- 1School of Information, Yunnan University of Finance and Economics, Kunming, China
- 2Yunnan Key Laboratory of Service Computing, Kunming, China
- 3Zhaotong People's Traditional Chinese Medicine Hospital, Zhaotong, China
Objective: Epidemics are sudden and rapidly spreading. Hospitals in underdeveloped areas are particularly vulnerable in case of an outbreak. This paper aims to assess the epidemic risk state and its change trend of hospitals in different epidemic stages, identify the key factors affecting hospital epidemic risk change, provide priority reference for hospital epidemic risk control, and enhance the hospital's ability to respond to sudden epidemics.
Methods: Based on Grounded theory, the epidemic risk indicators that affect hospital safety are summarized. The concept of epidemic risk state and its random state space is proposed according to Markov chain theory. The impact of each indicator on the random risk state and its change is comprehensively assessed from two aspects: risk occurrence probability and risk loss. Finally, the assessment of the hospital epidemic risk state and its change at different stages is achieved.
Results: The stable risk states of public hospitals in underdeveloped areas in non-epidemic stage t0, early epidemic stage t1, and outbreak stage t2 are , , and , respectively. In non-epidemic stage, the key factor in improving the hospital epidemic risk state is emergency funding. In early epidemic stage, the key factors in improving the hospital epidemic risk state are the training of medical staff in epidemic prevention skills and the management of public health. In outbreak state, the key factor in improving the hospital epidemic risk state is the training of medical staff in epidemic prevention skills and psychological awareness.
Conclusion: This paper proposes the concept of epidemic risk state, providing an effective assessment method for the epidemic risk state and its change trend in public hospitals. According to the assessment, public hospitals in underdeveloped areas in different epidemic stages should adopt different risk control strategies to improve their current risk state. Blind risk control is inefficient and may even cause the epidemic risk to transition toward a more dangerous state.
1 Introduction
According to “Law of the People's Republic of China on the Prevention and Control of Infectious Diseases,” “Regulations on Public Health Emergencies,” and “National Public Health Emergencies Emergency Plan,” public hospitals play an important role in the emergency response system for public health emergencies and undertake the key task of preventing infectious diseases. In recent years, sudden public health emergencies, such as infectious diseases and floods have occurred frequently, posing a serious threat to public health and social stability. Public hospitals, especially in underdeveloped areas, suffer from insufficient investment in medical and health resources, imperfect management and service mechanisms, and a shortage of professional and technical personnel (1, 2). As a result, when dealing with sudden epidemics, these public hospitals are particularly vulnerable to risks and hazards due to insufficient emergency response capabilities. If a local public hospital is unable to effectively control the epidemic, it can have serious consequences for public health, medical service quality, and hospital operating funds, and can even cause the spread of regional epidemic risk.
In China, infectious diseases are considered public health emergencies, accounting for 87.5% of all public health emergencies (3). These infectious diseases usually have the characteristics of sudden occurrence and rapid spread, with great uncertainty, which can easily cause serious harm to public health and the economy. In China's 14th Five Year Plan, the “Opinions on Promoting High Quality Development of Public Hospitals” have clearly included enhancing the emergency response capabilities of public hospitals in the development requirements (4). Hospitals, as the main bearers of infectious disease prevention and control tasks in China's sudden public health emergencies, need to comprehensively analyze potential risk factors and enhance their ability to respond to sudden epidemics.
To effectively enhance the hospital's ability to respond to sudden epidemics, it is necessary to first analyze the relevant risk factors that affect hospital safety. Scholars at home and abroad have discussed the risk factors that affect hospital safety from different perspectives and pointed out the necessity of risk control. Lingyu et al. (5) emphasized the need for strengthening emergency rescue drills and medical personnel training to improve their core emergency response capabilities during infectious disease outbreaks. LeBlanc et al. (6) had recognized that medical personnel tends to make more delegation and omission errors in high-pressure work environments. Kyron et al. (7) pointed out that emergency service personnel have a higher risk of developing mental health conditions. Zhou et al. (8) also well-acknowledged that during the epidemic, medical staff are more susceptible to psychological disorders than the general population, and it is necessary to arrange work hours reasonably during the epidemic. For instance, the following studies were conducted on ensuring the quality of medical service: Brandão et al. (9) have suggested that providing psychological training to medical personnel can effectively alleviate their anxiety, consequently ensuring the safety and quality of medical service. To effectively manage the risk of an outbreak, Gail et al. proposed a competency-based approach to health human resources (HHR) planning. This approach is used to estimate the number of professionals needed during an outbreak. It was emphasized that emergency professionals need to be adequately prepared (10). Shu et al. (11) pointed out that during major health events, existing hospitals may not have the capacity to accommodate, place, and provide medical assistance to all patients. In order to address the questions outlined above, priority should be given to the establishment of emergency medical public service facilities. For example, Wuhan Fangcang shelter hospital C Hall discharged 56% of patients after the cure, while the remaining 44% were transferred to designated hospitals for further treatment after their condition stabilized. No patient died during this transition (12). This shows that reasonable planning of emergency medical public service facilities can effectively control the epidemic risk. In addition to strengthening the response of public service facilities, the public health of hospitals also has a significant impact on the control of epidemic risk. Azuma et al. (13) found that strengthening public health management in hospitals has a direct and significant effect on preventing the spread of infectious diseases. Jie et al. (14) pointed out that there are loopholes in the emergency resource management of hospitals in China, indicating that the emergency resource management capabilities of hospitals in China need to be further strengthened, especially research based on theoretical and model levels. Weiping et al. (15) emphasized that emergency funds are crucial for providing financial support for the emergency management of public emergencies. By managing and using emergency funds scientifically and reasonably, the efficiency of emergency management can be effectively improved. In addition, Campos et al. (16) suggest that temporary hospitals can be used to relieve the pressure on hospitals during emergencies and ensure the safety of medical service facilities and personnel health. Rana et al. (17), emphasized the importance of effectively regulating hospitals during an outbreak to ensure the stability of the healthcare system. Performance assessments can help improve the efficiency and job satisfaction of medical personnel while reducing turnover rates. Kabego et al. (18) pointed out that reasonable performance management will have a significant positive impact on the quality of healthcare provided by medical institutions during outbreaks. Shen et al. (19) pointed out that by establishing administrative teams, renovating infrastructure, promoting medical staff training and patient education, the spread of the epidemic can be more effectively controlled. Zhi-jun et al. (20) pointed out the construction of hospital safety management system must strengthen investment in prevention and control of hospital infection.
