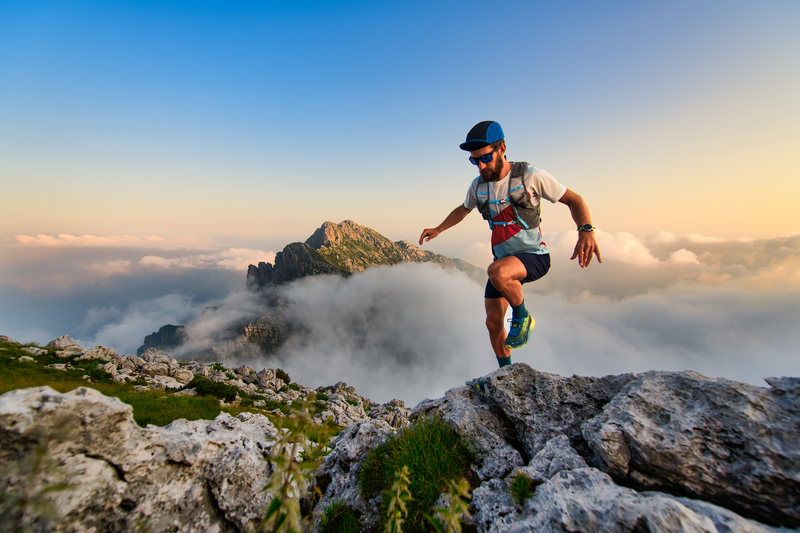
95% of researchers rate our articles as excellent or good
Learn more about the work of our research integrity team to safeguard the quality of each article we publish.
Find out more
ORIGINAL RESEARCH article
Front. Public Health , 16 May 2024
Sec. Public Health Policy
Volume 12 - 2024 | https://doi.org/10.3389/fpubh.2024.1403320
Introduction: Medicine innovation is crucial in promoting the sustainable development of medicine undertakings, which has significant economic and social benefits. China is the main force in global medicine consumption, with a huge demand for innovative medicines. Thus, the Chinese government releases a series of policies aimed at providing scientific and reasonable guidance for medicine innovation. However, there is inadequate quantitative evaluation and comparison of various medicine innovation policies in the existing studies.
Methods: This paper adopts the approach of text mining and the Policy Modeling Consistency Index (PMC-Index) model to construct an evaluation system and then quantitatively evaluates and compares the traditional Chinese medicine innovation policies (TCMIPs), the biological medicine innovation policies (BMIPs), and the multiple medicine innovation policies (MMIPs) in China.
Results: The results indicate that: (1) The three types of drug innovation policies have similarities in content and goal through comparative analysis of high-frequency words, while they also have their own characteristics. (2) The average PMC-Index of 29 TCMIPs is 5.77, which has the highest policy bad rate (21%); the average PMC-Index of 12 BMIPs is 6.21, which has the highest policy good rate (92%); moreover, the average PMC-Index of 35 MMIPs is 6.06, which has the highest policy excellence rate (26%). (3) The BMIPs, MMIPs, and TCMIPs have similar scores on policy object, policy orientation, policy timeliness, policy evaluation, and policy accessibility, while they differ significantly mainly on policy nature, incentive method, policy function, policy issuing agency, and policy instrument.
Discussion: This study contributes to a comprehensive understanding of medicine innovation policies in China, in order to provide theoretical support for future policy formulation and optimization in the medicine industry. Moreover, we expand the application scenarios of policy diffusion theory.
Medicine plays an important role in safeguarding human health and promoting medical progress (1). Innovative medicine is the frontier force of the pharmaceutical industry, which has huge social and economic benefits (2). Cancer and autoimmune diseases have become major health challenges globally, while innovative medicines can improve the cure rate of these difficult diseases (3). In 2022, the global market size of innovative medicines reached 1,027 billion dollars, accounting for approximately 69.5% of the global pharmaceutical market. However, the scale of the innovative medicine market in China is only 142 billion dollars in 2022. Moreover, due to a lack of competition among similar high-quality medicines, the prices of imported innovative medicines have remained high for a long time in China. Before the domestic programmed death-1 was available, patients spent about 70,000 to 80,000 dollars annually on imported innovative medicines such as Keytruda and Opdivo (4). However, the treatment cost for patients decreases to less than 7,000 dollars when domestic innovative medicines launch. Domestic innovative medicines are gradually replacing imported medicines, which contributes to controlling health insurance expenditures and reducing the burden on patients. Furthermore, China is aging much faster than the global average. The proportion of older adult people aged 65 years and older in China doubled to 14.2% from 2000 to 2021 (5). More and more older adult people mean a growing market space for the innovative medicine industry, and the demand for innovative medicines will continue to increase in the future. How to stimulate medicine innovation in China is significant and urgent, so the Chinese government has formulated a large number of medicine innovation policies.
These medicine innovation policies include development plans, guidelines, and implementation opinions to support and encourage the development of the medicine industry. This indicates that the government is highly concerned about public health (6, 7). Reviewing the medicine innovation policies in China, what are the similarities and differences among these policy texts? What are the overall quality and individual characteristics of the medicine innovation policy in China? How can we identify the strengths and weaknesses of medicine innovation policy design and provide targeted improvement strategies? Academics have yet to answer these questions. Thus, the motivation of this study is to assess medicine innovation policy in China, answer the above research questions, and help policymakers improve the medicine innovation system to promote medicine innovation development. However, the medicine innovation policy in China lacks a comprehensive and scientific method for evaluating the advantages and disadvantages of policies. There are various methods for policy evaluation (8–10), but the more cutting-edge at present is the PMC-Index model (11). The PMC-Index is a policy evaluation methodology proposed by Estrada in 2011 that assesses the internal consistency of policies in several dimensions and identifies the advantages and disadvantages of each policy (12). This paper attempts to use the PMC-Index method to quantitatively assess the consistency level of medicine innovation policies in China.
This study attempts to fill the gaps in the existing literature, and our major contributions are as follows: (1) There is insufficient comparative analysis of medicine policies in the existing literature, with some studies focusing on a single industry (13). However, this study conducts comparative analysis and selects comprehensive samples, including the traditional Chinese medicine innovation policies (TCMIPs), the biological medicine innovation policies (BMIPs), and the multiple medicine innovation policies (MMIPs) issued by the Chinese government; MMIPs cover the innovation of traditional Chinese medicine, biological medicine, and chemical medicine at the same time. Since the retrieved policies relate to the innovation of chemical medicines are technical guidelines without specific planning content, our study excludes policies that relate to chemical medicine innovation in the analysis. (2) Most of the existing literature is about the macro-evaluation of the implementation effect of medicine innovation policies, as it is the endpoint of policy evaluation (14–16), but neglects the analysis of policy content (17–19). However, text mining technology is adopted in this study to dig deeply into the policy texts of TCMIPs, BMIPs, and MMIPs so as to identify the basic elements and the internal logic of various medicine innovation policies. Moreover, this paper constructs the PMC-Index model to quantitatively evaluate and compare the TCMIPs, BMIPs, and MMIPs, respectively, which provides theoretical support for future policy formulation in the medicine industry. The research framework is illustrated in Figure 1.
The rest of this study is organized as follows: Section 2 reviews the literature on medicine innovation and policy evaluation, and identifies the shortcomings in existing research. Section 3 describes the research design, including sample selection and methodology. Section 4 reports the quantitative analysis results of TCMIPs, BMIPs, and MMIPs and proceeds with a comparative analysis and discussion of various policies. Section 5 summarizes this study and elaborates on the limitations.
