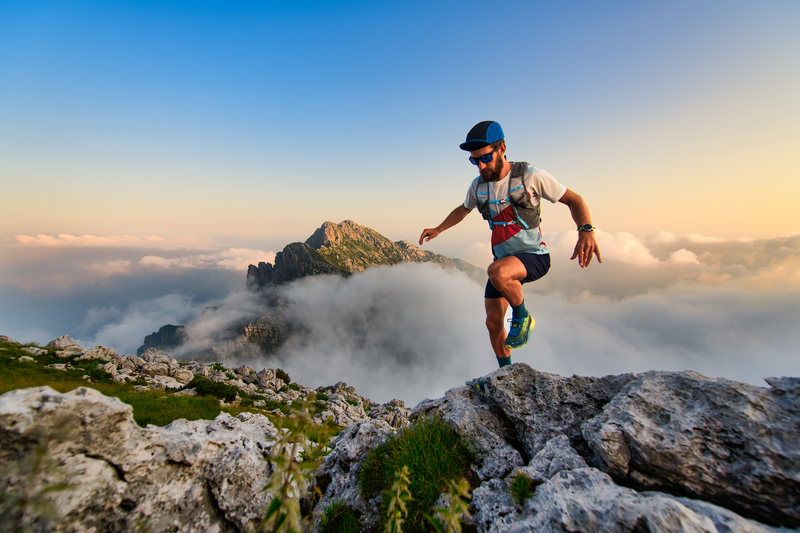
94% of researchers rate our articles as excellent or good
Learn more about the work of our research integrity team to safeguard the quality of each article we publish.
Find out more
ORIGINAL RESEARCH article
Front. Public Health , 01 August 2024
Sec. Environmental Health and Exposome
Volume 12 - 2024 | https://doi.org/10.3389/fpubh.2024.1401034
This article is part of the Research Topic Toxicity Mechanisms of Environmental Pollutants and Health Risk Assessment View all 20 articles
Background: Mounting evidence suggests a correlation between heavy metals exposure and diabetes. Diabetic retinopathy (DR) is a prevalent and irreversible complication of diabetes that can result in blindness. However, studies focusing on the effects of exposure to heavy metals on DR remain scarce. Thus, this study aimed to investigate the potential correlation between heavy metals exposure and DR.
Methods: A total of 1,146 diabetics from the National Health and Nutrition Examination Survey (NHANES) between 2005 and 2018 were included in this study. Heavy metal levels were measured via urine testing. Weighted logistic regression, Bayesian kernel machine regression (BKMR), weighted quantile sum (WQS) regression, and restricted cubic spline (RCS) were utilized to investigate the potential relationships between exposure to 10 heavy metals and DR. Finally, subgroup analysis was conducted based on the glycemic control status.
Results: Among the 1,146 participants, 239 (20.86%) were diagnosed with DR. Those with DR had worse glycemic control and a higher prevalence of chronic kidney disease compared to those without DR. Moreover, both the WQS regression and BKMR models demonstrated a positive relationship between exposure to mixed heavy metals and the risk of DR. The results of weighted logistic regression revealed a positive correlation between cobalt (Co) and antimony (Sb) exposure and the risk of DR (OR = 1.489, 95%CI: 1.064–2.082, p = 0.021; OR = 1.475, 95% CI: 1.084–2.008, p = 0.014), while mercury (Hg) exposure was found to promote DR exclusively in the group with good glycemic control (OR = 1.509, 95% CI: 1.157–1.967, p = 0.003). These findings were corroborated by the results of the RCS analysis.
Conclusion: Heavy metal exposure is associated with an increased risk of DR, especially Sb, Co, and Hg exposure. Nevertheless, well-designed prospective studies are warranted to validate these findings.
Diabetic retinopathy (DR) is a prevalent microvascular complication of diabetes mellitus that affects approximately one-third of diabetic patients (1). It causes varying degrees of visual impairment (2), which significantly impacts the quality of life of patients and imposes substantial economic burdens on society (3). Notably, its pathogenesis is complex and multifaceted, including oxidative stress, inflammation, and mitochondrial disorders, among others (4). At present, there is a pressing need to identify the risk factors and intervention strategies for DR in order to enhance the prognosis of patients with DR.
As is well documented, heavy metals are ubiquitously present in the air, soil, water, food, and manufactured products (5–9). Exposure to heavy metals may increase the risk of various ocular diseases, including DR, age-related macular degeneration (AMD), glaucoma, and cataracts (10–13). Zhu et al. demonstrated that the accumulation of serum cesium (Cs) and cadmium (Cd) was significantly correlated with the risk of developing DR (10). Similarly, the findings of Li et al. indicated that exposure to certain heavy metals, including lithium (Li), Cd, strontium (Sr), and magnesium (Mg), may increase the risk of developing proliferative DR, whereas selenium (Se) appears to be a protective factor (14). Zhang et al. observed a significant negative correlation between serum manganese (Mn) levels and DR prevalence in individuals with type 2 diabetes mellitus in the United States (15). However, the correlation between serum Cd, mercury (Hg), and lead (Pb), and DR was not statistically significant (15). Other studies have determined a potential association between cobalt (Co), barium (Ba), molybdenum (Mo), antimony (Sb), thallium (Tl), and tungsten (Tu) and the risk of diabetes (16–18), but their relationship with DR remains elusive. Although previous studies have preliminarily explored the link between heavy metals and DR, certain limitations remain. For instance, earlier studies exclusively investigated the association between the levels of serum heavy metals and DR risk, with a lack of research on the effect of urinary heavy metals on DR. Serum heavy metal levels may correlate with recent exposure, whereas urine heavy metal concentrations reflect long-term exposure (19). Furthermore, heavy metals are frequently co-exposed in the environment, and interactions between metals may also have an impact on human health (20, 21). However, studies on co-exposure to heavy metals and DR risk are lacking. Additionally, there is a lack of epidemiological studies to elucidate the effects of other heavy metals, such as Co and Sb, on the risk of developing DR.
