- 1Changchun University of Traditional Chinese Medicine, Changchun, Jilin, China
- 2Affiliated Hospital of Changchun University of Traditional Chinese Medicine, Changchun, Jilin, China
Objective: This study aimed to determine the risk factors associated with fluctuations in nucleic acid CT values in patients infected with the Omicron variant during an outbreak at a hospital in Changchun city.
Methods: A retrospective analysis was conducted on general information, medical history, vaccination history, and laboratory test data of COVID-19 patients infected with the Omicron variant and admitted to the hospital in Changchun from March 2022 to April 2022. The study aimed to explore the factors influencing nucleic acid CT value fluctuations in COVID-19 patients infected with the Omicron variant in Changchun city.
Results: Fluctuations in nucleic acid CT values were significantly correlated with occupation composition (p = 0.030), hospital stay duration (p = 0.000), heart rate (p = 0.026), creatinine (p = 0.011), platelet count (p = 0.000), glutamic-pyruvic transaminase (p = 0.045), and glutamic oxaloacetic transaminase (p = 0.017). Binary logistic regression analysis revealed significant correlations between hospital stay duration (p = 0.000), platelet count (p = 0.019), heart rate (p = 0.036), and nucleic acid CT value fluctuations (p < 0.05), indicating that they were independent risk factors. Red blood cell count was identified as a factor influencing nucleic acid CT value fluctuations in Group A patients. Occupation composition, direct bilirubin, and platelet count were identified as factors influencing nucleic acid CT value fluctuations in Group B patients. Further binary logistic regression analysis indicated that occupational composition and direct bilirubin are significant independent factors for nucleic acid CT value fluctuations in Group B patients, positively correlated with occupational risk and negatively correlated with direct bilirubin.
Conclusion: Therefore, enhancing patients’ immunity, increasing physical exercise to improve myocardial oxygen consumption, reducing the length of hospital stays, and closely monitoring liver function at the onset of hospitalization to prevent liver function abnormalities are effective measures to control fluctuations in nucleic acid CT values.
1 Introduction
In December 2019, the emergence of the novel coronavirus pneumonia (COVID-19, 2019-nCoV, or SARS-CoV-2) continued to perplex the entirety of human society. It has impacted not only the healthcare systems but also the equilibrium of the global socio-economic landscape (1, 2). The Omicron variant, known for its high transmissibility and immune evasion capabilities (3), can rapidly become the dominant strain in a region. However, it primarily infects the upper respiratory tract rather than the lower respiratory tractt (4), resulting in decreased pathogenicity and slower spread from the main bronchi to the distal bronchioles, leading to significant improvements in pulmonary histopathology and lung disease (5–7). The majority of patients may experience mild symptoms or remain asymptomatic (8, 9). Studies have indicated that Omicron possesses enhanced contagiousness and the ability to evade vaccine protection (10, 11). Real-time reverse transcription polymerase chain reaction (RT-PCR) can be employed for screening and diagnosing Omicron infections, closely associated with viral culture (12, 13). The virus may still be detected in individuals even 0–2 days after symptom resolution (14). Thus, monitoring the fluctuation of the patient’s nucleic acid cycle threshold (CT) values is crucial.
Over the past 3 years, there have been recurrent small-scale outbreaks of the pandemic, which have significantly affected the physical and mental health of the population. Research has revealed that a majority of individuals have developed a fear of the Omicron variant (15), with a temporary increase in emotional and anxiety disorders (16), leading to long-lasting psychological sequelae, albeit unevenly distributed (17). However, under the anti-pandemic measures implemented in China, it has been observed that the Chinese population’s fear of the Omicron variant has gradually diminished, while positive emotions have increased (18). As the number of infected individuals continues to rise, our clinical observations have indicated that fluctuations in the nucleic acid CT values of hospitalized COVID-19 patients can have an impact on their mood, potentially prolonging their recovery time and impacting their health. A plethora of systematic reviews and observational studies have established that nucleic acid CT values are not only correlated with the progression to severe illness, disease severity, and clinical biomarkers (19–21), but also bear significant associations with demographic factors and clinical histories (22–24). It has been observed that males exhibit higher viral loads compared to females (25), which can predict their infectiousness (26) and potentially reduce the likelihood of transmission (27). Consequently, CT values can serve as prognostic markers at an individual level (28, 29), identifying patients with higher morbidity and risk (30). Therefore, it is crucial to consider nucleic acid CT values in the management of COVID-19 infections (31), and to emphasize the importance of early testing (32). Further research indicates that fluctuations in CT values are linked with respiratory symptoms, comorbidities, and abnormalities in chest imaging (33), and may exacerbate the incidence of depression and insomnia (34). Prompt attention to the dynamic changes in CT values (35) will aid in monitoring the infection status of patients (36).
Therefore, this study aims to retrospectively analyze general information, medical history, vaccination records, laboratory tests, and other data of COVID-19 patients infected with the Omicron variant in Changchun, Jilin Province, China, in March 2022. Through statistical analysis of the collected data, we aim to explore the factors associated with fluctuations in patients’ nucleic acid CT values.
2 Materials and methods
2.1 General data of patients
The diagnostic criteria and fluctuation of nucleic acid CT values are referenced from the Diagnosis and Treatment Protocol for Novel Coronavirus Pneumonia (Trial Version 9) issued by the National Health Commission and the National Administration of Traditional Chinese Medicine (37). All COVID-19 patients undergo nucleic acid testing conducted by professionals at the same time and location, using specialized nucleic acid sampling techniques. The tests are uniformly processed using machines and reagent kits from the same batch and manufacturer. The Fluorescent Quantitative PCR method is employed, and results are interpreted strictly according to the kit’s specifications. The process includes initial screening, retesting, and CDC review to minimize potential errors. All test results adhere to the guidelines set forth in the “Diagnosis and Treatment Protocol for Novel Coronavirus Pneumonia (Trial Version 9)” issued by the National Health Commission and the National Administration of Traditional Chinese Medicine. The term “nucleic acid CT value fluctuation” refers to the scenario where a patient’s initial post-admission nucleic acid test is negative, marked as Day 1 with a CT value greater than 35, and the Day 2 test also shows a CT value greater than 35, but on Day 3, the CT value drops below 35, leading to a delayed discharge termed as nucleic acid CT value fluctuation.
2.2 Laboratory index and TCM diagnosis
Collection of general information, medical history, vaccination history, and laboratory examination data of COVID-19 patients who were hospitalized and infected with the Omicron variant at the Affiliated Hospital of Changchun University of Traditional Chinese Medicine from March 2022 to April 2022. Based on the results of the patients’ nucleic acid tests, they were divided into two groups: the nucleic acid CT value fluctuation group and the nucleic acid CT value non-fluctuation group. Patients were further divided based on the length of hospital stay: those hospitalized for more than 10 days were placed in Group A, while those hospitalized for 10 days or less were placed in Group B.
