- 1Department of Emergency Medicine, The First People’s Hospital of Kunshan, Kunshan, China
- 2Department of Endocrinology, The First People’s Hospital of Kunshan, Kunshan, China
Background: The inflammatory response holds paramount significance in the context of intracerebral hemorrhage (ICH) and exhibits a robust correlation with mortality rates. Biological markers such as the neutrophil-to-lymphocyte ratio (NLR), platelet-to-lymphocyte ratio (PLR), lymphocyte-to-monocyte ratio (LMR), systemic immune inflammation index (SII), and systemic inflammatory response index (SIRI) play crucial roles in influencing the systemic inflammatory response following ICH. This study aims to compare the predictive efficacy of NLR, PLR, LMR, SII, and SIRI concerning the risk of mortality in the intensive care unit (ICU) among critically ill patients with ICH. Such a comparison seeks to elucidate their early warning capabilities in the management and treatment of ICH.
Methods: Patients with severe ICH requiring admission to the ICU were screened from the Medical Information Marketplace for Intensive Care (MIMIC-IV) database. The outcomes studied included ICU mortality and 30 day ICU hospitalization rates, based on tertiles of the NLR index level. To explore the relationship between the NLR index and clinical outcomes in critically ill patients with ICH, we utilized receiver operating characteristic (ROC) analysis, decision curve analysis (DCA), and multivariate logistic regression analysis.
Results: A total of 869 patients (51.9% male) were included in the study, with an ICU mortality rate of 22.9% and a 30 day ICU hospitalization rate of 98.4%. Among the five indicators examined, both the ROC curve and DCA indicated that NLR (AUC: 0.660, 95%CI: 0.617–0.703) had the highest predictive ability for ICU mortality. Moreover, this association remained significant even after adjusting for other confounding factors during multivariate analysis (HR: 3.520, 95%CI: 2.039–6.077). Based on the results of the multivariate analysis, incorporating age, albumin, lactic acid, NLR, and GCS score as variables, we developed a nomogram to predict ICU mortality in critically ill patients with ICH.
Conclusion: NLR emerges as the most effective predictor of ICU mortality risk among critically ill patients grappling with ICH when compared to the other four indicators. Furthermore, the integration of albumin and lactic acid indicators into the NLR nomogram enhances the ability to promptly identify ICU mortality in individuals facing severe ICH.
Introduction
Spontaneous intracerebral hemorrhage (ICH) represents a prevalent subtype of stroke characterized by elevated morbidity, substantial disability, and heightened mortality rates. This condition poses a significant threat to the lives and well-being of over 3 million individuals globally each year (1). With the incidence of ICH increasing annually, it imposes a considerable burden on patients, their families, and society as a whole (2, 3). Even with interventions such as early hematoma removal surgery, blood pressure control, and hemostasis, the mortality rate associated with ICH remains alarmingly high, reaching up to 68% (4).
Indeed, ICH is recognized not only as a localized brain condition but also as a systemic disease impacting multiple organ systems. A growing body of evidence underscores the pivotal role played by systemic inflammation and immune responses in the pathophysiological processes of ICH (5). Brain damage caused by ICH triggers an inflammatory immune response. This response, mediated through the sympathetic nervous system and the hypothalamus-pituitary-adrenal (HPA) axis, leads to persistent inflammation, reducing systemic immune activity and inhibiting cellular immune response. Consequently, this exacerbates the condition of patients with ICH and significantly impacts their prognosis. White blood cell counts and its subtypes counts are associated with hematoma enlargement, mortality, and unfavorable prognosis in ICH (6, 7). The Neutrophil-to-Lymphocyte Ratio (NLR) has shown promise as a valuable biomarker for predicting the prognosis of stroke (8). Furthermore, the NLR and Platelet-to-Lymphocyte Ratio (PLR) have been identified as crucial indicators of prognosis in patients with ICH (9). The lymphocyte to monocyte ratio (LMR) may be associated with the long-term neurological prognosis of acute ischemic stroke and can serve as a supplementary marker for the diagnosis of chronic heart failure (10, 11). The systemic inflammatory response index (SIRI), derived from neutrophil, monocyte, and lymphocyte counts, stands as a novel systemic inflammatory marker. It has been identified as an independent prognostic indicator for various tumors and could predict the risk of aneurysmal subarachnoid hemorrhage (SAH) (12–14). The systemic immune-inflammation index (SII) is a novel biomarker for malignancy and inflammatory diseases, calculated based on platelet, neutrophil, and lymphocyte counts. It has been used to predict adverse outcomes following cerebral hemorrhage and cerebral vasospasm in late aneurysmal SAH patients (15, 16). Additionally, SII has proven useful in identifying high-risk groups for poor prognosis in chronic kidney disease and abdominal aortic calcification, thereby aiding in preventive measures for the general population (17, 18). Despite the existence of immune inflammation-related biomarkers, their predictive capabilities regarding the mortality of patients with ICH remain relatively insufficient. This limitation hinders their ability to serve as effective warning indicators in treatment decisions.
Therefore, the primary objective of this study was to assess the predictive capability of NLR, PLR, LMR, SIRI, and SII values upon admission for ICU mortality in patients with ICH. Additionally, the study aimed to explore the potential application of these markers in the early identification of ICH severity.
Methods
Study population
The current investigation utilized health-related data from the MIMIC-IV (version 2.2) repository, a widely-used extensive database developed and overseen by the MIT Computational Physiology Laboratory (19). GZ, a researcher, adhered to all access requirements and conducted the data extraction. This study included patients diagnosed with ICH based on the International Classification of Diseases, 9th and 10th Revision (ICD code: ICD-9th: 431, 7,670, 7,721; ICD-10th: I61, I62). Specific exclusion criteria were applied as follows: (1) individuals under the age of 18 upon their initial admission; (2) patients with ICH caused by traumatic brain injury, brain tumor, cerebral aneurysm, or cerebral arteriovenous malformation; (3) patients with multiple admissions for ICH, with data extracted only from the first admission; (4) patients with severe liver and kidney conditions, leukemia, lymphoma, other hematological diseases, or malignant tumors (to eliminate the potential bias caused by abnormal hematology indices on the study results); (5) patients lacking sufficient data, specifically Neutrophil and Lymphocyte counts, on the first day of admission. In total, 869 patients were enrolled in this research and categorized into three groups according to the tertiles of the NLR index (Figure 1).
