- 1Cardiology Department, The First Affiliated Hospital of Kunming Medical University, Kunming, China
- 2Institute of Medical Sociology, Centre for Health and Society, Faculty of Medicine, Heinrich Heine University Düsseldorf, Düsseldorf, Germany
- 3Departments of Environmental Health Sciences and Epidemiology, Fielding School of Public Health, School of Nursing, University of California, Los Angeles, Los Angeles, CA, United States
Background: Work stress is considered as a risk factor for coronary heart disease, but its link with heart rate variability (HRV) among heart attack survivors is unknown yet. The aim of this study was to investigate associations between baseline work stress and the changes of HRV over one-year after onset of acute coronary syndrome (ACS).
Methods: Hundred and twenty-two patients with regular paid work before their first ACS episode were recruited into this hospital-based longitudinal cohort study. During hospitalization (baseline), all patients underwent assessments of work stress by job strain (JS) and effort-reward imbalance (ERI) models, and were assigned into low or high groups; simultaneously, sociodemographic and clinical data, as well depression, anxiety, and job burnout, were collected. Patients were followed up 1, 6, and 12 months after discharge, with HRV measurements at baseline and each follow-up point. Generalized estimating equations were used to analyze the effects of baseline work stress on HRV over the following 1 year.
Results: After adjusting for baseline characteristics and clinical data, anxiety, depression, and burnout scores, high JS was not associated with any HRV measures during follow-up (all p > 0.10), whereas high ERI was significantly related to slower recovery of 5 frequency domain HRV measures (TP, HF, LF, VLF, and ULF) (all p < 0.001), and marginally associated with one time domain measure (SDNN) (p = 0.069). When mutually adjusting for both work stress models, results of ERI remained nearly unchanged.
Conclusion: Work stress in terms of ERI predicted lower HRV during the one-year period after ACS, especially frequency domain measures.
1 Introduction
Work stress has been identified as a risk factor for coronary heart disease (CHD). Several studies have revealed that work stress was associated with an increased risk of CHD by 20% (1–3), where work stress was widely defined by two theoretical models, the job strain (JS) model and the effort-reward imbalance (ERI) model. The former model focuses on task characteristics of combination of high job demand and low job control (4); while nonreciprocal social exchange, i.e., high cost and low gain at work, is emphasized in the latter model (5). Though several biological pathways have been proposed to explain the mechanisms between work stress and CHD (6, 7), heart rate variability (HRV) has received special attention given its powerful clinical prediction to heart attack (8). HRV refers to the variation in time interval between successive heartbeats, reflecting the balance between sympathetic and parasympathetic activity which is a measure of the autonomic nervous system’s influence on the heart (9). Recent studies have revealed that a decrease in HRV or a blunted increase in HRV is associated with both acute and chronic stress, suggesting that HRV can function as a marker of stress (10, 11). In the field of research on work stress and HRV, studies have shown that work stress in terms of both JS and ERI was associated with lower levels of HRV among apparently healthy workers (12, 13).
Clinical management of CHD has experienced significant progress over the past two decades, leading to substantial improvement in patient survival rates post-CHD (14). Despite these advances, approximately one-third of CHD patients still face a considerable risk, estimated to be between 20 and 30%, of experiencing recurrent cardiovascular events during the subsequent 10 years (15). Interestingly, a handful epidemiological studies indicated that, among workers with CHD, the risk of subsequent cardiac events was increased by 65% when exposed to JS or ERI, as suggested by our previous meta-analysis (16, 17). Regarded as an important prognostic marker in various cardiovascular diseases (CVD), HRV can predict increased risk of adverse outcomes (18). Especially in patients after myocardial infarction (MI), decreased HRV has been associated with an increased risk of arrhythmia events and elevated mortality rate (18, 19), suggesting that monitoring HRV may help identify those at greater risk of future cardiac events following MI. Hypothetically, HRV might also play an important role in explaining the bio-medical pathway from work stress to recurrent cardiac events. Yet, to the best of our knowledge, the link between work stress and HRV among employees who survived from heart attack was not explored.
Therefore, with an attempt to provide scientific evidence by exploring the potential role of HRV in explaining work stress and recurrent CHD, we carried out a longitudinal study among patients with first acute coronary syndrome (ACS), which is a severe condition of CHD, and aimed to investigate associations of work stress, being measured by both JS and ERI, with HRV over a 12-month course of post-ACS period. In addition, we compared the explanatory power of JS and ERI to predict changes in HRV.