To promote better practice of medicine innovation policies, scholars have studied these policies from both quantitative and qualitative perspectives. The quantitative research regarding medicine innovation policies mainly focuses on their implementation effects evaluation, while the qualitative research regarding these policies chiefly concentrates on their policy content evaluation. Compared with the qualitative research, the quantitative research on medicine innovation policies is more abundant. That is, most existing literature focuses on the implementation effectiveness of medicine innovation policies. For example, Bouet (20) utilized probit and logit techniques to evaluate the effect of TRIPs on Indian pharmaceutical industry innovation. Gamba (21) employed the zero-inflated negative binomial model to analyze the medicine intellectual property protection reform policies in developed and developing countries and believed that medicine innovation is highly sensitive to intellectual property protection. Aghmiuni et al. (22) discussed the supportive innovation policies in biological medicine and found that these policies have a significant impact on the development of biological medicine innovation. Moreover, existing studies also indicate that some medicine innovation policies in China contribute to innovation quality. For example, Liu et al. (23) applied the difference-in-differences model to find that the generic consistency evaluation policy has a positive impact on the innovation quality of the Chinese pharmaceutical industry. Gu et al. (24) adopted listed medicine companies in the Shanghai and Shenzhen A-share markets as samples and empirically found that centralized drug procurement policy has a significant improvement on the quality of medicine innovation. In addition, the qualitative evaluation of medicine innovation policies in existing research mainly concentrates on the specific interpretation of policy content. Doran et al. (25) reviewed the reforms of medicine innovation policies in Australia and elaborated on the impact of price control on innovation. Karampli et al. (26) outlined the study findings on the impact of medicine innovation on medicine expenditure growth and described the challenges faced by Greek drug innovation policies. Liu et al. (27) described the medicine innovation policies to accelerate medicine review and approval in China, stating that the development of innovative medicine benefits from these accelerated policies. Overall, the above literature helps researchers understand medicine innovation policies from different perspectives and provides insights for policy optimization. However, these studies have mainly examined medicine innovation policies at the macro level, lacking a systematic evaluation of medicine innovation policies in China. Thus, research on medicine innovation policies in China has yet to be expanded.
Policy evaluation is a complex and systematic program that plays a crucial role in guiding policy formulation and optimization (28–30). Choosing appropriate and scientific evaluation methods is the foundation of policy evaluation. The existing studies have proposed a variety of methods to evaluate policies, such as the five kinds of evaluation tools (31), the “3e “assessment framework (32), the index of legal changes (33), hierarchical analysis (34), Delphi method (35), content analysis method (36), and difference in difference analysis (37–39). As previously stated, the five kinds of evaluation tools, the “3e “assessment framework, and the index of legal changes are relatively outdated and one-sided in their assessment of policy. Moreover, hierarchical analysis, the Delphi method, and the content analysis method have more subjective evaluation processes (40). Furthermore, the difference in difference analysis focuses on evaluating the implementation effect of a certain policy (41–43), lacking systematic evaluation of a series of policies (44). These above policy evaluation methods are widely adopted, but they fall short in terms of objectivity and accuracy. In addition, these policy evaluation methods pay less attention to individual differences and the texts of policies. However, the PMC-Index model combines qualitative and quantitative approaches in a more comprehensive and objective way than the above methods, which are widely used to evaluate policies. It can provide an overall evaluation of policy consistency as well as systematically analyze individual policy differences from various dimensions. In previous literature, research on the PMC-Index model has been on the rise, and many satisfactory results have been achieved. For example, Liu et al. (45) utilized the PMC-Index model to discuss the power battery recycling policies of the central and local governments and found that the policymaking ability of the central government is stronger. Fan et al. (46) employed the PMC-Index model to investigate China’s municipal solid waste policies and identified that these policies are generally reasonable. Zhao et al. (47) utilized the PMC-Index model to explore energy security in China and believed that the administrative level of the issuing agency positively affects the PMC-Index. In addition, many studies have used this model for policy evaluation, including fire safety education policy (48), traditional Chinese medicine development policy (13), new energy vehicle policy (49, 50), and internet healthcare policy (51). These studies reflect that the PMC-Index model has good applicability for opening up the black box of policy formulation and promoting policy evaluation.
Overall, there is extensive literature on medicine innovation and policy evaluation, while the existing research still has some shortcomings. First, there is much literature on the implementation performance of medicine innovation policies, while little literature evaluates these policies from a policy formulation perspective. Second, the existing literature has not yet applied the PMC-Index model to evaluate medicine innovation policies, lacking the inclusion and comparative study of TCMIPs, BMIPs, and MMIPs simultaneously. Hence, this paper aims to narrow these gaps by investigating the TCMIPs, BMIPs, and MMIPs, constructing an evaluation indicator system, and utilizing the PMC-Index model to analyze these policies. The goal of this study is to gain insights into the current status of various medicine innovation policies and provide references for the formulation and improvement of these policies in the future.
The medicine innovation policies issued by the Chinese government are taken as the research object in this study. To obtain the policy texts on the medicine innovation policies systematically, we adopt three search paths. Firstly, relevant policy documents are retrieved on the portals of the State Council (SC), the National Health Commission (NHC), the National Medical Products Administration (NMPA), the National Administration of Traditional Chinese Medicine (NATCM), and other related government departments. Secondly, we search for relevant policy documents on the Peking University Law Website.1 Finally, search platforms such as Baidu and Google are used as supplements for policy document collection. We set search terms such as “medicine innovation,” “TCM innovation,” “biological medicine innovation,” and “chemical medicine innovation” in these databases. Considering the evolution characteristics of China’s medicine innovation policies, the retrieval period is from 2000 to 2023. Due to some repeated and invalid collection, the policy documents are screened according to the following principles: (1) only the national-level policy documents are selected in this study; (2) we eliminate some documents that have been revised or repealed; (3) policy documents such as working arrangements, letters, technical guidelines, and approvals are excluded; and (4) we focus on policies with specific plans. After eliminating the irrelevant policies, 76 policy documents are obtained, including 29 TCMIPs, 12 BMIPs, and 35 MMIPs (some policies are shown in Table 1). These policy documents mainly cover laws, regulations, plans, outlines, notices, and other relevant rules on medicine innovation in China.
Before the construction of the PMC-Index model, ROSTCM 6 software is adopted for text mining of the above policies (45, 48). We process the policy documents using the ROSTCM6 software, including policy integration, word segmentation, and high-frequency word statistics. Words that appear more frequently but are meaningless, such as “construct,” “increase,” and “development,” are deleted. Finally, the most relevant and frequent words are extracted for further analysis. High-frequency words can reflect the topic of general interest in policy documents (52). Besides, the top 30 high-frequency words are selected from TCMIPs, BMIPs, and MMIPs, and the Gephi software is adopted to establish a co-occurrence network to clearly show the difference and relevance of various types of medicine innovation policies.
The PMC-Index model is a scientific and quantitative measurement method for policy evaluation. This model is proposed by Estrada (12), which originates from the Omnia Mobilis hypothesis. The hypothesis believes that everything is in motion and interconnected, so any seemingly irrelevant variable should not be ignored, and the quantity and weights of variables are not restricted. The PMC-Index model analyzes the advantages and disadvantages of each policy and the consistency level of a policy in multiple dimensions by selecting variables comprehensively (47, 53). The PMC-Index model is composed of four main steps (52, 54) (see Figure 2).
