- Department of the Rehabilitation Medicine, The First Affiliated Hospital of Zhejiang Chinese Medical University, Hangzhou, China
Background: Inflammation and liver function are associated with cognitive decline and dementia. Little is known about the serum albumin-to-globulin ratio on cognitive function.
Objective: The objective of this study was to investigate the association between albumin-to-globulin ratio and cognitive function among the American older people.
Methods: The public data available on the US National Health and Nutrition Examination Survey (NHANES) from 2011 to 2014 was used for this cross-sectional study. Participants aged ≥60 years completed the cognitive function assessments, including word learning and recall modules from the Consortium to Establish a Registry for Alzheimer’s Disease (CERAD), the animal fluency (AF) test, and the digit symbol substitution test (DSST). A composite cognition score was calculated to evaluate global cognition. The univariate and multivariate linear regression analysis, curve fitting, a threshold effect, along with a subgroup analysis and interaction tests were conducted.
Results: Serum albumin-to-globulin ratio (per 0.1 unit) was positively associated DSST score (β = 0.36, 95% CI: 0.21, 0.51), AF score (β = 0.1, 95% CI: 0.04, 0.16) and global cognition score (β = 0.05, 95% CI: 0.02, 0.07), after being fully adjusted, while albumin-to-globulin ratio was not related to CERAD score (β = 0.05, 95% CI: −0.02, 0.12). A non-linear was observed in the dose–response relationship between albumin-to-globulin ratio and global cognition (P for non-linearity < 0.001). The subgroup analysis was overall stable, yet the interaction test was significant for age on global cognition (P for interaction = 0.036).
Conclusion: The findings of this cross-sectional study suggested a positive and non-linear association between albumin-to-globulin ratio and cognitive function in the American older people. Maintaining albumin-to-globulin ratio with an appropriate range may be one of the therapeutic strategies to limit the progression of cognitive decline for the older people.
Introduction
With the development of a hyper-aging society, the number of the older people with age-related cognitive decline increases, and the incidence of Alzheimer’s disease (AD) and Alzheimer’s disease-related dementia (ADRD) increases further, which almost doubles every 5 years after the age of 65 (1). The global prevalence of mild cognitive impairment (MCI) among individuals aged ≥65 is approximately 10–20% (2). MCI is characterized by a decline in cognition without significant impact on daily independent living, which represents a pre-stage on the continuum of cognitive decline between “normal aging” and dementia (3). Conservatively estimated, around 5–7% of individuals with MCI progress to dementia each year (4). While dementia progression is commonly viewed as irreversible, community-based studies suggested a reversion rate of approximately 25% for MCI (5). Dementia imposes a substantial burden on individuals, families, healthcare systems, and society at large. However, the therapeutic strategies for dementia are limited. Therefore, the proactive identification of pre-stage dementia and its associated factors, followed by primary interventions, is crucial in practice.
Research indicates that genetics, aging, nutrition, microbial exposure, infections, chronic inflammatory states, vascular risk, depression, and other factors are all associated with the etiology, development, and progression of cognitive impairment and dementia (6, 7). Current studies on cognitive impairment and dementia are focused on immunity and inflammation. Cells of the central nervous system are sensitive to peripheral inflammations and immune infiltration (8). The adaptive immunity decreases with aging, making older individuals more susceptible to infections and resulting in a chronic, low-degree inflammation, which is believed to be associated with cognition decline and dementia (9). Serum albumin is the most abundant protein in peripheral blood plasma and is associated with systemic inflammation. An elevated inflammatory index is an independent risk factor for hypoalbuminemia (10). The albumin could act as a chaperone to bind 90–95% of the β-amyloid protein (Aβ) in blood plasma, reducing the Aβ deposition in the brain (11). Shojai et al. reported an association between hypoalbuminemia and cognitive decline in the older people, as well as an adverse prognosis for other neurodegenerative diseases (12). The long-term chronic inflammation is often accompanied by an elevation in serum globulin levels, indicating excessive activity of the immune system, commonly observed in diseases such as chronic hepatitis, rheumatoid arthritis and cancer (13, 14). Both protein and globulin are synthesized by the liver, and many studies have increasingly focused on the significant role of liver in the development and progression of AD (15). The inflammatory response and immunization necessitate a comprehensive consideration of albumin and globulin levels, referred to as the albumin-to-globulin ratio (AGR) (16). There is emerging evidence that the lower AGR plays a significant role in the prognosis of cancers (16), heart failure (17), and infectious diseases (18).
At present, the evidence regarding the relationship between AGR and cognitive function is inadequate. Two studies conducted in Japan revealed a positive association between AGR and cognitive function (19, 20). The routine laboratory variables, albumin and globulin respectively, could predict cognitive decline through a machine learning algorithm study for those aged ≥75 years (21). Unfortunately, this study did not consider the ratio between the two. There is growing interest in identifying individuals with high risks of developing dementia. Therefore, more high-quality cohort studies are needed to explore the relationship between AGR and cognitive decline, AD, and ADRD, along with the underlying mechanisms.
In this study, we investigated the correlation between serum AGR and cognitive function among the American older people. In addition, we aimed to examine whether the association between AGR and cognition follows a linear pattern.
Materials and methods
Study population
NHANES began in the early 1960s and has been a major and continuous program conducted by the National Center for Health Statistics (NCHS). NHANES was designed in a stratified, multistage probability sampling survey to assess the health and nutritional statistics (22). Moreover, NHANES has a primary role in collecting extensive examinations for the US older people to increase the knowledge of the aging population. The National Center for Health Statistics (NCHS) Ethics Review Board subsequently approved the NHANES protocol (23). All participants provided written informed consent before participating. The public data and survey design are available at the NHANES website.1
This study used data from two NHANES cycles (2011–2012 and 2013–2014). SEQN stands for the respondent sequence number and is a unique identifier for each participant in NHANES, so that there are no duplicate subjects in these two cycles. A total of 2,765 participants with complete cognitive functioning assessment and AGR data were extracted from a data pool of 19,931 participants. The flowchart for participant enrollment is presented in (Supplementary Figure S1).
Serum albumin (g/dL) and globulin (g/dL) concentrations were measured by the DcX800 system as a biochromatic digital endpoint method. The albumin-to-globulin ratio (AGR) was calculated as the albumin divided by the globulin.