In summary, there are various risk influencing factors in hospitals during the epidemic period, which affect the health of personnel, the quality of medical services, and the operations safety of the entire hospital. To effectively prevent and control epidemic risk, it is necessary to conduct an assessment of epidemic risk around these related risk factors. Although there have been some studies on the assessment of epidemic risks and emergency plans (21–26), these studies have not conducted an assessment of the epidemic risk state and its change trend, ignoring the changes in epidemic risks at different stages. As a key institution for epidemic prevention and control, hospitals must accurately grasp the current epidemic risk and its change trend. By assessing the hospital epidemic risk state and its change trend, it will be possible to effectively identify key risk factors that affect hospital safety during sudden epidemics, providing priority reference for risk prevention and control in hospitals. On the other hand, a lack of understanding of potential risks can make it difficult for hospitals to allocate resources effectively in the event of an outbreak, making it challenging for hospitals to control the epidemic risk. Especially in underdeveloped areas, hospitals often lack the necessary resources and medical conditions to respond effectively to sudden epidemics. Therefore, it has become particularly important to strengthen the ability of hospitals in underdeveloped areas to respond to sudden epidemics.
This paper will focus on the characteristics of hospitals in underdeveloped areas of China, combined with the relevant influencing factors of hospital risk management during sudden outbreaks at home and abroad, to provide effective methods for assessing the epidemic risk state and its change trend of hospitals in underdeveloped areas. Through assessment, key factors affecting hospital epidemic risk in different epidemic stages will be identified, thereby providing reference for hospital risk prevention and control.
2 Methods
2.1 Epidemic risk assessment indicators
In order to assess the epidemic risk state of hospitals in underdeveloped areas, it is necessary to first sort out the risk indicators that affect the changes in hospital epidemic risk. Therefore, this paper selected medical staff from five hospitals located in underdeveloped areas of China as the interviewees, with a total of 48 interviewees. These interviewees have varying years of work experience, including managers from different hospital departments, front-line medical staff, and epidemic prevention experts involved in COVID-19 prevention and control work between 2019 and 2022. This survey involved three rounds of consultation, with 48 questionnaires issued in each round. The expert positive coefficient of respondents in all three rounds was more significant than 0.9. Apart from the interviews and surveys, this paper also summarizes and analyzes the emergent problems and potential risks that hospitals face during major health events. This analysis draws on relevant literature and media reports of significant health events from the past decade, including literature related to the COVID-19 pandemic, influenza outbreaks, and endemic disease outbreaks; this paper also paid attention to the management announcements and logs released on the official websites of different district and county hospitals during the outbreak period, and sorted out hospitals' relevant internal management information in response to the outbreak. As mentioned above, this paper collected data from multiple sources, which can analyze the risk factors that affect hospital safety from different perspectives, and cross-validate the analysis results.
Through collection, this paper has collected over 30,000 words of various types of raw data. After collecting the above raw data, this paper intends to extract a set of available hospital epidemic risk assessment indicators from the raw data based on Grounded theory. Grounded theory is a qualitative research method aimed at continuously refining core concepts based on experience and data, and ultimately classifying and summarizing relevant concepts to construct a usable theory (27). Based on Grounded theory, this paper first encodes the raw data according to different sources, using the letter Q to identify data from questionnaires, using the letter I to identify data from literature and reports, and using the letter R to identify data from hospital management materials and announcement logs. After encoding, this paper eliminated semantic duplicates through expert discussion and analysis, and sorted out 234 non-duplicate data. This paper randomly selected 170 pieces of data for constructing the epidemic risk indicator system, and the remaining 64 pieces of data were used to test the theoretical saturation of the indicators (28). Next, through semantic extraction and induction, this paper extracted 35 initial concepts with concise semantics and clear descriptions from the selected 170 pieces of data. From the perspective of hospital risk management, these initial concepts were further classified and summarized, resulting in nine categories, namely nine epidemic risk assessment indicators, as shown in Table 1.
Finally, to verify whether the initial concepts and categories extracted are theoretically saturated, this paper extracted and summarized the remaining 64 data, and no new concepts or categories were found, indicating that the epidemic risk assessment indicators constructed in this paper are theoretically saturated.
