawson and Willis. This is an open-access article distributed under the terms of the Creative Commons Attribution License (CC BY). The use, distribution or reproduction in other forums is permitted, provided the original author(s) and the copyright owner(s) are credited and that the original publication in this journal is cited, in accordance with accepted academic practice. No use, distribution or reproduction is permitted which does not comply with these terms.
*Correspondence: Laura J. Faherty, bGZhaGVydHlAcmFuZC5vcmc=