- Department of Epidemiology and Health Statistics, West China School of Public Health and West China Fourth Hospital, Sichuan University, Chengdu, China
Introduction: Premature death is a global health indicator, significantly impacted by obesity, especially in young and middle-aged population. Both body mass index (BMI) and waist circumference (WC) assess obesity, with WC specifically indicating central obesity and showing a stronger relationship with mortality. However, despite known associations between BMI and premature death, as well as the well-recognized correlation between WC and adverse health outcomes, the specific relationship between WC and premature death remains unclear. Therefore, focusing on young and middle-aged individuals, this study aimed to reliably estimate independent and combined associations between WC, BMI and premature death, thereby providing causal evidence to support strategies for obesity management.
Methods: This study involved 49,217 subjects aged 18–50 years in the United States from 1999 to 2018 National Health and Nutrition Examination Survey (NHANES). Independent and combined associations between WC and BMI with premature death across sex and age stratum were examined by Cox regression. Survey weighting and inverse probability weighting (IPW) were further considered to control selection and confounding bias. Robustness assessment has been conducted on both NHANES and China Health and Retirement Longitudinal Study (CHARLS) data.
Results: A linear and positive relationship between WC and all-cause premature death was found in both males and females, with adjusted HRs of 1.019 (95%CI = 1.004–1.034) and 1.065 (95%CI = 1.039–1.091), respectively. Nonlinear relationships were found with respect to BMI and all-cause premature death. For females aged 36–50 with a BMI below 28.6 kg/m2, the risk of premature death decreased as BMI increased, indicated by adjusted HRs of 0.856 (95%CI = 0.790–0.927). Joint analysis showed among people living with obesity, a larger WC increased premature death risk (HR = 1.924, 95%CI = 1.444–2.564).
Discussion: WC and BMI exhibited prominent associations with premature death in young and middle-aged population. Maintaining an appropriate WC and BMI bears significant implications for preventing premature death.
1 Introduction
Premature death, is defined as dying before reaching the average life expectancy. It is widely used as a measure of population health internationally (1–3), and could be used to assess the “unnecessary” or “avoidable” burden of mortality. Noncommunicable diseases (NCDs) are the primary cause of premature deaths worldwide, accounting for approximately 79% of deaths among people aged 30–70 (4–7). Significantly, obesity, a major risk factor for NCDs, is associated with premature mortality in adults (5, 8, 9), especially among young and middle-aged individuals (10, 11), indicating reducing obesity incidence is a key strategy to prevent premature death.
Nevertheless, the association between obesity and mortality substantially varies by studies with different obesity indicators (6, 12), populations and designs. Body mass index (BMI) is mostly used to define obesity and is thought to be associated with premature death (13–15). However, specifically in BMI-related literature, there’s discussion of the term “obesity paradox,” where people living with obesity or overweight may correlate with better outcomes (16–18). BMI is not an accurate body fat indicator and cannot identify sarcopenic obesity. The adverse consequences of obesity may be closely related to the amount of visceral fat, and simple generalized obesity is not sufficient to explain the risk of obesity-related comorbidities (19). Therefore, central obesity represented by waist circumference (WC) and waist-hip ratio (WHR) should also be considered, which is more related to mortality (20–22). Compared with WHR, WC could be a better tool in practice for its significant correlation with visceral fat and fewer anthropometric measurement requirements (23–25). Furthermore, WHO also suggests that although BMI and abdominal adiposity measures may be highly correlated, considering the utility of joint use of the two indicators is desirable (26). In addition, most studies evaluate the association between obesity and mortality in patients with specific diseases such as diabetes (27), cancer (28), and COVID-19 (29), rather than a nationally representative general population. In the study design, some studies use self-reported measures instead of technician-measured data to conduct analyses, which could result in an inaccurate assessment of obesity (6, 30). Additionally, the majority of effect estimation studies could not control important confounding factors, such as smoking, making it impossible to get a true effect (31, 32).
In addition to the limitations in existing research mentioned above, despite the well-recognized correlation between WC and adverse health outcomes, there is no research focusing on the pattern of association between WC and premature death, and it remains unclear whether WC is an independent risk factor in addition to BMI. Moreover, obesity is a long-term process, which runs through a person’s whole life course. The process of obesity management requires the joint management of individual-community-medical institutions. Therefore, focusing on young and middle-aged individuals, this study aimed to reliably estimate independent and combined associations between WC, BMI and premature death with controlling selection and confounding bias scientifically. By this approach, we aimed to ascertain the precise effects of BMI and WC on premature death across different subgroups, thereby providing causal evidence to support strategies for obesity management.
2 Methods
2.1 Study population
This study used 1999–2018 survey data from NHANES (33), which was a nationally representative survey conducted by the United States Centers for Disease Control and Prevention. A complex, stratified, multistage probability sampling design was applied to select representative participants of the civilian, noninstitutionalized resident population of the United States. Multiple data, such as questionnaires, physical exams, and laboratory measurements were collected, with individuals’ mortality status accessed through the National Death Index (NDI). Detailed information on the survey was described elsewhere (34). Written informed consent was provided by all participants, and the protocol was approved by the Ethics Review Board of the National Center for Health Statistics (NCHS) (35).
There were 49,217 participants aged between 18 and 50 years old (young and middle-aged people) in this study. Referring to previous literature (36–38), among these participants, individuals were excluded if they had a missing baseline WC or BMI (n = 3,977), had missing follow-up death outcomes (n = 78), or were pregnant (n = 1,499). In addition, to avoid reverse causation, individuals who died within 2 years of the baseline survey (n = 129) and had WC or BMI distributed below the 1st centile (n = 1,292) were further excluded. The final sample consisted of 42,242 participants.
2.2 Assessment of exposures and outcome
WC and BMI were the exposures of interest. WC was measured to the nearest 1 mm just above the iliac crest by using a steel tape at baseline survey, with results recorded in centimeter (cm). BMI was calculated as weight in kilograms divided by height in meters squared, rounded to one decimal place (39). The outcome was all-cause premature death, defined as death from any death caused before 70 years old (7).
2.3 Assessment of covariates
Based on the literature review and expert knowledge, this study drew a causal directed acyclic graph (DAG) (40). In a causal DAG, the backdoor path is the non-causal path between the treatment A and the outcome Y, linking A and Y through the common cause L (Supplementary Figure S4). It is the presence of the common cause L creates an additional source of association between A and Y, which we refer to as confounding for the effect of A on Y (Supplementary file). According to this DAG and the backdoor criterion (Figure 1), confounding covariates for WC and premature death were selected, including demographics (sex, age, marital status, race, birthplace) and socioeconomic factors (education level, job status, social support and economic level), lifestyle factors (smoking, alcohol drinking, electronic product use time, sleep hours, and physical activity), and diet (number of restaurant meals and healthy eating). Among them, job status included long working hours and job type. Social support included emotional support, financial support, and the number of close friends. The economic level was represented by the family poverty level index (Family PIR). Physical activity included moderate activity and vigorous activity. In addition, WC and BMI were mutually adjusted to assess their independent effects on premature death. Covariates such as hypertension and diabetes were not included as confounding covariates because they are on the causal pathway of obesity and premature death. Including them in the model may lead to incorrect adjustment and introduce bias (6, 24, 37, 41). As a result, a total of 21 confounding covariates were incorporated into the model, and their definitions are shown in Supplementary Table S1.