2.2 Epidemic risk state and its random state space
The common risk assessment usually establishes a risk matrix from two perspectives: the probability of risk occurrence and the severity of risk losses (29, 30), thus defining the level of risk. The results obtained through the above assessment can intuitively and quantitatively describe the size of the risk. However, in practical situations, the epidemic risks faced by hospitals cannot remain unchanged due to the influence of various factors. If a fixed risk level is used to describe the risk, it is obviously insufficient to effectively describe the real hospital epidemic risk environment. Therefore, after sorting out the indicators of hospital epidemic risk through Grounded theory, in order to effectively describe the process of epidemic risk changes and achieve the assessment of risk changes, this paper defines four common discrete states Sn, n = 1, 2, 3, 4 from two aspects: the probability of risk occurrence and the severity of risk losses. The meanings of each state are as follows.
(1) S1, risk disappearance state. This state is extremely safe, indicating that the risk is almost non-existent and the resulting losses can be ignored.
(2) S2, risk potential state. This state is relatively safe, indicating that the risk of the epidemic has already existed but has not caused significant damage. At this point, there is a small probability that the risk will occur, and the resulting losses will have a small impact, which can be quickly recovered.
(3) S3, risk critical state. This is a relatively dangerous state, indicating that the risk has clearly existed and the epidemic is on the verge of outbreak, causing certain losses. At this point, there is a high probability that the risk will occur and cause certain risk losses, which will take a long time to recover.
(4) S4, risk outbreak state. This state is very dangerous, indicating that the epidemic risk has fully erupted and caused extremely serious losses, and the damage caused is difficult to recover.
These above four states cover the entire process of changes in epidemic risk. The probability that the epidemic risk belongs to state Sn is represented by P(Sn), and the epidemic risk state at time t can be expressed as ,. It is known that in practical situations, the epidemic risk is always constantly changing due to the impacts of various risk indicators. It will randomly transition between the 4 states mentioned above, as shown in Figure 1.
In Figure 1, the four risk states are mutually reachable, together forming the random state space of epidemic risk (31). Under the influence of various indicators, the actual epidemic risk in the actual situation will be randomly changing, and the entire transformation process is a random change process. Therefore, in order to effectively measure the changes in the epidemic risk state, this paper intends to adopt the Markov chain prediction method to assess the epidemic risk state and its change. Markov chain have a mathematical definition and are classic theories in probability theory and mathematical statistics. It can effectively describe the random state of things and is suitable for predicting changes in the random state of things (32).
In order to effectively assess the random transition process of the epidemic risk state under the comprehensive influence of various indicators, this paper intends to use matrix to describe the random transition process of the epidemic based on Markov chain theory. As shown below.
The element Sn→m in the matrix represents the process of the epidemic risk transition from state Sn to Sm. The meanings of each element Sn→m are shown in Table 2.
2.3 Assessment of the impact of various indicators on hospital risk state
After defining the epidemic risk state and its random state space, this paper will comprehensively assess the impact of each indicator on the epidemic risk state from two aspects: the probability of risk occurrence and risk loss. The level definition of the risk occurrence probability P(aj) and risk loss L(aj) for each indicator aj are shown in Table 3.
Next, based on the definition of the four states of epidemic risk in this paper, the probability level P(aj) and risk loss level L(aj) of the risk occurrence are substituted into the risk matrix (33), which can intuitively and effectively distinguish different epidemic states, as shown in Table 4.
As shown in Table 4, the higher the P(aj) and L(aj), the higher the probability of risk occurrence and the greater the loss caused by the indicator. This indicator aj will lead the hospital risk state to transition toward a more dangerous risk state. As mentioned above, it is only necessary to assess the probability level P(aj) and risk loss level L(aj) of each indicator, the comprehensive impact of each indicator aj on the hospital's epidemic risk state can be determined. However, during the assessment process, due to the lack of expert experience, it is difficult to provide accurate judgments on P(aj) and L(aj) of a certain indicator, which can easily lead to inaccurate assessment results. To reduce the difficulty of expert assessment and ensure the accuracy of the assessment results, this paper proposes an epidemic risk state assessment method based on a risk matrix. As shown in Figure 2, experts only need to provide the value range of P(aj) and the value range of L(aj), thus the impact of indicator aj on risk state can be assessed.
In Figure 2, Pmax(aj) represents the maximum level of risk occurrence probability for aj, while Pmin(aj) represents the minimum level of risk occurrence probability for aj.Lmax(aj) represents the maximum level of risk loss for aj, while Lmin(aj) represents the minimum level of risk loss for aj. The following concepts can be derived from Figure 2.
(1) Square(aj) is a rectangular area composed of four points, Pmin(aj), Pmax(aj), Lmin(aj), and Lmax(aj).
(2) Square(Sn) represents the area occupied by risk state Sn in the risk matrix.
(3) The intersection of Square(aj) and Square(Sn) indicates the possibility that aj belongs to Sn. If Square(aj)∩Square(Sn) = 0, then the probability of aj belonging to Sn is 0.
According to the above concepts, dividing the intersection of Square(aj) and Square(Sn) by Square(aj) can calculate the probability that aj belongs to the risk state Sn, as shown in Formula (1).