Cognitive assessment
Participants aged ≥60 years are eligible for a series of cognitive assessments in NHANES (2011–2014), including word learning and recall modules from the Consortium to Establish a Registry for Alzheimer’s Disease (CERAD), the Animal Fluency test (AF) and the Digit Symbol Substitution test (DSST).
The CERAD Word Learning subtest, a major memory sub-domain to evaluate the immediate and delayed learning ability for new verbal information, includes three consecutive learning trials and a delayed recall. Every participant was instructed to read 10 unrelated words and was suggested to recall them immediately as many as possible for each trial. The delayed word recall occurred after completing AF and DSST. The maximum score for CERAD is 40.
The AF test was employed to evaluate the executive function by asking participants to name as many animals as possible in 1 min. The AF score is the total number of each named animal.
The DSST was a module from the Wechsler Adult Intelligence Scale (WAIS III) to assess processing speed, sustained attention, and working memory. Participants were asked to match the 133 corresponding symbols into the boxes next to the numbers in 2 min, with 133 being the highest score for DSST.
Additionally, a composite cognition score was created to represent global cognition and limit the uneven differences in individuals and the floor and ceiling effects (24), which was the summary of each standardized z-score of CERAD, AF, and DSST. The z-score was calculated as z = (x-m)/σ, where x shows the value of each individual, m presents the mean score of each test and σ is the standard deviation.
Covariates
The possible potential confounding factors were assessed, including sociodemographic [age, gender, race and ethnicity, education level, marital status and poverty-to-income ratios (PIR)], lifestyle [smoking habit, drinking habit, physical activity, body mass index (BMI)], and comorbidities (history of hypertension, diabetes, coronary heart disease, stroke, and depression).
Ages were classified as 60–69 years, 70–79 years, and ≥80 years. Gender included male and female. Race and ethnicity included five groups: Mexican American, other Hispanic, non-Hispanic White, non-Hispanic Black, and other races. Education was classified as <9, 9–12, and >12 years. Marital status was divided into two groups: married or living with a partner and living alone. The PIR was classified as low (<1.3), medium (1.3–3.5) and high (≥3.5).
Lifestyle consisted of smoking habit (at least 100 cigarettes in life), alcohol drinking habit (at least 12 alcoholic drinks per year), and physical activity (at least 10 min moderate-intensity). BMI was divided into normal (<25 kg/m2), overweight (25–30 kg/m2) and obese (≥30 kg/m2).
The comorbidities of hypertension, diabetes, coronary heart disease, and stroke were diagnosed by self-reported physician diagnosis. Depression was defined as ≥10 scores according to the Patient Health Questionnaire (PHQ-9).
For covariates with missing data, such as PIR (missing 8.25%), drinking habit (missing 1.70%), PHQ-9 (missing 1.99%), BMI (missing 1.45%), coronary heart disease (missing 0.54%), hypertension (missing 0.18%), stroke (missing 0.1%), physical activity (missing 0.11%), diabetes (missing 0.07%), marital status (missing 0.07%), smoke habit (0.07%) and education level (missing 0.07%), we used multiple imputation, based on 5 replications and a chained equation approach method in the R mice procedure, as described in Van Buuren and Groothuis-Oudshoorn (25).
Statistical analysis
No a priori calculation of statistical power was performed because the sample size was based on the available data from NHANES. All analyses were performed by R software (version 4.2.3; R Foundation for Statistical Computing)2 and Free Statistics software (version 1.9; Beijing Free Clinical Medical Technology Co., Ltd.). In all analyses, a two-sided p-value < 0.05 indicated statistical significance.
Firstly, the Kolmogorov–Smirnov test was used to determine the normality of continuous variables. Normally distributed variables were presented as mean (standard deviation), while skewed variables were presented as median (interquartile range, 25–75%). Categorical variables were represented by percentage (%). Statistical tests such as ANOVA, Kruskal–Wallis, and chi-squared tests were applied to compare differences across groups.
Then, the univariate and multivariate linear regression models were also used to examine the association of ARG and cognitive function in different dimensions. We converted AGR into a categorical variable according to the quartile and calculated the P for trend to verify the results of AGR as the continuous variable. Three models were adjusted based on clinical interest, previous scientific literature, or their associations with the outcomes of interest or a change in effect estimate of more than 10%. Model 1 was non-adjusted; Model 2 was adjusted for age, gender, race and ethnicity, education, marital status, and PIR; Model 3 was further adjusted for health habits (smoking, drinking, physical activity, and BMI) and comorbidities (hypertension, diabetes, coronary heart disease, stroke, depression).
We conducted a restricted cubic spline (RCS) with four knots (5th, 35th, 65th, and 95th) to explore the dose–response relationship between ARG and global cognition, modified by the cofounders consistent with model 3. We further developed a three-piecewise linear regression model of the relationship between AGR and global cognition. The subgroup analyses were also performed based on age, gender, race and ethnicity, PIR, BMI, and depression categories for interactions.
Finally, in the sensitivity analysis, we excluded individuals with missing covariate data and conducted multivariate linear regression and RCS to assess consistency with trends in individuals with complete data.
Results
Study population and baseline characteristics
A total number of 2,765 participants aged ≥60 years were included in the analysis. Table 1 shows the general characteristics of the participants according to AGR quartiles. Of those, the mean age was 69.5 (6.8) years, and 1,365 (49%) were male. Compared with other groups, group 4 (AGR ≥ 1.70) was more likely to be 60–69 years, male, Hispanic white, high education level, not living alone, and high level in PIR and a lower BMI. The higher AGR (≥1.70) was also associated with engagement in moderate physical activity, and a low incidence of diabetes and depression. In addition, participants had higher scores in all cognitive performance, including DSST, AF, CERAD, and global cognition. The basic characteristics of the excluded (AGR data missing) and included (AGR data completed) participants are shown in the Supplementary Table S1. Overall, the baseline data were stable, yet we cannot rule out the potential impact of non-random missing data on the results.
The association between AGR and cognitive function
The univariate analysis indicated that age, race and ethnicity, education level, marital status, PIR, drinking, moderate physical activity, hypertension, diabetes, coronary heart disease, stroke and depression were correlated with cognitive function (Supplementary Table S2).