Data collection
The information extraction process utilized PostgreSQL (version 14.10) software and Navicat Premium (version 16), with Structured Query Language (SQL) being the tool for extraction. The potential variables were grouped into four main categories: (1) demographic variables, including age, race, sex, and weight, (2) comorbidities, encompassing hypertension, diabetes, and hyperlipemia, (3) laboratory indicators, consisting of red blood cells (RBC), white blood cells (WBC), hemoglobin (Hb), platelet (PLT), neutrophil, lymphocyte, monocyte, lactic acid, and albumin, (4) the Glasgow Coma Scale score (GCS), with lower scores indicating a worse level of consciousness. Patients with ICH were stratified into three groups based on GCS scores: 13–15 for mild coma, 9–12 for moderate coma, and 3–8 for severe coma. Equations were applied to calculate various parameters, such as NLR, PLR, SII, and SIRI, as follows: NLR = count of neutrophils/count of lymphocytes; PLR = count of platelets/count of lymphocytes; LMR = count of lymphocytes/count of monocytes; SIRI = (count of neutrophils × count of monocytes)/count of lymphocytes; and SII = (count of neutrophils × count of platelets)/count of lymphocytes. All laboratory variables and disease severity scores were obtained from data generated within the initial 24 h of patient admission.
Statistical analysis
Continuous variables were presented as means ± standard deviations (SDs) or medians (interquartile ranges, IQRs) and compared using Student’s t-test or Kruskal–Wallis rank sum test, as appropriate. Categorical variables were expressed as frequencies and percentages, and Fisher exact tests were employed for comparison when appropriate. Logistic regression analyses were conducted to assess the independent association between NLR/PLR/LMR/SIRI/SII and ICU mortality. An extended logistic model approach was applied for different covariates-adjusted models, leading to the construction of three models: Model 1, adjusted for age, race, sex, marital status, and weight; Model 2, additionally adjusted for hypertension, diabetes, and hyperlipidemia. The presence of confusion was evaluated by including or excluding covariates in the logistic regression model, and regression coefficients were compared. A significance level of two-sided p values <0.05 was considered statistically significant. The predictive ability of NLR/PLR/LMR/SIRI/SII was determined by calculating the area under the receiver operating characteristic curve (AUC). Receiver operating characteristic (ROC) analyses and Decision Curve Analyses (DCAs) were utilized to evaluate predictive performance. Stratified analyses were conducted to assess potential modifications of the association between NLR and ICU mortality in ICH patients by sex, marital status, race, GCS, hypertension, diabetes, hyperlipidemia, and 30 day ICU hospitalization rate. Based on the results of multivariate logistic regression analysis, a nomogram was developed to predict ICU mortality. The appropriateness of the nomogram for clinical application was determined by the calibration curve. Statistical analyses were conducted using IBM SPSS 24.0 and Empowerstats software (version 6.0).
Results
Baseline characteristics
This study included a total of 869 ICU patients with ICH, divided into 2 groups based on ICU death. The baseline characteristics of ICH patients are detailed in Table 1. The average age was 69.5 years (60.3–81.9 years), with 451 cases (51.9%) being male. Within the total sample, there were 199 ICU deaths (22.9%) and 852 patients requiring 30 day ICU hospitalization (98.4%). The non-survival group tended to have older individuals, higher lactic acid levels on admission, and lower GCS scores. A lower GCS score indicates poorer neurological function and a lower level of consciousness, suggesting a severe ICH and a higher risk of death. In the non-survival group, NLR (p < 0.001), PLR (p = 0.033), SII (p < 0.001), and SIRI (p < 0.001) were significantly higher compared to the survival group, while LMR (p < 0.001) was lower (Table 1; Figure 2).
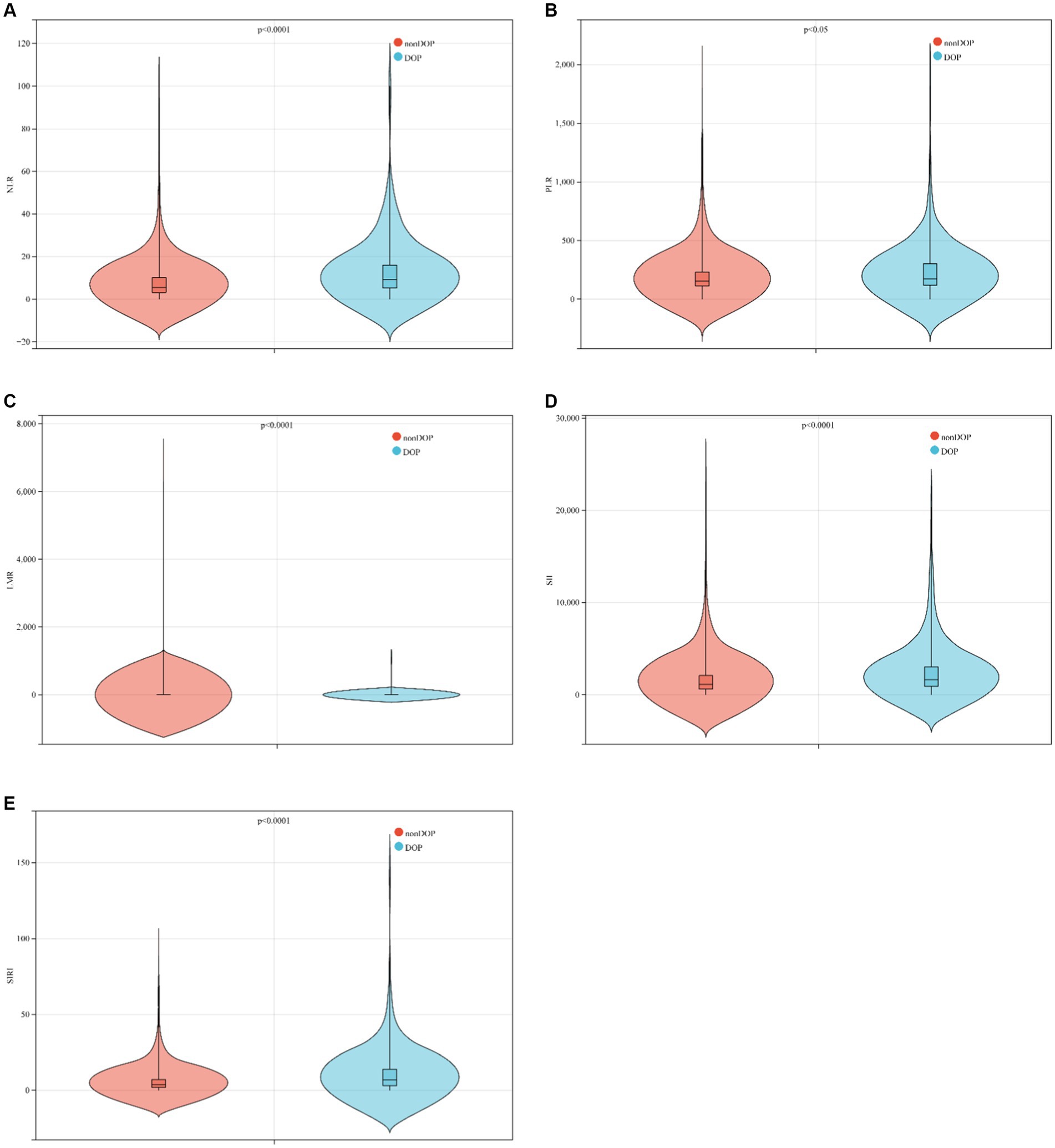
Figure 2. Boxplots of the NLR, PLR, LMR, SII and SIRI showing the distribution in the survivor group and non-survivor group. (A) The NLR of the non-survivor group was higher than that of the survivor group (p < 0.0001); (B) the PLR of the non-survivor group was higher than that of the survivor group (p < 0.05); (C) the LMR of the non-survivor group was lower than that of the survivor group (p < 0.0001); (D) the SII of the non-survivor group was higher than that of the survivor group (p < 0.0001); (E) the SII of the non-survivor group was higher than that of the survivor group (p < 0.0001).