2.3 Statistical analysis
A database was constructed using WPS 2023 spreadsheet, and SPSS 20.0 was used for statistical analysis. Descriptive statistics such as frequency and percentage were used for categorical data, and the chi-square test was used for between-group comparisons. For normally distributed continuous data, mean and standard deviation were used for description, while non-normally distributed continuous data were described using median [P25, P75]. Logistic regression analysis was utilized to identify risk factors of nucleic acid CT value fluctuation. A significance level of p < 0.05 was considered statistically significant.
2.4 Ethical review
This study has been approved by the Ethics Committee of the Affiliated Hospital of Changchun University of Traditional Chinese Medicine (Approval No: CCZYFYLL2022 Approval Letter-020; CCZYFYLL2022 Approval Letter-021). All patients provided written or verbal informed consent prior to participation.
3 Results
3.1 General information of patients
This research collected data from 927 COVID-19 patients, categorizing them based on their nucleic acid test results into two groups: a stable CT value group with 660 cases and a fluctuating CT value group with 267 cases. Among the group with stable nucleic acid CT values, there were 388 males and 272 females, with a mean age of 40.27 ± 16.102 years. In the group with fluctuating nucleic acid CT values, there were 162 males and 105 females, with a median age of 41 [31, 53] years. There were no statistically significant differences between the two groups in terms of age, gender composition, vaccination history, medical history, and pre-admission treatment (p > 0.05). However, there was a statistically significant difference in the occupational composition between the two groups (χ2 = 7.043, p = 0.03) (Table 1).
3.2 Laboratory indicators and traditional Chinese medicine (TCM) syndrome differentiation
There were statistically significant differences between the group with stable nucleic acid CT values and the group with fluctuating nucleic acid CT values in terms of hospital stay duration (z = −12.672, p = 0.000), heart rate (z = −2.224, p = 0.026), creatinine (z = −2.545, p = 0.011), platelet count (z = −3.502, p = 0.000), glutamic-pyruvic transaminase (z = −2.006, p = 0.045), and glutamic oxaloacetic transaminase (z = −2.388, p = 0.017). However, there were no statistically significant differences between the two groups in terms of TCM symptoms at admission, fever after admission, comprehensive evaluation of medical records, TCM diagnosis at discharge, and various indicators (temperature, breath, systolic blood pressure, diastolic blood pressure, oxygen saturation, total bilirubin, direct bilirubin, red blood cell count, white blood cell count, bacterial count, hemoglobin, albumin, blood urea nitrogen, NLR) (p > 0.05) (Tables 2, 3).
3.3 Logistic regression analysis of factors influencing nucleic acid CT value fluctuations
Factors that showed statistically significant differences in the univariate analysis, including hospital stay duration, heart rate, creatinine, platelet count, glutamic-pyruvic transaminase and glutamic oxaloacetic transaminase, were included in a binary logistic regression model. Nucleic acid CT value fluctuation was the dependent variable, and the six aforementioned variables, along with age as a forced variable, were entered into the model using a forward: LR method. The results showed that hospital stay duration (p = 0.000, OR = 1.399), heart rate (p = 0.036, OR = 0.979), and platelet count (p = 0.019, OR = 0.996) were significantly associated with nucleic acid CT value fluctuations. None of the other indicators were independent risk factors for nucleic acid CT value fluctuations (p > 0.05) (Table 4).
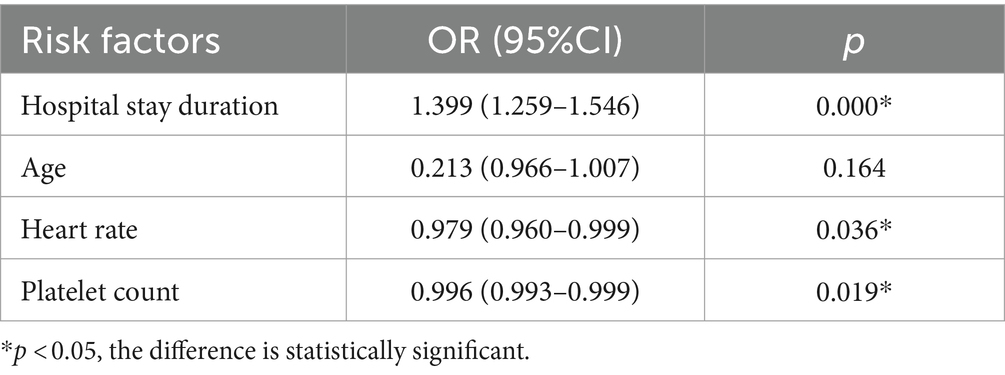
Table 4. Logistic regression analysis of the fluctuation factors of nucleic acid CT value of patients.
3.4 Comparison of baseline data between two groups
Since nucleic acid CT value fluctuations were positively correlated with hospital stay duration, the patients were further divided into Group A and Group B based on a median hospital stay duration of 10 days. In group A, there were 233 cases in the non-fluctuation group of nucleic acid CT value, including 139 males and 94 females, with a median age of 39 (30, 38) years, and 185 cases in the fluctuation group of nucleic acid CT value, including 115 males and 70 females, with a median age of 43 (31, 38) years. In group B, there were 427 cases in the non-fluctuation group of nucleic acid CT value, including 249 males and 178 females, with a median age of 39 (28, 38) years, and 82 cases in the fluctuation group of nucleic acid CT value, including 47 males and 35 females, with a median age of 34 (28, 39) years. There were no statistically significant differences between the two groups in terms of age, gender composition, vaccination history, medical history, and treatment before admission (p > 0.05). However, there was a statistically significant difference in the occupational composition between the two groups (χ2 = 6.012, p = 0.049) (Table 5).
3.5 Clinical data and laboratory examinations
In Group A, there was a statistically significant difference in red blood cell count (z = −2.800, p = 0.005). However, there were no statistically significant differences between the two groups in terms of TCM symptoms at admission, fever after admission, comprehensive evaluation of medical records, TCM diagnosis at discharge, and various indicators (temperature, breath, heart rate, systolic blood pressure, diastolic blood pressure, oxygen saturation, total bilirubin, direct bilirubin, creatinine, white blood cell count, bacterial count, hemoglobin, platelet count, albumin, glutamic-pyruvic transaminase, glutamic oxaloacetic transaminase, blood urea nitrogen, NLR) (p > 0.05). In Group B, there were statistically significant differences in direct bilirubin (z = −2.605, p = 0.009) and platelet count (z = −2.988, p = 0.003). However, there were no statistically significant differences between the two groups in terms of TCM symptoms at admission, fever after admission, comprehensive evaluation of medical records, TCM diagnosis at discharge, and various indicators (temperature, breath, heart rate, systolic blood pressure, diastolic blood pressure, oxygen saturation, total bilirubin, red blood cell count, creatinine, white blood cell count, bacterial count, hemoglobin, albumin, glutamic-pyruvic transaminase, glutamic oxaloacetic transaminase, blood urea nitrogen, NLR) (p > 0.05) (Tables 6, 7).