Table 2 presents the results of the multivariable linear regression analysis examining the association between AGR and DSST, AF, CERAD and global cognition. AGR (per 0.1 unit) was positively associated with DSST score (β = 0.36, 95% CI: 0.21, 0.51), AF score (β = 0.1, 95% CI: 0.04, 0.16) and global cognition (β = 0.05, 95% CI: 0.02, 0.07), after being fully adjusted. When comparing the individuals in the lowest quartile of AGR (Q1 ≤ 1.31), the fully adjusted effect β (95% CI) for DSST in Q4 (≥1.70) was 3.45 (95% CI: 2.04, 4.85); for AF, 1.1 (95% CI: 0.54, 1.65); for global cognition, 0.51 (95% CI: 0.3, 0.72). In addition, the results also indicated a similar trend for DSST, AF, and global cognition (P for trend < 0.001).
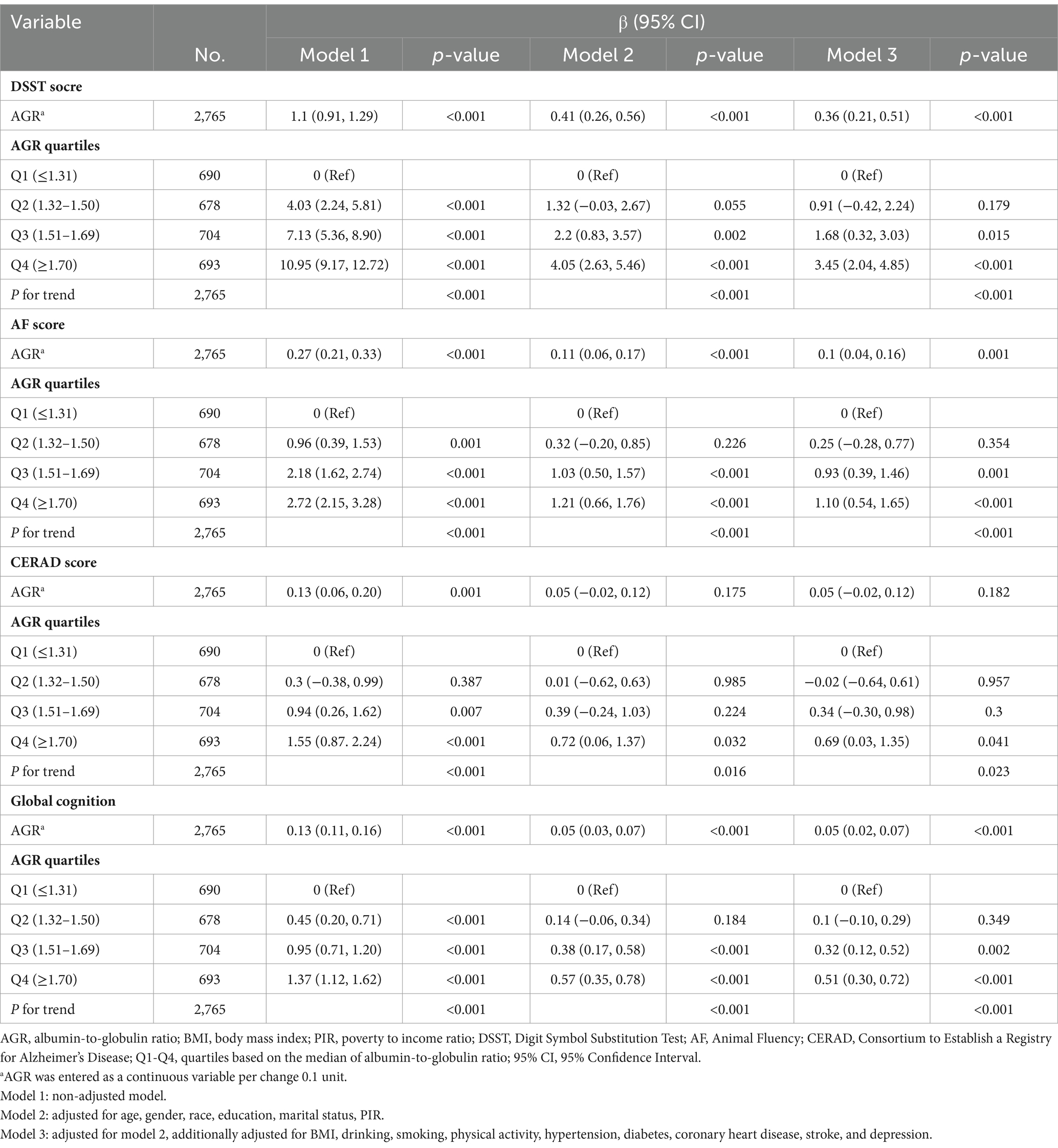
Table 2. Multivariable linear regression to assess the association of AGR with DSST, AF, CERAD, and global cognition.
However, AGR entered as a continuous variable was not related to the CERAD score (β = 0.05, 95% CI: −0.02, 0.12) in model 3. Interestingly, compared to the participants in Q1 (AGR ≤ 1.31), Q4 (AGR ≥ 1.70) (β = 0.69, 95% CI: 0.03, 1.35) had a higher score of CERAD in the fully adjusted model 3. The trend test also indicated a similar trend for CERAD (P for trend = 0.023).
Analysis of the non-linear relationship between AGR and cognition
The relationship between AGR and global cognition was found to be nonlinear rather than linear (P for nonlinearity = 0.017, Figure 1A). Additionally, the association between AGR and CERAD was also observed (P for non-linearity = 0.019, Figure 1B). However, linear relationships were observed between AGR and AF (P for non-linearity = 0.053, Figure 1C), and DSST (P for non-linearity = 0.322, Figure 1D). The non-linearity relationship between AGR and global cognition indicated an S-shaped pattern. Using a three-piecewise linear regression model with all cofounders being adjusted, the global cognition score remained relatively flat but inverse until AGR was 1.22; subsequently, it started to increase by 0.1 (β = 0.10, 95% CI: 0.05, 0.16) for every 0.1-unit increase when AGR was in the range of 1.22 to 1.8; when AGR was beyond 1.81, the global cognition did not increase significantly (Table 3).