The baseline characteristics of critically ill patients with ICH were further analyzed based on tertiles of NLR markers, as detailed in Table 2. Patients were stratified into three groups according to their NLR levels at admission: tertile (T)1 (0.46–4.14), T2 (4.15–9.22), and T3 (9.23–100). It was observed that patients in the highest tertile of NLR exhibited elevated levels of WBC, neutrophils, and monocytes, along with lower levels of lymphocytes and albumin. Furthermore, the highest NLR tertile was associated with higher ICU mortality compared to the lower group (10.345% vs. 24.221% vs. 34.138%, p < 0.001).
Primary outcomes
ROC analysis and DCA were employed to assess the predictive ability of the biomarkers for ICU mortality in ICH (Figure 3). AUC values were determined, revealing that NLR had the highest AUC (AUC: 0.657, 95%CI: 0.613–0.701) compared to PLR (AUC: 0.549, 95%CI: 0.501–0.597), LMR (AUC: 0.635, 95%CI: 0.591–0.680), SII (AUC: 0.609, 95%CI: 0.563–0.654), and SIRI (AUC: 0.649, 95%CI: 0.604–0.694). This suggests that NLR demonstrated a better ability to predict ICU mortality in ICH. Further details, including optimal cutoff values, specificity, and sensitivity, are provided in Table 3.
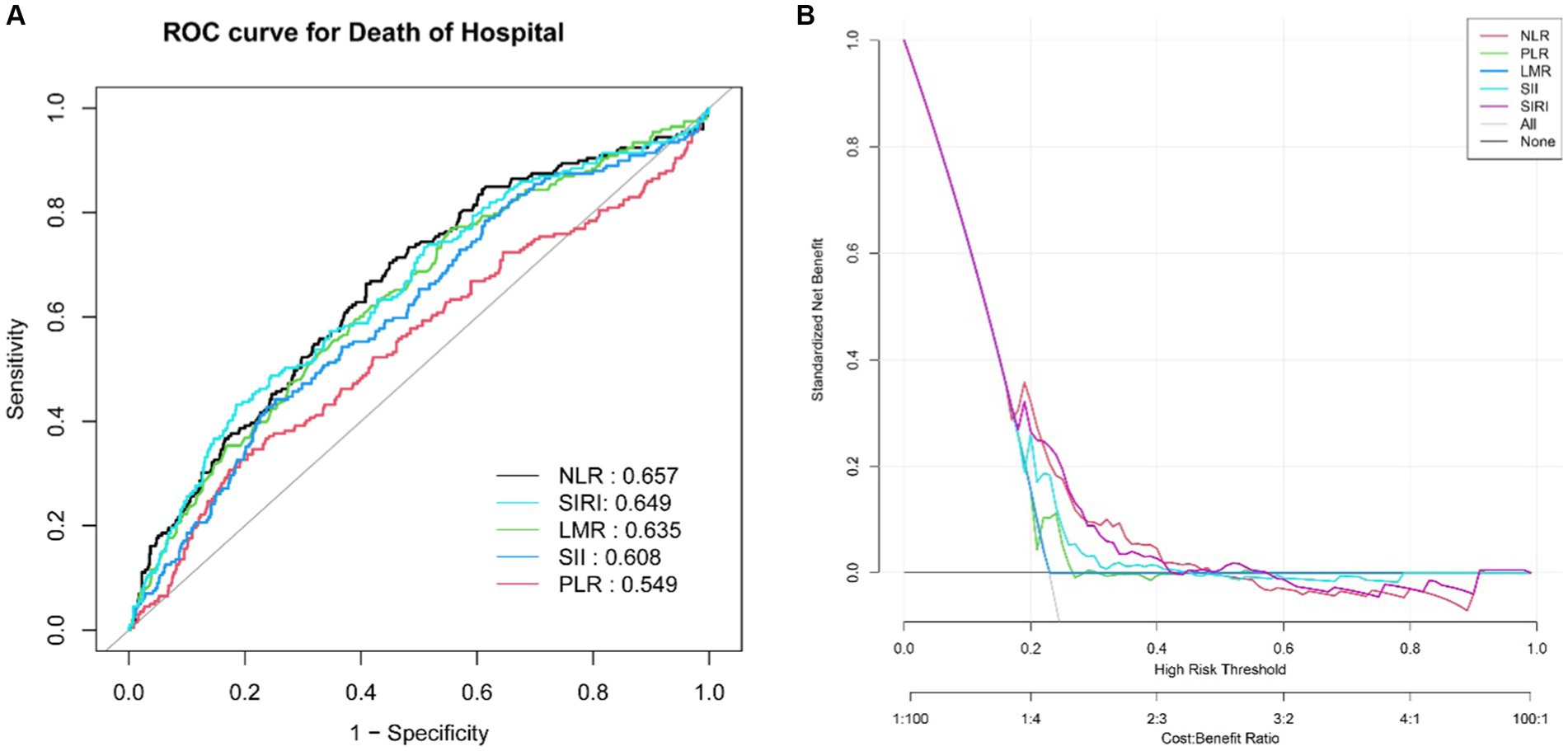
Figure 3. ROC curves of the NLR, PLR, LMR, SII and SIRI for predicting ICU mortality (A). The DCA for evaluating the accuracy and practicability of the NLR, PLR, LMR, SII and SIRI, respectively (B).