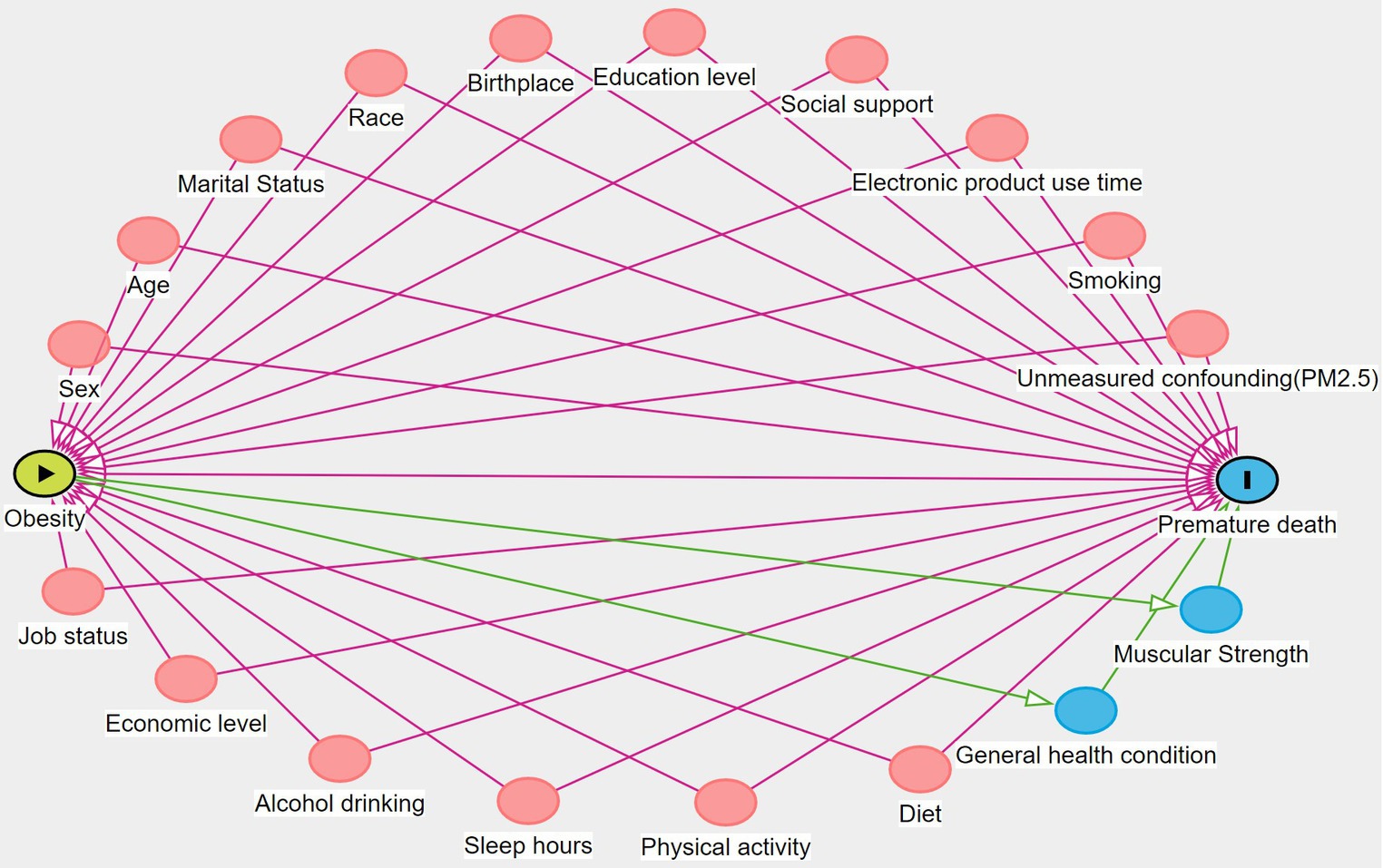
Figure 1. DAG for assessing confounding covariates in the relationship between obesity and premature death. The red circle represents confounding variables and the blue circle represents mediating variables.
2.4 Statistical methods
2.4.1 Participants characteristics study
Analysis of this study was conducted according to the official requirements (42) of NHANES and considered the complex survey design of the cohort. When describing the baseline characteristics of the respondents, categorical variables were summarized as number (percentage), and continuous variables as M (P25, P75). The χ2 test and rank sum test were, respectively, used for categorical variables and continuous variables to test whether there was a difference between the no premature death group and the premature death group.
2.4.2 Independent effect analysis
To investigate the independent association patterns between exposures and premature death by different sexes, analyses were conducted separately for WC and BMI. We calculated person-months from baseline to the date of death, or 31 December 2019, whichever came first. The proportional hazard assumption (PH assumption) was assessed using weighted Schoenfeld residuals (43). A survey-weighted stratified Cox regression was constructed by stratifying variables that failed to meet the PH assumption. We tested and revealed the nonlinearity by modeling exposures using classical restricted cubic splines with 4 knots (located at the 10th, 30th, 70th, and 90th percentiles). The reference value was the median (44, 45). In cases of nonlinearity, a recursive algorithm was utilized to ascertain the inflection point, signifying a pivotal shift in the relationship between exposures and premature mortality. Piecewise fitting was performed on both sides of the inflection point (46). If the results did not present non-linearity, the survey-weighted stratified Cox regression was constructed in a linear form. Results were presented as changes in the hazard ratio (HR) for a 1-cm increment in WC and a 1-kg/m2 increment in BMI.
Inverse probability weighting was further used in this study to control for confounding bias. Since the exposure was a continuous variable, a generalized propensity score model was first constructed (47, 48), with the exposure as the dependent variable and the confounding covariates as independent variables. To account for survey weights, we also incorporated them as covariates, following suggestions from the literature (49). Then inverse probability weights were calculated by estimating the probability of the exposure using a normal distribution. The balance test was performed by calculating the average absolute correlation between exposure and confounding covariates to ensure balance across confounding covariates, and all average absolute correlations of inverse probability weights in this study were smaller than 0.1, indicating balance across confounding covariates (Supplementary Figures S1, S2). In the second step, a stratified Cox proportional hazards regression model was constructed to study the association between exposures and premature death. Inverse probability weights and survey weights were considered at the same time. This study totally adjusted for 21 covariates selected by DAG. Referring to previous literature (50, 51), missing values were imputed via multiple imputation methods.
Moreover, referencing previous studies (52–54), we also did subgroup analyses to explore whether the association of exposures and premature death varied across age (18–35 years old and 36–50 years old), stratified by sex.
2.4.3 Joint analysis
To examine the combined effects of WC and BMI, we studied the association between WC and premature death within the BMI category (24). Because of the high correlation between BMI and WC, it was difficult to model the impact of WC on premature mortality across all categories of BMI (55). Therefore, participants were stratified by BMI category based on WHO standards (normal weight “18.5 to 24.9 kg/m2,” overweight “25 to 29.9 kg/m2,” and obesity “> 30 kg/m2”). The WC category was based on the sex-specific median cutting points, which was the median sex-specific WC of the population in each BMI category. In the joint analysis, owing to considering inverse probability weighting with binary exposure, a balance test was performed by calculating the Standardized Mean Difference (SMD) to ensure balance across confounding covariates (Supplementary Figure S3).
2.4.4 Robustness assessment
To assess the possible impact of unmeasured confounding, the classical E-value was calculated (56). The E-value is defined as the minimum strength of association on the risk ratio scale that an unmeasured confounder would need to have with both the treatment and the outcome to fully explain away a specific treatment-outcome association, conditional on the measured covariates (Supplementary file). The model without considering the inverse probability weights was also constructed. In addition, the joint analysis of this study was repeated in the China Health and Retirement Longitudinal Study (CHARLS) data (57).
All analyses were performed with R 4.0.5, using the package ‘survey’ and ‘rms’ to account for survey design and restricted cubic spline fitting (58), respectively. Self-written functions were used to determine inflection points. In all tests, a two-tailed p < 0.05 was considered statistically significant.
3 Results
3.1 Study population characteristics
There were 42,242 young and middle-aged participants in the study, with a median age of 34 years at the time of this study participation and 21,277 were males (50.37%). The survey-weighted median WC and BMI were 93.30 (IQR = 83.30–104.40) cm and 26.97 (IQR = 23.43–31.38) kg/m2, respectively. Over a median follow-up of 140 (IQR = 100–156) months, a total of 1,005 all-cause premature deaths were observed. The baseline characteristics were presented in Supplementary Table S2.
Comparisons of the premature death group with the no premature death group showed significant differences in sex, age, race, birthplace, education level, family PIR, smoking, sleep hours, and WC (all P < 0.05). In general, young and middle-aged people who died prematurely were more likely to be males, 36–50 years old, non-Hispanic white, born domestically (in the 50 US states or Washington of DC), having a high school degree or less, having a family poverty index >1.85, smoking every day, sleeping 6 h or less, and with larger WC.
3.2 Association of WC and premature death
In Figure 2, restricted cubic splines were used to flexibly model and visualize the relationship of WC with premature mortality, and linear associations were observed in males and females (Pnonlinear = 0.112, Pnonlinear = 0.458, respectively). Hence, WC was included in the models in a linear form, with statistically significant results (P < 0.01). The risk of premature death increased with WC gain in males and females, with adjusted HRs of 1.019 (1.004–1.034) and 1.065 (1.039–1.091), respectively (Table 1).