In Formula (1), represents the probability that aj belongs to the risk state Sn. The larger its value, the greater the probability that aj belongs to Sn, indicating a higher probability of the epidemic risk state transitioning to Sn under the influence of aj.
As mentioned above, through the method proposed in this paper, experts do not need to provide an exact numerical value for the frequency level or loss severity level of each risk indicator aj in the assessment process. Experts only need to provide the value range [Pmin(aj), Pmax(aj)] and [Lmin(aj), Lmax(aj)] for each indicator according to the definition in Table 3, thus can calculate the value of according to Formula (1). effectively reflects the impact of indicator aj on the risk state of the epidemic. This method reduces the difficulty of expert assessment and effectively achieves the assessment of the impact of each indicator on the epidemic risk state.
2.4 Assessment of the change trend of epidemic risk state
It is known that represents the probability that indicator aj belongs to the risk state Sn. When > 0, it indicates that under the influence of aj, the epidemic risk state of the hospital may transition to Sn. Therefore, this paper intends to comprehensively assess the impact of aj on the epidemic risk state of hospitals, and further assess the changes in the overall epidemic risk state of hospitals. As shown in Formula (2).
In Formula (2), represents the total probability of the epidemic risk state transitioning from Sn to Sm due to the comprehensive impact of indicator aj. total represents the total number of risk indicators aj. For example, when n = 1, the values of , and can be calculated sequentially according to Formula (2). Finally, according to Formula (2, the following matrix can be calculated.
This matrix corresponds to the random state space of epidemic risk proposed in Section 2.2 of this paper, which describes the random transition probability of the epidemic risk state under the influence of various indicators. Next, normalize the elements in each row of the above matrix, and the Markov chain transition matrix of the epidemic state can be obtained. The matrix is shown below.
STM is the state transition matrix of epidemic risk. According to Markov chain theory, once the state transition matrix of epidemic risk is established, the stable state of things in long-term random changes can be predicted (34). The calculation formula is as follows.
In Formula 3, represents the epidemic risk state at time t, and represents the epidemic risk state at time t+k. k is the number of state transitions, and k > 0. According to Markov chain theory, when the value of k is sufficiently large or approaches infinity, will tend to stabilize and no longer change. The risk state at this time is called the stable state of epidemic risk, denoted as . is the state where the epidemic risk state is most likely to transition. It represents the change trend of the epidemic risk state under the comprehensive influence of various indicators in a long-term risk environment. Therefore, by calculating the , it will be possible to grasp the future risk trends of hospitals and effectively predict the future hospital epidemic risk state.
At this point, this paper proposes the concept of epidemic risk state, and combines risk matrix and Markov chain to propose an assessment method for epidemic risk state and its change trend. In subsequent chapters, this paper will use this method to assess the risk state and its change trend of hospitals in underdeveloped areas. The above methods were carried out in accordance with relevant guidelines and regulations, and did not involve experiments on humans and/or the use of human tissue samples.
3 Results
Hospitals in underdeveloped areas of China, due to their inadequate conditions, are unable to effectively respond to sudden epidemics, which can easily lead to the spread of the epidemic from remote areas and cause extremely serious local epidemic disasters. In order to effectively assess the epidemic risk state and its change trend of such hospitals, this paper selected 10 personnel with hospital risk control experience as assessment experts. These 10 experts are five hospital managers and five epidemic prevention experts from research institutes among the 48 respondents mentioned above. Based on the risk indicators proposed in this paper, through research and expert discussions on five county-level hospitals in underdeveloped areas of southwestern China, the risk indicator characteristics of public hospitals in these underdeveloped areas were summarized, as shown in Table 5.
Firstly, this paper selected 10 personnel with hospital risk management experience as assessment experts. In order to identify the key factors affecting the hospital epidemic risk state change in the region, this paper intends to assess the risk state of hospitals in different epidemic stages separately. These three stages are the non-epidemic stage t0, the early epidemic stage t1, and the epidemic outbreak stage t2.
According to the assessment method proposed in Section 2.3, 10 experts assessed the risk occurrence probability level and risk loss level of each indicator in the above three stages, and the results are shown in Table 6.
The data in Table 6 are the value range given by experts for the risk occurrence probability level and risk loss level of various indicators. Next, substitute Table 6 data into Formula 1, the of each indicator can be calculated, as shown in Table 7.