2 Methods
2.1 Study subjects
Patients admitted to the First Affiliated Hospital of Kunming Medical University between March 2018 and December 2019 participated in this study. They had experienced their first ACS episode and previously maintained regular paid employment prior to the ACS event. ACS covers various acute myocardial ischemic states including ST-segment elevation myocardial infarction (STEMI), non-ST segment elevation myocardial infarction (NSTEMI) and unstable angina (UA) (20, 21). Exclusion criteria included arrhythmia frequency disrupting the investigation of HRV, potential comorbidities influencing symptom onset, and severe systemic disease. Patients with a history of antipsychotic drug use, those were incapable of accomplishing questionnaires even with assistance, and individuals who refused or were unable to undergo a 24-h Holter ECG monitoring were also excluded from the study. Coronary angiography was performed for every included patient during the hospital stay period. Figure 1 is a flowchart showing that 122 patients were ultimately recruited into this study.
2.2 Study design
In this hospital-based longitudinal cohort study, patients’ work stress levels were evaluated during their hospital stay, which served as the study baseline. Participants were subsequently designated into either low or high work stress groups (see below for details). Additionally, sociodemographic and clinical data, as well as anxiety, depression, and job burnout levels, were collected at baseline. Follow-ups were conducted 1, 6, and 12 months post-discharge, with HRV parameters measured at baseline and at each follow-up for a total of four times. The Ethics Committee of Kunming Medical University (Kunming, China) issued an approval for the study, which was carried out in accordance with the Declaration of Helsinki guidelines. The informed consent form was signed by all subjects.
2.3 Demographic and medical data collection
Clinical and demographic data were extracted from patients’ medical records. This information included age, sex, education level, number of family members, monthly family income, type of ACS experienced (UA, STEMI, NSTEMI), Killip’s grade at admission, prior medical history such as hypertension, diabetes, hyperlipemia, and stroke, current health-related behaviors including smoking and heavy drinking, along with family medical history with regards to CVD and medication usage.
2.4 Work stress evaluation
The Job Content Questionnaire (JCQ) and the ERI Questionnaire corresponding to the JS and ERI models, respectively, were used to evaluate work stress. The JCQ, developed by Karasek (4), has been demonstrated to be reliable and valid in its Chinese version (22). For the present study, a shortened version of the JCQ was utilized, highlighting job demand and control with four questions (two items for demand and two items for control), which was highly consistent with the original (23). The ERI Questionnaire, developed by Siegrist (5), also has a reliable and valid Chinese version (24). In this study, we used a simplified ERI Questionnaire consisting of nine questions concerning effort and reward (three items for effort and six items for reward), which was in parallel with the original version (25). Then, two groups were dichotomized based on the JS and ERI results, i.e., low (ratio ≤ 1) and high (ratio > 1). In the present study, Cronbach’s α coefficients were 0.77 for job demand, 0.75 for job control, 0.77 for effort, and 0.79 for reward.
2.5 Anxiety, depression, and job burnout assessment
As previously recognized, anxiety and depression are important risk factors that impact CHD prognosis (26, 27). Job burnout is another significant factor associated with poor prognosis in ACS according to our past study (28–30). These three factors were assessed before discharge as confounding factors in this study. Anxiety and depression were evaluated with the 14-item Hospital Anxiety and Depression Scale (HADS) with HADS-anxiety and HADS-depression subscales. Both subscales were scored 0–21 points, where a higher score suggested more severe anxiety and depression symptoms (31). This scale has successfully applied in Chinese CHD patients (32). The total HADS score, which is the sum of the anxiety and depression scores (33), was used to adjust for the impact of anxiety and depression on HRV in subsequent statistical analysis. Job burnout was measured with the job burnout subscale of the Copenhagen Burnout Inventory (CBI). With a range of 0–100 points, a high CBI score represented more severe job burnout (34). The Chinese version of CBI has been shown to be reliable and valid by other studies (35). In the current study, Cronbach’s alpha coefficients were 0.71 for HADS-anxiety, 0.73 for HADS-depression, and 0.72 for the CBI job burnout subscale, respectively.