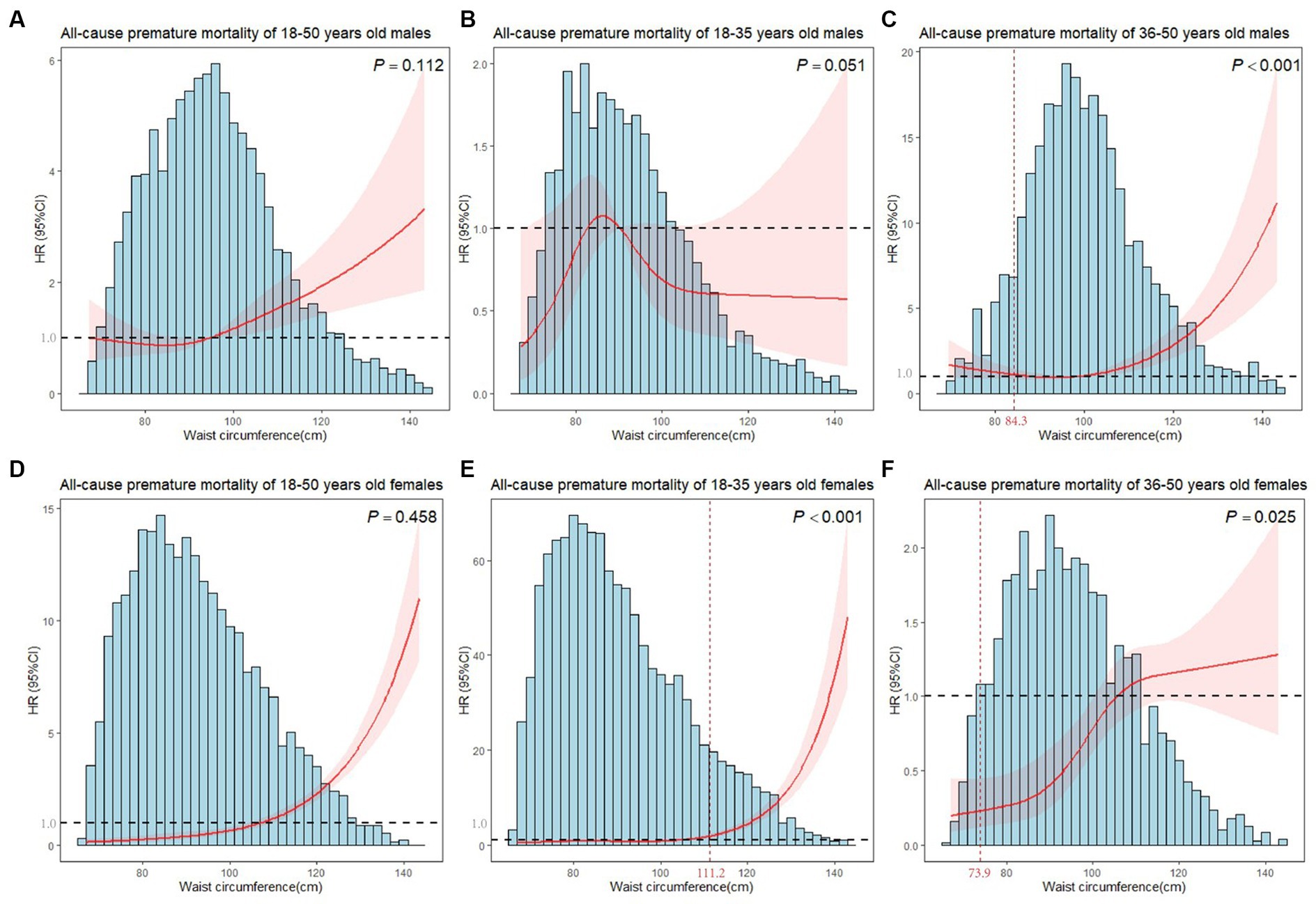
Figure 2. Plots of all-cause premature mortality HR from multivariate adjusted stratified Cox regression analysis using restricted cubic splines of WC with 4 degrees of freedom. (A) Model for 18–50 years old males. (B) Model for 18–35 years old males. (C) Model for 36–50 years old males. (D) Model for 18–50 years old females. (E) Model for 18–35 years old females. (F) Model for 36–50 years old females. P showed the test for nonlinearity. (B,C,E,F) models were adjusted for marital status, race, birthplace, education level, long working hours, job type, emotional support, financial support, number of close friends, economic level, smoking, alcohol drinking, electronic product use time, sleep hours, moderate activity, vigorous activity, number of restaurant meals, healthy eating, BMI. (A,D) models additionally adjusted for age. The horizontal line in the graph indicated a premature death HR of 1, and the vertical line marked the inflection point.
The age-specific analysis presented nonlinear associations between WC and premature mortality for 36–50 years old males, 18–35 years old females and 36–50 years old females (all Pnonlinear < 0.001). We estimated the premature mortality risk of the 36–50 years old males to reach the inflection point at WC of 84.3 cm, with inverse associations below (HR = 0.904, 95%CI = 0.845–0.966), and positive associations above (HR = 1.052, 95%CI = 1.033–1.072). For 36–50 years old females, a marked increase in risk at the middle WC, but minimal elevation in risk at lower and higher WC was observed (Figure 2). WC was a significant predictor of all-cause premature mortality in the 18–35 years old and 36–50 years old female subgroups when WCs were larger than inflection points (111.2 cm and 73.9 cm, respectively). However, no significant results (P > 0.05) were found in females when WCs were smaller than inflection points. Moreover, WC did not affect premature death in 18–35 years old males (Table 1).
3.3 Association of BMI and premature death
Figure 3 showed nonlinear associations of BMI with premature mortality in both males and females (all Pnonlinear < 0.001). The inflection point of males was 21.29 kg/m2, below which a significant inverse relationship was observed (HR = 0.562, 95%CI = 0.421–0.752). In females, a reduction of the risk within the lower range of BMI was found (HR = 0.854, 95%CI = 0.796–0.916) which reached the lowest risk around 30.12 kg/m2 and increased thereafter (HR = 1.132, 95%CI = 1.069–1.199, Table 2).