The present study extracted U.S. demographic data from the National Health and Nutrition Examination Survey (NHANES) between 2005 and 2018 to investigate the relationship between heavy metals and the risk of DR. A total of 10 urinary heavy metals, namely Ba, Cd, Co, Cs, Mo, Pb, Sb, Tl, Tu, and Hg were analyzed. The effect of single and multiple metals on DR risk was evaluated using weighted logistic regression analysis. Furthermore, weighted quantile sum (WQS) regression and Bayesian kernel machine regression (BKMR) model were applied to investigate the relationship between heavy metals co-exposure and DR. In addition, dose–response relationships between heavy metals and DR were explored using restricted cubic spline (RCS) regression. Lastly, subgroup analysis was conducted based on glycemic control levels. Our findings are anticipated to provide new epidemiological evidence to enhance the understanding of the correlation between heavy metals and DR and assist in the prevention of DR.
The NHANES aimed to assess the health and nutritional status of the US population. By employing a complex multistage probability sampling technique, the NHANES collects information on the nation’s civilian population every 2 years (22). In the current study, data derived from NHANES between 2005 and 2018 (seven NHANES cycles) were analyzed, given that participants underwent relatively comprehensive urine testing for heavy metals during these cycles. NHANES was approved by the Ethics Review Committee at the National Center for Health Statistics, and all participants provided informed consent. Among the 70,190 participants across the NHANES cycles conducted between 2005 and 2018, several groups were excluded according to the following criteria: (1) participants who were pregnant or lacked data on diabetes (n = 3,744); (2) participants with incomplete urinary metal levels (n = 48,050); (3) participants who had missing covariate data (n = 8,806); (4) non-diabetic individuals (n = 7,852); and (5) participants with other missing information on diabetic retinopathy (n = 592). The final study cohort comprised 1,146 subjects, as illustrated in Figure 1.
Diagnostic criteria for diabetes comprised any of the following: (1) diagnosis by medical professionals during a non-gestational period, (2) hemoglobin A1c level (HbA1c) (%) ≥ 6.5, (3) fasting plasma glucose level (FPG) ≥ 7.0 mmol/L, (4) random blood glucose level ≥ 11.1 mmol/L, (5) 2-h oral glucose tolerance test (OGTT) blood glucose ≥11.1 mmol/L, and (6) receiving anti-diabetic medication (23). DR was determined through self-report using a dichotomous approach. Participants were informed by medical professionals that diabetes had affected their eyes (24).
Between 2005 and 2018, the levels of 10 heavy metals, namely Ba, Cd, Co, Cs, Mo, Pb, Sb, Tl, Tu, and Hg, were detected in urine samples. The NHANES website provides all laboratory methods and quality control information. Briefly, the concentration of 10 urinary metals was determined using inductively coupled plasma-mass spectrometry (ICP-MS). If the metal concentration was below the limit of detection (LOD), the LOD divided by the square root of two was used as the surrogate. In addition, all urinary metal levels were normalized to urinary creatinine and reported as μg/g creatinine (25).
Demographic characteristics [gender, age, ethnicity, educational background, and family poverty income ratio (PIR)], along with data on body mass index (BMI), HbA1c levels, history of hypertension and chronic kidney disease (CKD), smoking status, and drinking habits, were acquired through either home interviews or laboratory assessments. Ethnicity was classified into five groups: non-Hispanic white, non-Hispanic black, Mexican American, other Hispanic, and other race/multiracial. PIR was categorized into three levels: <1.30, 1.30–3.5, and ≥ 3.5 (21). Similarly, BMI was divided into three levels: <25, 25–30, and > 30 kg/m2. Glycemic control was classified as well (HbA1c < 7%) and poor (HbA1c ≥7%). Drinking status was self-reported by the participants. Smoking status was determined through the evaluation of serum cotinine levels, with a cutoff value of ≤0.011 ng/mL for nonsmokers and higher levels indicating smoking status for both active and second-hand smokers (26). Hypertension was defined as any of the following: self-reported hypertension, ever or currently taking anti-hypertensive drugs, a systolic blood pressure over 140 mmHg, or a diastolic blood pressure exceeding 90 mmHg. CKD was defined as any of the following: an estimated glomerular filtration rate (eGFR) < 60 mL/min/1.73 m2 or the presence of elevated albuminuria (urine albumin creatinine ratio ≥ 30 mg/g) (27).
WTSA2YR is considered the appropriate NHANES sampling weight to analyze data on urinary heavy metals. Given the complex sampling design of NHANES, weights (1/7 * WTSA2YR) were constructed in accordance with the analytic guidelines of NHANES. Weighted means (standard errors) were employed to present continuous variables, while unweighted frequencies (weighted percentages) were utilized to present categorical variables. Baseline comparisons were made based on DR status stratification. The t-test was used to compare continuous variables, whereas the chi-square test was used to compare categorical variables. Given the upward trend in heavy metal concentration in the human body, an Ln transformation was performed on heavy metal concentration data to approximate a normal distribution (continuous variable) and divided the heavy metal concentration data into quartiles. The relationships between the concentrations of the 10 metals were determined using Pearson correlation analysis.
First and foremost, weighted logistic regression was employed to explore the impact of each metal on the risk of DR. The reference group was set as the first quartile (Q1), and the results were expressed as odds ratios (ORs) and their corresponding 95% confidence intervals (CIs). All covariates, including age, gender, ethnicity, educational background, PIR, glycemic control, smoking and drinking status, BMI, hypertension, and CKD, were adjusted. Furthermore, a weighted logistic regression analysis encompassing all heavy metals was conducted to adjust for the effects of other metals.