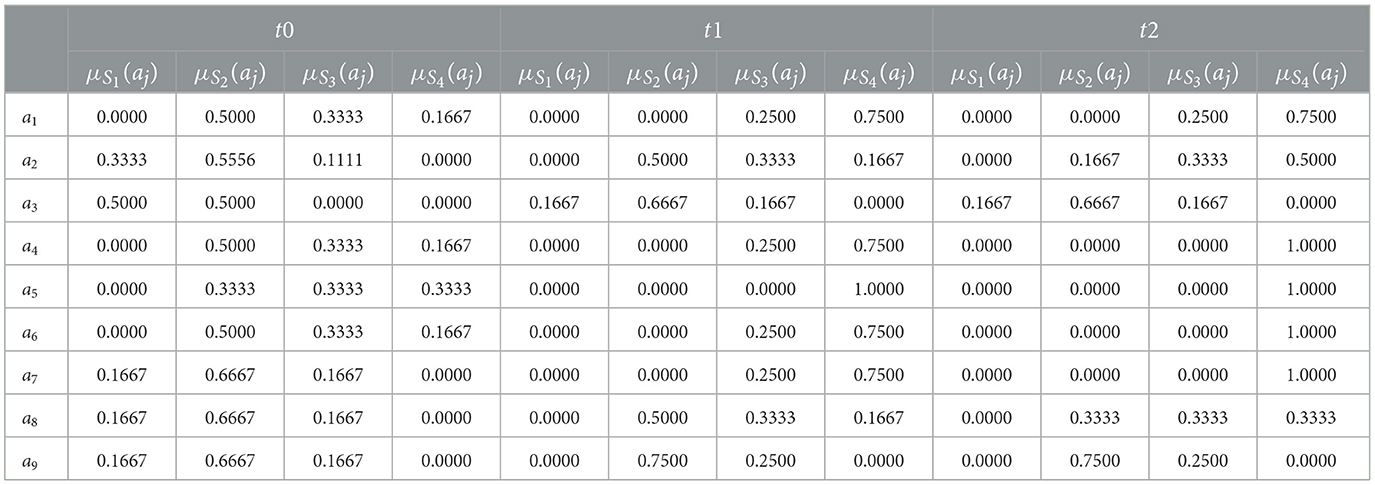
Table 7. Assessment results of each indicator's impact on the epidemic risk state in different epidemic stages.
In Table 7, represents the probability of each indicator belonging to different epidemic risk states during different epidemic stages. For example, in the t0 stage, when μS3(a1)>0, it indicates that under the influence of a1, the overall epidemic risk state may transition to S3. Next, by substituting Table 7 data into Formula 2 and normalizing it, the state transition matrices of hospital epidemic risk in three different stages can be obtained. The results are shown below.
After calculating the risk state transition matrix STM of hospitals in different epidemic stages, according to Formula 3, it can be concluded that the final stable risk state of the epidemic only depends on STM and is independent of the risk state at time t. Therefore, it can be assumed that in the t0, t1, and t2 stages, the epidemic risk state of hospitals in the region is , and . According to Formula 3, the epidemic stable risk states in three different stages can be calculated as , , and , respectively. The change trend of the epidemic risk state in these three stages is shown in Figure 3.
4 Discussion
4.1 Epidemic risk state and its change trend in different stages
It can be inferred from the assessment results of the risk state and its change trend in the three stages mentioned above.
(1) In stage t0, the value of is significantly highest, indicating that there is a high probability that the epidemic risk state of hospitals in the region will transition toward S2 during non-epidemic stage. In the non-epidemic stage, these hospitals are in a relatively safe risk state, but there is already a relatively small epidemic risk in these hospitals.
(2) In stage t1, the high values of and indicate a high probability that these hospitals' risk state will transition toward S2 or S4 in the early epidemic stage. In the early epidemic stage, hospitals in the region faced significant risks and hidden dangers. The values of and are relatively close, indicating that these hospitals may transition toward the high-risk state of S4 if there is a slight mistake at this stage.
(3) In stage t2, the value is significantly highest, indicating a high probability that these hospitals' risk state will transition toward S4 during the epidemic outbreak stage. During the epidemic outbreak stage, hospitals in the region are facing extremely serious risks, with a high probability of suffering serious losses. The values of and are both small, indicating that if hospitals in the region do not make timely adjustments at this stage, there is only a small probability that they can recover to a safe state.
As mentioned above, in the t0 stage, hospitals in the region are in a relatively safe state, but there is always a potential small risk of the epidemic. In stages t1 and t2, there is a high probability that the epidemic risk state will transition toward a more dangerous state.
4.2 Risk control priority analysis in different stages
Next, in order to assist the hospital in reasonable risk management in different stages, this paper intends to use the proposed assessment method to compare the effectiveness of different risk management plans, identify key risk indicators that affect these hospitals' risk state change, and provide priority reference for risk management.
In stages t1 and t2, considering that the impact of risk loss in the short term is not easily changed in the actual risk control process, hospitals can only reduce the risk occurrence probability to a certain extent. Therefore, in the assessment process of each indicator control plan, this paper intends to reduce the minimum occurrence probability level Pmin(aj) and the maximum occurrence probability level Pmax(aj) by one level, respectively, to simulate different risk indicator control plans.
Finally, the proposed method was used to assess the risk state after different controls, and the results are shown in Tables 8, 9.
According to the assessment data shown in Table 8, if , it indicates that the control of indicator Cj is effective in stage t1, which can enable the hospital to transition toward a safety risk state, as shown in Figure 4. By comparison, the following conclusions can be drawn.
(1) In the early epidemic stage, risk indicators that can improve these hospitals' risk state include: C2, C3, C8, andC9.
(2) In the early epidemic stage, risk control for C1, C4, C5, C6, or C7 will not effectively improve these hospitals' epidemic risk state.
(3) In the early epidemic stage, compared to other risk indicators, risk control for C2 and C8 is the most effective way to improve the hospital's epidemic risk state. This indicates that in the early epidemic stage, hospitals should prioritize the training of epidemic prevention skills for medical staff and the management of public health in hospitals. Secondly, it is the risk control for C3, namely the management of work rights, work responsibilities and performance assessment during the epidemic period. Finally, it is the risk control for C9, namely strengthening the epidemic supervision and management in hospitals.