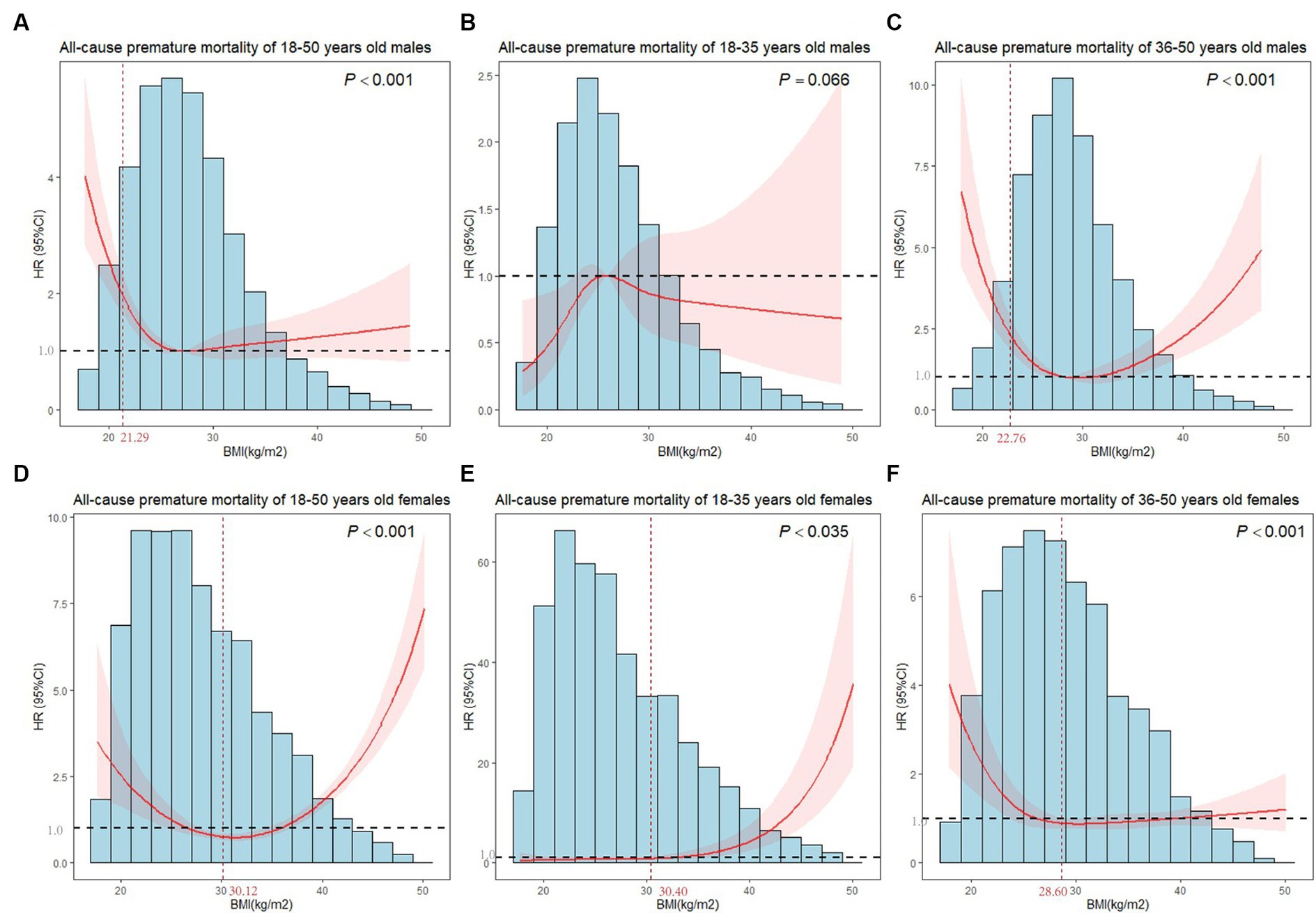
Figure 3. Plots of all-cause premature mortality HR from multivariate adjusted stratified Cox regression analysis using restricted cubic splines of BMI with 4 degrees of freedom. (A) Model for 18–50 years old males. (B) Model for 18–35 years old males. (C) Model for 36–50 years old males. (D) Model for 18–50 years old females. (E) Model for 18–35 years old females. (F) Model for 36–50 years old females. (B,C,E,F) models were adjusted for marital status, race, birthplace, education level, long working hours, job type, emotional support, financial support, number of close friends, economic level, smoking, alcohol drinking, electronic product use time, sleep hours, moderate activity, vigorous activity, number of restaurant meals, healthy eating, BMI. (A,D) models additionally adjusted for age. The horizontal line in the graph indicated a premature death HR of 1, and the vertical line marked the inflection point.
After age-stratified, we observed nonlinear associations between BMI and premature mortality in 36–50 years old males, 18–35 years old females and 36–50 years old females (Pnonlinear < 0.005). As shown in Table 2, the inflection point for all-cause mortality risk among 36–50 years old males was at a BMI of 22.76 kg/m2, with inverse associations below (HR = 0.597, 95%CI = 0.485–0.736), and positive associations above (HR = 1.069, 95%CI = 1.022–1.118). The inflection point for the effect of BMI of 18–35 years old females on premature death was 30.40 kg/m2. When the BMI of females was larger than 30.40 kg/m2, the risk of premature death increased with an increment in BMI (HR = 1.227, 95%CI = 1.156–1.302). In 36–50 years old females, we found a substantial reduction in risk below BMI of 28.60 kg/m2 (HR = 0.856, 95%CI = 0.790–0.927), whereas a nonsignificant positive association was observed above 28.60 kg/m2. Similarly, BMI did not affect premature death in 18–35 years old males.
3.4 Joint analysis
We also determined the combined association of WC and BMI with premature death. Table 3 showed that in the group with normal weight or overweight, WC larger than the median did not increase the risk of premature death (HR = 1.346, 95%CI = 0.937–1.934; HR = 1.136, 95%CI = 0.863–1.495). In the group with obesity, the premature death risk of individuals with WC larger than the median was 1.92 times of individuals with WC smaller than the median (HR = 1.924, 95%CI = 1.444–2.564).
3.5 Robustness assessment
The E-values of HR values obtained from different models were calculated for men and women, respectively. Results indicated that only the HR of unmeasured confounding exceeded the corresponding E-value would change the relationship between WC, BMI, and premature death found in this study (Tables 3, 4). However, since this study had adjusted for a sufficient number of confounding covariates, the likelihood of including unmeasured confounding with this strength to distort the findings was relatively low. Besides, the survey-weighted model without considering inverse probability weight showed similar results, demonstrating the robustness of the study. In addition, consistent results were observed based on the joint effect of CHARLS, particularly among individuals who are overweight, with an adjusted HR of 1.245 (95% CI = 0.614–2.528).
4 Discussion
In this prospective study involving 49,217 young and middle-aged Americans, with controlling selection and confounding bias scientifically, we first found a linear and positive relationship between WC and all-cause premature death in young and middle-aged people, and nonlinear relationships were found with respect to BMI and all-cause premature death. The results varied slightly across subgroups defined by gender and age. Nonlinear relationships between WC and all-cause premature death were found in males aged 36–50 years old and in females aged 18–35 years old and 36–50 years old. Additionally, we also discovered the combined effect of BMI and WC, with a larger WC being a risk factor for premature death among individuals classified with obesity based on BMI category.
Although the outcome of most studies was death rather than premature death, they still supported the results of this study (6). A pooled analysis of 650,000 adults from 11 prospective cohort studies also found a positive linear association between men and women. The association between WC and premature mortality may be due to the fact that a larger WC is highly correlated with visceral fat and is a reflection of visceral fat accumulation. Inflammation of visceral adipose tissue mediates metabolic disturbances and is associated with an increased risk of cancer, cardiovascular disease, and even death (36, 37, 59, 60).
When the BMI was low, a protective effect was found, consistent with other literature (61). This may be because there is an adverse effect on premature death when BMI is below the lower limit of normal weight. Lower BMI is related to reduced muscle mass, which leads to lower mobility. In addition, a study has also pointed out a protective effect of moderately increased body fat (15). Subcutaneous fat provides additional metabolic reserves, which are needed in stressful situations when the activation of the neurohormonal system and inflammation require additional energy. However, people with low BMI have less subcutaneous fat and are unable to provide extra energy to cope with stressful situations (62, 63).
We also found that for females with a BMI below 30.12 kg/m2, who are living with overweight or obesity, the risk of premature death decreased with the increase in BMI, suggesting the existence of an obesity paradox (64). Through further subgroup analysis, it was found that the obesity paradox appeared in 36–50 years old females living with overweight, similar to other studies (61, 65, 66). Some studies have proposed that age was a confounding factor leading to the obesity paradox. With the increase of age, fat loss becomes slower, and nutrition status is relatively better, so obesity becomes a protective factor (67). It may also be related to the distribution of body composition. Young and middle-aged people have more fat distributed in the limbs, larger muscle mass, and relatively less visceral fat, which is conducive to the prognosis of the disease (68). Other possible reasons are better compliance and higher rates of treatment after an illness among them (65). Furthermore, the influence of risk factors in young and middle-aged people is relatively small. Similar to the study by Vest et al., the phenomenon of the obesity paradox in women, while not present in men, may be due to differences in the distribution of fat in the sexes (69–71). Men tend to store excessive fat in visceral fat deposits, whereas women usually store fat in peripheral subcutaneous distributions. Excessive visceral fat could increase the risk of developing metabolic syndrome and cardiovascular diseases, whereas femoral-gluteal fat might be beneficial as a “sink” for lipids (72).
Neither WC nor BMI was associated with premature death among men aged 18–35 in this study. Previous literature has mentioned that men aged 18–35 are often highly active physically (73, 74), which could decrease the risk of premature death associated with obesity. Therefore, the results of this study were that obesity had little effect on premature death among men aged 18–35 (75). Despite this, men aged 18–35 should still avoid obesity, as neglecting to do so could increase the risk of premature death as they age. While this study accounted for moderate and vigorous physical activities as confounding factors, they may not fully represent the actual total physical activity situation. For example, daily walking was not included, so the confounding effect caused by physical activity in men aged 18–35 cannot be completely controlled.