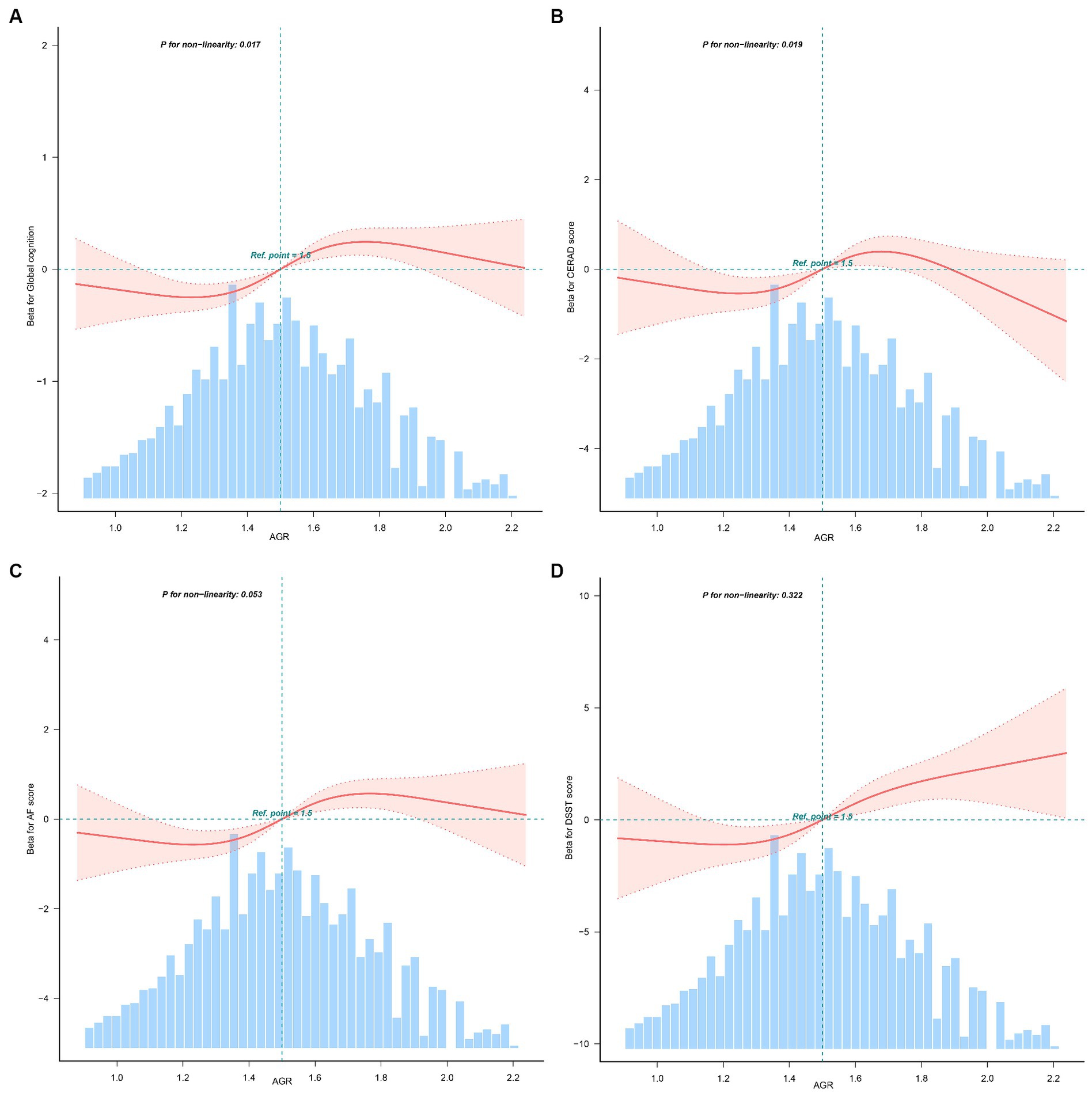
Figure 1. Non-linear relationship between AGR and global cognition, CERAD, AF, DSST (n = 2,765). AGR, albumin-to-globulin ratio; CERAD, Consortium to Establish a Registry for Alzheimer’s Disease; AF, Animal Fluency; DSST, Digit Symbol Substitution Test. (A) Relationship between AGR and global cognition; (B) relationship between AGR and CERAD; (C) relationship between AGR and AF; (D) relationship between AGR and DSST. Solid and dashed lines represent the predicted value and 95% confidence intervals. They were adjusted for age, gender, race, education, marital status, poverty-to-income ratio, body mass index, drinking, smoking, physical activity, hypertension, diabetes, coronary heart disease, stroke, and depression. Only 97.5% of the data is presented.
Stratified and sensitivity analysis
We further performed stratified analyses of the relationship between AGR and global cognition, AF, CERAD and DSST (Figure 2). The effect (β) of global cognition, CERAD, AF, and DSST, in subgroups was stable overall. Yet, the interaction test was significant for age based on global cognition (P for interaction = 0.036).