In the multivariate logistic regression analysis, three models were established (Table 4): an unadjusted model, a partly adjusted model (adjusted for age, sex, weight, race, and marital status), and a fully adjusted model (adjusted for age, sex, weight, race, marital status, hypertension, diabetes, hyperlipidemia, and GCS score). The results across these models consistently identified NLR, albumin, lactic acid, SII, and SIRI as noteworthy risk factors for ICU mortality in patients with ICH. When the NLR index was considered as a nominal variable, patients with a higher NLR index were associated with a significantly elevated risk of ICU mortality compared with patients in the lowest tertile of NLR index across all three established logistic proportional hazards models: Unadjusted model (HR: 4.492, 95%CI: 2.867–7.039), partly adjusted model (HR: 3.549, 95%CI: 2.076–6.068), and fully adjusted model (HR: 3.520, 95%CI: 2.039–6.077). The NLR index exhibited an increasing trend with ICU mortality (Table 4).
Based on the results of multivariate logistic regression analysis, a nomogram was developed to predict the risk of ICU death in patients with ICH. Using age, albumin, lactic acid, NLR, and GCS score as variables, the calibration curve demonstrated good consistency between predicted and actual probabilities (C-index: 0.694, 95%CI: 0.643–0.745), indicating acceptable accuracy in predicting ICU mortality (Figure 4).
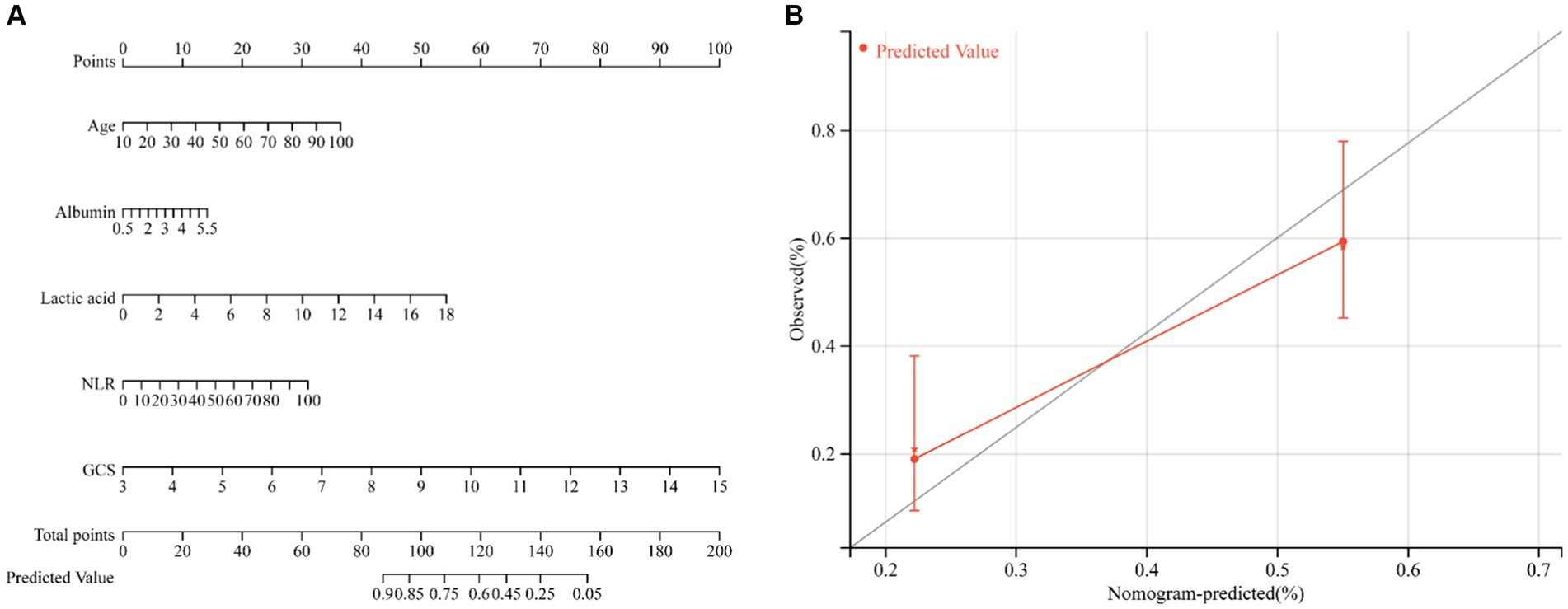
Figure 4. Nomogram to estimate the risk of ICU mortality in patients with ICH (A). The calibration curve was used to evaluate the utility of the nomogram (B).
Subgroup analysis
The results of further stratified analyses indicate a significant association between the NLR index and a higher risk of in-hospital ICU mortality in specific subgroups of patients with ICH (Figure 5). The subgroups that showed a significant association include patients who were married (HR: 1.045, 95%CI: 1.017–1.073), of white ethnicity (HR: 1.045, 95%CI: 1.022–1.067), and those with a GCS score indicating mild coma (HR: 1.041, 95%CI: 1.024–1.058). Notably, subgroup analysis based on sex, hypertension, diabetes mellitus, and hyperlipidemia did not yield significant results, likely due to the predominantly older adult population in this study.