Studies supporting the obesity paradox utilized BMI for defining obesity, which did not discriminate between lean mass and fatmass (76). Therefore, many studies have recommended the use of WC as a better means of identifying obesity (77). Geum Joon Cho et al. also reported that WC should be considered in the assessment of obesity-related health risks (14). However, for the strong association between BMI and death, BMI was still broadly used as an indicator of obesity in practical work. Considering the unique characteristics of BMI and WC, combining BMI and WC could better improve the ability to identify the risk of premature death (6, 78). Some studies have reported findings similar to this research, indicating increased mortality in adults living with obesity (79). The visceral microenvironment may be intrinsically toxic to arterial health, and WC is highly correlated with harmful visceral abdominal tissue (80–83). People who have larger WC and are living with obesity have higher amounts of visceral fat mass, which increases the risk of cardiometabolic diseases and is positively and significantly associated with a higher risk of all-cause mortality (84, 85). Therefore, we also recommend that the combined assessment of WC and BMI was conducive to identifying high-risk groups of premature death among people living with obesity more effectively.
We observed that young and middle-aged individuals, at a critical stage in the life course where the incidence of obesity and adverse outcomes is increasing, had both waist circumference (WC) and body mass index (BMI) associated with premature death. Based on our findings, we suggested that young and middle-aged people should avoid abdominal obesity and maintain a suitable BMI, rather than striving for a low BMI. Furthermore, the impact of obesity on the human body runs through the whole life course. Young and middle-aged people can establish a strong foundation for their health in later life by prioritizing this issue now. In addition to the individual’s own emphasis on obesity, communities and hospitals also play an important role in this process. There are often slogans in the community to remind residents to avoid obesity, increase exercise activities, and pay attention to a healthy diet (86–88). Medical service institutions and related societies may offer lifestyle guidelines for people living with obesity at various stages, while medical and social organizations can provide weight management programs (89–91).
This study used a large and nationally representative sample of the population, with long-term follow-up of death outcomes to ensure the quality of research data. Further, with in-depth consideration of the study population, a comprehensive selection of confounding covariates was performed to investigate the relationships between WC and BMI with premature death. The results of the inverse probability weighted balance assessment showed that confounding factors distributed balanced by inverse probability weighting and survey weighting, which enhanced the interpretability and validity of our analysis to provide evidence for effective research. Robustness assessment also suggested robustness of the association between exposures and premature death in young and middle-aged people. However, this study also has some limitations. First, WC and BMI were measured at baseline only, so we were unable to assess the effect of exposure change on premature death in the follow-up assessment. Second, due to the limitation of sample size, this study was also unable to study the specific causes of premature death. Third, due to data limitations, we cannot study the relationship between WHR and premature death. Fourthly, although NHANES employed standardized operating procedures and measurements of anthropometric indices like WC by trained technicians, with rigorous quality control measures implemented (24, 92, 93), there were slight differences in measurement standards compared to the WHO (94). While this limitation was not expected to affect the effect estimation of this study, which was the main aim of the study. Considering the possible influence on the calculation of inflection points, problems such as measurement methods are worth further study in the future. Nevertheless, the results of this study with causal inference methods controlling for possible selection bias and confounding bias, still could provide non-negligible support for further research on the obesity paradox and causal mechanism in the future. In the next studies, the pattern of association between WHR and premature death, WC and BMI with different causes of premature death will also be further explored, and possible mechanisms underlying the different patterns of associations will be explored in greater depth.
In conclusion, based on the results of this study, we concluded that WC and BMI exhibited prominent associations with all-cause premature death. WC and BMI were risk factors for the occurrence of premature death in young and middle-aged adults, except for males with small BMI and females with BMI below obesity. For young and middle-aged people living with obesity, regardless of gender, there is a need to control the growth of WC, and thus reduce the risk of premature death. The clinical importance of maintaining an appropriate WC and BMI bears significant implications for preventing premature mortality attributable to obesity.
Data availability statement
Publicly available datasets were analyzed in this study. This data can be found here: US National Health and Nutrition Examination Survey (NHANES): https://www.cdc.gov/nchs/nhanes/index.htm; China Health and Retirement Longitudinal Study (CHARLS): https://charls.pku.edu.cn/.
Ethics statement
All methods were carried out in accordance with the Data Security Law of the People’s Republic of China, the World Medical Association's Declaration of Helsinki, and other relevant guidelines and regulations. All the data involved in this research were obtained from NHANES and CHARLS. NHANES was approved by the Ethics Review Board of the National Center for Health Statistics. CHARLS was approved by the Ethics Review Committee of Peking University.
Author contributions
LH: Supervision, Writing – original draft, Writing – review & editing, Conceptualization, Data curation, Formal analysis, Investigation, Methodology, Project administration, Resources, Software, Validation, Visualization. XH: Data curation, Investigation, Validation, Writing – review & editing. MC: Data curation, Investigation, Writing – review & editing. TZ: Writing – review & editing, Funding acquisition, Supervision, Writing – original draft.
Funding
The author(s) declare financial support was received for the research, authorship, and/or publication of this article. This research work was funded by the National Key R&D Program of China (2022YFC3600600), National Natural Science Foundation of China (81602935), Natural Science Foundation of Sichuan Province of China (24NSFC0028), Sichuan Science and Technology Program (2022YFS0140, 2022YFS0229), and Tianfu New Area Project (H230821).
Acknowledgments
We also express our gratitude to Jiayuan Li, Xia Jiang, Jiaqiang Liao, Mengyu Fan, and Jingwen Jiang for their invaluable contributions to the drafting and revision of this manuscript.
Conflict of interest
The authors declare that the research was conducted in the absence of any commercial or financial relationships that could be construed as a potential conflict of interest.
Publisher’s note
All claims expressed in this article are solely those of the authors and do not necessarily represent those of their affiliated organizations, or those of the publisher, the editors and the reviewers. Any product that may be evaluated in this article, or claim that may be made by its manufacturer, is not guaranteed or endorsed by the publisher.
Supplementary material
The Supplementary material for this article can be found online at: https://www.frontiersin.org/articles/10.3389/fpubh.2024.1389766/full#supplementary-material
Abbreviations
WC, Waist circumference; CI, Confidence interval; NCDs, Noncommunicable diseases; WHO, World Health Organization; BMI, Body Mass Index; WHR, Waist-Hip Ratio; NHANES, National Health and Nutrition Examination Survey; NDI, National Death Index; NCHS, National Center for Health Statistics; DAG, Directed acyclic graph; Family PIR, Family Poverty Level Index; PH assumption, Proportional hazard assumption; HR, Hazard ratio; DC, The District of Columbia; IPW, Inverse Probability Weighting
References
1. Mazzuco, S, Suhrcke, M, and Zanotto, L. How to measure premature mortality? A proposal combining "relative" and "absolute" approaches. Popul Health Metrics. (2021) 19:41. doi: 10.1186/s12963-021-00267-y
2. WHO. World health statistics 2022: Monitoring health for the SDGs, sustainable development goals Organization GWH (2022). Available at: https://www.who.int/publications-detail-redirect/9789240051157
3. Xi, JY, Zhang, WJ, Chen, Z, Zhang, YT, Chen, LC, Zhang, YQ, et al. Potential gains in health-adjusted life expectancy by reducing burden of noncommunicable diseases in 188 countries: a population-based study. Value Health. (2023) 26:802–9. doi: 10.1016/j.jval.2022.12.008
4. Wang, YQ, Xiao, M, Lyu, J, Yu, CQ, Guo, Y, Pei, P, et al. A prospective cohort study of premature death and influencing factors in adults aged 56-69 years from 10 regions of China. Zhonghua Liu Xing Bing Xue Za Zhi. (2022) 43:1010–8. doi: 10.3760/cma.j.cn112338-20211210-00968
5. Liao, R, Hu, L, Liao, Q, Zhu, T, Yang, H, and Zhang, T. Analysis of death causes of residents in poverty-stricken areas in 2020: take Liangshan Yi autonomous prefecture in China as an example. BMC Public Health. (2022) 22:89. doi: 10.1186/s12889-022-12504-6
6. Cerhan, JR, Moore, SC, Jacobs, EJ, Kitahara, CM, Rosenberg, PS, Adami, HO, et al. A pooled analysis of waist circumference and mortality in 650,000 adults. Mayo Clin Proc. (2014) 89:335–45. doi: 10.1016/j.mayocp.2013.11.011
7. Norheim, OF, Jha, P, Admasu, K, Godal, T, Hum, RJ, Kruk, ME, et al. Avoiding 40% of the premature deaths in each country, 2010-30: review of national mortality trends to help quantify the UN sustainable development goal for health. Lancet. (2015) 385:239–52. doi: 10.1016/S0140-6736(14)61591-9
8. WHO. Global status report on noncommunicable diseases. Geneva SWHO: (2014). Available at: https://www.who.int/publications-detail-redirect/9789241564854
9. Fernandes, DPS, Lopes Juvanhol, L, Nobre, AA, de Souza, ÂMN, and Ribeiro, AQ. Central adiposity as a predictor of mortality in older adults: identification of cutoffs using generalized additive models. Front Nutr. (2023) 10:1132006. doi: 10.3389/fnut.2023.1132006
10. Dilishati, N. (2017). Study on the correlation between obesity index and hypertension susceptibility of Uygur young and middle-aged people in Tianshan District of Urumqi City.