According to the assessment data shown in Table 9, if , it indicates that the control of indicator Cj is effective in stage t2, which can enable these hospitals to transition toward a safety risk state, as shown in Figure 5. By comparison, the following conclusions can be drawn.
(1) In the epidemic outbreak stage, risk indicators that can improve these hospitals' risk state include: C1, C2, C3, C4, C6, C7, and C9.
(2) In the epidemic outbreak stage, risk control for C5 or C8 will not effectively improve these hospitals' epidemic risk state.
(3) In the epidemic outbreak stage, hospitals in the region first need to carry out risk control for C2 and C1, that is, prioritize training on epidemic prevention skills and psychological awareness for medical staff. Secondly, it is necessary to carry out risk control for the C3, namely strengthen the management of work rights, responsibilities, and performance evaluation during the epidemic period. Finally, it is also necessary to pay attention to the supply of emergency supplies in hospitals C4, the preparation of emergency personnel C6, and the management of public service facilities in hospitals C7.
The next step is to analyze the risk control plan for non-epidemic stage t0. In order to provide a priority reference for the risk control of hospitals in the region, this paper uses the same method to reduce the minimum probability level Pmin(aj) and maximum probability level Pmax(aj) of each risk indicator by one level. In this stage, if the Pmin(aj) of a certain risk indicator is already level 1, it will not decrease. Finally, by assessing the risk state after different controls, the results are shown in Table 10.
According to the assessment data shown in Table 10, if , it indicates that the control of indicator Cj is effective in stage t0, which can enable these hospitals to transition toward a safety risk state, as shown in Figure 6. By comparison, the following conclusions can be drawn.
(1) In the non-epidemic stage, risk indicators that can improve these hospitals' risk state include: C1, C2, C3, C4, C5, C6, C7, C8, andC9.
(2) In the non-epidemic stage, risk control for C5 is the most effective, indicating that in the non-epidemic stage, the preparation of emergency funds in hospitals is the most important for ensuring hospital safety. In addition, training on the psychology and awareness of medical staff C1, the supply of emergency supplies C4, and the preparation of emergency personnel C6 are also important for ensuring hospital safety.
In summary, hospitals in underdeveloped areas should have different control strategies at different epidemic stages, that is, risk control for each indicator should have different priorities. By assessing the epidemic risk state and its change trend in different stages, scientific and reasonable risk control can be carried out (35). On the contrary, in the absence of effective assessment data, blind risk control may not be efficient and may even lead epidemic risk state to transition toward more dangerous state.
The method proposed in this paper takes into account the randomness and uncertainty of epidemic risk (36), viewing epidemic risk as a stochastic process under the influence of various risk indicators, and defining four random states of epidemic risk, achieving dynamic assessment of epidemic risk status and its change trend. Compared to general static risk assessment methods, this method establishes a random risk state space for the epidemic based on Markov theory, which is closer to the real epidemic risk environment. The assessment results can more effectively reflect the real epidemic risk situation in different periods, and the assessment results provided have higher support for decision-making.
5 Conclusion
This paper proposes the concept of epidemic risk state and proposes an effective assessment method for epidemic risk state and its change trend based on the risk matrix and Markov chain. Through assessment, reasonable priority references have been provided for risk management in underdeveloped area hospitals at different epidemic stages. For hospitals in underdeveloped areas, in the non-epidemic stage, hospitals should prioritize the preparation of emergency funds. In the early epidemic stage, hospitals should prioritize the training of epidemic prevention skills for medical staff and public health management in hospitals. During the epidemic outbreak stage, hospitals should prioritize training medical staff in epidemic prevention skills and psychological awareness. By using the method proposed in this paper to assess the epidemic risk state and its change trend in different stages, hospitals can effectively strengthen their ability to respond to sudden epidemics by implementing reasonable risk control measures in different stages. In subsequent research, in order to narrow the gap between the proposed method's assessment results and the actual epidemic risk state, it is necessary to further improve the epidemic risk indicator system established in this paper, establish a multi-level risk indicator system, and establish risk evidence corresponding to the risk occurrence probability and risk loss of each indicator. By assessing the risk level of each indicator through risk evidence, the accuracy and objectivity of the assessment can be improved.
Data availability statement
The original contributions presented in the study are included in the article/Supplementary material, further inquiries can be directed to the corresponding author.
Ethics statement
This study falls under the category of routine risk management policy evaluation in hospitals, does not require ethical approval or consent, all methods used in this paper were carried out in accordance with relevant guidelines and regulations, and all interview surveys in the study have obtained verbal authorization from all interviewees.
Author contributions
MY: Funding acquisition, Writing – original draft, Writing – review & editing. JM: Conceptualization, Data curation, Writing – original draft. TL: Data curation, Methodology, Validation, Writing – review & editing. RJ: Formal analysis, Funding acquisition, Investigation, Methodology, Writing – review & editing. MJ: Data curation, Investigation, Writing – review & editing.
Funding
The author(s) declare financial support was received for the research, authorship, and/or publication of this article. This research was supported by the National Natural Science Foundation of China (No. 72261033), Yunnan Fundamental Research Projects (Nos. 202101AT070211, 202201AT070142), and the Foundation of Yunnan Key Laboratory of Service Computing (No. YNSC23110).
Conflict of interest
The authors declare that the research was conducted in the absence of any commercial or financial relationships that could be construed as a potential conflict of interest.