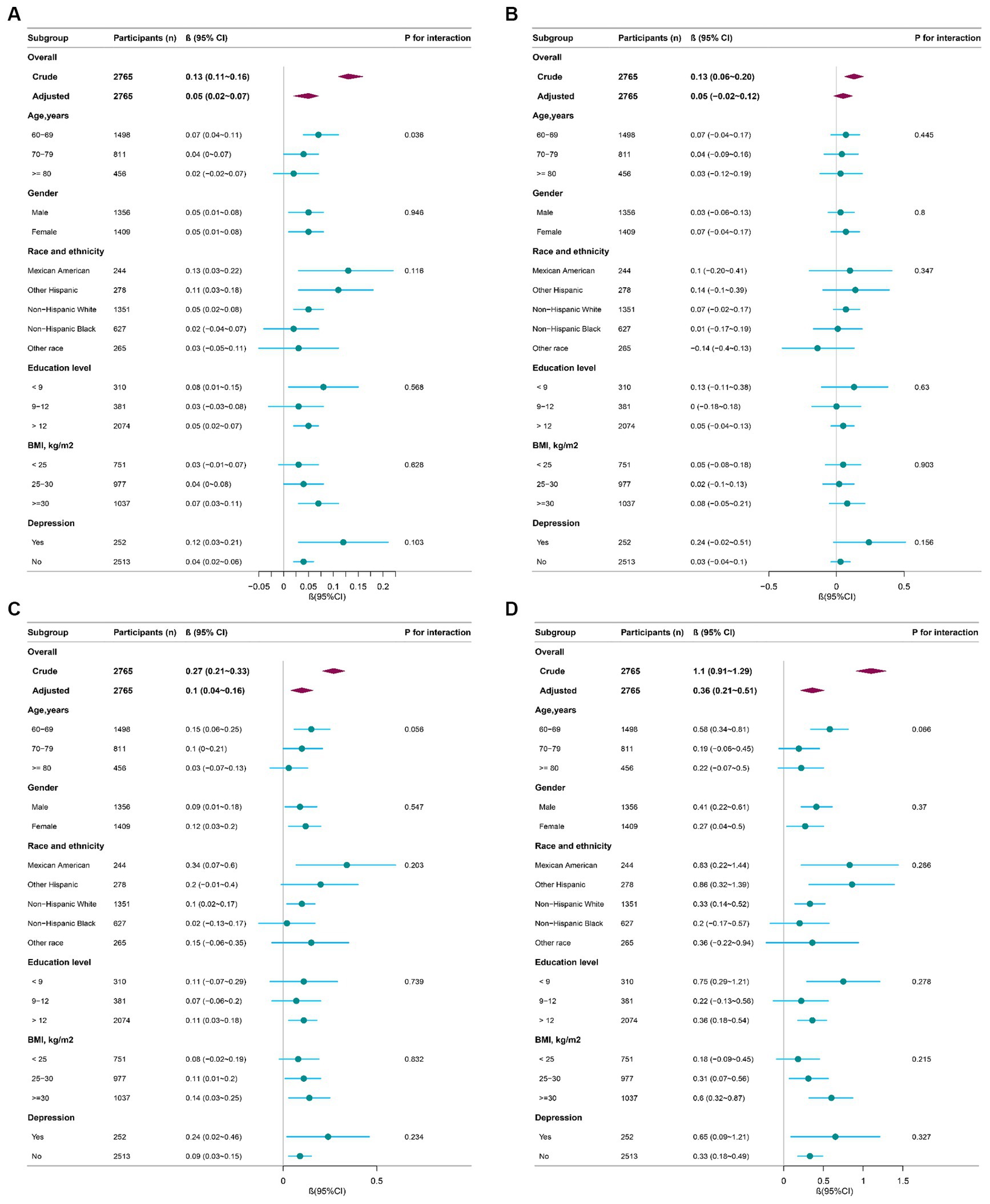
Figure 2. Effect size of AGR on global cognition, CERAD, AF, DSST in subgroups (n = 2,765). AGR, albumin-to-globulin ratio; CERAD, Consortium to Establish a Registry for Alzheimer’s Disease; AF, Animal Fluency; DSST, Digit Symbol Substitution Test. (A) Effect size of AGR on global cognition; (B) effect size of AGR on CERAD; (C) effect size of AGR on AF; (D) effect size of AGR on DSST. Adjusted for age, gender, race, education, marital status, poverty-to-income ratio, body mass index, drinking, smoking, physical activity, hypertension, diabetes, coronary heart disease, stroke, and depression.
During the sensitivity analysis, we compared differences between groups in the imputed variables (Supplementary Table S3), as the five datasets were generated after performing multiple imputations. The results indicated no statistically significant differences were found among the five datasets (p > 0.05). The results all suggested a non-linear relationship between AGR and Global cognition and CERAD (P for non-linearity < 0.05) for these five datasets. Similar results were observed when we conducted a multivariable linear regression and RCS (excluding the missing data of covariates, n = 2418) (Supplementary Table S4, Supplementary Figure S2).
Discussion
This population-based cross-sectional study found a positive relationship between AGR and cognitive function among 2,765 American older people. Moreover, we identified a non-linear relationship between AGR and global cognition. The global cognition started to increase by 0.1 for every 0.1-unit increase in AGR when it reached 1.22 (P for nonlinearity < 0.001).
Our findings are in line with several recent studies. A large prospective cohort study conducted in China observed the ratio of AGR was significantly decreased in both the pathological and clinical progression of AD, in which AGR is significantly associated with cerebrospinal fluid β-amyloid1–42 (Aβ1–42) (β = 0.182, pFDR < 0.001) (26). The positive association between AGR and cognitive function was observed in a case–control study and cross-sectional study in Japan, where the Mini-Mental State Examination (MMSE) and Montreal Cognitive Assessment (MoCA) were applied to assess cognitive function, respectively (19, 20). Chen et al. reported a higher AGR was associated with increased gray matter volume in the olfactory cortex and parahippocampal gyrus in young adults (27). Higher AGR means higher albumin and lower globulin (28). An elevated serum concentration of γ-globulin is an independent hematologic marker associated with cognitive decline in the dementia population (29). Therefore, it is reasonable to find out that AGR may serve as a protective factor and a potential indicator of cognitive performance.
A cross-sectional NHANES study detected a non-linear relationship between serum globulin and both AF and CERAD (30). The serum albumin presented a significant non-linear relationship with the risk of all-cause dementia (ACD) (31). Our study also detected a non-linear relationship between serum AGR and cognitive function which had never been reported before. Despite the clinical normal range of AGR being 1.0–2.0, there might be a possibility of cognitive decline associated with AGR even within this normal range. Therefore, we encourage maintaining AGR at an appropriate higher level. This is consistent with the finding of a prospective study conducted in Korea, indicating even within the normal range of serum albumin, individuals with albumin below 4.4 g/dL have an approximately three times higher risk of pathologic Aβ deposition (32). Our finding still needs further confirmation, but it indicated that poor nutrition or high inflammation in the older people may be linked to cognitive decline.
Additionally, our results showed a significant correlation between AGR and cognitive processing speed, sustained attention, and executive function (DSST, AF). However, there is no significant association with the immediate and delayed learning abilities related to the memory sub-domain (CERAD). This is largely consistent with several other cognitive studies based on the NHANES database (24, 30). A neuroimaging study identified an association between elevated AGR and the olfactory cortex and parahippocampal gyrus (27), which is involved in episodic memory and visuospatial processing (33). One explanation for this study may suggest the ability of execution and processing is more likely to be related to AGR. However, the underlying mechanism of AGR and cognitive dimensions needs further studies.