11. Yano, Y, and Lloyd-Jones, DM. Isolated systolic hypertension in Young and middle-aged adults. Curr Hypertens Rep. (2016) 18:78. doi: 10.1007/s11906-016-0686-x
12. Pavela, G, Yi, N, Mestre, L, Xun, P, and Allison, DB. Birth weight moderates the association between obesity and mortality rate. Ann Epidemiol. (2023) 82:26–32. doi: 10.1016/j.annepidem.2023.03.010
13. Bradbury, KE, and Cairns, BJ. Understanding the relation between BMI and mortality. BMJ. (2019) 364:l1219. doi: 10.1136/bmj.l1219
14. Cho, GJ, Yoo, HJ, Hwang, SY, Choi, J, Lee, KM, Choi, KM, et al. Differential relationship between waist circumference and mortality according to age, sex, and body mass index in Korean with age of 30-90 years; a nationwide health insurance database study. BMC Med. (2018) 16:131. doi: 10.1186/s12916-018-1114-7
15. Eslami, A, Naghibi Irvani, SS, Ramezankhani, A, Fekri, N, Asadi, K, Azizi, F, et al. Incidence and associated risk factors for premature death in the Tehran lipid and glucose study cohort. Iran BMC Public Health. (2019) 19:719. doi: 10.1186/s12889-019-7056-y
16. Dramé, M, and Godaert, L. The obesity paradox and mortality in older adults: a systematic review. Nutrients. (2023) 15:1780. doi: 10.3390/nu15071780
17. Lavie, CJ, De Schutter, A, Parto, P, Jahangir, E, Kokkinos, P, Ortega, FB, et al. Obesity and prevalence of cardiovascular diseases and prognosis-the obesity paradox updated. Prog Cardiovasc Dis. (2016) 58:537–47. doi: 10.1016/j.pcad.2016.01.008
18. Zhang, J, Na, X, Li, Z, Ji, JS, Li, G, Yang, H, et al. Sarcopenic obesity is part of obesity paradox in dementia development: evidence from a population-based cohort study. BMC Med. (2024) 22:133. doi: 10.1186/s12916-024-03357-4
19. Freedman, DS, and Ford, ES. Are the recent secular increases in the waist circumference of adults independent of changes in BMI? Am J Clin Nutr. (2015) 101:425–31. doi: 10.3945/ajcn.114.094672
20. Parra-Soto, S, Petermann-Rocha, F, Boonpor, J, Gray, SR, Pell, JP, Celis-Morales, C, et al. Combined association of general and central obesity with incidence and mortality of cancers in 22 sites. Am J Clin Nutr. (2021) 113:401–9. doi: 10.1093/ajcn/nqaa335
21. Zhang, Y, Chen, GC, Sotres-Alvarez, D, Perreira, KM, Daviglus, ML, Pirzada, A, et al. General or central obesity and mortality among US Hispanic and Latino adults. JAMA Netw Open. (2024) 7:e2351070. doi: 10.1001/jamanetworkopen.2023.51070
22. Coutinho, T, Goel, K, Corrêa de Sá, D, Carter, RE, Hodge, DO, Kragelund, C, et al. Combining body mass index with measures of central obesity in the assessment of mortality in subjects with coronary disease: role of "normal weight central obesity". J Am Coll Cardiol. (2013) 61:553–60. doi: 10.1016/j.jacc.2012.10.035
23. Gažarová, M, Bihari, M, Lorková, M, Lenártová, P, and Habánová, M. The use of different anthropometric indices to assess the body composition of Young women in relation to the incidence of obesity, sarcopenia and the premature mortality risk. Int J Environ Res Public Health. (2022) 19:12449. doi: 10.3390/ijerph191912449
24. Reis, JP, Macera, CA, Araneta, MR, Lindsay, SP, Marshall, SJ, and Wingard, DL. Comparison of overall obesity and body fat distribution in predicting risk of mortality. Obesity (Silver Spring). (2009) 17:1232–9. doi: 10.1038/oby.2008.664
25. Perez-Cornago, A, Dunneram, Y, Watts, EL, Key, TJ, and Travis, RC. Adiposity and risk of prostate cancer death: a prospective analysis in UK biobank and meta-analysis of published studies. BMC Med. (2022) 20:143. doi: 10.1186/s12916-022-02336-x
26. WHO. Waist circumference and waist-hip ratio: Report of a WHO expert consultation, Geneva, 8–11 December 2008. WHO. (2011). Available at: https://www.who.int/publications/i/item/9789241501491
27. Effects of intensive lifestyle intervention on all-cause mortality in older adults with type 2 diabetes and overweight/obesity: results from the look AHEAD study. Diabetes Care. (2022) 45:1252–9. doi: 10.2337/dc21-1805
28. Zhou, J, Luo, L, Xie, L, Hu, S, Tan, L, Lei, X, et al. Sarcopenic obesity by the ESPEN/EASO criteria for predicting mortality in advanced non-small cell lung cancer. Clin Nutr. (2023) 42:817–24. doi: 10.1016/j.clnu.2023.04.010
29. den Uil, CA, Termorshuizen, F, Rietdijk, WJR, Sablerolles, RSG, van der Kuy, HPM, Haas, LEM, et al. Age moderates the effect of obesity on mortality risk in critically ill patients with COVID-19: a Nationwide observational cohort study. Crit Care Med. (2023) 51:484–91. doi: 10.1097/CCM.0000000000005788
30. Roswall, N, Li, Y, Sandin, S, Ström, P, Adami, HO, and Weiderpass, E. Changes in body mass index and waist circumference and concurrent mortality among Swedish women. Obesity (Silver Spring). (2017) 25:215–22. doi: 10.1002/oby.21675
31. Howell, CR, Mehta, T, Ejima, K, Ness, KK, Cherrington, A, and Fontaine, KR. Body composition and mortality in Mexican American adults: results from the National Health and nutrition examination survey. Obesity (Silver Spring). (2018) 26:1372–80. doi: 10.1002/oby.22251
32. Muller, DC, Murphy, N, Johansson, M, Ferrari, P, Tsilidis, KK, Boutron-Ruault, MC, et al. Modifiable causes of premature death in middle-age in Western Europe: results from the EPIC cohort study. BMC Med. (2016) 14:87. doi: 10.1186/s12916-016-0630-6