Publisher's note
All claims expressed in this article are solely those of the authors and do not necessarily represent those of their affiliated organizations, or those of the publisher, the editors and the reviewers. Any product that may be evaluated in this article, or claim that may be made by its manufacturer, is not guaranteed or endorsed by the publisher.
Supplementary material
The Supplementary Material for this article can be found online at: https://www.frontiersin.org/articles/10.3389/fpubh.2024.1384118/full#supplementary-material
References
1. Chen L, Wu Y, Li J, Zhu L. Development of informatization in primary care in China's regions inhabited by ethnic minorities. Chin General Pract. (2022) 25:2003–7. doi: 10.12114/j.issn.1007-9572.2022.0139
2. Gao H, Chen H, Feng J, Qin X, Wang X, Liang S, et al. Balanced scorecard-based performance evaluation of Chinese county hospitals in underdeveloped areas. J Int Med Res. (2018) 46:1947–62. doi: 10.1177/0300060518757606
3. Liguo Z, Xu-hua G, Shu-qiong H. Characteristics of public health emergencies in Hubei province, 2008-2014. Chin J Public Health. (2016) 32:521–3. doi: 10.11847/zgggws2016-32-04-28
4. Lu B, Lv P, Wu X, Bai H, Yang F, Zhu J. Research on strategies of public hospitals to deal with major public health emergencies under 3S theory. Chin Hosp. (2023) 27:19–21. doi: 10.19660/j.issn.1671-0592.2023.03.05
5. Liu L, Zhang B, Yang Z. Investigation on medical workers' core emergency response competences in epidemics of infectious diseases. J Nurs Sci. (2019) 34:75–7.
6. LeBlanc VR, Regehr C, Tavares W, Scott AK, Macdonald R, King K. The impact of stress on paramedic performance during simulated critical events. Prehosp Disaster Med. (2012) 27:369–74. doi: 10.1017/S1049023X12001021
7. Kyron MJ, Rees CS, Lawrence D, Carleton RN, McEvoy PM. Prospective risk and protective factors for psychopathology and wellbeing in civilian emergency services personnel: a systematic review. J Affect Disord. (2020) 281:517–32. doi: 10.1016/j.jad.2020.12.021
8. Zhou Y, Wang W, Sun Y, Qian W, Liu Z, Wang R, et al. The prevalence and risk factors of psychological disturbances of frontline medical staff in china under the COVID-19 epidemic: Workload should be concerned. J Affect Disord. (2020) 277:510–4. doi: 10.1016/j.jad.2020.08.059
9. Brandão CFS, Bergamasco EC, Vaccarezza GF, Barba MLF, Andrade EFM, Cecilio-Fernandes D. Training in healthcare during and after COVID-19: proposal for simulation training. Rev Associação Méd Bras. (2021) 67:12–7. doi: 10.1590/1806-9282.67.suppl1.20200710
10. Gail TM, MacKenzie A, Alder R, Langley J, Hickey M, Cook A. Pilot-testing an applied competency-based approach to health human resources planning. Health Policy Plann. (2013) 28:739–49. doi: 10.1093/heapol/czs115
11. Shu L, Ji N, Chen X, Feng G. Ark of life and hope: the role of the Cabin hospital in facing COVID-19. J Hosp Infect. (2020) 105:351–2. doi: 10.1016/j.jhin.2020.03.032
12. Liu P, Zhang H, Long X, Wang W, Zhan D, Meng X, et al. Management of COVID-19 patients in Fangcang shelter hospital: clinical practice and effectiveness analysis. Clin Respir J. (2021) 15:280–6. doi: 10.1111/crj.13293
13. Azuma K, Yanagi U, Kagi N, Kim H, Ogata M, Hayashi M. Environmental factors involved in SARS-CoV-2 transmission: effect and role of indoor environmental quality in the strategy for COVID-19 infection control. Environ Health Prev Med. (2020) 25:1–16. doi: 10.1186/s12199-020-00904-2
14. Song J, Zhang F, Zhou H, Wang Y, Wu X, Wenhan Q, et al. Research on emergency medical resources allocation management for dealing with serious emerging infectious diseases. Chin Hosp Manag. (2021) 42:9–12.
15. Weiping W, Minglei Z, Minna F. Research on the problems and countermeasures of emergency fund management and use for public emergencies in China. Rev Econ Res. (2017) 2017:11–4. doi: 10.16110/j.cnki.issn2095-3151.2017.33.002
16. Campos AT, Dos Santos CH, Gabriel GT, Montevechi JAB. Safety assessment for temporary hospitals during the COVID-19 pandemic: a simulation approach. Saf Sci. (2022) 147:105642. doi: 10.1016/j.ssci.2021.105642
17. Rana W, Mukhtar S, Mukhtar S. Job satisfaction, performance appraisal, reinforcement and job tasks in medical healthcare professionals during the COVID-19 pandemic outbreak. Int J Health Plann Manage. (2022) 37:2345–53. doi: 10.1002/hpm.3476
18. Kabego L, Kourouma M, Ousman K, Baller A, Milambo J-P, Kombe J, et al. Impact of multimodal strategies including a pay for performance strategy in the improvement of infection prevention and control practices in healthcare facilities during an Ebola virus disease outbreak. BMC Infect Dis. (2023) 23:1–7. doi: 10.1186/s12879-022-07956-5
19. Shen Y, Cui Y, Li N, Tian C, Chen M, Zhang Y-W, et al. Emergency responses to Covid-19 outbreak: experiences and lessons from a general hospital in Nanjing, China. Cardiovasc Intervent Radiol. (2020) 43:810–9. doi: 10.1007/s00270-020-02474-w
20. Zhi-jun Q, Xiao-hong H, Ting Y. Efficiency and deficiency of hospital infection management based on monitoring data. Chin Hosp Manag. (2019) 39:49–50.