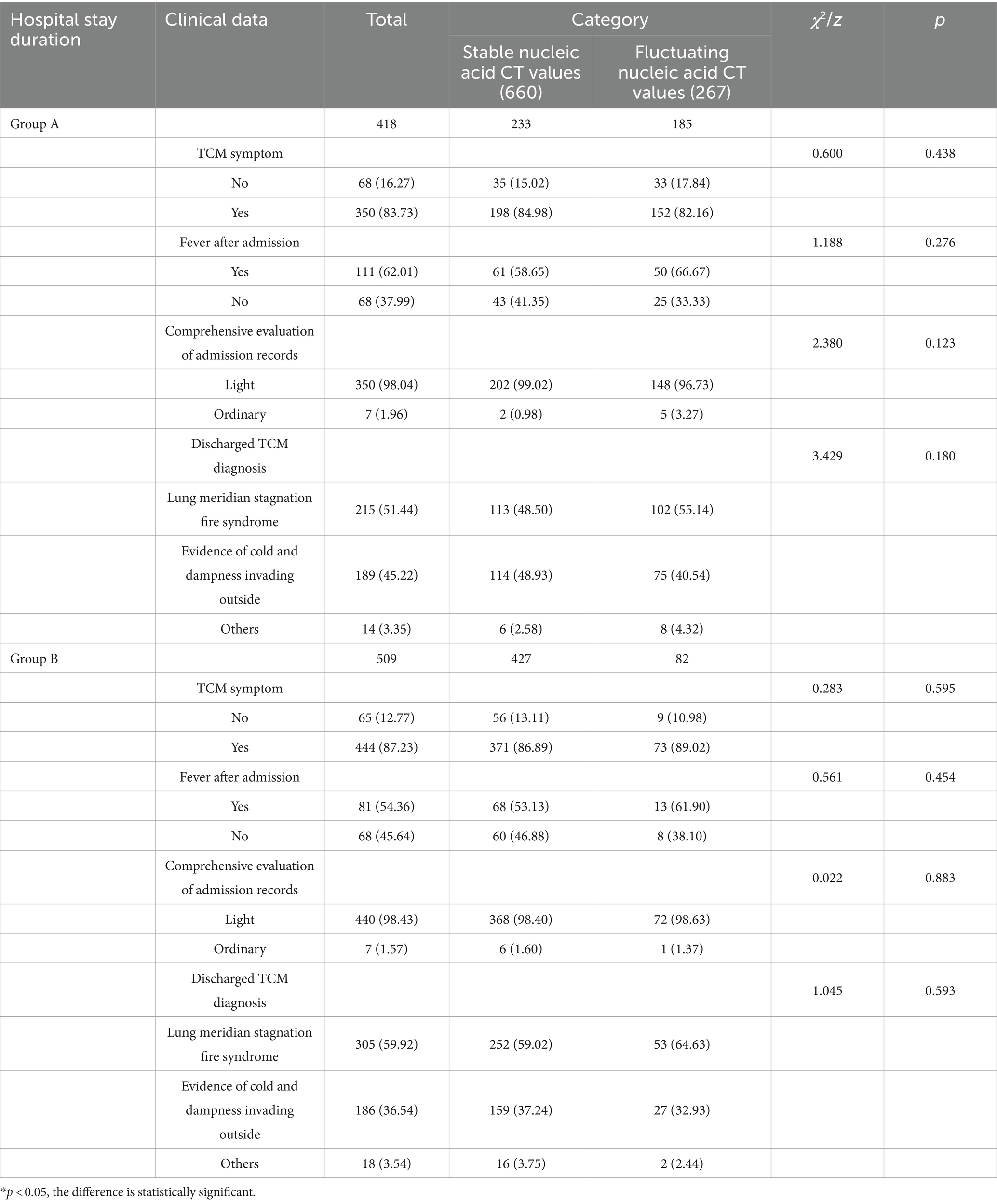
Table 6. Comparison of clinical data of patients with different length of stay after admission [P25, P75].
3.6 Logistic regression analysis of factors influencing nucleic acid CT value fluctuations in Group B
Factors that showed statistically significant differences in the univariate analysis of Group B, including occupational composition, direct bilirubin, and platelet count, were included in a binary logistic regression model. Nucleic acid CT value fluctuation was the dependent variable, and the three aforementioned variables, along with age as a forced variable, were entered into the model using a forward: LR method. The results showed that occupational composition (high-risk occupation, p = 0.005, OR = 3.159) and direct bilirubin (p = 0.029, OR = 0.997) were significantly associated with nucleic acid CT value fluctuations. None of the other indicators were independent risk factors for nucleic acid CT value fluctuations (p > 0.05) (Table 8).
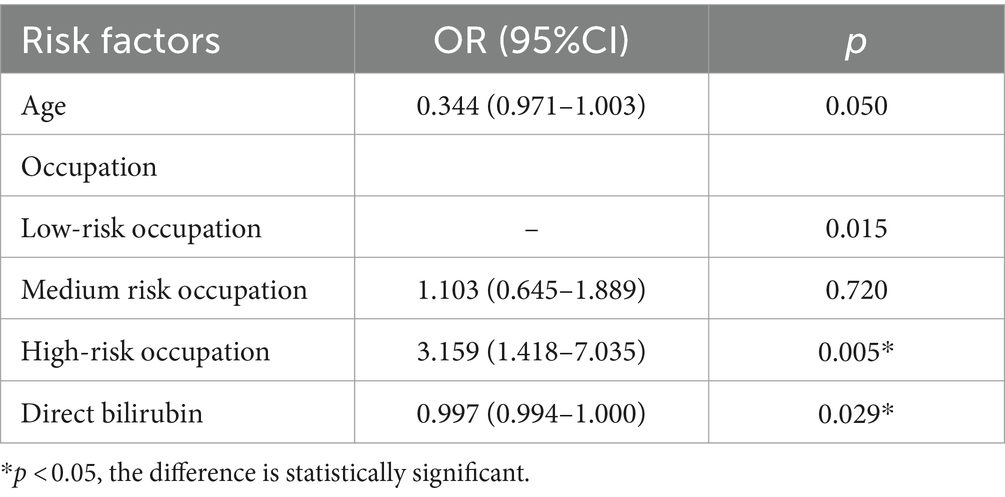
Table 8. Logistic regression analysis of fluctuations in nucleic acid CT values of patients in group B.