Increasing evidence suggests that inflammation is the leading culprit to dementia in the interaction between the down-regulated immune components, increased inflammation, and decreased neurogenesis (34). The amyloid cascade-inflammatory hypothesis has been proposed that Aβ peptide induces an inflammatory response that is enhanced by the presence of tau proteins, triggering the inflammatory activity of microglia, resulting in increased levels of inflammatory cytokines [tumor necrosis factor α, Interleukin 6, C-reactive protein (CRP)] (35). One meta-analysis investigated that chronic inflammatory diseases, elevated CRP, and other inflammatory factors increase vascular permeability, penetrate the blood–brain barrier, and infiltrate the brain, potentially developing dementia (36). The imbalance of AGR may reflect infection, malnutrition, chronic systematic inflammation, hepatic functional impairment, and autoimmunity (14, 37). AGR is related to cognition via reflection of homeostatic (non-high-density protein, high-CRP) (19). As the medium to long-term inflammatory, AGR was more sensitively related to the cognitive assessment than levels of CRP (20). Moreover, AGR has been demonstrated to be a valuable index for systemic inflammation in various diseases such as sepsis, systemic lupus erythematosus and rheumatoid arthritis (13, 38, 39).
New research is suggesting the role of the liver-brain axis for cognitive function as they might have potential communication pathways (27). Nho and colleagues highlighted the role of the liver in the involvement of metabolic disturbances in the progression of AD (40). The liver is the origin of brain Aβ deposits and is involved in the peripheral clearance of plasma Aβ through enzymes, albumin and lipid metabolism (15). There is a significant correlation between liver dysfunction and the volumes of the cognitive-related brain structures (hippocampus, thalamus, and amygdala regions) according to a prospective cohort study of 431,699 adults (31). The low AGR has been reported to be sensitively associated with liver malignancies (41). Besides the liver enzymes, albumin, globulin and the ratio of these two, play an active role in liver disease recovery (42).
There are some strengths of our study. The relationship between AGR and cognitive function was first investigated based on a national health and nutrition survey. Additionally, we considered a wide range of cofounders in this study. Further, a non-linear relationship between AGR and cognitive function was detected, suggesting a suitable range of AGR for better cognitive function. Although the mechanisms are not fully understood, our findings may have the potential to identify individuals at high risk of cognitive decline or dementia.
Our study also has limitations. First, all participants were Americans aged ≥60 years and the findings may not be generalizable to younger or populations of other races. Second, the cognitive assessments were DSST, AF, and CERAD, instead of the commonly used MMSE, MoCA, and the golden standard (European Consortium Criteria) for screening cognitive decline or mild cognitive impairment (43). Considering the reliability and validity of these cognitive assessments were compromised, we created a composite cognition z-score based on each test, assessing the global cognition domain (processing speed, sustained attention, working memory, executive function, and immediate and delayed memory). Moreover, our study was to investigate the relationship between AGR and cognitive performance, not MCI, AD, or ADRD. Thirdly, some residual confounders may exist, such as diet, medication interference, and other blood biomarkers. Finally, we could not make any causal inferences due to the cross-sectional study design. Future, prospective cohort studies are necessary to explore the cause-and-effect relationship between AGR and cognitive function.
Conclusion
The findings of this cross-sectional study suggested a positive and non-linear association between AGR and cognitive function in American older people. Maintaining AGR with an appropriate range (we suggested over 1.22) may be one of the therapeutic strategies to limit the progression of cognitive decline for the older people.
Data availability statement
The datasets presented in this study can be found in online repositories. The names of the repository/repositories and accession number(s) can be found below: http://www.cdc.gov/nchs/nhanes.htm.
Ethics statement
The studies involving humans were approved by The National Center for Health Statistics (NCHS) Ethics Review Board. The studies were conducted in accordance with the local legislation and institutional requirements. The participants provided their written informed consent to participate in this study.
Author contributions
HY: Conceptualization, Data curation, Formal analysis, Funding acquisition, Investigation, Methodology, Writing – original draft, Writing – review & editing. ZL: Conceptualization, Data curation, Investigation, Methodology, Writing – review & editing. YZ: Data curation, Investigation, Writing – original draft. ZG: Data curation, Formal analysis, Writing – review & editing. YM: Conceptualization, Supervision, Writing – review & editing, Writing – original draft.
Funding
The author(s) declare financial support was received for the research, authorship, and/or publication of this article. This study was supported by grants from Zhejiang Traditional Chinese Medicine Science and Technology Program [2023ZL055] and Medical Health Science and Technology Project of Zhejiang Provincial Health Commission [2022KY922].
Acknowledgments
We thank Dr. Liren Zeng for checking the language of this manuscript and the team of physician scientists for the consultation of statistics.
Conflict of interest
The authors declare that the research was conducted in the absence of any commercial or financial relationships that could be construed as a potential conflict of interest.
Publisher’s note
All claims expressed in this article are solely those of the authors and do not necessarily represent those of their affiliated organizations, or those of the publisher, the editors and the reviewers. Any product that may be evaluated in this article, or claim that may be made by its manufacturer, is not guaranteed or endorsed by the publisher.