33. Centers for Disease Control and Prevention: NHANES. Available at: http://www.cdc.gov/nchs/nhanes.htm.
34. Wang, J, Fan, J, Yang, Y, Moazzen, S, Chen, D, Sun, L, et al. Vitamin D status and risk of all-cause and cause-specific mortality in osteoarthritis patients: results from NHANES III and NHANES 2001-2018. Nutrients. (2022) 14:4629. doi: 10.3390/nu14214629
35. Ruan, Z, Lu, T, Chen, Y, Yuan, M, Yu, H, Liu, R, et al. Association between psoriasis and nonalcoholic fatty liver disease among outpatient US adults. JAMA Dermatol. (2022) 158:745–53. doi: 10.1001/jamadermatol.2022.1609
36. Huai, P, Liu, J, Ye, X, and Li, WQ. Association of Central Obesity with all Cause and Cause-Specific Mortality in US adults: a prospective cohort study. Front Cardiovasc Med. (2022) 9:816144. doi: 10.3389/fcvm.2022.816144
37. Sahakyan, KR, Somers, VK, Rodriguez-Escudero, JP, Hodge, DO, Carter, RE, Sochor, O, et al. Normal-weight central obesity: implications for Total and cardiovascular mortality. Ann Intern Med. (2015) 163:827–35. doi: 10.7326/M14-2525
38. Berrington de Gonzalez, A, Hartge, P, Cerhan, JR, Flint, AJ, Hannan, L, MacInnis, RJ, et al. Body-mass index and mortality among 1.46 million white adults. N Engl J Med. (2010) 363:2211–9. doi: 10.1056/NEJMoa1000367
39. Steele, CC, Steele, TJ, Rosenkranz, SK, Lee, J, and Ade, CJ. Health behaviors and patient-practitioner communication in cancer patients and the general population: an analysis of the National Health and nutrition examination survey (NHANES) 2005-2014. Support Care Cancer. (2021) 29:3877–84. doi: 10.1007/s00520-020-05940-w
40. Ferguson, KD, McCann, M, Katikireddi, SV, Thomson, H, Green, MJ, Smith, DJ, et al. Evidence synthesis for constructing directed acyclic graphs (ESC-DAGs): a novel and systematic method for building directed acyclic graphs. Int J Epidemiol. (2020) 49:322–9. doi: 10.1093/ije/dyz150
41. Hu, FB (ed.). Obesity and mortality. In: Obesity epidemiology. New York: Oxford Academic (2008). doi: 10.1093/acprof:oso/9780195312911.001.0001
42. NHANES Tutorials. National health and nutrition examination survey. Available at: https://wwwn.cdc.gov/nchs/nhanes/tutorials/default (Accessed May 2022).
43. PATRICIA, M, GRAMBSCH,, TERRY, M, and THERNEAU,. Proportional hazards tests and diagnostics based on weighted residuals. Biometrika. (1995) 82:668. doi: 10.1093/biomet/82.3.668
44. Desquilbet, L, and Mariotti, F. Dose-response analyses using restricted cubic spline functions in public health research. Stat Med. (2010) 29:1037–57. doi: 10.1002/sim.3841
45. Greenland, S. Dose-response and trend analysis in epidemiology: alternatives to categorical analysis. Epidemiology. (1995) 6:356–65. doi: 10.1097/00001648-199507000-00005
46. Xiao, Q, Cai, B, Yin, A, Huo, H, Lan, K, Zhou, G, et al. L-shaped association of serum 25-hydroxyvitamin D concentrations with cardiovascular and all-cause mortality in individuals with osteoarthritis: results from the NHANES database prospective cohort study. BMC Med. (2022) 20:308. doi: 10.1186/s12916-022-02510-1
47. Xu, H, Yang, T, Guo, B, Silang, Y, Dai, Y, Baima, K, et al. Increased allostatic load associated with ambient air pollution acting as a stressor: cross-sectional evidence from the China multi-ethnic cohort study. Sci Total Environ. (2022) 831:155658. doi: 10.1016/j.scitotenv.2022.155658
48. Hirano, K, and Imbens, GW. The propensity score with continuous treatments. In: WA Shewhart, SS Wilks, A Gelman, X-L Meng, editors. Applied Bayesian Modeling and Causal Inference from Incomplete-Data Perspectives. (2005).
49. Dugoff, EH, Schuler, M, and Stuart, EA. Generalizing observational study results: applying propensity score methods to complex surveys. Health Serv Res. (2014) 49:284–303. doi: 10.1111/1475-6773.12090
50. Lu, Y, Zhang, J, Li, H, and Li, T. Association of non-alcoholic fatty liver disease with self-reported osteoarthritis among the US adults. Arthritis Res Ther. (2024) 26:40. doi: 10.1186/s13075-024-03272-2
51. Gong, R, Pu, X, Cheng, Z, Ding, J, Chen, Z, and Wang, Y. The association between serum cadmium and diabetes in the general population: a cross-sectional study from NHANES (1999-2020). Front Nutr. (2022) 9:966500. doi: 10.3389/fnut.2022.966500
52. Taing, KY, Ardern, CI, and Kuk, JL. Effect of the timing of weight cycling during adulthood on mortality risk in overweight and obese postmenopausal women. Obesity (Silver Spring). (2012) 20:407–13. doi: 10.1038/oby.2011.207
53. Pierce, JP. Quitting smoking by age 35 years-a goal for reducing mortality. JAMA Netw Open. (2022) 5:e2231487. doi: 10.1001/jamanetworkopen.2022.31487
54. Bhaskaran, K, Dos-Santos-Silva, I, Leon, DA, Douglas, IJ, and Smeeth, L. Association of BMI with overall and cause-specific mortality: a population-based cohort study of 3·6 million adults in the UK. Lancet Diabetes Endocrinol. (2018) 6:944–53. doi: 10.1016/S2213-8587(18)30288-2
55. Pischon, T, Boeing, H, Hoffmann, K, Bergmann, M, Schulze, MB, Overvad, K, et al. General and abdominal adiposity and risk of death in Europe. N Engl J Med. (2008) 359:2105–20. doi: 10.1056/NEJMoa0801891
56. VanderWeele, TJ, and Ding, P. Sensitivity analysis in observational research: introducing the E-value. Ann Intern Med. (2017) 167:268–74. doi: 10.7326/M16-2607
57. Zhao, Y, Strauss, J, Yang, G, Giles, J, Hu, P(P), Hu, Y, et al. China health and retirement longitudinal study: 2011–2012 National Baseline User’s guide: National School of development. Beijing: Peking University (2013).
58. FE H. RMS: regression modeling strategies. Available at: http://CRAN.R-project.org/package=rms.
59. Sun, Y, Liu, B, Snetselaar, LG, Wallace, RB, Caan, BJ, Rohan, TE, et al. Association of Normal-Weight Central Obesity with all-Cause and Cause-Specific Mortality among Postmenopausal Women. JAMA Netw Open. (2019) 2:e197337. doi: 10.1001/jamanetworkopen.2019.7337
60. Cameron, AJ, Romaniuk, H, Orellana, L, Dallongeville, J, Dobson, AJ, Drygas, W, et al. Combined influence of waist and hip circumference on risk of death in a large cohort of European and Australian adults. J Am Heart Assoc. (2020) 9:e015189. doi: 10.1161/JAHA.119.015189
61. Chen, Y, Yang, Y, Jiang, H, Liang, X, Wang, Y, and Lu, W. Associations of BMI and waist circumference with all-cause mortality: a 22-year cohort study. Obesity (Silver Spring). (2019) 27:662–9. doi: 10.1002/oby.22423
62. Kanic, V, Vollrath, M, Frank, B, and Kanic, Z. An obesity paradox in patients with myocardial infarction undergoing percutaneous intervention. Nutr Metab Cardiovasc Dis. (2021) 31:127–36. doi: 10.1016/j.numecd.2020.08.024
63. Itoh, H, Kaneko, H, Kiriyama, H, Kamon, T, Fujiu, K, Morita, K, et al. Reverse J-shaped relationship between body mass index and in-hospital mortality of patients hospitalized for heart failure in Japan. Heart Vessel. (2021) 36:383–92. doi: 10.1007/s00380-020-01699-6
64. Bosello, O, Donataccio, MP, and Cuzzolaro, M. Obesity or obesities? Controversies on the association between body mass index and premature mortality. Eat Weight Disord. (2016) 21:165–74. doi: 10.1007/s40519-016-0278-4
65. Wang, J, Wang, C, Zeng, Z, and Zuo, H. Impact of BMI on Long-term outcomes in patients with ST-segment elevation myocardial infarction after primary percutaneous coronary intervention. Int J Clin Pract. (2022) 2022:1–8. doi: 10.1155/2022/6210204
66. Lee, HJ, Kim, HK, Han, KD, Lee, KN, Park, JB, Lee, H, et al. Age-dependent associations of body mass index with myocardial infarction, heart failure, and mortality in over 9 million Koreans. Eur J Prev Cardiol. (2022) 29:1479–88. doi: 10.1093/eurjpc/zwac094