4 Discussion
Since the onset of the COVID-19 pandemic in 2019, more than 5 years have elapsed. During this period, the virus has evolved numerous variants, posing a significant public health challenge globally. Patients who test negative for nucleic acid may still be affected by COVID-19 (40). Among the variant strains, the Omicron variant has shown a decrease in pathogenicity but possesses strong infectivity and rapid transmission, causing significant impact in certain regions (41, 42). The fluctuation in nucleic acid CT values has undoubtedly posed challenges to the normalization of epidemic prevention and control, severely affecting people’s normal lives and mental well-being (43). Studies have found that approximately one-fourth of patients experience fluctuations in nucleic acid CT values (44), which may persist or recur over a prolonged period (45). Significant correlations have been observed between nucleic acid CT values and factors such as length of hospital stay, vaccination status (46), age (47), and albumin levels (48).
In this study, there was a statistically significant difference in the occupational composition between the group with nucleic acid CT value fluctuations and the group without fluctuations (χ2 = 7.043, p = 0.03). It was found that the viral shedding time of healthcare workers was prolonged (49), and the probability of nucleic acid CT value fluctuations increased with the nature of their occupation (39). The likelihood of contracting COVID-19 is inevitably associated with the workplace and occupation (50, 51). Univariate analysis of nucleic acid CT value fluctuations revealed a significant correlation with hospitalization duration (z = −12.672, p = 0.000), consistent with previous research that showed a correlation between nucleic acid CT value fluctuations and hospitalization duration (p = 0.00018, 13). Therefore, nucleic acid CT fluctuations are an important factor related to length of stay (38, 52). This suggests a significant correlation between nucleic acid CT value fluctuations and hospitalization duration, and binary logistic regression analysis results indicate a positive correlation between nucleic acid CT value fluctuations and hospital stay duration, indicating that the longer the hospitalization duration, the greater the risk of nucleic acid CT value fluctuations. Some studies have indicated that older adult patients, due to their weaker immune system and higher prevalence of underlying diseases such as hypertension and diabetes, have a lower clearance rate and tolerance to the virus, making them more prone to nucleic acid CT value fluctuations (53, 54). However, the results of this study showed no significant correlation between nucleic acid CT value fluctuations and age or medical history, which is inconsistent with other related studies (39, 48, 55). Given the weakened immune response and decreased organ reserve capacity in the older adult, leukocyte count and C-reactive protein levels are associated with nucleic acid CT values (56). Therefore, it is crucial to pay attention to this population to prevent the occurrence of nucleic acid CT value fluctuations, which can cause irreversible damage to the body, aligning with the research on nucleic acid CT value fluctuations in the Northeast region (57). This suggests that the influence of gender composition on nucleic acid CT value fluctuations may be region-specific, while age may be related to the fact that the subjects in this study were predominantly young and middle-aged individuals.
Although this study found no statistically significant difference between the fluctuation of patient nucleic acid CT values and vaccination history, COVID-19 vaccines have shown high efficacy and good tolerability against the original strain and related variants. Both two-dose and three-dose regimens provide good protection against Omicron, although efficacy may decrease over time and compared to other strains (58–60). This research does not establish a significant correlation between the fluctuations in the nucleic acid CT values and vaccination. However, it still emphasizes the effectiveness of COVID-19 vaccination in preventing and controlling the Omicron variant, and encourages people to get vaccinated to prevent reinfection. Nucleic acid CT values have certain relationships with factors such as NLR, white blood cell count, C-reactive protein, and platelet count (56, 61–63). This study shows significant correlations between nucleic acid CT fluctuation and patient creatinine, platelet count, alanine aminotransferase, and aspartate aminotransferase, but no significant correlation with white blood cell count, NLR (neutrophil count/lymphocyte count), or hemoglobin. This suggests that the strength of a patient’s immune system and the normal function of the liver are related to nucleic acid CT fluctuation. Binary logistic regression analysis reveals that platelet count (p = 0.019, OR = 0.996) is an independent factor influencing nucleic acid CT value fluctuation and is negatively correlated, which is consistent with previous studies. This indicates that the stronger the patient’s immune system, the lower the risk of the fluctuation of nucleic acid CT values fluctuation. Studies have found a high risk of arrhythmias in COVID-19 patients (64). The reasons for arrhythmias in COVID-19 are still unclear and may be a direct effect of the virus or adverse reactions to medications used for treatment. This is consistent with the statistically significant difference in nucleic acid CT fluctuation and heart rate (z = −2.224, p = 0.026) observed in this study. Binary logistic regression analysis shows that heart rate (p = 0.036, OR = 0.979) is an independent factor influencing nucleic acid CT value fluctuation and is negatively correlated. This suggests that paying attention to cardiovascular health and receiving anti-arrhythmia treatment has a significant effect on preventing nucleic acid CT fluctuation. Further research has found that regular aerobic exercise and its effect on heart rate are consistent with the median heart rate results in both groups (65). This indicates that regular aerobic exercise, which increases myocardial oxygen consumption and enhances immune function, is an effective approach to control nucleic acid CT fluctuation.
The study found that red blood cell count is an influencing factor for nucleic acid CT fluctuation in Group A, which is consistent with the well-established manifestation of anemia in COVID-19 infection (66–68). Although anemia does not directly affect the mortality rate of COVID-19 patients, its impact on the older adult should not be overlooked. Due to their diminished immunity and general frailty, older adult individuals are less likely to recover quickly from COVID-19, leading to prolonged hospital stays and severely diminishing their quality of life. In Group B, occupational composition, direct bilirubin, and platelet count are influencing factors for nucleic acid CT fluctuation, and binary logistic regression analysis shows that occupational composition and direct bilirubin are independent factors influencing nucleic acid CT value fluctuation and are positively and negatively correlated, respectively. This indicates that short-term hospitalized patients in high-risk occupations are more prone to nucleic acid CT fluctuation, which is consistent with the factors influencing nucleic acid CT fluctuation mentioned earlier and further emphasizes the higher risk of nucleic acid CT fluctuation in short-term hospitalized patients engaged in high-risk occupations. For short-term hospitalized patients, the lower the direct bilirubin within the normal range, the higher the risk of nucleic acid CT fluctuation, which is consistent with research showing that direct bilirubin prolongs viral shedding time in Omicron patients (69).