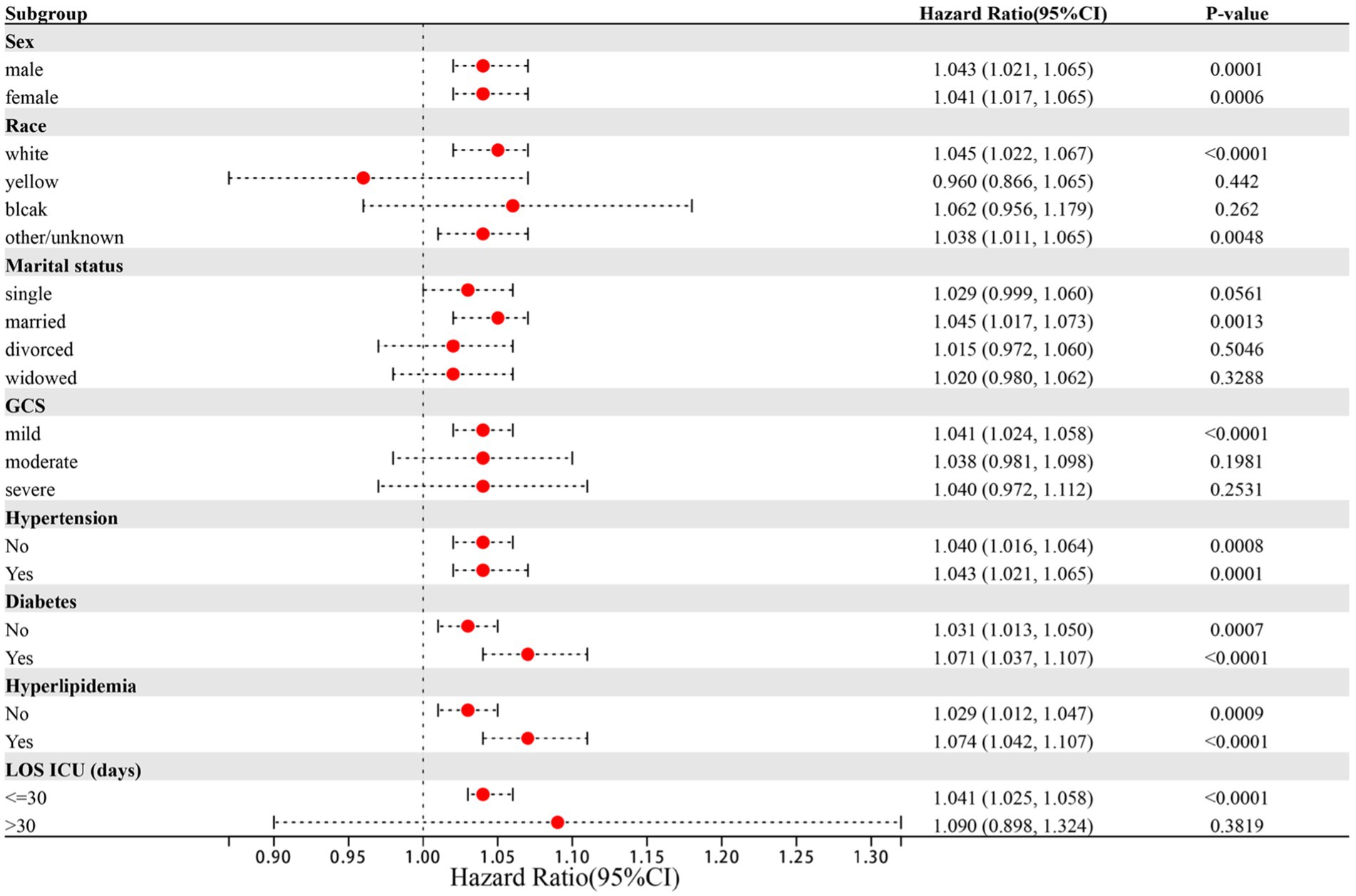
Figure 5. Forest plots of hazard ratios for the ICU mortality in different subgroups. HR, hazard ratio; CI, confidence interval.
Discussion
In clinical practice, ICH is a prevalent critical disease associated with a high mortality rate (20). This prospective study aims to assess the association between inflammatory indexes and clinical outcomes in critically ill patients with ICH focusing on inflammatory immune cells. For the first time, we conducted a comparative analysis of the predictive value of NLR, PLR, LMR, SII, and SIRI for ICU mortality risk in patients with ICH. The results revealed that NLR, SIRI, and LMR exhibited high predictive value for ICU mortality, with NLR demonstrating a significant association with ICU mortality in patients with ICH. These findings are anticipated to enhance early prediction and identification of ICU mortality in ICH patients.
During the process of ICH, the rupture of blood vessels and subsequent formation of hematoma initiate a range of inflammatory and immune responses. These include the activation of inflammatory cells as well as the release of inflammatory mediators (21). The hematoma triggers the activation of microglia, while immune cells such as neutrophils, monocytes, and lymphocytes from the peripheral blood are recruited through the disrupted blood–brain barrier (BBB). Consequently, they rapidly infiltrate the brain tissue, each exerting their distinct roles in the occurrence and progression of neuroinflammation (22). Previous studies have provided evidence linking leukocytosis with severe disability, unfavorable neurological outcomes, and increased mortality rates (23, 24). In terms of neutrophils and monocytes, their accumulation both in the bloodstream and at the site of injury represents a crucial characteristic of inflammation, serving as a response to either infection or the intensity of the inflammatory process (25). Lymphocytes, on the other hand, act as primary regulators of the immune system and hold a significant role in defending the host against pathogens. A decrease in lymphocyte count results in weakened immune capabilities and heightened susceptibility to infections (26, 27). Furthermore, abnormalities in platelet function directly reflect the coagulation response following ICH, thus influencing its prognosis (28, 29).
In this study, we conducted an investigation into the forecasting of five indicators to assess the risk of mortality in ICU for ICH patients. The outcomes demonstrated that the following indicators, namely, NLR, SIRI, SII, and LMR, exhibited strong predictive capabilities, with an AUC greater than 0.600. Through the process of adjusted multivariate logistic regression analysis, it was determined that NLR, SIRI, and SII independently contributed to the risk factors, but subsequent comparison revealed that NLR presented the highest predictive ability compared to the other four indicators. Therefore, it is crucial to calculate the values of NLR, SII, and SIRI upon admission for critically ill ICH patients, with particular emphasis on NLR, as a means to establish early intervention strategies and ensure a favorable prognosis for patients with severe ICH. It should be noted that SII and SIRI serve as novel inflammatory biomarkers. SIRI effectively reflects the overall homeostasis between inflammatory response and immune function, while SII integrates thrombotic activity, inflammatory response, and adaptive immune response to identify high-risk individuals who are likely to experience unfavorable outcomes or death subsequent to ICH (30–32). Nevertheless, it is worth mentioning that the predictive capacity of SII on mortality risk is affected by the time frame in which the baseline blood sample is obtained. Multiple studies have confirmed that a median SII value at approximately 29 h after ICH onset corresponds to the prognosis of 90 days after, whereas the baseline SII value in our research was obtained within 24 h, thus leading to a potential discrepancy due to a certain lag (33). NLR and SIRI exhibit substantial similarities in terms of their components, yet NLR surpasses SIRI in its ability to predict ICU mortality in cases of ICH. Additionally, this study delved into a comprehensive analysis of the risk stratification within each subgroup. Our subgroup analysis revealed that the predictive value of NLR concerning ICU mortality risk remained consistent across both male and female patients. Nevertheless, more attention should be given to factors such as marital status, race, and the Glasgow Outcome Scale. While the Glasgow Coma Scale is commonly used to assess the severity of intracerebral hemorrhage, it is important to also consider cases of mild coma. In this study, we found that racial differences also impact the risk of ICU death. This can be attributed to genetic variations between races, which may influence the structure and function of cerebral vasculature (34). Additionally, lifestyle differences, such as high-salt and high-fat diets, along with unhealthy habits like alcohol and tobacco use, can contribute to conditions such as diabetes, high cholesterol, and obesity, thereby increasing the risk of ICH death (35). Similarly, differences in social roles and the levels of stress individuals experience can also affect the risk of death from ICH. Married patients often play crucial roles in their families, such as caring for children, spouses, and parents. The physical stress from these responsibilities, the financial pressures of earning money, and the psychological stress from marital discord can negatively impact their health, leading to a higher risk of ICH and a worse prognosis (36, 37). Epidemiological studies suggest that marital instability may reduce patients’ ability to prevent and treat diseases, potentially increasing the risk of death from ICH (38, 39). Additionally, studies have confirmed that adults who remarry experience more serious physical and psychological diseases than those in stable and healthy marriages (40, 41). Therefore, maintaining a healthy and stable marriage can help patients reduce anxiety and stress, promote active treatment, and lower the risk of death from ICH.