67. Antonopoulos, AS, Oikonomou, EK, Antoniades, C, and Tousoulis, D. From the BMI paradox to the obesity paradox: the obesity-mortality association in coronary heart disease. Obes Rev. (2016) 17:989–1000. doi: 10.1111/obr.12440
68. Lin, JC, Zheng, JJ, Wu, GH, Chen, ZH, Chen, XD, and Jiang, Q. Differences by age in the obesity paradox in severe burns. Burns. (2022) 48:547–54. doi: 10.1016/j.burns.2022.02.004
69. Vest, AR, Wu, Y, Hachamovitch, R, Young, JB, and Cho, L. The heart failure overweight/obesity survival paradox: the missing sex link. JACC Heart Fail. (2015) 3:917–26. doi: 10.1016/j.jchf.2015.06.009
70. Streng, KW, Voors, AA, Hillege, HL, Anker, SD, Cleland, JG, Dickstein, K, et al. Waist-to-hip ratio and mortality in heart failure. Eur J Heart Fail. (2018) 20:1269–77. doi: 10.1002/ejhf.1244
71. Soeters, PB, and Sobotka, L. The pathophysiology underlying the obesity paradox. Nutrition. (2012) 28:613–5. doi: 10.1016/j.nut.2011.10.010
72. Borgeraas, H, Hertel, JK, Svingen, GF, Seifert, R, Pedersen, EK, Schartum-Hansen, H, et al. Association of body mass index with risk of acute myocardial infarction and mortality in Norwegian male and female patients with suspected stable angina pectoris: a prospective cohort study. BMC Cardiovasc Disord. (2014) 14:68. doi: 10.1186/1471-2261-14-68
73. Nagata, JM, Vittinghoff, E, Pettee Gabriel, K, Garber, AK, Moran, AE, Sidney, S, et al. Physical activity and hypertension from Young adulthood to middle age. Am J Prev Med. (2021) 60:757–65. doi: 10.1016/j.amepre.2020.12.018
74. Nagata, JM, Vittinghoff, E, Pettee Gabriel, K, Garber, AK, Moran, AE, Rana, JS, et al. Moderate-to-vigorous intensity physical activity from young adulthood to middle age and metabolic disease: a 30-year population-based cohort study. Br J Sports Med. (2022) 56:847–53. doi: 10.1136/bjsports-2021-104231
75. Sanchez-Lastra, MA, Ding, D, Dalene, KE, Ekelund, U, and Tarp, J. Physical activity and mortality across levels of adiposity: a prospective cohort study from the UK biobank. Mayo Clin Proc. (2021) 96:105–19. doi: 10.1016/j.mayocp.2020.06.049
76. Després, JP. Body fat distribution and risk of cardiovascular disease: an update. Circulation. (2012) 126:1301–13. doi: 10.1161/CIRCULATIONAHA.111.067264
77. Pujilestari, CU, Nyström, L, Norberg, M, and Ng, N. Waist circumference and all-cause mortality among older adults in rural Indonesia. Int J Environ Res Public Health. (2019) 16:116. doi: 10.3390/ijerph16010116
78. Yang, M, Zhang, Y, Zhao, W, Ge, M, Sun, X, Zhang, G, et al. Individual and combined associations of body mass index and waist circumference with components of metabolic syndrome among multiethnic middle-aged and older adults: a cross-sectional study. Front Endocrinol. (2023) 14:1078331. doi: 10.3389/fendo.2023.1078331
79. Staiano, AE, Reeder, BA, Elliott, S, Joffres, MR, Pahwa, P, Kirkland, SA, et al. Body mass index versus waist circumference as predictors of mortality in Canadian adults. Int J Obes. (2012) 36:1450–4. doi: 10.1038/ijo.2011.268
80. Ouchi, N, Parker, JL, Lugus, JJ, and Walsh, K. Adipokines in inflammation and metabolic disease. Nat Rev Immunol. (2011) 11:85–97. doi: 10.1038/nri2921
81. Després, JP. Cardiovascular disease under the influence of excess visceral fat. Crit Pathw Cardiol. (2007) 6:51–9. doi: 10.1097/HPC.0b013e318057d4c9
82. Fox, CS, Massaro, JM, Hoffmann, U, Pou, KM, Maurovich-Horvat, P, Liu, CY, et al. Abdominal visceral and subcutaneous adipose tissue compartments: association with metabolic risk factors in the Framingham heart study. Circulation. (2007) 116:39–48. doi: 10.1161/CIRCULATIONAHA.106.675355
83. Farb, MG, Ganley-Leal, L, Mott, M, Liang, Y, Ercan, B, Widlansky, ME, et al. Arteriolar function in visceral adipose tissue is impaired in human obesity. Arterioscler Thromb Vasc Biol. (2012) 32:467–73. doi: 10.1161/ATVBAHA.111.235846
84. Jayedi, A, Soltani, S, Zargar, MS, Khan, TA, and Shab-Bidar, S. Central fatness and risk of all cause mortality: systematic review and dose-response meta-analysis of 72 prospective cohort studies. BMJ. (2020) 370:m3324. doi: 10.1136/bmj.m3324
85. Tran, NTT, Blizzard, CL, Luong, KN, Truong, NLV, Tran, BQ, Otahal, P, et al. The importance of waist circumference and body mass index in cross-sectional relationships with risk of cardiovascular disease in Vietnam. PLoS One. (2018) 13:e0198202. doi: 10.1371/journal.pone.0198202
86. Jensen, MD, Ryan, DH, Apovian, CM, Ard, JD, Comuzzie, AG, Donato, KA, et al. 2013 AHA/ACC/TOS guideline for the management of overweight and obesity in adults: a report of the American College of Cardiology/American Heart Association task force on practice guidelines and the Obesity Society. Circulation. (2014) 129:S102–38. doi: 10.1161/01.cir.0000437739.71477.ee
87. Dietz, WH, Baur, LA, Hall, K, Puhl, RM, Taveras, EM, Uauy, R, et al. Management of obesity: improvement of health-care training and systems for prevention and care. Lancet. (2015) 385:2521–33. doi: 10.1016/S0140-6736(14)61748-7
88. Garvey, WT, Mechanick, JI, Brett, EM, Garber, AJ, Hurley, DL, Jastreboff, AM, et al. American association of clinical endocrinologists and AMERICAN COLLEGE of endocrinology comprehensive clinical practice guidelines for medical care of patients with obesity. Endocr Pract. (2016) 22:1–203. doi: 10.4158/EP161365.GL
89. Yang, X, Chattopadhyay, K, Hubbard, R, Li, JL, Li, L, and Lin, Y. 36-month evaluation of a weight management Programme in Chinese overweight and obese adults. Front Public Health. (2021) 9:749302. doi: 10.3389/fpubh.2021.749302
90. Soni, A, Beeken, RJ, McGowan, L, Lawson, V, Chadwick, P, and Croker, H. ‘Shape-up’, a modified cognitive-Behavioural community Programme for weight management: real-world evaluation as an approach for delivering public health goals. Nutrients. (2021) 13:2807. doi: 10.3390/nu13082807
91. Bray, GA, Heisel, WE, Afshin, A, Jensen, MD, Dietz, WH, Long, M, et al. The science of obesity management: an Endocrine Society scientific statement. Endocr Rev. (2018) 39:79–132. doi: 10.1210/er.2017-00253
92. Statistics NCfH. NHANES 1999-2000 Procedure Manuals: Centers for Disease Control and Prevention. (2001). Available at: https://wwwn.cdc.gov/nchs/nhanes/continuousnhanes/manuals.aspx?BeginYear=1999.
93. Sakharkar, P, and Ansari, N. Application of exploratory factor analysis and item response theory to validate NHANES ADL scale in patients reporting rheumatoid arthritis. Pharmacy (Basel). (2022) 10. doi: 10.3390/pharmacy10060138
Keywords: premature death, waist circumference, BMI, young and middle-aged people, inverse probability weighting, NHANES
Citation: Hu L, Han X, Chen M and Zhang T (2024) Association of waist circumference and BMI with premature death in young and middle-aged population. Front. Public Health. 12:1389766. doi: 10.3389/fpubh.2024.1389766
Edited by:
Laura A. Gray, The University of Sheffield, United KingdomReviewed by:
Zbigniew Kułaga, Children's Memorial Health Institute (IPCZD), PolandAlison Dunkley, University of Leicester, United Kingdom
Sarah Bates, The University of Sheffield, United Kingdom
Copyright © 2024 Hu, Han, Chen and Zhang. This is an open-access article distributed under the terms of the Creative Commons Attribution License (CC BY). The use, distribution or reproduction in other forums is permitted, provided the original author(s) and the copyright owner(s) are credited and that the original publication in this journal is cited, in accordance with accepted academic practice. No use, distribution or reproduction is permitted which does not comply with these terms.
*Correspondence: Tao Zhang, statzhangtao@scu.edu.cn