Secondly, to assess the combined effect of exposure to multiple metals on DR risk, a WQS regression analysis was carried out. This method was selected owing to its effective characterization of environmental mixtures (28). The R package (“gWQS”) was utilized to compute the WQS index, which is a weighted sum of the concentrations of individual heavy metals (21). The WQS index (ranging from 0 to 1) indicated the level of mixed exposure to the 10 heavy metals. The weight of each metal reflected its relative importance for the risk of DR. The WQS analysis results provided information about the concurrent influence of adding a quartile to heavy metals mixtures on DR risk.
BKMR is a developing statistical method that utilizes kernel functions to effectively model the individual and joint impacts of mixture exposure on health results (29). The common influences of heavy metal mixtures on DR were examined by analyzing the DR estimates for every 5 percent increase/decrease in the median concentration of metal mixtures (reference) (25). The posterior probability of inclusion (PIP) was calculated with a threshold of 0.5 to assess the relative contribution of each metal component to the outcome (30). The BKMR model was generated via the R package “bkmr” through 10,000 iterations (31).
Subsequently, an RCS regression analysis was conducted using the R package “rms” to investigate the dose–response association of heavy metal exposure with DR risk. RCS regression was used to analyze both the linear and nonlinear relationships between heavy metals levels and DR risk (32). The number of nodes was selected to maintain the best fit and prevent overfitting the principal spline, with a range of 3–7 nodes considered according to the minimum absolute value of Akaike’s information criterion (33). Finally, the 3 knots corresponding to the 10th, 50th, and 90th percentiles were chosen.
Finally, the same statistical analysis procedures previously outlined were applied to the subgroups based on glycemic control (well-controlled group: HbA1c value <7%, poorly-controlled group: HbA1c value ≥7%).
Statistical analyses were performed using R software (v4.3.1), with p-values less than 0.05 considered statistically significant.
This study included 1,146 participants from seven NHANES cycles, comprising 550 women (weighted survey sample of 7,898,021) and 596 men (weighted survey sample of 8,162,870). Among them, 239 (20.86%) were diagnosed with DR, including 134 males and 105 females. Table 1 presents a summary of the baseline characteristics of the study participants with and without DR. Consistent with the findings of previously published studies, our study confirmed that participants with DR exhibited poorer glycemic control than those with diabetes without DR. Furthermore, the prevalence of CKD was higher in individuals with DR compared to those without DR. The two groups were comparable in age, gender, ethnicity, educational background, PIR, BMI, drinking and smoking status, and prevalence of hypertension.
Supplementary Table 1 lists the distribution of concentrations for 10 heavy metals, with detection rates exceeding 93.0% for each metal. Interestingly, Mo was the most metal with the highest level. Additionally, patients with DR had significantly higher levels of Sb compared to those without DR (p = 0.021). The correlations between the 10 heavy metals are detailed in Supplementary Figure 1. Co and Tl (r = 0.58), Co and Ba (r = 0.43), Cs and Ba (r = 0.35), Co and Cs (r = 0.34), Tu and Mo (r = 0.34), and Tl and Ba (r = 0.31) exhibited positive correlations. Other metals had relatively weak correlations.
As displayed in Table 2, weighted logistic regression was applied to analyze the association between each metal and DR risk after adjusting for all covariates. When considering the concentrations of Co and Sb as continuous variables, an increase of one unit in Ln-Co and Ln-Sb concentrations resulted in a 48.9 and 47.5% increase in the risk of DR, respectively (all p < 0.05). In addition, a positive correlation was observed between Sb and DR when metal concentrations were divided into quartiles (p for trend = 0.036). Notably, there was a significantly positive correlation found between DR risk and Hg concentration in the third quartile (Q3) (OR = 2.322, 95% CI: 1.158–4.655, p = 0.018), whereas no significant correlation was detected for concentrations in the highest quartile (Q4).
Subgroup analysis based on the level of glycemic control was also performed (Table 2). In the poorly-controlled group, Sb exerted the most significant effect (OR = 1.596, 95% CI: 1.022–2.493, p = 0.04), but this relationship was not significantly different from that in the well-controlled group. In the well-controlled group, Ba concentration in Q3 (OR = 0.274, 95% CI: 0.120–0.627, p = 0.003) was significantly and negatively correlated with DR risk, but Ba concentration in Q4 did not. Both Hg concentrations in Q4 and Ln-Hg significantly increased the risk of DR (OR = 3.608, 95% CI: 1.695–7.681, p = 0.001; OR = 1.509, 95% CI: 1.157–1.967, p = 0.003) in the well-controlled group. Other metals have not been shown to have a meaningful association with DR.
To account for the potential influence of other heavy metals, weighted logistic regression models that considered all heavy metals were applied. As demonstrated in Supplementary Table 2, Hg concentration in Q3 significantly increased the risk of DR (OR = 2.407, 95% CI: 1.264–4.585, p = 0.008). Additionally, each per-unit increase in Ln-Co and Ln-Sb concentrations led to a 62.7 and 42.7% higher risk of DR, respectively (all p < 0.05).
WQS regression was conducted to investigate the correlation between heavy metal mixtures and DR risk while adjusting for all covariates. In our study, the WQS index was positively correlated with DR risk (OR = 1.5, 95%CI: 1.07–2.10, p = 0.019). In the subgroup analysis stratified by glycemic control, the correlation between exposure to heavy metals and DR risk was not statistically significant in either the well-controlled or poorly-controlled group (all p > 0.05). Among the 10 heavy metals, Pb, Mo, Hg, Sb, and Co exhibited the highest weight in the whole population (Figure 2A). In the well-controlled group, Sb was determined to be the highest weighted metal (Figure 2B), whereas Cs and Co were the most heavily weighted metals in the poorly-controlled group (Figure 2C).
Figure 2. Estimated weights of heavy metals for DR by WQS models were adjusted for age, sex, ethnicity, education, poverty income ratio, body mass index, drinking alcohol status, smoking status, glycemic control, hypertension and CKD. (A) total population, (B) good glycemic control group, (C) poor glycemic control group. Ba, Barium; Cd, Cadmium; Co, Cobalt; Cs, Cesium; Mo, Molybdenum; Pb, lead; Sb, Antimony; Tl, Thallium; Tu, Tungsten; lead, Pb; mercury, Hg.