The classification of variables and identification of parameters are essential bases for comprehensive policy evaluation. According to the existing studies (45–47) and the specific characteristics of medicine innovation policy, we establish 10 primary variables, namely policy nature (X1), policy issuing agency (X2), policy object (X3), policy timeliness (X4), policy instrument (X5), policy orientation (X6), incentive method (X7), policy function (X8), policy evaluation (X9), and policy accessibility (X10). The sub-variables are set for the primary variable by the relevant literature and policy text mining, as shown in Table 2. After classifying, it is essential to identify the parameters of the variables. The binary method is adopted to assign equal weight to all sub-variables (48, 55). If the policy content conforms to the sub-variable, the parameter is set to 1; otherwise, the parameter is set to 0 (46).
The multi-input-output table is an analysis framework capable of data storage that evaluates an individual variable in multiple dimensions (60, 61). Building a multi-input-output table is a precondition for the PMC-Index calculation of the medicine innovation policy. In this study, the multi-input-output tables consist of 10 primary variables and 40 sub-variables. The primary variables are not specially ordered and are mutually independent of each other. The sub-variables refine the primary variables in different aspects. In this paper, all researchers analyze and determine whether the policy content involves the sub-variables, respectively. The evaluation results of all researchers are almost the same, apart from a few variables. The controversial variables are further analyzed and discussed collectively on the basis of the policy content and evaluation criteria. After parameter identification, the multi-input-output tables for 29 TCMIPs, 12 BMIPs, and 35 MMIPs are established, as shown in Table 3.
The PMC-Index calculation includes the specific four steps (46, 55). Firstly, the primary variables and sub-variables are integrated into the multi-input-output tables of TCMIPs, BMIPs, and MMIPs, respectively. Secondly, the binary method is adopted to assign the value of each sub-variable according to text analysis and Eqs 1, 2. Thirdly, the values of 10 primary variables are calculated individually based on Eq. 3. Fourthly, we sum up all primary variables to obtain the PMC-Index of policies according to Eq. 4.
Where Xi refers to the ith primary variable, i = 1, 2, 3, …, 10. Xij refers to the ijth sub-variable, j = 1, 2, 3, …, n. T (Xij) refers to the number of sub-variables of the ith primary variable.
The PMC indexes of 29 TCMIPs, 12 BMIPs, and 35 MMIPs are calculated based on the above steps. The PMC-Index can evaluate the comprehensiveness and degree of policy consistency. Due to the 10 primary variables selected in our evaluation system, the value of the PMC-Index should be [0, 10]. According to existing studies (12, 52, 55), we classify the values of PMC-Index into 4 evaluation levels (see Table 4).
The PMC-Surface is constructed to visualize the strengths and weaknesses of policies in multiple dimensions. We plot the PMC-Surface by calculating the PMC matrix. To meet the balance and symmetry of the matrix, X10 is left out of this research (11, 46, 47). After removing X10, a 3 × 3 matrix is generated by the remaining 9 primary variables, as shown in Eq. 5. Then we utilize the above matrix to draw the PMC-Surface. The concave-convex degree and color depth of the PMC-Surface reflect the strengths and weaknesses of each policy visually. MATLAB software is applied to draw the PMC-Surface diagrams.
The central zone surrounded by the high-frequency words reflects the common concern of the TCMIPs, BMIPs, and MMIPs. As shown in Figure 3, it can be seen that “innovation” is located in the center area and has a high frequency, for “innovation” is the core theme of TCMIPs, BMIPs, and MMIPs. Table 5 illustrates that 30% of high-frequency words extracted from the TCMIPs, BMIPs, and MMIPs are the same, with shared highly-frequency words such as “science and technology,” “innovation,” “system,” “mechanism,” and “resource,” suggesting that the common focus of policy is on promoting science and technology development, allocating relevant resources, and improving the medicine innovation system.
The TCMIPs and BMIPs all involve high-frequency words such as “talent” and “capability,” indicating that talent cultivation is an important guarantee for the innovation of traditional Chinese medicine and biological medicine. The TCMIPs and MMIPs all involve high-frequency words such as “health,” “clinical,” and “institution,” suggesting that these policies emphasize the clinical efficacy of innovative medicines. The BMIPs and MMIPs all involve high-frequency words such as “enterprise,” “platform,” “treatment,” “disease,” and “safety,” which indicates that pharmaceutical enterprises are encouraged to build innovation platforms to develop efficient and safe innovative medicine. In addition, there is also important information at the edge region in Figure 3, which should not be ignored and reflects the different concerns of TCMIPs, BMIPs, and MMIPs.
The dedicated high-frequency words in TCMIPs involve “nation,” “culture,” “civilization,” “protection,” “criteria,” “evaluation,” and “cooperation,” which indicates that the government emphasizes protection and inheritance for TCMI culture, construction evaluation criteria for TCMI standardized management, and greater international cooperation on TCMI. The dedicated high-frequency words in BMIPs include “economics,” “industrialization,” “strategy,” etc. Due to the small scale of most biopharmaceutical enterprises, they are encouraged to carry out an industrialization strategy to boost BMI development. The dedicated high-frequency words in MMIPs involve “approval,” “regulation,” “achievement,” and “market.” These high-frequency words illustrate that the MMIPs prefer to create a favorable external environment to promote medicine innovation, for example, by speeding up innovative medicine approval, strengthening medicine regulation, encouraging achievement transformation, and cultivating the market environment.
Based on the above evaluation system and criteria, we calculate the PMC-Index and determine the level of TCMIPs, as shown in Table 6. The average PMC-Index of 29 TCMIPs is 5.77, which indicates good overall consistency in TCMIPs. Specifically, there are 23 TCMIPs with good consistency and 6 TCMIPs with bad consistency, while there are no excellent and no perfect among the 29 TCMIPs. In addition, these policies are mainly released by the NHC, the NMPA, and the NATCM, suggesting that the Chinese government attaches great importance to TCMI.
With the development of the TCM industry, the focus of TCMIPs has shifted from a general outline (P2, P5) to a specific implementation plan (P20, P21, and P27). For instance, P20 proposes detailed promotion measures for TCM innovation, P21 focuses on medical insurance to support TCM innovation, and P27 emphasizes scientific supervision to stimulate TCM innovation. This reflects the tendency of TCMIP formulations to shift from “rough” to “refined,” which is more conducive to policy implementation (62, 63). It has become one of the strategies emphasized for how to encourage the TCMI in the coming decades.
In this study, we select P2 (Good level) and P9 (Bad level) to display the differences between TCMIPs visually. The PMC-Surfaces of these selected TCMIPs are drawn according to the PMC matrix (see Figures 4A,B). The convex surface means a higher score on the corresponding primary variable, whereas the concave surface indicates a lower score. The PMC-Index of P2 is 6.90, ranking first among the 29 TCMIPs. P2 is jointly issued by seven government departments, making relatively comprehensive arrangements to promote TCMI. As shown in Figure 4A, the surface shape of P2 is relatively smooth except for the X6 (Policy orientation). This is because the policy orientation of P2 only involves encouragement and support, which neglects normative guidance and compulsory requirements. Due to the lower score of P2 on policy orientation, the improvement for P2 should take normative guidance and compulsory requirement on policy orientation into account.
The PMC-Index of P9 is 4.25, ranking 29th among the 29 TCMIPs. As shown in Figure 4B, there is obviously convexity in X8 of P9, while the overall surface shape of P9 is concave compared to P2. This indicates that P9 performs poorly on most of the primary variables. This is due to P9, which measures the registration of scientific and technological achievements in TCM and focuses mainly on the X8 (policy function) but ignores the other primary variables. The improvement path for policy is determined according to the difference between the primary variables and the average (47–49). Thus, the improvement path of P9 is X9-X3-X1-X5-X7-X2-X6.