Supplementary material
The Supplementary material for this article can be found online at: https://www.frontiersin.org/articles/10.3389/fpubh.2024.1375379/full#supplementary-material
Footnotes
References
1. Alzheimer’s Association . 2019 Alzheimer’s disease facts and figures. Alzheimers Dement. (2019) 15:321–87. doi: 10.1016/j.jalz.2019.01.010
2. Langa, KM, and Levine, DA. The diagnosis and management of mild cognitive impairment: a clinical review. JAMA. (2014) 312:2551–61. doi: 10.1001/jama.2014.13806
3. Albert, MS, DeKosky, ST, Dickson, D, Dubois, B, Feldman, HH, Fox, NC, et al. The diagnosis of mild cognitive impairment due to Alzheimer's disease: recommendations from the National Institute on Aging-Alzheimer's Association workgroups on diagnostic guidelines for Alzheimer's disease. Alzheimers Dement. (2011) 7:270–9. doi: 10.1016/j.jalz.2011.03.008
4. Mitchell, AJ, and Shiri-Feshki, M. Rate of progression of mild cognitive impairment to dementia--meta-analysis of 41 robust inception cohort studies. Acta Psychiatr Scand. (2009) 119:252–65. doi: 10.1111/j.1600-0447.2008.01326.x
5. Canevelli, M, Grande, G, Lacorte, E, Quarchioni, E, Cesari, M, Mariani, C, et al. Spontaneous reversion of mild cognitive impairment to normal cognition: a systematic review of literature and meta-analysis. J Am Med Dir Assoc. (2016) 17:943–8. doi: 10.1016/j.jamda.2016.06.020
6. Zhang, YR, Xu, W, Zhang, W, Wang, HF, Ou, YN, Qu, Y, et al. Modifiable risk factors for incident dementia and cognitive impairment: an umbrella review of evidence. J Affect Disord. (2022) 314:160–7. doi: 10.1016/j.jad.2022.07.008
7. Jaroudi, W, Garami, J, Garrido, S, Hornberger, M, Keri, S, and Moustafa, AA. Factors underlying cognitive decline in old age and Alzheimer's disease: the role of the hippocampus. Rev Neurosci. (2017) 28:705–14. doi: 10.1515/revneuro-2016-0086
8. McManus, RM, and Heneka, MT. Role of neuroinflammation in neurodegeneration: new insights. Alzheimers Res Ther. (2017) 9:14. doi: 10.1186/s13195-017-0241-2
9. Ferrucci, L, and Fabbri, E. Inflammageing: chronic inflammation in ageing, cardiovascular disease, and frailty. Nat Rev Cardiol. (2018) 15:505–22. doi: 10.1038/s41569-018-0064-2
10. Eckart, A, Struja, T, Kutz, A, Baumgartner, A, Baumgartner, T, Zurfluh, S, et al. Relationship of nutritional status, inflammation, and serum albumin levels during acute illness: a prospective study. Am J Med. (2020) 133:713–722.e7. doi: 10.1016/j.amjmed.2019.10.031
11. Biere, AL, Ostaszewski, B, Stimson, ER, Hyman, BT, Maggio, JE, and Selkoe, DJ. Amyloid beta-peptide is transported on lipoproteins and albumin in human plasma. J Biol Chem. (1996) 271:32916–22. doi: 10.1074/jbc.271.51.32916
12. Shojai, S, Rohani, H, Seyed-Ali, M-M, Akbar, A, and Habibi-Rezaei, M. Human serum albumin in neurodegeneration. Rev Neurosci. (2022) 33:803–17. doi: 10.1515/revneuro-2021-0165
13. Chen, Y, Chen, Y, Zhao, L, He, H, Wei, L, Lai, W, et al. Albumin/globulin ratio as Yin-Yang in rheumatoid arthritis and its correlation to Inflamm-aging cytokines. J Inflamm Res. (2021) 14:5501–11. doi: 10.2147/JIR.S335671
14. Zhang, CC, Zhang, CW, Xing, H, Wang, Y, Liang, L, Diao, YK, et al. Preoperative inversed albumin-to-globulin ratio predicts worse oncologic prognosis following curative hepatectomy for hepatocellular carcinoma. Cancer Manag Res. (2020) 12:9929–39. doi: 10.2147/CMAR.S275307
15. Bassendine, MF, Taylor-Robinson, SD, Fertleman, M, Khan, M, and Neely, D. Is Alzheimer's disease a liver disease of the brain? J Alzheimers Dis. (2020) 75:1–14. doi: 10.3233/JAD-190848
16. Salciccia, S, Frisenda, M, Bevilacqua, G, Viscuso, P, Casale, P, de Berardinis, E, et al. Prognostic value of albumin to globulin ratio in non-metastatic and metastatic prostate Cancer patients: a Meta-analysis and systematic review. Int J Mol Sci. (2022) 23:11501. doi: 10.3390/ijms231911501
17. Niedziela, JT, Hudzik, B, Szygula-Jurkiewicz, B, Nowak, JU, Polonski, L, Gasior, M, et al. Albumin-to-globulin ratio as an independent predictor of mortality in chronic heart failure. Biomark Med. (2018) 12:749–57. doi: 10.2217/bmm-2017-0378
18. Ye, Y, Chen, W, Gu, M, Xian, G, Pan, B, Zheng, L, et al. Serum globulin and albumin to globulin ratio as potential diagnostic biomarkers for periprosthetic joint infection: a retrospective review. J Orthop Surg Res. (2020) 15:459. doi: 10.1186/s13018-020-01959-1
19. Koyama, T, Kuriyama, N, Ozaki, E, Matsui, D, Watanabe, I, Miyatani, F, et al. Serum albumin to globulin ratio is related to cognitive decline via reflection of homeostasis: a nested case-control study. BMC Neurol. (2016) 16:253. doi: 10.1186/s12883-016-0776-z
20. Maeda, S, Takeya, Y, Oguro, R, Akasaka, H, Ryuno, H, Kabayama, M, et al. Serum albumin/globulin ratio is associated with cognitive function in community-dwelling older people: the septuagenarians, octogenarians, nonagenarians investigation with centenarians study. Geriatr Gerontol Int. (2019) 19:967–71. doi: 10.1111/ggi.13751
21. Gomes, KB, Pereira, RG, Braga, AA, Guimarães, HC, Resende, EPF, Teixeira, AL, et al. Machine learning-based routine laboratory tests predict one-year cognitive and functional decline in a population aged 75+ years. Brain Sci. (2023) 13:690. doi: 10.3390/brainsci13040690
22. Zipf, G, Chiappa, M, Porter, KS, Ostchega, Y, Lewis, BG, and Dostal, J. National health and nutrition examination survey: plan and operations, 1999-2010. Vital Health Stat 1. (2013) 1–37.
23. Centers for Disease Control and Prevention, National Center for Health Statistics . NCHS Research Ethics Review Board (ERB) Approval. Available at: https://www-cdc-gov.ezproxy.uwc.ac.za/nchs/nhanes/irba98.htm (Accessed December 1, 2020).