Although no statistically significant effect was observed, there was a discernible increase in the risk of DR when heavy metal mixture concentrations were at or exceeded the 60th percentile (Figure 3A). Similar associations were observed in both the well-controlled and poorly-controlled groups, as depicted in Figure 3A. When concentrations of other metals were fixed at the 25th, 50th, and 75th percentiles, Co, Mo, Sb, Tu, Pb, and Hg concentrations all displayed a positive correlation with DR risk, with PIP values exceeding 0.55 (Figure 3B and Supplementary Table 3). Similar trends were observed in both the well-controlled and poorly-controlled groups, although the correlations were not statistically significant, as delineated in Figure 3B. The univariate exposure-response relationship exhibited a monotonic upward trend between DR and Co, Sb, Tu, and Pb concentrations when the other metals were fixed at the median level. However, Ba, Tl, and Cd displayed a monotonic downward trend (Supplementary Figure 2). Based on the moderate correlations between some metals, the interactions among the 10 heavy metals were separately analyzed, revealing underlying interactions between specific heavy metals. Supplementary Figure 3 delineates that Co interacts with Cs, Sb, Tl, Hg, and Pb, whilst Sb interacts with Tu, Cd, and Hg, and Hg interacts with most metals.
Figure 3. The associations of metal mixtures and DR risk evaluated by BKMR model. (A) The joint effects of heavy metal mixtures on DR risk were estimated by BKMR models in total population and subgroups, when all the metals at particular percentiles were compared to all the metals at their 50th percentile. (B) Associations of single heavy metals with DR risk were estimated by BKMR models in total population and subgroups, when other all metals were held at their corresponding 25th (red), 50th (green) or 75th (blue) percentile, respectively. Models were adjusted for age, sex, ethnicity, education, poverty income ratio, body mass index, drinking alcohol status, smoking status, glycemic control, hypertension and CKD. Ba, Barium; Cd, Cadmium; Co, Cobalt; Cs, Cesium; Mo, Molybdenum; Pb, lead; Sb, Antimony; Tl, Thallium; Tu, Tungsten; lead, Pb; mercury, Hg.
Co, Sb, and Hg concentrations, which were closely related to DR risk, were further analyzed. The dose–response relationships were evaluated in the RCS analysis (Figure 4). Linear and positive associations with DR risk were identified for the Ln-transformed concentrations of Co and Sb (all pnonlinearity > 0.05, all poverall < 0.05), except for Hg (pnonlinearity = 7e-04). In both the well-controlled and poorly-controlled groups, Co and Sb concentrations had a linear relationship with DR risk. In the well-controlled group, the risk of DR generally increased with increasing Co concentration (poverall = 0.011, pnonlinearity = 0.262). Conversely, in the poorly-controlled group, a positive linear dose correlation between Sb concentrations and DR risk was noted (poverall = 0.045, pnonlinearity = 0.790). Finally, a linear and positive correlation between Hg concentrations and the risk of DR was observed solely in the well-controlled group (poverall = 0.01, pnonlinearity = 0.385).
Figure 4. Dose–response relationship between Sb, Co and Hg with DR risk were estimated by RCS models in total population and subgroups. Models were adjusted for age, sex, ethnicity, education, poverty income ratio, body mass index, drinking alcohol status, smoking status, glycemic control, hypertension and CKD. (A) total population, (B) good glycemic control group, (C) poor glycemic control group. Solid line, odds ratios; red-shade, 95% CI.
To the best of our knowledge, this is the first cross-sectional study to investigate the effects of urinary heavy metals on the risk of DR in a substantial, nationally representative sample utilizing various statistical techniques. Herein, the results of weighted logistic regression demonstrated that Co, Sb, and Hg were associated with DR risk in the single-metal model, and this association was also observed in the multi-metal model. Both the WQS and BMKR models suggested that mixed exposure to these 10 heavy metals was positively associated with DR risk. Furthermore, the results of the RCS regression displayed a linear and positive correlation between Co and Sb and DR risk but a non-linear correlation between Hg concentrations and DR risk. The results of the subgroup analyses signaled that the aforementioned associations appeared to be more pronounced in the poorly-controlled group.
Co is widely distributed in nature. Humans are commonly exposed to Co through multiple routes, including food, environmental pollution, occupational exposure, and medical interventions (34). Besides, it is an essential element for human health, serving as the metallic component of vitamin B12 (35). Despite its vital importance, its potential toxicity can elicit adverse health effects after prolonged exposure. A cross-sectional study identified a positive correlation between diabetes and urinary Co concentrations (16). Consistently, a study discovered a strong correlation between elevated urinary Co levels and increased levels of FPG and HbA1c in male participants (36). At the same time, Cancarini et al. concluded that the Co concentration in the tear film of diabetic patients was higher than that in the control group (37). This increase may be attributed to the rise in conjunctival vascular permeability caused by diabetes, similar to the increase in retinal vascular permeability driven by diabetes (a characteristic of DR) (38). In our study, diabetic patients with higher urinary concentrations of Co were more likely to develop DR. This may be ascribed to the oxidizing effect of Co promoting the formation of free radicals, inducing oxidative stress responses, and contributing to mitochondrial dysfunction (39). Of note, accumulating evidence suggests that oxidative damage and mitochondrial dysfunction promote the development of DR (40, 41).