As shown in Table 6, we calculate the PMC-Index and determine the level of BMMIPs based on the above evaluation system and criteria. The average PMC-Index of 12 BMIPs is 6.21, which indicates good overall consistency in BMIPs. Moreover, these policies are mainly released by the SC, the National Development and Reform Commission (NDRC), and the Ministry of Science and Technology (MST), which reflects that the Chinese government attaches much significance to BMI. There are 1 BMIP with excellent consistency and 11 BMIPs with good consistency, while there are no bad or perfect BMIPs among the 12 BMIPs.
Q3 has excellent consistency due to five powerful primary variables: policy object (X3), policy instrument (X5), incentive method (X7), policy function (X8), and policy evaluation (X9). Q3 is a notice on the biological “11th Five-Year Plan,” which is issued by the SC. This document covers a comprehensive range of policy objects, including government departments, medical institutions, enterprises, and scientific research institutions. Previous studies have divided policy instruments into supply side instruments (SSI), demand side instruments (DSI), and environmental side instruments (ESI) (45, 59). The above three types of policy instruments are used in Q3, including SSI that directly supports BMI development, DSI that directly pulls BMI development, and ESI that creates a favorable external environment for BMI development. Q3 adopts tax benefits, investment subsidies, and intellectual property protection to inspire BMI. The function of Q3 involves five specific aspects of X8, which aim to systematically promote innovation in biological medicine. Thus, Q3 is well designed according to five strong primary variables.
Q3 (Excellent level) and Q2 (Good level) are selected to display the differences between BMIPs visually in this study. The PMC-Index of Q3 is 7.02 ranking first among the 12 BMIPs, while the PMC-Index of Q2 is 5.38 ranking 12th among the 12 BMIPs. The PMC-Surfaces of these selected BMIPs are drawn according to the PMC matrix, as shown in Figures 5A,B. It can be seen that the PMC-Surface of Q3 lies at a higher location than that of Q2, which indicates that Q3 has better consistency. However, Q2 is a special announcement and aims to provide funding for BMI, which is relatively single on the policy scope and has bad consistency. Except for policy timeliness (X4) and policy orientation (X6), other primary variables in Q2 are lower than the average in different degrees; thereby, the improvement path of Q2 is X8-X7-X3-X9-X5-X1-X2.
We calculate the PMC-Index and determine the level of MMIPs based on the above evaluation system and criteria, as shown in Table 6. The average PMC-Index of 35 MMIPs is 6.06, which indicates good overall consistency in MMIPs. Specifically, there are 9 MMIPs with excellent consistency, 20 MMIPs with good consistency, 6 MMIPs with bad consistency, and no perfect MMIP among the 35 MMIPs. In addition, these policies are mainly released by the SC, the MST, the NHC, and the NMPA, suggesting that there is a higher authority in MMIP policy issuance.
As the medical industry develops, the MMIPs emphasis has shifted from a broad plan (R1, R4) to a detailed incentive scheme (R25, R32, and R34). For instance, R25 focuses on technology transfer to drive medicine innovation; R32 proposes detailed evaluation measures to accelerate innovative medicine to the market; and R34 emphasizes regulatory capacity construction to supervise innovative medicine development. Hence, the current MMIPs focus is to formulate practical and concrete implementation plans to promote medicine innovation.
The R33 (Excellent level) and R28 (Bad level) are selected to display the differences between MMIPs visually in this study. The PMC-Surfaces of these selected MMIPs are drawn according to the PMC matrix (see Figures 6A,B). The PMC-Index of R33 is 7.88, ranking first among the 35 MMIPs. It can be seen that the PMC -Surface of R33 is overall convex, for there are four coordinate points at scale “1” in Figure 6A. This policy is jointly issued by nine government departments, manifesting sufficient coordination and cooperation among departments. R33 aims to clarify the primary goals of medical industry development and accelerate the improvement of the medicine innovation system during the 14th Five-Year Plan period. Overall, R33 has comprehensive content, complete support, and abundant policy instruments, which is a scientifically reasonable policy.
The PMC-Index of R28 is 4.48, ranking 35th among the 35 MMIPs. As shown in Figure 6B, the PMC-Surface of R28 displays fewer convex points, while most surfaces are dented, which suggests that P2 scores lower on various variables. This is because R28 only adopts the means of legal constraints to guide medicine innovation without making specific arrangements in other aspects. The policy issuing agency is single, the policy instrument is deficient, and the policy function is narrow, leading to the dented surfaces of R28. Except for policy object (X3) and policy timeliness (X4), other primary variables in R28 are lower than the average in different degrees; thereby, the improvement path of R28 is X8-X9-X5-X7-X2-X1-X6.
To clarify the characteristics and differences of various medicine innovation policies in China, we compare the TCMIPs, BMIPs, and MMIPs for further analysis. Upon comparing the average PMC-Index of three types of medicine innovation policies, the order from high to low is BMIPs (6.21) > MMIPs (6.06) > TCMIPs (5.77), which suggests that BMIPs and MMIPs are superior to TCMIPs. In addition, the PMC-Index of TCMIPs range from 4.25 to 6.90, the PMC-Index of BMIPs range from 5.38 to 7.02, and the PMC-Index of MMIPs range from 4.48 to 7.88. In order to clearly display the distribution of the three types of policies across each evaluation level, we create the Table 7. As shown in Table 7, the MMIPs have the highest policy excellence rate (26%), the BMIPs have the highest policy good rate (92%), and the TCMIPs have the highest policy bad rate (21%). Next, we will explore the reasons for the differences through a comparative analysis of primary variables.
To show the differences of the various medicine innovation policies more intuitively, we construct a comparative radar chart of the average scores of the primary variables for BMIPs, MMIPs, and TCMIPs. As shown in Figure 7, the BMIPs, MMIPs, and TCMIPs have similar scores on X3, X4, X6, X9, and X10, while they differ significantly mainly on X1, X2, X5, X7, and X8. The specific analysis is as follows:
(1) X1 (Policy nature). Generally, policy nature is closely related to the purpose of policy formulation; in this paper, policy nature includes prediction, guidance, description, supervision, planning, and support (64, 65). The policy nature of TCMIPs is relatively homogeneous, most only involving guidance and description. Compared with TCMIPs and MMIPs, most BMIPs cover multiple policy natures (i.e., prediction, guidance, description, plan, and support) simultaneously. There are 49% of MMIPs that contain four types of policy natures (i.e., prediction, guidance, description, and plan) at the same time, ranging between TCMIPs and BMIPs. Overall, the three types of medicine innovation policies have less involvement in supervision, which should be incorporated into future policy formulation.
(2) X2 (policy issuing agency). The existing studies find that cooperation among policy issuing agencies affects the coordination ability of policy implementation (47, 66, 67). TCMIPs are mainly released independently or jointly by the NHC, the NMPA, and the NATCM, wherein 41% of TCMIPs are released independently by the NATCM and 24% of TCMIPs are released jointly by multiple departments. Moreover, BMIPs are mainly released by the SC, the NDRC, and the MST, whereas only 8% of BMIPs are released jointly by multiple departments. Further, MMIPs are mainly released by the SC, the MST, the NHC, and the NMPA, wherein 54% of MMIPs are released jointly by multiple departments. This suggests that, compared with the BMIPs and the TCMIPs, the MMIPs concentrate more on the coordination and cooperation among departments when formulating policies, which is more conducive to policy implementation.