24. Zhang, Q, Zhang, M, Chen, Y, Cao, Y, and Dong, G. Nonlinear relationship of non-high-density lipoprotein cholesterol and cognitive function in American elders: a cross-sectional NHANES study (2011-2014). J Alzheimers Dis. (2022) 86:125–34. doi: 10.3233/JAD-215250
25. van Buuren, S, and Groothuis-Oudshoorn, K. mice: Multivariate imputation by chained equations in R. J Stat Softw. (2011) 45:1–67. doi: 10.18637/jss.v045.i03
26. Gao, PY, Ou, YN, Huang, YM, Wang, ZB, Fu, Y, Ma, YH, et al. Associations between liver function and cerebrospinal fluid biomarkers of Alzheimer's disease pathology in non-demented adults: the CABLE study. J Neurochem. (2024) 168:39–51. doi: 10.1111/jnc.16025
27. Chen, J, Liu, S, Wang, C, Zhang, C, Cai, H, Zhang, M, et al. Associations of serum liver function markers with brain structure, function, and perfusion in healthy young adults. Front Neurol. (2021) 12:606094. doi: 10.3389/fneur.2021.606094
28. Zhong, X, Liao, Y, Chen, X, Mai, N, Ouyang, C, Chen, B, et al. Abnormal serum bilirubin/albumin concentrations in dementia patients with Aβ deposition and the benefit of intravenous albumin infusion for Alzheimer's disease treatment. Front Neurosci. (2020) 14:859. doi: 10.3389/fnins.2020.0085
29. Klimkowicz, A, Dziedzic, T, Polczyk, R, Pera, J, Słowik, A, and Szczudlik, A. Journal of neurology, 251, 599-603. Factors associated with pre-stroke dementia. J Neurol. (2004) 251:599–603. doi: 10.1007/s00415-004-0384-5
30. Huang, J, Li, R, Zhu, H, Huang, D, Li, W, Wang, J, et al. Association between serum globulin and cognitive impairment in older American adults. Front. Public Health. (2023) 11:1193993. doi: 10.3389/fpubh.2023.1193993
31. Gao, PY, Ou, YN, Wang, HF, Wang, ZB, Fu, Y, He, XY, et al. Associations of liver dysfunction with incident dementia, cognition, and brain structure: a prospective cohort study of 431 699 adults. J Neurochem. (2024) 168:26–38. doi: 10.1111/jnc.15988
32. Kim, JW, Byun, MS, Lee, JH, Yi, D, Jeon, SY, Sohn, BK, et al. Serum albumin and beta-amyloid deposition in the human brain. Neurology. (2020) 95:e815–26. doi: 10.1212/WNL.0000000000010005
33. Aminoff, EM, Kveraga, K, and Bar, M. The role of the parahippocampal cortex in cognition. Trends Cogn Sci. (2013) 17:379–90. doi: 10.1016/j.tics.2013.06.009
34. Varnum, MM, and Ikezu, T. The classification of microglial activation phenotypes on neurodegeneration and regeneration in Alzheimer's disease brain. Arch Immunol Ther Exp. (2012) 60:251–66. doi: 10.1007/s00005-012-0181-2
35. Bolós, M, Perea, JR, and Avila, J. Alzheimer’s disease as an inflammatory disease. Biomol Concepts. (2017) 8:37–43. doi: 10.1515/bmc-2016-0029
36. Cooper, J, Pastorello, Y, and Slevin, M. A meta-analysis investigating the relationship between inflammation in autoimmune disease, elevated CRP, and the risk of dementia. Front Immunol. (2023) 14:1087571. doi: 10.3389/fimmu.2023.1087571
37. Takeuchi, I, Yanagawa, Y, Ota, S, Muramatsu, KI, Taniguchi, Y, Kiriu, N, et al. Usefulness of evaluating the albumin-to-globulin ratio in investigating the etiology of severe hypokalemia. J Emerg Trauma Shock. (2023) 16:72–3. doi: 10.4103/jets.jets_19_23
38. Hao, X, Wang, X, Ding, H, Zheng, S, Li, Z, Yin, H, et al. A model for sepsis prediction after retrograde intrarenal surgery and the use of the preoperative/postoperative white blood cell ratio to predict progression from sepsis to septic shock. World J Urol. (2022) 40:2979–90. doi: 10.1007/s00345-022-04182-1
39. Liu, XR, Qi, YY, Zhao, YF, Cui, Y, Wang, XY, and Zhao, ZZ. Albumin-to-globulin ratio (AGR) as a potential marker of predicting lupus nephritis in Chinese patients with systemic lupus erythematosus. Lupus. (2021) 30:412–20. doi: 10.1177/0961203320981139
40. Nho, K, Kueider-Paisley, A, Ahmad, S, MahmoudianDehkordi, S, Arnold, M, Risacher, SL, et al. Association of Altered Liver Enzymes with Alzheimer Disease Diagnosis, cognition, neuroimaging measures, and cerebrospinal fluid biomarkers. JAMA Netw Open. (2019) 2:e197978. doi: 10.1001/jamanetworkopen.2019.7978
41. Suh, B, Park, S, Shin, DW, Yun, JM, Keam, B, Yang, HK, et al. Low albumin-to-globulin ratio associated with cancer incidence and mortality in generally healthy adults. Ann Oncol. (2014) 25:2260–6. doi: 10.1093/annonc/mdu274
42. Ye, B, Zhang, J, Tan, Z, Chen, J, Pan, X, Zhou, Y, et al. Association of liver function with health-related physical fitness: a cross-sectional study. BMC Public Health. (2023) 23:1797. doi: 10.1186/s12889-023-16701-9
43. Alagiakrishnan, K, Mah, D, Dyck, JR, Senthilselvan, A, and Ezekowitz, J. Comparison of two commonly used clinical cognitive screening tests to diagnose mild cognitive impairment in heart failure with the golden standard European consortium criteria. Int J Cardiol. (2017) 228:558–62. doi: 10.1016/j.ijcard.2016.11.193
Keywords: albumin-to-globulin ratio, cognition, aging, cross-sectional study, NHANES
Citation: Yang H, Liao Z, Zhou Y, Gao Z and Mao Y (2024) Non-linear relationship of serum albumin-to-globulin ratio and cognitive function in American older people: a cross-sectional national health and nutrition examination survey 2011–2014 (NHANES) study. Front. Public Health. 12:1375379. doi: 10.3389/fpubh.2024.1375379
Edited by:
José Daniel Jiménez García, University of Jaén, SpainReviewed by:
Chun-Hua Zhao, Suzhou Municipal Hospital, ChinaAlena Sidenkova, Ural State Medical University, Russia
Copyright © 2024 Yang, Liao, Zhou, Gao and Mao. This is an open-access article distributed under the terms of the Creative Commons Attribution License (CC BY). The use, distribution or reproduction in other forums is permitted, provided the original author(s) and the copyright owner(s) are credited and that the original publication in this journal is cited, in accordance with accepted academic practice. No use, distribution or reproduction is permitted which does not comply with these terms.
*Correspondence: Yajun Mao, maoyaj008@163.com
†These authors have contributed equally to this work