Sb is a toxic heavy metal to which humans are primarily exposed through the consumption of food and air, soil, and water exposure. Numerous studies have demonstrated that it exerts various toxic effects on vital organs, including but not limited to the pancreas, liver, lungs, intestines, and spleen (42). A cross-sectional study conducted in the USA demonstrated an association between urine Sb concentrations and insulin resistance (16). Likewise, a cross-sectional study conducted in China found that urinary Sb levels are linked to an increased risk of increased FPG levels, impaired fasting glucose, and diabetes (18). Furthermore, a prospective study indicated that pregnant women with higher exposure to Sb may face an increased risk of gestational diabetes mellitus (43). Xiao et al. reported that elevated urinary Sb concentrations are linked to a higher incidence of type 2 diabetes, and this process is partially implicated in oxidative DNA damage (44). These studies collectively imply that Sb exposure may contribute to the development of diabetes. However, to date, there has been no report on the correlation between Sb levels and DR. Our study uncovered that diabetic patients with elevated urinary Sb levels have a significantly increased risk of developing DR, especially in those with poor glycemic control.
Hg is a highly toxic heavy metal that can cause significant harm to numerous organs in the human body (45). Currently, research on the relationship between Hg levels and diabetes risk remains inconclusive. Earlier studies found no significant association between blood or urine Hg concentrations and an increased risk of diabetes in adults (46–48). However, Tsai et al. observed a significant increase in Hg levels in the red blood cells of type 2 diabetes patients compared to those without the condition (49). A large prospective cohort study determined that people with high Hg exposure during early adulthood were at a higher risk of developing diabetes in the future (50). Furthermore, research has demonstrated that Hg can selectively affect β cells in the pancreas, resulting in cellular dysfunction and apoptosis (51). In this study, urine Hg levels among diabetes patients with DR were higher compared to those without DR, although the difference was not statistically significant. Additionally, a clear non-monotonic relationship was identified between Hg levels and the risk of DR. This may be due to the fact that the chief source of human exposure to Hg is the consumption of marine fish, which are rich in omega-3 fatty acids that counteract the toxicity of Hg (52, 53). A significant positive correlation was observed between Hg concentrations and DR risk only in the well-controlled group, warranting further investigation.
Heavy metals stimulate reactive oxygen species production, leading to oxidative damage, which is one of the mechanisms involved in disease development (54). The retina is a high-oxygen-consuming tissue that is highly susceptible to damage from oxidative stress. Previous studies have shown a robust correlation between oxidative stress and retinal vascular impairment under hyperglycemic conditions (55). However, the role of heavy metals in DR development via oxidative stress mechanisms remains unclear. Thus, further experimental validation is necessary.
This study has several advantages. Firstly, it is the first study that investigated the correlation between urinary heavy metals and DR risk, considering both the single and co-exposure effects of heavy metals. In contrast, Zhang et al. focused on the relationship between blood heavy metals and DR risk without exploring the combined effects of heavy metals on DR (15). Furthermore, our study included a higher number of metals than those conducted by Zhang et al. (15) and reported for the first time that urinary levels of Co, Sb, and Hg may be associated with DR risk. Secondly, weighted logistic regression, WQS regression model, BMKR model, and RCS regression were employed to investigate the correlation between heavy metals and DR risk in a diabetes population from multiple perspectives. These statistical methods have been extensively utilized to explore the effects of heavy metals on diabetes and hypertension (21, 56). Finally, previous research has demonstrated a correlation between heavy metal exposure and HbA1c levels. High HbA1c level has been established as a risk factor for DR. Therefore, subgroup analysis was initially conducted based on glycemic control (determined by HbA1c value) to investigate the correlation between urine levels of heavy metals and DR risk.
Nevertheless, some limitations of this study merit acknowledgment. Given the inherent shortcomings of cross-sectional studies (57), this study could not establish a causal relationship between metal exposure and the risk of DR. Furthermore, relying on self-report questionnaires for DR diagnosis may introduce recall bias. In addition, the dataset lacked precise information regarding retinopathy severity, thereby limiting further analysis. The concentrations of heavy metals in urine are affected by various factors, not all of which were accounted for in this study, potentially compromising the reliability of the results. Additionally, selection bias selection bias may be present due to missing data and the exclusion of participants with incomplete information. Therefore, further studies are necessitated to corroborate our findings and to investigate the relationship between metal concentrations and DR severity, as well as to elucidate the underlying mechanisms by which metals affect DR.
Overall, our cross-sectional study demonstrated that several heavy metals, including Co, Sb, and Hg, were significantly associated with an elevated risk of DR. Furthermore, a linear and positive correlation was observed between the concentrations of Co and Sb and the risk of DR, while a non-linear correlation was identified between Hg levels and DR risk. The results of the subgroup analyses signaled that the aforementioned associations appeared to be more pronounced in the poorly-controlled group. The results of the mixture exposure analysis indicated a positive association between mixed metal exposure and the risk of DR. This association was observed in both the well-controlled group and the poorly-controlled group. Due to the limitations of the present study, subsequent investigations are required to substantiate these findings and to clarify the mechanisms by which heavy metals affect DR.
The original contributions presented in the study are included in the article/Supplementary material, further inquiries can be directed to the corresponding author.
CM: Conceptualization, Formal analysis, Methodology, Writing – original draft, Writing – review & editing. CG: Data curation, Formal analysis, Methodology, Writing – original draft, Writing – review & editing. CC: Formal analysis, Methodology, Writing – review & editing. SH: Formal analysis, Methodology, Writing – original draft. DL: Data curation, Methodology, Writing – original draft. QQ: Conceptualization, Supervision, Writing – review & editing.
The author(s) declare that financial support was received for the research, authorship, and/or publication of this article. This work has been supported by the National Natural Science Foundation of China (82371072).
The authors declare that the research was conducted in the absence of any commercial or financial relationships that could be construed as a potential conflict of interest.
All claims expressed in this article are solely those of the authors and do not necessarily represent those of their affiliated organizations, or those of the publisher, the editors and the reviewers. Any product that may be evaluated in this article, or claim that may be made by its manufacturer, is not guaranteed or endorsed by the publisher.