(3) X5 (policy instrument). As mentioned earlier, policy instruments are generally divided into SSI, ESI, and DSI (45, 59). SSI mainly involves the support of financial, infrastructure construction, and technical information. ESI mainly involves regulation, supervision, and public opinion publicity. DSI mainly involves government procurement and service outsourcing. The policy instruments adopted by TCMIPs are SSI and ESI, while BMIPs and MMIPs cover three types of policy instruments. In addition, the usage frequency of DSI in BMIPs is slightly higher than that in MMIPs.
(4) X7 (incentive method). Incentive methods refer to measures that promote policy implementation, such as tax benefits, investment subsidies, intellectual property protection, regulatory and evaluation, and administrative approval incentives. From the content of the policy text, some BMIPs and MMIPs adopt multiple incentive methods, while most TCMIPs only involve one incentive method. Thus, in terms of incentive methods, BMIPs and MMIPs are superior to TCMIPs. However, the average score of the incentive methods for BMIPs and MMIPs is 0.42, indicating that they are generally unsatisfactory and that there is much room for improvement.
(5) X8 (policy function). The policy function means the social effect that can be achieved after policy implementation (68, 69). In this paper, policy functions mainly include talent cultivation, encouraging innovations, industry-academia-research collaboration, achievement transformation, and international exchange. There are most BMIPs covering the above six functions, followed by TMIPs and MMIPs. It is worth noting that the average policy functions (X7) score of MMIPs is 0.71, which indicates that the functions of medicine policies are generally good.
Based on the above analysis results, we will further discuss the following aspects. First, we find that the nature of medicine innovation policy mainly focuses on guidance, description, and plan, which is similar to the findings of existing studies. For example, Zhang et al. (52) researched coal power policy and concluded that these policies are mainly manifested as guidance and description. Yang et al. (13) found that more than 90% of TCM development policies involved guidance and planning. This study, using a larger policy sample, responds to their results. Moreover, we have new findings compared with their research. We find that the nature of supervision is rarely incorporated in medicine innovation policies, and there is a lack of supervision over implementation effectiveness.
Second, there is an overall lack of cooperation among departments in this study from the perspective of the policy issuing agency. This is similar to some previous research findings, which also believe that there is weak collaboration among policy issuing agencies (11, 46, 62). This may be due to differences in specific responsibilities and potential competition among departments, which result in a reluctance to cooperate and coordinate with each other. However, existing research suggests that cross-departmental cooperation is more likely to enhance administrative efficiency (70–72). Moreover, many TCMIPs are independently issued by the NATCM, which is a vice-ministerial department with a relatively low administrative level. These findings are similar to those of Yang et al. (49). In general, the administrative level of the policy issuing agency has a positive impact on the efficiency of policy implementation (66). Furthermore, the two departments that are most relevant to the issuance of medicine innovation policies in China are the NMPA and the NATCM, which are under the State Administration for Market Regulation and the NHC, respectively. Hence, it is difficult to coordinate the management of these two departments and jointly formulate medicine innovation policies in practice.
Third, in terms of policy instruments, BMIPs and MMIPs perform well overall, while TCMIPs need to improve. Specifically, we find that the TCMIPs lack the application of DSI. This paper complements the study of Yang et al. (13), in which policy instruments are not included in the evaluation system. Moreover, Xiong et al. (48) analyzed the application of policy instruments in the fire safety education policy, involving voluntary policy tools, mandatory tools, and mixed policy tools. The classification of policy instrument types differs between Xiong and us, which belong to different schools. Overall, we have further enriched the research on policy instruments.
Finally, the incentive method for the medicine innovation policy is overall insufficient. Specifically, medicine innovation policy in China takes financial subsidies and intellectual property protection as the main incentive methods, while other incentive methods are seldom involved. Similar findings have been reported in previous studies. For example, Yang et al. (49) found that the new energy vehicle policy has inadequate incentives for infrastructure construction. Yang et al. (11) conducted a study on 37 health promotion policies that seldom involve incentives. These findings indicate that insufficient incentives are a common problem with policies. However, a lack of appropriate incentive methods will hinder the rapid growth of the industry.
This study quantitatively evaluates and analyzes the medicine innovation policies in China since 2000 through mining the text and the PMC-Index model. Specifically, we summarize the respective characteristics of the TCMIPs, BMIPs, and MMIPs and further compare the similarities and differences of the three types of policies from horizontal perspectives. Then, further discussion and analysis are conducted, eliciting suggestions for medicine innovation policy improvement. As far as we know, this study is the first to quantitatively explore the consistency of medicine innovation policies across different types in China and fills the gap in existing literature.
Based on the analysis of the high-frequency words of medicine innovation policy, the results show that the common focus of policy is to promote the development of science and technology, allocate related resources, and improve the pharmaceutical innovation system. In addition, various medicine innovation policies also have their own unique focus. Specifically, the TCMIPs emphasize the inheritance and innovation of TCM; the BMIPs focus on encouraging biopharmaceutical companies to implement industrialization strategies; and the MMIPs tend to create a favorable external environment to promote medicine innovation.
This study utilizes the PMC-Index model to systematically evaluate the TCMIPs, BMIPs, and MMIPs, respectively. The results showed that the average PMC-Index of 29 TCMIPs is 5.77, wherein the policy at the good and bad levels accounts for 79 and 21%, respectively. Compared to BMIPs and MMIPs, the average primary variable values of policy nature (X1), policy instrument (X5), and incentive method (X7) are relatively lower in TCMIPs, so priority could be given to improving TCMIPs in these areas in the future. The average PMC-Index of 12 BMIPs is 6.21, wherein the policy at excellent level accounts for 8% and the policy at good level accounts for 92%, respectively. Moreover, the average PMC-Index of 35 MMIPs is 6.06, wherein the policy at excellent level accounts for 26%, the policy at good level accounts for 57%, and the policy at bad level accounts for 17%, respectively. These results indicate that none of the medicine innovation policies in China reach a perfect consistency level, so there is still room for improvement. In addition, the BMIPs, MMIPs, and TCMIPs have similar scores on X3, X4, X6, X9, and X10, while they differ significantly mainly on X1, X2, X5, X7, and X8.
The theoretical significance of this study is reflected in several aspects. First, this study provides a theoretical reference for the medicine innovation policy formulation in China. In other words, policymakers can design more effective policies by drawing on our study when formulating medicine innovation policies in the future. Second, the PMC-Index model has not previously been used to evaluate medicine innovation policies. Thus, the PMC-Index model is utilized to evaluate the medicine innovation policy in this study, which enriches this stream of literature. Finally, we extend the application context of policy diffusion theory to evaluate medicine innovation policy. Specifically, this paper verifies that the formulation of medicine innovation policy in China conforms to the connotation of policy diffusion theory.
Based on the above analysis, the policy implications proposed in this study are as follows. (1) According to the different policy timeliness, corresponding assessment times should be set when formulating policies, such as long-term monitoring of more than 5 years, medium-term monitoring of 3–5 years, short-term monitoring of 1–3 years, and monitoring within 1 year. Then, establish monitoring standards based on different innovation policy objectives and monitor when the assessment time is reached. Through the establishment of a cross-departmental monitoring information sharing platform, all departments can share their supervision results of medicine innovation policies. Based on the results of supervisory feedback, problems existing in medicine innovation policies should be optimized when formulating policies in the future. (2) The joint superior department of the NMPA and the NATCM can be set up when institutional reform is carried out in the future. This joint superior department plays a connecting role among the SC, other ministerial-level departments, the NMPA, and the State Administration of TCM. The joint superior department can coordinate multiple departments to release medicine innovation policies based on the goals and contents of the policies, improve the administrative level of policy release, and thereby promote medicine innovation. (3) In the formulation of BMIPs and MMIPs, the three policy instruments should be further integrated to bring into play the complementarity among them. Government departments should refer to the application of DSI in BMIPs and MMIPs and incorporate DSI (i.e., government procurement and government purchasing) into the formulation of future TCMIPs, taking into account the characteristics of TCMIPs. DSI can directly stimulate policy receptors and produce significant effects. (4) Incentives should be appropriately enriched and diversified to provide sufficient incentives for medicine innovation in policy formulation. Provide the implementation details of the corresponding incentives, such as the application conditions of the incentive method, to prevent the abuse of the incentives.