In the study, we unexpectedly found that lactic acid and albumin were also independent risk factors for ICU mortality risk in ICH. Lactic acid is widely regarded as an indicator of tissue hypoperfusion and has been shown to be closely related to mortality in severe diseases such as sepsis, shock, and trauma (42–44). In the non-surviving patients, lactic acid levels were significantly higher than in the surviving group. Hematoma compression of the brain parenchyma leads to insufficient blood flow, which in turn causes astrocytes to take up glucose and metabolize it into lactic acid under the stimulation of large amounts of glutamate. The generated lactic acid is transferred to neurons and enters the tricarboxylic acid cycle to meet the energy demand of the brain (45, 46). Although higher serum lactic acid is beneficial for energy supplementation, the adverse pathophysiological conditions indicated by higher serum lactic acid may have a greater impact on outcomes than the relatively transient and small effects of energy supplementation. Albumin is produced by hepatocytes to maintain the physiological functions of the healthy body in various ways. Low albumin levels are also considered a valid marker of malnutrition (47). In this study, the albumin level of the non-survivors was obviously lower than that of the survivors, which further confirmed the decrease of albumin after ICH and its relationship with mortality (48, 49). Based on this, the present study demonstrates the utility of age, albumin, lactic acid levels, NLR value, and GCS score as predictive variables for assessing the risk of ICU mortality among patients with ICH. This model holds particular promise in facilitating timely symptomatic interventions and serving as a warning system for critically ill patients with ICH to prevent disease progression and avoid the deterioration of the disease.
This study has some limitations that need to be acknowledged. Firstly, we lacked information on whether patients with ICH underwent surgical treatment or not. Secondly, patients’ personal histories, including smoking and alcohol consumption, were not available, and their nutritional status or the severity of comorbid conditions were not captured in the database. Additionally, we only recorded inflammatory markers for the first 24 h after admission. The inflammatory response may evolve over several days following the onset of ICH. Therefore, further investigations are warranted to explore the changes in inflammatory factors at different time points after onset and their predictive value.
Conclusion
In conclusion, our study reveals that NLR, SIRI, and LMR serve as effective predictors of ICU mortality risk in patients with ICH. Notably, NLR exhibits superior predictive performance. Simultaneously, constructing a nomogram with NLR, lactic acid, and albumin as variables proves beneficial for clinical decision-making and disease management, mitigating the risk of ICH deterioration.
Data availability statement
Publicly available datasets were analyzed in this study. This data can be found at: doi: 10.13026/6mm1-ek67.
Ethics statement
Ethical review and approval was not required for the study on human participants in accordance with the local legislation and institutional requirements. Written informed consent from the [patients/participants OR patients/participants legal guardian/next of kin] was not required to participate in this study in accordance with the national legislation and the institutional requirements.
Author contributions
GZ: Writing – original draft, Writing – review & editing, Data curation, Methodology, Resources, Software. YG: Data curation, Writing – review & editing. ZW: Methodology, Software, Writing – review & editing. YC: Formal analysis, Validation, Writing – review & editing. XX: Writing – review & editing, Formal analysis, Validation.
Funding
The author(s) declare that financial support was received for the research, authorship, and/or publication of this article. This project is supported by Suzhou Municipal Youth Science and Technology Project (No. KJXW2023075).
Conflict of interest
The authors declare that the research was conducted in the absence of any commercial or financial relationships that could be construed as a potential conflict of interest.
Publisher’s note
All claims expressed in this article are solely those of the authors and do not necessarily represent those of their affiliated organizations, or those of the publisher, the editors and the reviewers. Any product that may be evaluated in this article, or claim that may be made by its manufacturer, is not guaranteed or endorsed by the publisher.
Abbreviations
ICH, intracerebral hemorrhage; ICU, intensive care unit; NLR, neutrophil-to-lymphocyte ratio; PLR, platelet-to-lymphocyte ratio; LMR, lymphocyte-to-monocyte ratio; SII, systemic immune inflammation index; SIRI, systemic inflammatory response index; MIMIC-IV, Medical Information Marketplace for Intensive Care; GCS, Glasgow coma scale score; BBB, blood–brain barrier.
References
1. Xue, M, and Yong, VW. Neuroinflammation in intracerebral haemorrhage: immunotherapies with potential for translation. Lancet Neurol. (2020) 19:1023–32. doi: 10.1016/s1474-4422(20)30364-1
2. Huang, J, Chen, H, Deng, J, Liu, X, Shu, T, Yin, C, et al. Interpretable machine learning for predicting 28-day all-cause in-hospital mortality for hypertensive ischemic or hemorrhagic stroke patients in the ICU: a multi-center retrospective cohort study with internal and external cross-validation. Front Neurol. (2023) 14:85447. doi: 10.3389/fneur.2023.1185447
3. Peng, S, Liu, X, Cao, W, Liu, Y, Liu, Y, Wang, W, et al. Global, regional, and national time trends in mortality for stroke, 1990–2019: an age-period-cohort analysis for the global burden of disease 2019 study and implications for stroke prevention. Int J Cardiol. (2023) 383:117–31. doi: 10.1016/j.ijcard.2023.05.001