The Supplementary material for this article can be found online at: https://www.frontiersin.org/articles/10.3389/fpubh.2024.1401034/full#supplementary-material
1. Yau, JW, Rogers, SL, Kawasaki, R, Lamoureux, EL, Kowalski, JW, Bek, T, et al. Global prevalence and major risk factors of diabetic retinopathy. Diabetes Care. (2012) 35:556–64. doi: 10.2337/dc11-1909
2. Zhou, C, Li, S, Ye, L, Chen, C, Liu, S, Yang, H, et al. Visual impairment and blindness caused by retinal diseases: a nationwide register-based study. J Glob Health. (2023) 13:04126. doi: 10.7189/jogh.13.04126
3. Marques, AP, Ramke, J, Cairns, J, Butt, T, Zhang, JH, Jones, I, et al. The economics of vision impairment and its leading causes: a systematic review. EClinicalMedicine. (2022) 46:101354. doi: 10.1016/j.eclinm.2022.101354
4. Li, H, Liu, X, Zhong, H, Fang, J, Li, X, Shi, R, et al. Research progress on the pathogenesis of diabetic retinopathy. BMC Ophthalmol. (2023) 23:372. doi: 10.1186/s12886-023-03118-6
5. Wang, X, Mukherjee, B, and Park, SK. Associations of cumulative exposure to heavy metal mixtures with obesity and its comorbidities among U.S. adults in NHANES 2003-2014. Environ Int. (2018) 121:683–94. doi: 10.1016/j.envint.2018.09.035
6. Fatma, UK, Nizami, G, Ahamad, S, Saquib, M, and Hussain, MK. Activated green tamarind pulp (Agtp) as an efficient adsorbent for removal of Pb2+, Cu2+, Zn2+ & Ni2+ from contaminated water. J Water Proc Eng. (2024) 59:105048. doi: 10.1016/j.jwpe.2024.105048
7. Fatma, UK, Nizami, G, Ahamad, S, and Hussain, MK. Efficient removal of Pb2+, Cu2+ and Zn2+ by waste tea-derived cost-effective bioadsorbent. ChemistrySelect. (2023) 8:e202300944. doi: 10.1002/slct.202300944
8. Alloway, BJ. Sources of heavy metals and metalloids in soils In: BJ Alloway, editor. Heavy metals in soils: trace metals and metalloids in soils and their bioavailability. Dordrecht: Springer Netherlands (2013). 11–50.
9. Bosch, AC, O'Neill, B, Sigge, GO, Kerwath, SE, and Hoffman, LC. Heavy metals in marine fish meat and consumer health: a review. J Sci Food Agric. (2016) 96:32–48. doi: 10.1002/jsfa.7360
10. Zhu, X, and Hua, R. Serum essential trace elements and toxic metals in Chinese diabetic re tinopathy patients. Medicine. (2020) 99:e23141. doi: 10.1097/MD.0000000000023141
11. Park, SJ, Lee, JH, Woo, SJ, Kang, SW, and Park, KHEpidemiologic Survey Committee of Korean Ophthalmologic Society. Five heavy metallic elements and age-related macular degeneration: Korean National Health and Nutrition Examination Survey, 2008-2011. Ophthalmology. (2015) 122:129–37. doi: 10.1016/j.ophtha.2014.07.039
12. Vennam, S, Georgoulas, S, Khawaja, A, Chua, S, Strouthidis, NG, and Foster, PJ. Heavy metal toxicity and the aetiology of Glaucoma. Eye. (2020) 34:129–37. doi: 10.1038/s41433-019-0672-z
13. Wang, W, Schaumberg, DA, and Park, SK. Cadmium and lead exposure and risk of cataract surgery in U.S. adults. Int J Hyg Environ Health. (2016) 219:850–6. doi: 10.1016/j.ijheh.2016.07.012
14. Li, Y-Q, Zhang, S-T, Ke, N-Y, Fang, Y-C, Hu, W-L, Li, G-A, et al. The impact of multiple metals exposure on the risk of developing proli ferative diabetic retinopathy in Anhui, China: a case-control study. Environ Sci Pollut Res Int. (2023) 30:112132–43. doi: 10.1007/s11356-023-30294-1
15. Zhang, Y, Liu, X, Zhang, X, Li, L, Li, Q, Geng, H, et al. Association between serum heavy metal levels and diabetic retinopathy in NHANES 2011-2020. Sci Rep. (2024) 14:1268. doi: 10.1038/s41598-024-51749-6
16. Menke, A, Guallar, E, and Cowie, CC. Metals in urine and diabetes in U.S. adults. Diabetes. (2016) 65:164–71. doi: 10.2337/db15-0316
17. Hendryx, M, Luo, J, Chojenta, C, and Byles, JE. Exposure to heavy metals from point pollution sources and risk of incident type 2 diabetes among women: a prospective cohort analysis. Int J Environ Health Res. (2021) 31:453–64. doi: 10.1080/09603123.2019.1668545
18. Feng, W, Cui, X, Liu, B, Liu, C, Xiao, Y, Lu, W, et al. Association of urinary metal profiles with altered glucose levels and diabetes risk: a population-based study in China. PLoS One. (2015) 10:e0123742. doi: 10.1371/journal.pone.0123742
19. Wilhelm, M, Müller, F, and Idel, H. Biological monitoring of mercury vapour exposure by scalp hair analysis in comparison to blood and urine. Toxicol Lett. (1996) 88:221–6. doi: 10.1016/0378-4274(96)03741-1
20. Chen, L, Sun, Q, Peng, S, Tan, T, Mei, G, Chen, H, et al. Associations of blood and urinary heavy metals with rheumatoid arthritis risk among adults in NHANES, 1999-2018. Chemosphere. (2022) 289:133147. doi: 10.1016/j.chemosphere.2021.133147
21. Wu, Z, Guan, T, Cai, D, and Su, G. Exposure to multiple metals in adults and diabetes mellitus: a cross-sectional analysis. Environ Geochem Health. (2023) 45:3251–61. doi: 10.1007/s10653-022-01411-9