This study investigates the strengths and weaknesses of medicine innovation policies from the perspective of policy formulation, which provides new insights and a theoretical basis for the evaluation of medicine innovation policies in the future. However, some limitations in our research should be realized. First, there may be some subjectivity in the variable identification. To obtain a more objective and scientific policy evaluation, we can optimize the shortcomings in future research through various methods such as grounded theory, crawler mining, and big data methods. Second, this study mainly selects medicine innovation policies from the national level while not including the local level. In future studies, medicine innovation policies issued by local governments can be included for comparative analysis because they have local characteristics and provide richer references for policy formulation. Finally, this study only evaluates the text of medicine innovation policies in China without analyzing the performance of policy implementation. The difference in difference econometric analysis model can be used to further analyze the implementation effect of medicine innovation policies in the future.
The original contributions presented in the study are included in the article/Supplementary materials, further inquiries can be directed to the corresponding author.
DG: Conceptualization, Data curation, Formal analysis, Investigation, Methodology, Software, Writing – original draft. LQ: Data curation, Formal analysis, Methodology, Supervision, Writing – review & editing. XS: Conceptualization, Data curation, Formal analysis, Funding acquisition, Resources, Supervision, Writing – review & editing.
The author(s) declare financial support was received for the research, authorship, and/or publication of this article. This research was funded by the National Social Science Foundation of China (16ZDA236).
The authors declare that the research was conducted in the absence of any commercial or financial relationships that could be construed as a potential conflict of interest.
All claims expressed in this article are solely those of the authors and do not necessarily represent those of their affiliated organizations, or those of the publisher, the editors and the reviewers. Any product that may be evaluated in this article, or claim that may be made by its manufacturer, is not guaranteed or endorsed by the publisher.
The Supplementary material for this article can be found online at: https://www.frontiersin.org/articles/10.3389/fpubh.2024.1403320/full#supplementary-material
PMC-Index, Policy Modeling Consistency Index; TCM, Traditional Chinese medicine; TCMIPs, Traditional Chinese medicine innovation policies; BMIPs, Biological medicine innovation policies; MMIPs, Multiple medicine innovation policies; SSI, Supply side instruments; ESI, Environmental side instruments; DSI, Demand side instruments; SC, State Council; NHC, National Health Commission; NMPA, National Medical Products Administration; NATCM, National Administration of traditional Chinese medicine; NDRC, National Development and Reform Commission; MST, Ministry of Science and Technology.
1. Debaveye, S, De Soete, W, De Meester, S, Vandijck, D, Heirman, B, Kavanagh, S, et al. Human health benefits and burdens of a pharmaceutical treatment: discussion of a conceptual integrated approach. Environ Res. (2016) 144:19–31. doi: 10.1016/j.envres.2015.10.027
2. Kanavos, P, Visintin, E, and Gentilini, A. Algorithms and heuristics of health technology assessments: a retrospective analysis of factors associated with HTA outcomes for new drugs across seven OECD countries. Soc Sci Med. (2023) 331:116045. doi: 10.1016/j.socscimed.2023.116045
3. Pe, M, Alanya, A, Falk, RS, Amdal, CD, Bjordal, K, Chang, J, et al. Setting international standards in analyzing patient- reported outcomes and quality of life endpoints in cancer clinical trials-innovative medicines initiative (SISAQOL-IMI) stakeholder views, objectives, and procedures. Lancet Oncol. (2023) 24:E270–83. doi: 10.1016/s1470-2045(23)00157-2
4. Chen, Y. How to boost long-term confidence in China’s innovative drugs. China Reform. (2023) 1:59–65.
5. Chen, XX, Giles, J, Yao, Y, Yip, WN, Meng, QQ, Berkman, L, et al. The path to healthy ageing in China: a Peking University lancet commission. Lancet. (2022) 400:1967–2006. doi: 10.1016/s0140-6736(22)01546-x
6. Li, Y, Gao, Y, Meng, X, Liu, X, and Feng, Y. Assessing the air pollution abatement effect of prefabricated buildings in China. Environ Res. (2023) 239:117290. doi: 10.1016/j.envres.2023.117290
7. Shen, Q, Pan, YX, and Feng, YC. Identifying impacts of industrial co-agglomeration on carbon emissions: evidence from China. Front Public Health. (2023) 11:1154729. doi: 10.3389/fpubh.2023.1154729
8. Gao, X, Zhang, G, Zhang, Z, Wei, Y, Liu, D, and Chen, Y. How does new energy demonstration city pilot policy affect carbon dioxide emissions? Evidence from a quasi-natural experiment in China. Environ Res. (2024) 244:117912. doi: 10.1016/j.envres.2023.117912
9. Shen, Q, Pan, Y, Meng, X, Ling, X, Hu, S, and Feng, Y. How does the transition policy of mineral resource-exhausted cities affect the process of industrial upgrading? New empirical evidence from China. Resour Policy. (2023) 86:104226. doi: 10.1016/j.resourpol.2023.104226
10. Sun, CW, Xu, SH, Yang, M, and Gong, X. Urban traffic regulation and air pollution: a case study of urban motor vehicle restriction policy. Energ Policy. (2022) 163:112819. doi: 10.1016/j.enpol.2022.112819
11. Yang, YL, Tang, J, Li, ZX, and Wen, J. How effective is the health promotion policy in Sichuan, China: based on the PMC-index model and field evaluation. BMC Public Health. (2022) 22:2391. doi: 10.1186/s12889-022-14860-9
12. Estrada, MAR. Policy modeling: definition, classification and evaluation. J Policy Model. (2011) 33:523–36. doi: 10.1016/j.jpolmod.2011.02.003
13. Yang, CR, Yin, SC, Cui, D, Mao, ZF, Sun, Y, Jia, CL, et al. Quantitative evaluation of traditional Chinese medicine development policy: a PMC index model approach. Front Public Health. (2022) 10:1041528. doi: 10.3389/fpubh.2022.1041528
14. Jiang, YT, Xiao, YN, Zhang, ZH, and Zhao, S. How does central-local interaction affect local environmental governance? Insights from the transformation of central environmental protection inspection in China. Environ Res. (2024) 243:117668. doi: 10.1016/j.envres.2023.117668
15. Niu, SH, Chen, YD, Zhang, RW, Luo, RF, and Feng, YC. Identifying and assessing the global causality among energy poverty, educational development, and public health from a novel perspective of natural resource policy optimization. Resour Policy. (2023) 83:103770. doi: 10.1016/j.resourpol.2023.103770