5 Conclusion
Based on the retrospective study, it was found that the occupation composition, hospital stay duration, heart rate, creatinine, platelet count, alanine transaminase, and aspartate transaminase were factors influencing the fluctuation of nucleic acid CT values in COVID-19 patients. Among them, hospital stay duration, platelet count, and heart rate were identified as independent factors affecting the fluctuation of nucleic acid CT values, with a positive correlation observed with the hospital stay duration and a negative correlation with platelet count and heart rate. Analyzing the relationship between hospital stay duration and the fluctuation of nucleic acid CT values, it was determined that red blood cell count was a factor influencing the fluctuation of nucleic acid CT values fluctuation in Group A patients, while occupation composition, direct bilirubin, and platelet count were factors influencing the fluctuation of nucleic acid CT values fluctuation in Group B patients. In Group B, occupation composition and direct bilirubin were identified as independent factors affecting the fluctuation of nucleic acid CT values, positively correlated with occupational risk and negatively correlated with direct bilirubin.
Therefore, enhancing patients’ immune function, increasing physical exercise to improve myocardial oxygen consumption, reducing hospital stay duration, and closely monitoring liver function to prevent liver function abnormalities at the initial stage of hospitalization are effective measures for controlling the fluctuation of nucleic acid CT values in COVID-19 patients. However, due to the limited clinical data collected, these findings may not comprehensively and accurately reflect the factors influencing the fluctuation of nucleic acid CT values in COVID-19. Additionally, the regional limitations of the data collection may not represent all regions. Therefore, future research should adopt large sample sizes and a multicenter approach to provide reliable evidence for comprehensive and accurate prediction of nucleic acid CT value fluctuation in COVID-19, thus supporting data-driven strategies for epidemic prevention and control in the post-pandemic era.
Data availability statement
The raw data supporting the conclusions of this article will be made available by the authors, without undue reservation.
Ethics statement
The studies involving humans were approved by the Ethics Committee of the Affiliated Hospital of Changchun University of Traditional Chinese Medicine (Approval No: CCZYFYLL2022 Approval Letter-020; CCZYFYLL2022 Approval Letter-021). The studies were conducted in accordance with the local legislation and institutional requirements. Written informed consent for participation was not required from the participants or the participants’ legal guardians/next of kin in accordance with the national legislation and institutional requirements.
Author contributions
ZC: Writing – original draft. FS: Data curation, Writing – original draft. HD: Data curation, Writing – original draft. ZT: Data curation, Writing – original draft. YC: Conceptualization, Data curation, Writing – review & editing. WY: Data curation, Writing – review & editing. SH: Writing – review & editing, Conceptualization, Funding acquisition. LS: Conceptualization, Writing – review & editing, Funding acquisition.
Funding
The author(s) declare financial support was received for the research, authorship, and/or publication of this article. This study was funded by the following projects: A randomized, open, positive control drug, fibrate test clinical study of Jiejixuanfeichuyi granules against COVID-19 (Omicron variant) infection (2022ZYLCYJ04-1); A cross-sectional study of post-coronavirus disease 2019 syndrome in Jilin province and a clinical study of TCM efficacy evaluation (20230203189SF); A retrospective study of the prevention and treatment of COVID-19 in Changchun University of Traditional Chinese Medicine (2022219). We gratefully acknowledge the funding of the above projects. The funders had no role in study design, data collection and analysis, decision to publish, or preparation of the manuscript.
Conflict of interest
The authors declare that the research was conducted in the absence of any commercial or financial relationships that could be construed as a potential conflict of interest.
Publisher’s note
All claims expressed in this article are solely those of the authors and do not necessarily represent those of their affiliated organizations, or those of the publisher, the editors and the reviewers. Any product that may be evaluated in this article, or claim that may be made by its manufacturer, is not guaranteed or endorsed by the publisher.
Supplementary material
The Supplementary material for this article can be found online at: https://www.frontiersin.org/articles/10.3389/fpubh.2024.1377135/full#supplementary-material
References
1. Chilamakuri, R, and Agarwal, S. COVID-19: characteristics and therapeutics. Cells. (2021) 10:206. doi: 10.3390/cells10020206
2. Muhammad Waqar, M, Javaria, M, Saira, S, Masooma Batool, S, and Hira, A. Severe acute respiratory syndrome (Saras Covid-2): a comprehensive review. Quantum J Med Health Sci. (2021) 1:25–33.
3. Fan, Y, Li, X, Zhang, L, Wan, S, Zhang, L, and Zhou, F. SARS-CoV-2 Omicron variant: recent progress and future perspectives. Signal Transduct Target Ther. (2022) 7:141. doi: 10.1038/s41392-022-00997-x
4. Powell, AA, Kirsebom, F, Stowe, J, Ramsay, ME, Lopez-Bernal, J, Andrews, N, et al. Protection against symptomatic infection with delta (B.1.617.2) and Omicron (B.1.1.529) BA.1 and BA.2 SARS-CoV-2 variants after previous infection and vaccination in adolescents in England, August, 2021-March, 2022: a national, observational, test-negative, case-control study. Lancet Infect Dis. (2023) 23:435–44. doi: 10.1016/S1473-3099(22)00729-0