4. Virani, SS, Alonso, A, Benjamin, EJ, Bittencourt, MS, Callaway, CW, Carson, AP, et al. Heart disease and stroke Statistics-2020 update: a report from the American Heart Association. Circulation. (2020) 141:e139–596. doi: 10.1161/cir.0000000000000757
5. Mei, S, Shao, Y, Fang, Y, Lu, J, Zheng, J, Xu, S, et al. The changes of leukocytes in brain and blood after intracerebral hemorrhage. Front Immunol. (2021) 12:617163. doi: 10.3389/fimmu.2021.617163
6. Morotti, A, Phuah, CL, Anderson, CD, Jessel, MJ, Schwab, K, Ayres, AM, et al. Leukocyte count and intracerebral hemorrhage expansion. Stroke. (2016) 47:1473–8. doi: 10.1161/strokeaha.116.013176
7. Walsh, KB, Sekar, P, Langefeld, CD, Moomaw, CJ, Elkind, MS, Boehme, AK, et al. Monocyte count and 30-day case fatality in intracerebral hemorrhage. Stroke. (2015) 46:2302–4. doi: 10.1161/strokeaha.115.009880
8. Lattanzi, S, Cagnetti, C, Provinciali, L, and Silvestrini, M. Neutrophil-to-lymphocyte ratio predicts the outcome of acute intracerebral hemorrhage. Stroke. (2016) 47:1654–7. doi: 10.1161/strokeaha.116.013627
9. Ozgen, E, Guzel, M, Akpinar, CK, Yucel, M, Demir, MT, and Baydin, A. The relationship between neutrophil/lymphocyte, monocyte/ /lymphocyte, platelet/lymphocyte ratios and clinical outcomes after ninety days in patients who were diagnosed as having acute ischemic stroke in the emergency room and underwent a mechanical thro. Bratisl Lek Listy. (2020) 121:634–9. doi: 10.4149/bll_2020_102
10. Gong, P, Liu, Y, Gong, Y, Chen, G, Zhang, X, Wang, S, et al. The association of neutrophil to lymphocyte ratio, platelet to lymphocyte ratio, and lymphocyte to monocyte ratio with post-thrombolysis early neurological outcomes in patients with acute ischemic stroke. J Neuroinflammation. (2021) 18:51. doi: 10.1186/s12974-021-02090-6
11. Liu, Z, Xv, Y, Liu, X, and Zhou, X. Associations of systemic inflammatory markers with the risks of chronic heart failure: a case-control study. Clinics. (2022) 77:100056. doi: 10.1016/j.clinsp.2022.100056
12. Geng, Y, Zhu, D, Wu, C, Wu, J, Wang, Q, Li, R, et al. A novel systemic inflammation response index (SIRI) for predicting postoperative survival of patients with esophageal squamous cell carcinoma. Int Immunopharmacol. (2018) 65:503–10. doi: 10.1016/j.intimp.2018.10.002
13. Xie, QK, Chen, P, Hu, WM, Sun, P, He, WZ, Jiang, C, et al. The systemic immune-inflammation index is an independent predictor of survival for metastatic colorectal cancer and its association with the lymphocytic response to the tumor. J Transl Med. (2018) 16:273. doi: 10.1186/s12967-018-1638-9
14. Topkan, E, Selek, U, Kucuk, A, Haksoyler, V, Ozdemir, Y, Sezen, D, et al. Prechemoradiotherapy systemic inflammation response index stratifies stage IIIB/C non-small-cell lung cancer patients into three prognostic groups: a propensity score-matching analysis. J Oncol. (2021) 2021:1–9. doi: 10.1155/2021/6688138
15. Trifan, G, and Testai, FD. Systemic immune-inflammation (SII) index predicts poor outcome after spontaneous supratentorial intracerebral hemorrhage. J Stroke Cerebrovasc Dis. (2020) 29:105057. doi: 10.1016/j.jstrokecerebrovasdis.2020.105057
16. Geraghty, JR, Lung, TJ, Hirsch, Y, Katz, EA, Cheng, T, Saini, NS, et al. Systemic immune-inflammation index predicts delayed cerebral vasospasm after aneurysmal subarachnoid hemorrhage. Neurosurgery. (2021) 89:1071–9. doi: 10.1093/neuros/nyab354
17. Chen, Y, Nie, Y, Wu, J, Li, C, Zheng, L, Zhu, B, et al. Association between systemic inflammatory indicators with the survival of chronic kidney disease: a prospective study based on NHANES. Front Immunol. (2024) 15:365591. doi: 10.3389/fimmu.2024.1365591
18. Xie, R, Liu, X, Wu, H, Liu, M, and Zhang, Y. Associations between systemic immune-inflammation index and abdominal aortic calcification: results of a nationwide survey. Nutr Metab Cardiovasc Dis. (2023) 33:1437–43. doi: 10.1016/j.numecd.2023.04.015
19. Johnson, AEW, Bulgarelli, L, Shen, L, Gayles, A, Shammout, A, Horng, S, et al. MIMIC-IV, a freely accessible electronic health record dataset. Sci Data. (2023) 10:1. doi: 10.1038/s41597-022-01899-x
20. Kim, IS, Hwang, CW, Yang, WS, and Kim, CH. Current perspectives on the physiological activities of fermented soybean-derived Cheonggukjang. Int J Mol Sci. (2021) 22:115746. doi: 10.3390/ijms22115746
21. Keep, RF, Hua, Y, and Xi, G. Intracerebral haemorrhage: mechanisms of injury and therapeutic targets. Lancet Neurol. (2012) 11:720–31. doi: 10.1016/s1474-4422(12)70104-7
22. Wang, J. Preclinical and clinical research on inflammation after intracerebral hemorrhage. Prog Neurobiol. (2010) 92:463–77. doi: 10.1016/j.pneurobio.2010.08.001
23. Kim, Y, Han, MH, Kim, CH, Kim, JM, Cheong, JH, and Ryu, JI. Increased short-term mortality in patients with spontaneous intracerebral hemorrhage and its association with admission glucose levels and leukocytosis. World Neurosurg. (2017) 98:503–11. doi: 10.1016/j.wneu.2016.11.087
24. Li, L, Zhang, H, and Feng, GL. Neutrophil-to-lymphocyte ratio predicts in-hospital mortality in intracerebral hemorrhage. J Stroke Cerebrovasc Dis. (2022) 31:106611. doi: 10.1016/j.jstrokecerebrovasdis.2022.106611
25. Kratofil, RM, Kubes, P, and Deniset, JF. Monocyte conversion during inflammation and injury. Arterioscler Thromb Vasc Biol. (2017) 37:35–42. doi: 10.1161/atvbaha.116.308198
26. Giede-Jeppe, A, Bobinger, T, Gerner, ST, Sembill, JA, Sprügel, MI, Beuscher, VD, et al. Neutrophil-to-lymphocyte ratio is an independent predictor for in-hospital mortality in spontaneous intracerebral hemorrhage. Cerebrovasc Dis. (2017) 44:26–34. doi: 10.1159/000468996
27. Liesz, A, Rüger, H, Purrucker, J, Zorn, M, Dalpke, A, Möhlenbruch, M, et al. Stress mediators and immune dysfunction in patients with acute cerebrovascular diseases. PLoS One. (2013) 8:e74839. doi: 10.1371/journal.pone.0074839
28. Mrochen, A, Sprügel, MI, Gerner, ST, Sembill, JA, Lang, S, Lücking, H, et al. Thrombocytopenia and clinical outcomes in intracerebral hemorrhage: a retrospective multicenter cohort study. Stroke. (2021) 52:611–9. doi: 10.1161/strokeaha.120.031478