16. Zhang, ZH, Shang, YZ, Zhang, GX, Shao, S, Fang, JY, Li, PX, et al. The pollution control effect of the atmospheric environmental policy in autumn and winter: evidence from the daily data of Chinese cities. J Environ Manag. (2023) 343:118164. doi: 10.1016/j.jenvman.2023.118164
17. Chun, N, and Park, M. The impact of health insurance mandates on drug innovation: evidence from the United States. Eur J Health Econ. (2013) 14:323–44. doi: 10.1007/s10198-012-0379-6
18. Um, SI, Sohn, UD, Jung, SY, You, SH, Kim, C, Lee, S, et al. Longitudinal study of the impact of three major regulations on the Korean pharmaceutical industry in the last 30 years. Health Res Policy Syst. (2022) 20:4. doi: 10.1186/s12961-021-00797-9
19. Wu, GY, Sun, MM, and Feng, YC. How does the new environmental protection law affect the environmental social responsibility of enterprises in Chinese heavily polluting industries? Hum Soc Sci Commun. (2024) 11:1–14. doi: 10.1057/s41599-024-02674-6
20. Bouet, D. A study of intellectual property protection policies and innovation in the Indian pharmaceutical industry and beyond. Technovation. (2015) 38:31–41. doi: 10.1016/j.technovation.2014.10.007
21. Gamba, S. The effect of intellectual property rights on domestic innovation in the pharmaceutical sector. World Dev. (2017) 99:15–27. doi: 10.1016/j.worlddev.2017.06.003
22. Aghmiuni, SK, Siyal, S, Wang, Q, and Duan, YZ. Assessment of factors affecting innovation policy in biotechnology. J Innov Knowl. (2020) 5:179–89. doi: 10.1016/j.jik.2019.10.002
23. Liu, Q, Huang, Z, and Mao, ZB. Has the consistency evaluation policy of generic drugs promoted the innovation quality of Chinese pharmaceutical manufacturing industry? An empirical study based on the difference-in-differences model. Front Public Health. (2023) 11:1265756. doi: 10.3389/fpubh.2023.1265756
24. Gu, Y, and Zhuang, Q. Does China’s centralized volume-based drug procurement policy facilitate the transition from imitation to innovation for listed pharmaceutical companies? Empirical tests based on double difference model. Front Pharmacol. (2023) 14:1192423. doi: 10.3389/fphar.2023.1192423
25. Doran, E, and Henry, DA. Australian pharmaceutical policy: Price control, equity, and drug innovation in Australia. J Public Health Policy. (2008) 29:106–20. doi: 10.1057/palgrave.jphp.3200170
26. Karampli, E, Souliotis, K, Polyzos, N, Kyriopoulos, J, and Chatzaki, E. Pharmaceutical innovation: impact on expenditure and outcomes and subsequent challenges for pharmaceutical policy, with a special reference to Greece. Hippokratia. (2014) 18:100–6.
27. Liu, Y, Zhang, N, Xie, CC, Jiang, YL, Qin, YH, Zhou, LY, et al. Evolution of drug regulations and regulatory innovation for anticancer drugs in China. Acta Pharm Sin B. (2022) 12:4365–77. doi: 10.1016/j.apsb.2022.08.004
28. Dommarco, JAR, de Cosío, TG, García-Chávez, CG, and Colchero, MA. The role of public nutrition research organizations in the construction, implementation and evaluation of evidence-based nutrition policy: two National Experiences in Mexico. Nutrients. (2019) 11:594. doi: 10.3390/nu11030594
29. Führ, M, and Bizer, K. REACh as a paradigm shift in chemical policy: responsive regulation and behavioural models. J Clean Prod. (2007) 15:327–34. doi: 10.1016/j.jclepro.2005.10.003
30. Zhang, JX, Cheng, C, and Feng, YC. The heterogeneous drivers of CO2 emissions in China’s two major economic belts: new evidence from spatio-temporal analysis. Environ Dev Sustain. (2023) 26:10653–10679. doi: 10.1007/s10668-023-03169-1
31. Bigman, SK. Evaluative research: principles and practice in public service and social action programs. By Edward A Suchman. J Leis Res. (1969) 1:209–11. doi: 10.1080/00222216.1969.11969732
32. OF Poland. Program evaluation and administrative theory. Public Adm Rev. (1974) 34:333–8. doi: 10.2307/975243
33. Libecap, GD. Economic variables and the development of the law: the case of Western mineral rights. J Econ Hist. (1978) 38:338–62. doi: 10.1017/S0022050700105121
34. Saaty, TL. A new macroeconomic forecasting and policy evaluation method using the analytic hierarchy process. Math Model. (1987) 9:219–31. doi: 10.1016/0270-0255(87)90479-9
35. Deloe, RC. Exploring complex policy questions using the policy delphi- a multi round, interactive survey method. Appl Geogr. (1995) 15:53–68. doi: 10.1016/0143-6228(95)91062-3
36. Foote, SB, Halpern, R, and Wholey, DR. Variation in Medicare’s local coverage policies: content analysis of local medical review policies. Am J Manag Care. (2005) 11:181–7.
37. Zhang, SJ, Dong, R, Jiang, JX, Yang, SY, Cifuentes-Faura, J, Peng, SH, et al. Whether the green credit policy effectively promote green transition of enterprises in China? Empirical analysis and mechanism verification. Environ Res. (2024) 244:117910. doi: 10.1016/j.envres.2023.117910
38. Yang, M, Liu, X, and Li, Z. Can the construction of digital infrastructure promote labor force employment? — evidence from the pilot policy of “broadband China”. Sys Eng Theory Pract. (2024) 44:190–206. doi: 10.12011/SETP2023-1579
39. Wu, G, Gao, Y, and Feng, Y. Assessing the environmental effects of the supporting policies for mineral resource-exhausted cities in China. Resour Policy. (2023) 85:103939. doi: 10.1016/j.resourpol.2023.103939
40. Cahuc, P, and Le Barbanchon, T. Labor market policy evaluation in equilibrium: some lessons of the job search and matching model. Labour Econ. (2010) 17:196–205. doi: 10.1016/j.labeco.2009.08.012
41. Ling, X, Luo, ZW, Feng, YC, Liu, X, and Gao, Y. How does digital transformation relieve the employment pressure in China? Empirical evidence from the national smart city pilot policy. Hum Soc Sci Commun. (2023) 10:1–17. doi: 10.1057/s41599-023-02131-w
42. Hua, C, Zhang, Z, Miao, J, Sun, H, and Jia, F. Do urban agglomeration planning policies promote the discharge reduction of industrial wastewater: evidence from the Yellow River Basin in China. Environ Res. (2023) 239:117445. doi: 10.1016/j.envres.2023.117445
43. Niu, SH, Chen, YD, Zhang, RW, and Feng, YC. How does the air pollution prevention and control action plan affect sulfur dioxide intensity in China? Front Public Health. (2023) 11:1119710. doi: 10.3389/fpubh.2023.1119710
44. Vogel, M, Kakani, P, Chandra, A, and Conti, RM. Medicare price negotiation and pharmaceutical innovation following the inflation reduction act. Nat Biotechnol. (2024) 42:406–12. doi: 10.1038/s41587-023-02096-w
45. Liu, F, Tang, J, Rustam, A, and Liu, Z. Evaluation of the central and local power batteries recycling policies in China: a PMC-index model approach. J Clean Prod. (2023) 427:139073. doi: 10.1016/j.jclepro.2023.139073
46. Fan, XH, Chu, ZJ, Chu, X, Wang, S, Huang, WC, and Chen, JC. Quantitative evaluation of the consistency level of municipal solid waste policies in China. Environ Impact Assess Rev. (2023) 99:107035. doi: 10.1016/j.eiar.2023.107035