5. Suzuki, R, Yamasoba, D, Kimura, I, Wang, L, Kishimoto, M, Ito, J, et al. Attenuated fusogenicity and pathogenicity of SARS-CoV-2 Omicron variant. Nature. (2022) 603:700–5. doi: 10.1038/s41586-022-04462-1
6. Shuai, H, Chan, JF, Hu, B, Chai, Y, Yuen, TT, Yin, F, et al. Attenuated replication and pathogenicity of SARS-CoV-2 B.1.1.529 Omicron. Nature. (2022) 603:693–9. doi: 10.1038/s41586-022-04442-5
7. Halfmann, PJ, Iida, S, Iwatsuki-Horimoto, K, Maemura, T, Kiso, M, Scheaffer, SM, et al. SARS-CoV-2 Omicron virus causes attenuated disease in mice and hamsters. Nature. (2022) 603:687–92. doi: 10.1038/s41586-022-04441-6
8. Chekol Abebe, E, Tiruneh, GMM, Behaile, TMA, Asmamaw Dejenie, T, Mengie Ayele, T, Tadele Admasu, F, et al. Mutational pattern, impacts and potential preventive strategies of Omicron SARS-CoV-2 variant infection. Infect Drug Resist. (2022) 15:1871–87. doi: 10.2147/IDR.S360103
9. Buitrago-Garcia, D, Ipekci, AM, Heron, L, Imeri, H, Araujo-Chaveron, L, Arevalo-Rodriguez, I, et al. Occurrence and transmission potential of asymptomatic and presymptomatic SARS-CoV-2 infections: update of a living systematic review and meta-analysis. PLoS Med. (2022) 19:e1003987. doi: 10.1371/journal.pmed.1003987
10. Hansen, CH, Friis, NU, Bager, P, Stegger, M, Fonager, J, Fomsgaard, A, et al. Risk of reinfection, vaccine protection, and severity of infection with the BA.5 Omicron subvariant: a nation-wide population-based study in Denmark. Lancet Infect Dis. (2023) 23:167–76. doi: 10.1016/S1473-3099(22)00595-3
11. Zhu, KL, Jiang, XL, Zhan, BD, Wang, XJ, Xia, X, Cao, GP, et al. Durability of neutralization against Omicron subvariants after vaccination and breakthrough infection. Cell Rep. (2023) 42:112075. doi: 10.1016/j.celrep.2023.112075
12. Singanayagam, A, Patel, M, Charlett, A, Lopez Bernal, J, Saliba, V, Ellis, J, et al. Duration of infectiousness and correlation with RT-PCR cycle threshold values in cases of COVID-19, England, January to may 2020. Euro Surveill. (2020) 25:2001483. doi: 10.2807/1560-7917.ES.2020.25.32.2001483
13. Lu, J, Peng, J, Xiong, Q, Liu, Z, Lin, H, Tan, X, et al. Clinical, immunological and virological characterization of COVID-19 patients that test re-positive for SARS-CoV-2 by RT-PCR. EBioMedicine. (2020) 59:102960. doi: 10.1016/j.ebiom.2020.102960
14. Takahashi, K, Ishikane, M, Ujiie, M, Iwamoto, N, Okumura, N, Sato, T, et al. Duration of infectious virus shedding by SARS-CoV-2 Omicron variant-infected vaccinees. Emerg Infect Dis. (2022) 28:998–1001. doi: 10.3201/eid2805.220197
15. Qureshi, A, Syed Sulaiman, SA, Kumar, N, and Qureshi, P. Knowledge, perception, and fear among the global population towards newly evoked variant Omicron (B.1.1.529). PLoS One. (2022) 17:e0270761. doi: 10.1371/journal.pone.0270761
16. Taquet, M, Sillett, R, Zhu, L, Mendel, J, Camplisson, I, Dercon, Q, et al. Neurological and psychiatric risk trajectories after SARS-CoV-2 infection: an analysis of 2-year retrospective cohort studies including 1 284 437 patients. Lancet Psychiatry. (2022) 9:815–27. doi: 10.1016/S2215-0366(22)00260-7
17. Rogers, JP, and Lewis, G. Neuropsychiatric sequelae of COVID-19: long-lasting, but not uniform. Lancet Psychiatry. (2022) 9:762–3. doi: 10.1016/S2215-0366(22)00302-9
18. Wang, H, Sun, K, and Wang, Y. Exploring the Chinese Public's perception of Omicron variants on social media: LDA-based topic modeling and sentiment analysis. Int J Environ Res Public Health. (2022) 19:8377. doi: 10.3390/ijerph19148377
19. Rao, SN, Manissero, D, Steele, VR, and Pareja, J. A systematic review of the clinical utility of cycle threshold values in the context of COVID-19. Infect Dis Ther. (2020) 9:573–86. doi: 10.1007/s40121-020-00324-3
20. Rajyalakshmi, B, Samavedam, S, Reddy, PR, and Aluru, N. Prognostic value of "cycle threshold" in confirmed COVID-19 patients. Indian J Crit Care Med. (2021) 25:322–6. doi: 10.5005/jp-journals-10071-23765
21. Sikhosana, ML, Welch, R, Musekiwa, A, Makatini, Z, Ebonwu, J, Blumberg, L, et al. Association between SARS-CoV-2 gene specific Ct values and COVID-19 associated in-hospital mortality. Front Epidemiol. (2024) 4:1375975. doi: 10.3389/fepid.2024.1375975
22. Shoaib, N, Noureen, N, Faisal, A, Zaheer, M, Imran, M, Ahsan, A, et al. Factors associated with cycle threshold values (Ct-values) of SARS-CoV2-rRT-PCR. Mol Biol Rep. (2022) 49:4101–6. doi: 10.1007/s11033-022-07360-x
23. Penney, J, Jung, A, Koethe, B, and Doron, S. Cycle threshold values and SARS-CoV-2: relationship to demographic characteristics and disease severity. J Med Virol. (2022) 94:3978–81. doi: 10.1002/jmv.27752
24. Acer, O, Genc Bahce, Y, and Ozudogru, O. Association of viral load with age, gender, disease severity, and death in severe acute respiratory syndrome coronavirus 2 variants. J Med Virol. (2022) 94:3063–9. doi: 10.1002/jmv.27677
25. Ur Rehman, M, Sajjad Naqvi, S, Ullah, R, Arshad, N, Ammad, M, Ul Ain, Q, et al. Elucidation of correlation between SARS-CoV-2 RdRp and N gene cycle threshold (Ct) by RT-PCR with age and gender. Clin Chim Acta. (2022) 533:42–7. doi: 10.1016/j.cca.2022.06.008
26. Trunfio, M, Richiardi, L, Alladio, F, Staffilano, E, Longo, B, Venuti, F, et al. Determinants of SARS-CoV-2 contagiousness in household contacts of symptomatic adult index cases. Front Microbiol. (2022) 13:829393. doi: 10.3389/fmicb.2022.829393
27. Karahasan Yagci, A, Sarinoglu, RC, Bilgin, H, Yanilmaz, O, Sayin, E, Deniz, G, et al. Relationship of the cycle threshold values of SARS-CoV-2 polymerase chain reaction and total severity score of computerized tomography in patients with COVID 19. Int J Infect Dis. (2020) 101:160–6. doi: 10.1016/j.ijid.2020.09.1449