2.6 HRV measurement
HRV was measured at four time points: 1 day prior to discharge, and 1, 6, and 12 months post-discharge. Eligible subjects underwent 24-h Holter monitoring between 08:00 and 09:00 AM, with continuous electrocardiogram (ECG) signals recorded using a 12-channel Holter system (Biomedical Systems, USA). The hardware of the system sampled at a rate of 500 Hz. The recorded analog ECG signals were digitized and analyzed using the BMS Century 3,000 software package for HRV analysis (version 2.0). Before calculating the HRV parameters, the raw RR interval data were processed to remove artifacts. Two specific criteria were applied to identify artifacts within the RR interval data: An RR interval was flagged as an artifact if it was outside the 300–2000 ms range, or if the ratio of a consecutive RR interval to its predecessor was not within the 0.8–1.2 range. If artifacts represented less than 5% of the total RR intervals, and there were no extended sequences of consecutive artifacts, the HRV recording was deemed to meet quality standards; otherwise, a new recording was required. For HRV recordings that passed the quality assessment, artifacts were removed, and linear interpolation was employed to estimate the values of missing RR intervals (9). HRV parameters were calculated with ECG data over the entire 24-h period. The standard deviation for all normal-to-normal RR intervals (SDNN) and the root mean square of successive differences (RMSSD) were derived as the common time-domain parameters for HRV. Fast Fourier transform was utilized for transformation of the heart rate power spectrum from a 24-h normal RR interval, yielding five frequency-domain parameters covering high frequency power (HF = 0.15–0.40 Hz), low frequency power (LF = 0.04–0.15 Hz), very low frequency power (VLF = 0.0033–0.04 Hz), ultra low frequency power (ULF < 0.0033 Hz), and total power (TP ≤ 0.40 Hz). To accommodate the skewed distribution, the natural logarithms of seven HRV parameters obtained by conversion were as follows: ln(SDNN), ln(RMSSD), ln(TP), ln(HF), ln(LF), ln(VLF), and ln(ULF), which were in line with normal distribution. All HRV data collection and analysis were performed according to the guidelines for reporting articles on psychiatry and heart rate variability (GRAPH) (36).
2.7 Statistical analysis
All data were processed and analyzed using Stata 10 (Stata, College Station, TX, USA). Mean ± standard deviation (SD), number and percentage (%) were used to express continuous and categorical variables, respectively. First, the t-test or χ2 test was conducted to compare baseline characteristics between high and low work stress groups. Second, repeated measures analysis of variance (ANOVA) was applied to study the variations of HRV measures over a 12-month period. Third, generalized estimating equations (GEEs), a statistical method for dealing with repeated measurement data (37, 38), were employed to examine the longitudinal associations of JS or ERI models at the onset with shifts in HRV measures at different intervals (baseline, together with 1, 6, and 12 months). GEE regression was modeled via four steps. Demographic and clinical variables from the baseline were incorporated into Model I. The HADS sum score was also included in Model II, which accounts for depression and anxiety. Model III built upon Model II with further adjustment for job burnout. Notably, we looked at the effects of JS or ERI on HRV individually, without mutual adjustment from Model I to III. Ultimately, we estimated the link between JS, ERI, and HRV in Model IV by adjusting each work stress model for the others. Such mutual adjustment aimed to assess how each work stress model independently affected the longitudinal changes in HRV (39).
3 Results
3.1 Characteristics of subjects at baseline
As is indicated in Figure 1, a total of 122 patients (aged between 27 and 62 years with a median age of 49.5 years; 103 males and 19 females) participated in the study. No cardiac events such as cardiac death, heart failure, recurrent ACS events, and rehospitalization due to ACS-related symptoms were observed during the follow-up period. The characteristics of subjects at baseline are presented in Table 1. There were significant differences in job burnout score and HADS score between the high JS group and the low JS group. Between the high ERI group and the low ERI group, there were statistical differences in ACS type and job burnout score. Other characteristics did not show significant differences between the high/low JS groups, or the high/low ERI groups.
3.2 The HRV measures over 12 months after ACS
Table 2 presents the natural logarithmized HRV measures over 12 months after ACS. Two time domain measures (SDNN, RMSSD) and 5 frequency domain measures (LF, HF, VLF, ULF, and TP) gradually increased within 12 months, which was consistent with the variation pattern of HRV during ACS recovery process. The raw HRV measures can be seen in Supplementary Table S1.
3.3 Associations of work stress at baseline with changes in HRV during 12 months after ACS
After adjusting for demographic and clinical data, HADS score, and burnout score (Model III), work stress measured by JS was not associated with any HRV measures during the follow-up period (all p > 0.10); while high ERI was significantly related to slow recovery of 5 frequency measures of HRV (TP, HF, LF, VLF, and ULF) (all p < 0.001) and marginally associated with reduced one time domain of HRV (SDNN) (p = 0.069). The mutual adjustment for JS and ERI did not change the results (see Model IV). Figure 2 intuitively reflected the trend of HRV changes between the ERI high and low groups, which is consistent with Table 3.