47. Zhao, XC, Jiang, M, Wu, ZJ, and Zhou, Y. Quantitative evaluation of China’s energy security policy under the background of intensifying geopolitical conflicts: based on PMC model. Resour Policy. (2023) 85:104032. doi: 10.1016/j.resourpol.2023.104032
48. Xiong, YC, Zhang, CL, and Qi, H. How effective is the fire safety education policy in China? A quantitative evaluation based on the PMC-index model. Saf Sci. (2023) 161:106070. doi: 10.1016/j.ssci.2023.106070
49. Yang, T, Xing, C, and Li, XY. Evaluation and analysis of new-energy vehicle industry policies in the context of technical innovation in China. J Clean Prod. (2021) 281:125126. doi: 10.1016/j.jclepro.2020.125126
50. Liu, Q, Jia, MT, and Xia, D. Dynamic evaluation of new energy vehicle policy based on text mining of PMC knowledge framework. J Clean Prod. (2023) 392:136237. doi: 10.1016/j.jclepro.2023.136237
51. Wei, Q, Wang, XY, Zhang, GR, Li, XG, Yang, XJ, and Gu, DX. Internet healthcare policy analysis, evaluation, and improvement path: multidimensional perspectives. Healthcare (Basel). (2023) 11:1905. doi: 10.3390/healthcare11131905
52. Zhang, CH, Li, XW, Sun, YF, Chen, J, and Streimikiene, D. Policy modeling consistency analysis during energy crises: evidence from China’s coal power policy. Technol Forecast Soc Change. (2023) 197:122931. doi: 10.1016/j.techfore.2023.122931
53. Chen, TG, Rong, JT, Peng, LJ, Yang, JJ, Cong, GD, and Fang, J. Analysis of social effects on employment promotion policies for college graduates based on data Mining for Online use Review in China during the COVID-19 pandemic. Healthcare (Basel). (2021) 9:846. doi: 10.3390/healthcare9070846
54. Li, SQ, Shen, JQ, Sun, FH, Jia, YZ, and Han, HK. Quantitative evaluation of ecological compensation policies for the watershed in China: based on the improved policy modeling consistency index. Environ Sci Pollut R. (2022) 29:66659–74. doi: 10.1007/s11356-022-20503-8
55. Li, ZH, and Guo, XS. Quantitative evaluation of China’s disaster relief policies: a PMC index model approach. Int J Disaster Risk Reduct. (2022) 74:102911. doi: 10.1016/j.ijdrr.2022.102911
56. Liu, LM, Chen, JF, Wang, CB, and Wang, Q. Quantitative evaluation of China’s basin ecological compensation policies based on the PMC index model. Environ Sci Pollut R. (2023) 30:17532–45. doi: 10.1007/s11356-022-23354-5
57. Zhang, YK, Wang, T, Wang, CB, and Cheng, CG. Quantitative evaluation of China’s CSR policies based on the PMC-index model. Sustain For. (2023) 15:7194. doi: 10.3390/su15097194
58. Liu, YW, Li, J, and Xu, Y. Quantitative evaluation of high-tech industry policies based on the PMC-index model: a case study of China’s Beijing-Tianjin-Hebei region. Sustain For. (2022) 14:9338. doi: 10.3390/su14159338
59. Roy, R. Industrial innovation and public policy preparing for the 1980s and the 1990s. Design. (1981) 13.
60. Xu, Y, Li, J, and Liu, YW. Quantitative evaluation of provincial government plans for public health emergencies in China. Int J Disaster Risk Reduct. (2022) 81:103292. doi: 10.1016/j.ijdrr.2022.103292
61. Jiang, ZZ, and Gao, XW. Text mining and quantitative evaluation of China’s green consumption policies based on green consumption objects. Environ Dev Sustain. (2023) 26:6601–6622. doi: 10.1007/s10668-023-02976-w
62. He, ZY, Chu, ZJ, Zhao, MY, Zhuang, J, and Liu, FR. Policy-making coordination of municipal solid waste policies in China: a content analysis. J Mater Cycles Waste Manag. (2018) 20:1073–84. doi: 10.1007/s10163-017-0668-3
63. Zhang, ZH, Cheng, SQ, Wang, C, Song, SF, and Feng, YC. Climate policy uncertainty and corporate investment efficiency: evidence from China. J Environ Plan Manag. (2023) 1–21. doi: 10.1080/09640568.2023.2276062
64. Dolowitz, DP, and Marsh, D. Learning from abroad: the role of policy transfer in contemporary policy-making. Governance. (2000) 13:5–24. doi: 10.1111/0952-1895.00121
65. O’Faircheallaigh, C. Public participation and environmental impact assessment: purposes, implications, and lessons for public policy making. Environ Impact Assess Rev. (2010) 30:19–27. doi: 10.1016/j.eiar.2009.05.001
66. Dong, F, and Liu, YJ. Policy evolution and effect evaluation of new-energy vehicle industry in China. Resour Policy. (2020) 67:101655. doi: 10.1016/j.resourpol.2020.101655
67. Kuang, B, Han, J, Lu, XH, Zhang, XP, and Fan, XY. Quantitative evaluation of China’s cultivated land protection policies based on the PMC-index model. Land Use Policy. (2020) 99:105062. doi: 10.1016/j.landusepol.2020.105062
68. Ghazinoory, S, and Aghaei, P. Differences between policy assessment & policy evaluation; a case study on supportive policies for knowledge-based firms. Technol Forecast Soc Change. (2021) 169:120801. doi: 10.1016/j.techfore.2021.120801
69. Yu, JK, Yin, W, and Liu, DH. Evolution of mariculture policies in China: experience and challenge. Mar Policy. (2020) 119:104062. doi: 10.1016/j.marpol.2020.104062
70. Liu, F, Liu, G, Wang, X, and Feng, Y. Whether the construction of digital government alleviate resource curse? Empirical evidence from Chinese cities. Resour Policy. (2024) 90:104811. doi: 10.1016/j.resourpol.2024.104811
71. Chu, ZJ, Wu, BY, He, ZY, Zhuang, J, and Wang, WN. The policy-making trend analysis of municipal solid waste in China 1980–2015. Waste Manag Res. (2019) 37:601–10. doi: 10.1177/0734242x19836722
Keywords: PMC-Index model, medicine innovation policy, China, quantitative evaluation, policy formulation
Citation: Guo D, Qi L and Song X (2024) Quantitative evaluation of the medicine innovation policy in China: based on the PMC-Index model. Front. Public Health. 12:1403320. doi: 10.3389/fpubh.2024.1403320
Received: 19 March 2024; Accepted: 01 May 2024;
Published: 16 May 2024.
Edited by:
Yanchao Feng, Zhengzhou University, ChinaReviewed by:
Feng Hu, Zhejiang Gongshang University, ChinaCopyright © 2024 Guo, Qi and Song. This is an open-access article distributed under the terms of the Creative Commons Attribution License (CC BY). The use, distribution or reproduction in other forums is permitted, provided the original author(s) and the copyright owner(s) are credited and that the original publication in this journal is cited, in accordance with accepted academic practice. No use, distribution or reproduction is permitted which does not comply with these terms.
*Correspondence: Dan Guo, MTkxMTIyNEB0b25namkuZWR1LmNu
Disclaimer: All claims expressed in this article are solely those of the authors and do not necessarily represent those of their affiliated organizations, or those of the publisher, the editors and the reviewers. Any product that may be evaluated in this article or claim that may be made by its manufacturer is not guaranteed or endorsed by the publisher.
Research integrity at Frontiers
Learn more about the work of our research integrity team to safeguard the quality of each article we publish.