28. El Zein, S, Chehab, O, Kanj, A, Akrawe, S, Alkassis, S, Mishra, T, et al. SARS-CoV-2 infection: initial viral load (iVL) predicts severity of illness/outcome, and declining trend of iVL in hospitalized patients corresponds with slowing of the pandemic. PLoS One. (2021) 16:e0255981. doi: 10.1371/journal.pone.0255981
29. Kawasuji, H, Morinaga, Y, Tani, H, Yoshida, Y, Takegoshi, Y, Kaneda, M, et al. SARS-CoV-2 RNAemia with a higher nasopharyngeal viral load is strongly associated with disease severity and mortality in patients with COVID-19. J Med Virol. (2022) 94:147–53. doi: 10.1002/jmv.27282
30. Maltezou, HC, Raftopoulos, V, Vorou, R, Papadima, K, Mellou, K, Spanakis, N, et al. Association between upper respiratory tract viral load, comorbidities, disease severity, and outcome of patients with SARS-CoV-2 infection. J Infect Dis. (2021) 223:1132–8. doi: 10.1093/infdis/jiaa804
31. Tom, MR, and Mina, MJ. To interpret the SARS-CoV-2 test, consider the cycle threshold value. Clin Infect Dis. (2020) 71:2252–4. doi: 10.1093/cid/ciaa619
32. Salvatore, PP, Dawson, P, Wadhwa, A, Rabold, EM, Buono, S, Dietrich, EA, et al. Epidemiological correlates of polymerase chain reaction cycle threshold values in the detection of severe acute respiratory syndrome coronavirus 2 (SARS-CoV-2). Clin Infect Dis. (2021) 72:e761–7. doi: 10.1093/cid/ciaa1469
33. Zhuang, X, Zheng, Y, Wei, S, Zhai, W, Song, Q, Chen, M, et al. Can the nucleic acid Ct value of discharged patients infected with SARS-CoV-2 Omicron variant be 35?--a retrospective study on fluctuation of nucleic acid Ct values in SNIEC mobile cabin hospital. Front Cell Infect Microbiol. (2022) 12:1059880. doi: 10.3389/fcimb.2022.1059880
34. Wang, X, Fan, Q, Li, Y, Xiao, J, Huang, Y, Guo, T, et al. The changes in psychological symptoms of COVID-19 patients after "re-positive". Front Psych. (2022) 13:1010004. doi: 10.3389/fpsyt.2022.1010004
35. Wang, J, Zhang, SX, Na, JR, Zhang, LL, Zhang, YH, Chu, JJ, et al. A comparative study of virus nucleic acid re-positive and non-re-positive patients infected with SARS-CoV-2 Delta variant strain in the Ningxia Hui Autonomous Region. Front Public Health. (2022) 10:1023797. doi: 10.3389/fpubh.2022.1023797
36. Bustos, P, Tambley, C, Acevedo, A, Andrade, W, Leal, G, Vidal, D, et al. Quantitative detection of SARS-CoV-2 RNA in nasopharyngeal samples from infected patients with mild disease. J Med Virol. (2021) 93:2439–45. doi: 10.1002/jmv.26761
37. National Health Commission of the People’s Republic of China (2022). Guideline of diagnosis and treatment of novel coronavirus pneumonia (the 9th version) [EB/OL].
38. Hu, C, Liu, YK, Sun, QD, Du, Z, Fang, YQ, Guo, F, et al. Clinical characteristics and risk factors for a prolonged length of stay of patients with asymptomatic and mild COVID-19 during the wave of Omicron from Shanghai, China. BMC Infect Dis. (2022) 22:947. doi: 10.1186/s12879-022-07935-w
39. Li, R, Jin, C, Zhang, L, Kong, D, Hu, K, Xuan, M, et al. Clinical characteristics and risk factors analysis of viral shedding time in mildly symptomatic and asymptomatic patients with SARS-CoV-2 Omicron variant infection in Shanghai. Front Public Health. (2022) 10:1073387. doi: 10.3389/fpubh.2022.1073387
40. Mazhar, MW, Raza, A, Shaheen, T, Zubair, M, Mahmood, J, Tahir, H, et al. Detection of SARS-CoV-2 by using real-time PCR nasopharyngeal swabs in suspected patients and their clinical medication. Sens Int. (2022) 3:100148. doi: 10.1016/j.sintl.2021.100148
41. Team, CC-R. SARS-CoV-2 B.1.1.529 (Omicron) variant – United States, December 1-8, 2021. MMWR Morb Mortal Wkly Rep. (2021) 70:1731–4. doi: 10.15585/mmwr.mm7050e1
42. Hirabara, SM, Serdan, TDA, Gorjao, R, Masi, LN, Pithon-Curi, TC, Covas, DT, et al. SARS-COV-2 variants: differences and potential of immune evasion. Front Cell Infect Microbiol. (2021) 11:781429. doi: 10.3389/fcimb.2021.781429
43. Nainu, F, Abidin, RS, Bahar, MA, Frediansyah, A, Emran, TB, Rabaan, AA, et al. SARS-CoV-2 reinfection and implications for vaccine development. Hum Vaccin Immunother. (2020) 16:3061–73. doi: 10.1080/21645515.2020.1830683
44. Yang, Y, You, Y, Liu, Y, Geng, L, Huang, L, Zhou, H, et al. Factors associated with negative conversion of viral RNA in hospitalized children infected with SARS-CoV-2 Omicron variant in Shanghai, China: a retrospective analysis. BMC Infect Dis. (2023) 23:264. doi: 10.1186/s12879-023-08223-x
45. Li, Y, Guo, D, Zhang, B, Wu, W, Cai, Y, and Zhang, X. Tracking analysis of viral nucleic acid Ct value in patients with re-positive SARS-CoV-2 infection. Zhonghua Wei Zhong Bing Ji Jiu Yi Xue. (2023) 35:427–30. doi: 10.3760/cma.j.cn121430-20230107-01130
46. Zhang, W, Zhou, S, Wang, G, Cao, M, Sun, D, Lu, W, et al. Clinical predictors and RT-PCR profile of prolonged viral shedding in patients with SARS-CoV-2 Omicron variant in Shanghai: a retrospective observational study. Front Public Health. (2022) 10:1015811. doi: 10.3389/fpubh.2022.1015811
47. Hu, X, Xing, Y, Jia, J, Ni, W, Liang, J, Zhao, D, et al. Factors associated with negative conversion of viral RNA in patients hospitalized with COVID-19. Sci Total Environ. (2020) 728:138812. doi: 10.1016/j.scitotenv.2020.138812
48. Cogliati Dezza, F, Oliva, A, Cancelli, F, Savelloni, G, Valeri, S, Mauro, V, et al. Determinants of prolonged viral RNA shedding in hospitalized patients with SARS-CoV-2 infection. Diagn Microbiol Infect Dis. (2021) 100:115347. doi: 10.1016/j.diagmicrobio.2021.115347
49. Keske, S, Guney-Esken, G, Vatansever, C, Besli, Y, Kuloglu, ZE, Nergiz, Z, et al. Duration of infectious shedding of SARS-CoV-2 Omicron variant and its relation with symptoms. Clin Microbiol Infect. (2023) 29:221–4. doi: 10.1016/j.cmi.2022.07.009
50. Gomez-Ochoa, SA, Franco, OH, Rojas, LZ, Raguindin, PF, Roa-Diaz, ZM, Wyssmann, BM, et al. COVID-19 in health-care workers: a living systematic review and Meta-analysis of prevalence, risk factors, clinical characteristics, and outcomes. Am J Epidemiol. (2021) 190:161–75. doi: 10.1093/aje/kwaa191