22. Kim, D, Lee, S, Choi, J-Y, Lee, J, Lee, H-J, Min, J-Y, et al. Association of Α-klotho and lead and cadmium: a cross-sectional study. Sci Total Environ. (2022) 843:156938. doi: 10.1016/j.scitotenv.2022.156938
23. American Diabetes Association. 2. Classification and diagnosis of diabetes: standards of medical care in diabetes—2018. Diabetes Care. (2018, 2018) 41:S13–27. doi: 10.2337/dc18-S002
24. Xu, H, Dong, X, Wang, J, Cheng, X, Qu, S, Jia, T, et al. Association of calcium, magnesium, zinc, and copper intakes with diabetic retinopathy in diabetics: National Health and Nutrition Examination Survey, 2007–2018. Curr Eye Res. (2023, 2023) 48:485–91. doi: 10.1080/02713683.2023.2165105
25. Chen, Y, Pan, Z, Shen, J, Wu, Y, Fang, L, Xu, S, et al. Associations of exposure to blood and urinary heavy metal mixtures wit H psoriasis risk among U.S. adults: a cross-sectional study. Sci Total Environ. (2023) 887:164133. doi: 10.1016/j.scitotenv.2023.164133
26. Xu, C, Liang, J, Xu, S, Liu, Q, Xu, J, and Gu, A. Increased serum levels of aldehydes are associated with cardiovascular disease and cardiovascular risk factors in adults. J Hazard Mater. (2020) 400:123134. doi: 10.1016/j.jhazmat.2020.123134
27. Webster, AC, Nagler, EV, Morton, RL, and Masson, P. Chronic kidney disease. Lancet. (2017) 389:1238–52. doi: 10.1016/s0140-6736(16)32064-5
28. Carrico, C, Gennings, C, Wheeler, DC, and Factor-Litvak, P. Characterization of weighted quantile sum regression for highly correlated data in a risk analysis setting. J Agric Biol Environ Stat. (2015) 20:100–20. doi: 10.1007/s13253-014-0180-3
29. Bobb, JF, Valeri, L, Claus Henn, B, Christiani, DC, Wright, RO, Mazumdar, M, et al. Bayesian kernel machine regression for estimating the health effects of multi-pollutant mixtures. Biostatistics. (2015) 16:493–508. doi: 10.1093/biostatistics/kxu058
30. Ma, Y, Hu, Q, Yang, D, Zhao, Y, Bai, J, Mubarik, S, et al. Combined exposure to multiple metals on serum uric acid in NHANES under three statistical models. Chemosphere. (2022) 301:134416. doi: 10.1016/j.chemosphere.2022.134416
31. Weng, X, Tan, Y, Fei, Q, Yao, H, Fu, Y, Wu, X, et al. Association between mixed exposure of phthalates and cognitive function among the U.S. elderly from NHANES 2011-2014: three statistical models. Sci Total Environ. (2022) 828:154362. doi: 10.1016/j.scitotenv.2022.154362
32. Desquilbet, L, and Mariotti, F. Dose-response analyses using restricted cubic spline functions in public health research. Stat Med. (2010) 29:1037–57. doi: 10.1002/sim.3841
33. Johannesen, CDL, Langsted, A, Mortensen, MB, and Nordestgaard, BG. Association between low density lipoprotein and all cause and cause specific mortality in Denmark: prospective cohort study. BMJ. (2020) 371:m4266. doi: 10.1136/bmj.m4266
34. Tvermoes, BE, Unice, KM, Paustenbach, DJ, Finley, BL, Otani, JM, and Galbraith, DA. Effects and blood concentrations of cobalt after ingestion of 1 mg/d by human volunteers for 90 d. Am J Clin Nutr. (2014) 99:632–46. doi: 10.3945/ajcn.113.071449
35. Reynolds, E. Vitamin B12, folic acid, and the nervous system. Lancet Neurol. (2006) 5:949–60. doi: 10.1016/S1474-4422(06)70598-1
36. Yang, J, Lu, Y, Bai, Y, and Cheng, Z. Sex‐specific and dose‐response relationships of urinary cobalt and molybdenum levels with glucose levels and insulin resistance in U.S. adults. J Environ Sci. (2023) 124:42–9. doi: 10.1016/j.jes.2021.10.023
37. Cancarini, A, Fostinelli, J, Napoli, L, Gilberti, ME, Apostoli, P, and Semeraro, F. Trace elements and diabetes: assessment of levels in tears and serum. Exp Eye Res. (2017) 154:47–52. doi: 10.1016/j.exer.2016.10.020
38. Rudraraju, M, Narayanan, SP, and Somanath, PR. Regulation of blood-retinal barrier cell-junctions in diabetic retinopathy. Pharmacol Res. (2020) 161:105115. doi: 10.1016/j.phrs.2020.105115
39. Karovic, O, Tonazzini, I, Rebola, N, Edström, E, Lövdahl, C, Fredholm, BB, et al. Toxic effects of cobalt in primary cultures of mouse astrocytes. Biochem Pharmacol. (2007) 73:694–708. doi: 10.1016/j.bcp.2006.11.008
40. Kang, Q, and Yang, C. Oxidative stress and diabetic retinopathy: molecular mechanisms, pathogenetic role and therapeutic implications. Redox Biol. (2020) 37:101799. doi: 10.1016/j.redox.2020.101799
41. Wu, M-Y, Yiang, G-T, Lai, T-T, and Li, C-J. The oxidative stress and mitochondrial dysfunction during the pathogenesis of diabetic retinopathy. Oxidative Med Cell Longev. (2018) 2018:3420187. doi: 10.1155/2018/3420187
42. Coelho, DR, Miranda, ES, Saint?Pierre, TD, and Paumgartten, FJR. Tissue distribution of residual antimony in rats treated with multiple doses of meglumine antimoniate. Mem Inst Oswaldo Cruz. (2014) 109:420–7. doi: 10.1590/0074-0276140030
43. Zhang, G, Wang, X, Zhang, X, Li, Q, Xu, S, Huang, L, et al. Antimony in urine during early pregnancy correlates with increased risk of gestational diabetes mellitus: a prospective cohort study. Environ Int. (2019) 123:164–70. doi: 10.1016/j.envint.2018.11.072