21. Shi R, Lu B, Zhong Y. Driving factors of urban community epidemic prevention and control capability: QCA analysis based on typical cases of 20 anti-epidemic communities in China. Front Public Health. (2024) 11:1296269. doi: 10.3389/fpubh.2023.1296269
22. Bellini L, Bossi E, Bucci D, Caprara M, Chiappa F, Ambrosio A. Preventive and responsive plans to COVID-19 epidemic in a leading teaching hospital in Milan, Italy. Eur J Public Health. (2020) 30(Suppl_5):618. doi: 10.1093/eurpub/ckaa166.618
23. Olayinka F, Sauer M, Menning L, Summers D, Wonodi C, Mackay S, et al. Improving community resilience through the development of an epidemic risk and priority framework. Int J Infect Dis. (2019) 79:25–6. doi: 10.1016/j.ijid.2018.11.077
24. Xuesong X, Yajie P, Xiaohong C, Dong X, Yingjie W, Chuhan Y. Construction of risk assessment model for sudden compound epidemic based on granular computing. Syst Eng Theory Pract. (2023) 43:583–97. doi: 10.12011/SETP2022-0646
25. Ajisegiri WS, Chughtai A, MacIntyre CR. A risk analysis approach to prioritizing epidemics: Ebola Virus Disease in West Africa as a case study. Risk Analysis. (2018) 38:429–41. doi: 10.1111/risa.12876
26. Mao Q, Chen J, Lv J, Chen S. Emergency plan selection for epidemic prevention and control based on cumulative prospect theory and hybrid-information MADM. Kybernetes. (2023) 52:1903–33. doi: 10.1108/K-08-2021-0736
27. Khan SN. Qualitative research method: grounded theory. Int J Bus Manage. (2014) 9:224–33. doi: 10.5539/ijbm.v9n11p224
28. Aldiabat KM, Le Navenec C-L. Data saturation: the mysterious step in grounded theory methodology. Qual Rep. (2018) 23:245–61. doi: 10.46743/2160-3715/2018.2994
29. Ratnayake R, Antosz K. Development of a risk matrix and extending the risk-based maintenance analysis with fuzzy logic. Proc Eng. (2017) 182:602–10. doi: 10.1016/j.proeng.2017.03.163
30. Albery S, Borys D, Tepe S. Advantages for risk assessment: evaluating learnings from question sets inspired by the FRAM and the risk matrix in a manufacturing environment. Saf Sci. (2016) 89:180–9. doi: 10.1016/j.ssci.2016.06.005
31. Yang M, Jia L, Xie W, Gao T. Research on risk assessment model of epidemic diseases in a certain region based on markov chain and ahp. IEEE Access. (2021) 9:75826–39. doi: 10.1109/ACCESS.2021.3081720
32. Gao T, Jia X, Jiang R, He Y, Yang M. SaaS service combinatorial trustworthiness measurement method based on markov theory and cosine similarity. Sec Commun Netw. (2022) 2022:7080367. doi: 10.1155/2022/7080367
33. Zhu Q, Kuang X, Shen Y. Risk matrix method and its application in the field of technical project risk management. Eng Sci. (2003) 5:89–94.
34. Yang M, Jiang R, Gao T, Xie W, Wang J. Research on cloud computing security risk assessment based on information entropy and Markov Chain. Int J Netw Sec. (2018) 20:664–73. doi: 10.6633/IJNS.20180720(4).08
35. Liu Y, Zheng F, Du Z, Li J, Gu J, Jiang M, et al. Evaluation of China's Hubei control strategy for COVID-19 epidemic: an observational study. BMC Infect Dis. (2021) 21:820. doi: 10.1186/s12879-021-06502-z
Keywords: epidemic risk, risk state, public hospital, risk assessment, hospital risk
Citation: Yang M, Miao J, Li T, Jiang R and Jiang M (2024) Assessment of epidemic risk state and its change trend of public hospital in underdeveloped area in different stages. Front. Public Health 12:1384118. doi: 10.3389/fpubh.2024.1384118
Received: 21 February 2024; Accepted: 16 July 2024;
Published: 06 August 2024.
Edited by:
Shusen Sun, Western New England University, United StatesReviewed by:
Nirmal Kumar Ganguly, Indraprastha Apollo Hospitals, IndiaRichard M. Mariita, Crystal IS Inc., United States
Copyright © 2024 Yang, Miao, Li, Jiang and Jiang. This is an open-access article distributed under the terms of the Creative Commons Attribution License (CC BY). The use, distribution or reproduction in other forums is permitted, provided the original author(s) and the copyright owner(s) are credited and that the original publication in this journal is cited, in accordance with accepted academic practice. No use, distribution or reproduction is permitted which does not comply with these terms.
*Correspondence: Tiebing Li, zz1121@ynufe.edu.cn