51. Gholami, M, Fawad, I, Shadan, S, Rowaiee, R, Ghanem, H, Hassan Khamis, A, et al. COVID-19 and healthcare workers: a systematic review and meta-analysis. Int J Infect Dis. (2021) 104:335–46. doi: 10.1016/j.ijid.2021.01.013
52. Yin, C, Hu, B, Li, K, Liu, X, Wang, S, He, R, et al. Clinical characteristics and prognostic nomograms of 12555 non-severe COVID-19 cases with Omicron infection in Shanghai. BMC Infect Dis. (2023) 23:606. doi: 10.1186/s12879-023-08582-5
53. Ren, X, Ren, X, Lou, J, Wang, Y, Huang, Q, Shi, Y, et al. A systematic review and meta-analysis of discharged COVID-19 patients retesting positive for RT-PCR. EClinicalMedicine. (2021) 34:100839. doi: 10.1016/j.eclinm.2021.100839
54. Kang, W, Yang, P, Dang, B, Zhang, W, Gang, Y, Wang, W, et al. Dynamics of disease characteristics and viral RNA decay in patients with asymptomatic and mild infections during the Omicron wave in Shanghai, China: a retrospective cohort study. Int J Infect Dis. (2023) 130:60–70. doi: 10.1016/j.ijid.2023.02.020
55. Perlis, RH, Santillana, M, Ognyanova, K, Safarpour, A, Lunz Trujillo, K, Simonson, MD, et al. Prevalence and correlates of long COVID symptoms among US adults. JAMA Netw Open. (2022) 5:e2238804. doi: 10.1001/jamanetworkopen.2022.38804
56. Wang, M, Mi, H, Li, N, Shi, Q, Sun, W, He, T, et al. Association between the overall burden of comorbidity and Ct values among the older patients with Omicron infection: mediated by inflammation. Front Immunol. (2023) 14:1145044. doi: 10.3389/fimmu.2023.1145044
57. Li, H, Zhu, M, Zhang, P, Yan, X, Niu, J, Wang, Z, et al. Milder symptoms and shorter course in patients with re-positive COVID-19: a cohort of 180 patients from Northeast China. Front Microbiol. (2022) 13:989879. doi: 10.3389/fmicb.2022.989879
58. Fiolet, T, Kherabi, Y, MacDonald, CJ, Ghosn, J, and Peiffer-Smadja, N. Comparing COVID-19 vaccines for their characteristics, efficacy and effectiveness against SARS-CoV-2 and variants of concern: a narrative review. Clin Microbiol Infect. (2022) 28:202–21. doi: 10.1016/j.cmi.2021.10.005
59. Lin, DY, Xu, Y, Gu, Y, Zeng, D, Wheeler, B, Young, H, et al. Effectiveness of bivalent boosters against severe Omicron infection. N Engl J Med. (2023) 388:764–6. doi: 10.1056/NEJMc2215471
60. Accorsi, EK, Britton, A, Fleming-Dutra, KE, Smith, ZR, Shang, N, Derado, G, et al. Association between 3 doses of mRNA COVID-19 vaccine and symptomatic infection caused by the SARS-CoV-2 Omicron and Delta variants. JAMA. (2022) 327:639–51. doi: 10.1001/jama.2022.0470
61. Salman, E, Celikbilek, N, Aydogan, S, Ozdem, B, Gokay, S, Kirca, F, et al. Investigation of the relationship of systemic immune-inflammation index, C-reactive protein and Interleukin-6 with viral dynamics in patients with COVID-19. Mikrobiyol Bul. (2021) 55:539–52. doi: 10.5578/mb.20219706
62. Stegeman, I, Ochodo, EA, Guleid, F, Holtman, GA, Yang, B, Davenport, C, et al. Routine laboratory testing to determine if a patient has COVID-19. Cochrane Database Syst Rev. (2020) 11:CD013787. doi: 10.1002/14651858.CD013787
63. Zhang, G, Zhang, J, Wang, B, Zhu, X, Wang, Q, and Qiu, S. Analysis of clinical characteristics and laboratory findings of 95 cases of 2019 novel coronavirus pneumonia in Wuhan, China: a retrospective analysis. Respir Res. (2020) 21:74. doi: 10.1186/s12931-020-01338-8
64. Manolis, AS, Manolis, AA, Manolis, TA, Apostolopoulos, EJ, Papatheou, D, and Melita, H. COVID-19 infection and cardiac arrhythmias. Trends Cardiovasc Med. (2020) 30:451–60. doi: 10.1016/j.tcm.2020.08.002
65. Alawna, M, Amro, M, and Mohamed, AA. Aerobic exercises recommendations and specifications for patients with COVID-19: a systematic review. Eur Rev Med Pharmacol Sci. (2020) 24:13049–55. doi: 10.26355/eurrev_202012_24211
66. Bergamaschi, G, Borrelli de Andreis, F, Aronico, N, Lenti, MV, Barteselli, C, Merli, S, et al. Anemia in patients with COVID-19: pathogenesis and clinical significance. Clin Exp Med. (2021) 21:239–46. doi: 10.1007/s10238-020-00679-4
67. Taneri, PE, Gómez-Ochoa, SA, Llanaj, E, Raguindin, PF, Rojas, LZ, Roa-Díaz, ZM, et al. Anemia and iron metabolism in COVID-19: a systematic review and meta-analysis. Eur J Epidemiol. (2020) 35:763–73. doi: 10.1007/s10654-020-00678-5
68. Yuan, X, Huang, W, Ye, B, Chen, C, Huang, R, Wu, F, et al. Changes of hematological and immunological parameters in COVID-19 patients. Int J Hematol. (2020) 112:553–9. doi: 10.1007/s12185-020-02930-w
Keywords: COVID-19, Omicron variant, fluctuation of nucleic acid CT values, retrospective analysis, risk factors
Citation: Cao Z, Sun F, Ding H, Tian Z, Cui Y, Yang W, Hu S and Shi L (2024) A retrospective analysis of the influencing factors of nucleic acid CT value fluctuation in COVID-19 patients infected with Omicron variant virus in Changchun city. Front. Public Health. 12:1377135. doi: 10.3389/fpubh.2024.1377135
Edited by:
Jessica L. Jones, United States Food and Drug Administration, United StatesReviewed by:
Muhammad Waqar Mazhar, Government College University, Faisalabad, PakistanAlfi Sophian, The National Agency of Drug and Food Control, Indonesia
Copyright © 2024 Cao, Sun, Ding, Tian, Cui, Yang, Hu and Shi. This is an open-access article distributed under the terms of the Creative Commons Attribution License (CC BY). The use, distribution or reproduction in other forums is permitted, provided the original author(s) and the copyright owner(s) are credited and that the original publication in this journal is cited, in accordance with accepted academic practice. No use, distribution or reproduction is permitted which does not comply with these terms.
*Correspondence: Shaodan Hu, 734168300@qq.com; Li Shi, shili0648@163.com