44. Xiao, L, Zhou, Y, Ma, J, Sun, W, Cao, L, Wang, B, et al. Oxidative DNA damage mediates the association between urinary metals and prevalence of type 2 diabetes mellitus in Chinese adults. Sci Total Environ. (2018) 627:1327–33. doi: 10.1016/j.scitotenv.2018.01.317
45. Rice, KM, Walker, EM Jr, Wu, M, Gillette, C, and Blough, ER. Environmental mercury and its toxic effects. J Prev Med Public Health. (2014) 47:74–83. doi: 10.3961/jpmph.2014.47.2.74
46. Moon, MK, Lee, I, Lee, A, Park, H, Kim, MJ, Kim, S, et al. Lead, mercury, and cadmium exposures are associated with obesity but not with diabetes mellitus: Korean National Environmental Health Survey (KONEHS) 2015-2017. Environ Res. (2022) 204:111888. doi: 10.1016/j.envres.2021.111888
47. Ghorbani Nejad, B, Raeisi, T, Janmohammadi, P, Mehravar, F, Zarei, M, Dehghani, A, et al. Mercury exposure and risk of type 2 diabetes: a systematic review and meta-analysis. Int J Clin Pract. (2022) 2022:1–13. doi: 10.1155/2022/7640227
48. Moon, SS. Association of lead, mercury and cadmium with diabetes in the Korean population: the Korea National Health and Nutrition Examination Survey (KNHANES) 2009-2010. Diabet Med. (2013) 30:e143–8. doi: 10.1111/dme.12103
49. Tsai, T-L, Kuo, C-C, Pan, W-H, Wu, T-N, Lin, P, and Wang, S-L. Type 2 diabetes occurrence and mercury exposure – from the National Nutrition and Health Survey in Taiwan. Environ Int. (2019) 126:260–7. doi: 10.1016/j.envint.2019.02.038
50. He, K, Xun, P, Liu, K, Morris, S, Reis, J, and Guallar, E. Mercury exposure in young adulthood and incidence of diabetes later in life: the cardia trace element study. Diabetes Care. (2013) 36:1584–9. doi: 10.2337/dc12-1842
51. Yang, C-Y, Liu, S-H, Su, C-C, Fang, K-M, Yang, T-Y, Liu, J-M, et al. Methylmercury induces mitochondria-and endoplasmic reticulum stress-D ependent pancreatic Β-cell apoptosis via an oxidative stress-mediated JNK signaling pathway. Int J Mol Sci. (2022) 23:2858. doi: 10.3390/ijms23052858
52. Karapehlivan, M, Ogun, M, Kaya, I, Ozen, H, Deveci, HA, and Karaman, M. Protective effect of omega-3 fatty acid against mercury chloride intoxication in mice. J Trace Elem Med Biol. (2014) 28:94–9. doi: 10.1016/j.jtemb.2013.08.004
53. Smith, KL, and Guentzel, JL. Mercury concentrations and omega-3 fatty acids in fish and shrimp: preferential consumption for maximum health benefits. Mar Pollut Bull. (2010) 60:1615–8. doi: 10.1016/j.marpolbul.2010.06.045
54. Rehman, K, Fatima, F, Waheed, I, and Akash, MSH. Prevalence of exposure of heavy metals and their impact on health consequences. J Cell Biochem. (2018) 119:157–84. doi: 10.1002/jcb.26234
55. Kruk, J, Kubasik-Kladna, K, and Aboul-Enein, HY. The role oxidative stress in the pathogenesis of eye diseases: current status and a dual role of physical activity. Mini Rev Med Chem. (2015) 16:241–57. doi: 10.2174/1389557516666151120114605
56. Zhao, S, Fan, L, Wang, Y, Dong, S, Han, M, Qin, Y, et al. Combined exposure to multiple metals on hypertension in NHANES under four statistical models. Environ Sci Pollut Res Int. (2023) 30:92937–49. doi: 10.1007/s11356-023-28902-1
Keywords: heavy metal exposure, diabetic retinopathy, public health, NHANES, risk factors
Citation: Meng C, Gu C, Cai C, He S, Lai D and Qiu Q (2024) Associations of heavy metal exposure with diabetic retinopathy in the U.S. diabetic population: a cross-sectional study. Front. Public Health. 12:1401034. doi: 10.3389/fpubh.2024.1401034
Received: 14 March 2024; Accepted: 18 July 2024;
Published: 01 August 2024.
Edited by:
Yizhong Yan, Shihezi University, ChinaReviewed by:
Rossanna Rodriguez-Canul, Center for Research and Advanced Studies – Mérida Unit, MexicoCopyright © 2024 Meng, Gu, Cai, He, Lai and Qiu. This is an open-access article distributed under the terms of the Creative Commons Attribution License (CC BY). The use, distribution or reproduction in other forums is permitted, provided the original author(s) and the copyright owner(s) are credited and that the original publication in this journal is cited, in accordance with accepted academic practice. No use, distribution or reproduction is permitted which does not comply with these terms.
*Correspondence: Qinghua Qiu, cWluZ2h1YXFpdUAxNjMuY29t
†These authors have contributed equally to this work
Disclaimer: All claims expressed in this article are solely those of the authors and do not necessarily represent those of their affiliated organizations, or those of the publisher, the editors and the reviewers. Any product that may be evaluated in this article or claim that may be made by its manufacturer is not guaranteed or endorsed by the publisher.
Research integrity at Frontiers
Learn more about the work of our research integrity team to safeguard the quality of each article we publish.