29. Naidech, AM, Bernstein, RA, Levasseur, K, Bassin, SL, Bendok, BR, Batjer, HH, et al. Platelet activity and outcome after intracerebral hemorrhage. Ann Neurol. (2009) 65:352–6. doi: 10.1002/ana.21618
30. Chen, Y, Lin, YX, Pang, Y, Zhang, JH, Gu, JJ, Zhang, GQ, et al. Systemic inflammatory response index improves the prediction of postoperative pneumonia following meningioma resection. Chin Med J. (2020) 134:728–30. doi: 10.1097/cm9.0000000000001298
31. Zhang, J, Shi, K, Li, Z, Li, M, Han, Y, Wang, L, et al. Organ-and cell-specific immune responses are associated with the outcomes of intracerebral hemorrhage. FASEB J. (2018) 32:220–9. doi: 10.1096/fj.201700324R
32. Wang, J, Du, Y, Wang, A, Zhang, X, Bian, L, Lu, J, et al. Systemic inflammation and immune index predicting outcomes in patients with intracerebral hemorrhage. Neurol Sci. (2023) 44:2443–53. doi: 10.1007/s10072-023-06632-z
33. Li, Y, Wen, D, Cui, W, Chen, Y, Zhang, F, Yuan, M, et al. The prognostic value of the acute phase systemic immune-inflammation index in patients with intracerebral hemorrhage. Front Neurol. (2021) 12:628557. doi: 10.3389/fneur.2021.628557
34. Wang, Y, Xiao, Y, Xing, Y, Yu, M, Wang, X, Ren, J, et al. Morphometric similarity differences in drug-naive Parkinson’s disease correlate with transcriptomic signatures. CNS Neurosci Ther. (2024) 30:e14680. doi: 10.1111/cns.14680
35. Geng, YJ, Smolensky, MH, Sum-Ping, O, Hermida, R, and Castriotta, RJ. Circadian rhythms of risk factors and management in atherosclerotic and hypertensive vascular disease: modern chronobiological perspectives of an ancient disease. Chronobiol Int. (2023) 40:33–62. doi: 10.1080/07420528.2022.2080557
36. Law, RW, and Sbarra, DA. The effects of church attendance and marital status on the longitudinal trajectories of depressed mood among older adults. J Aging Health. (2009) 21:803–23. doi: 10.1177/0898264309338300
37. Li, K, Ma, X, Yuan, L, and Ma, J. Age differences in the association between marital status and hypertension: a population-based study. J Hum Hypertens. (2022) 36:670–80. doi: 10.1038/s41371-021-00558-9
38. LaPierre, TA. The enduring effects of marital status on subsequent depressive symptoms among women: investigating the roles of psychological, social and financial resources. J Epidemiol Community Health. (2012) 66:1056–62. doi: 10.1136/jech-2011-200383
39. Lavelle, B, and Smock, PJ. Divorce and women’s risk of health insurance loss. J Health Soc Behav. (2012) 53:413–31. doi: 10.1177/0022146512465758
40. Dupre, ME, George, LK, Liu, G, and Peterson, ED. Association between divorce and risks for acute myocardial infarction. Circ Cardiovasc Qual Outcomes. (2015) 8:244–51. doi: 10.1161/circoutcomes.114.001291
41. Hughes, ME, and Waite, LJ. Marital biography and health at mid-life. J Health Soc Behav. (2009) 50:344–58. doi: 10.1177/002214650905000307
42. Ryoo, SM, Lee, J, Lee, YS, Lee, JH, Lim, KS, Huh, JW, et al. Lactate level versus lactate clearance for predicting mortality in patients with septic shock defined by Sepsis-3. Crit Care Med. (2018) 46:e489–95. doi: 10.1097/ccm.0000000000003030
43. Raux, M, Le Manach, Y, Gauss, T, Baumgarten, R, Hamada, S, Harrois, A, et al. Comparison of the prognostic significance of initial blood lactate and base deficit in trauma patients. Anesthesiology. (2017) 126:522–33. doi: 10.1097/aln.0000000000001490
44. Johnson, MC, Alarhayem, A, Convertino, V, Carter, R, Chung, K, Stewart, R, et al. Comparison of compensatory reserve and arterial lactate as markers of shock and resuscitation. J Trauma Acute Care Surg. (2017) 83:603–8. doi: 10.1097/ta.0000000000001595
45. Simpson, IA, Carruthers, A, and Vannucci, SJ. Supply and demand in cerebral energy metabolism: the role of nutrient transporters. J Cereb Blood Flow Metab. (2007) 27:1766–91. doi: 10.1038/sj.jcbfm.9600521
46. Chen, T, Qian, YZ, Di, X, Zhu, JP, and Bullock, R. Evidence for lactate uptake after rat fluid percussion brain injury. Acta Neurochir Suppl. (2000) 75:76359. doi: 10.1007/978-3-7091-6346-7_75
47. Luo, HC, Fu, YQ, You, CY, Liu, CJ, and Xu, F. Comparison of admission serum albumin and hemoglobin as predictors of outcome in children with moderate to severe traumatic brain injury: a retrospective study. Medicine. (2019) 98:e17806. doi: 10.1097/md.0000000000017806
48. Montalcini, T, Moraca, M, Ferro, Y, Romeo, S, Serra, S, Raso, MG, et al. Nutritional parameters predicting pressure ulcers and short-term mortality in patients with minimal conscious state as a result of traumatic and non-traumatic acquired brain injury. J Transl Med. (2015) 13:305. doi: 10.1186/s12967-015-0660-4
Keywords: ICH, NLR, PLR, LMR, SII, SIRI, ICU mortality
Citation: Zhao G, Gu Y, Wang Z, Chen Y and Xia X (2024) The clinical value of inflammation index in predicting ICU mortality of critically ill patients with intracerebral hemorrhage. Front. Public Health. 12:1373585. doi: 10.3389/fpubh.2024.1373585
Edited by:
Wenle Li, Xiamen University, ChinaReviewed by:
Sanjeeb Sudarshan Bhandari, UPMC Western Maryland Medical Center, United StatesEylem Acartürk Tuncay, Health Sciences University Süreyyapaşa Chest Diseases and Thoracic Surgery Training and Research Hospital, Türkiye
Xiaozhu Liu, Capital Medical University, China
Hao Chi, Southwest Medical University, China
Zhendong Ding, Third Affiliated Hospital of Sun Yat-sen University, China
Yi Chen, Xinjiang Medical University, China
Copyright © 2024 Zhao, Gu, Wang, Chen and Xia. This is an open-access article distributed under the terms of the Creative Commons Attribution License (CC BY). The use, distribution or reproduction in other forums is permitted, provided the original author(s) and the copyright owner(s) are credited and that the original publication in this journal is cited, in accordance with accepted academic practice. No use, distribution or reproduction is permitted which does not comply with these terms.
*Correspondence: Guang Zhao, emhhb3ppeWl4QDEyNi5jb20=
†These authors have contributed equally to this work and share first authorship