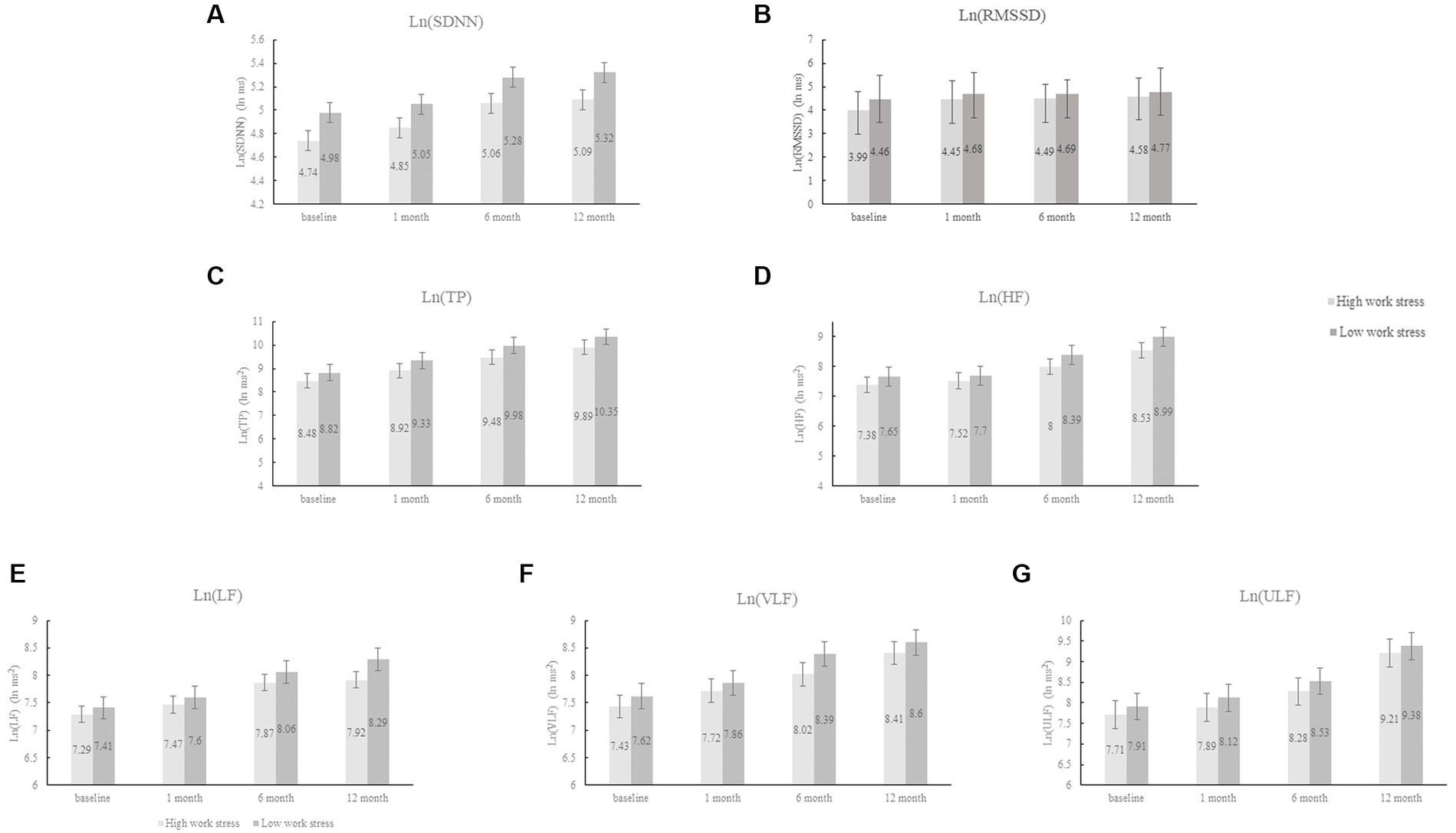
Figure 2. The changes in HRV between the ERI high and low groups. HRV, heart rate variability; ERI, effort-reward imbalance; SDNN, standard deviation of NNintervals; RMSSD, root mean square of successive differences; TP, total power; HF, high frequency; LF, low frequency; VLF, very low frequency; ULF, ultra-low frequency; Ln, natural logarithm.
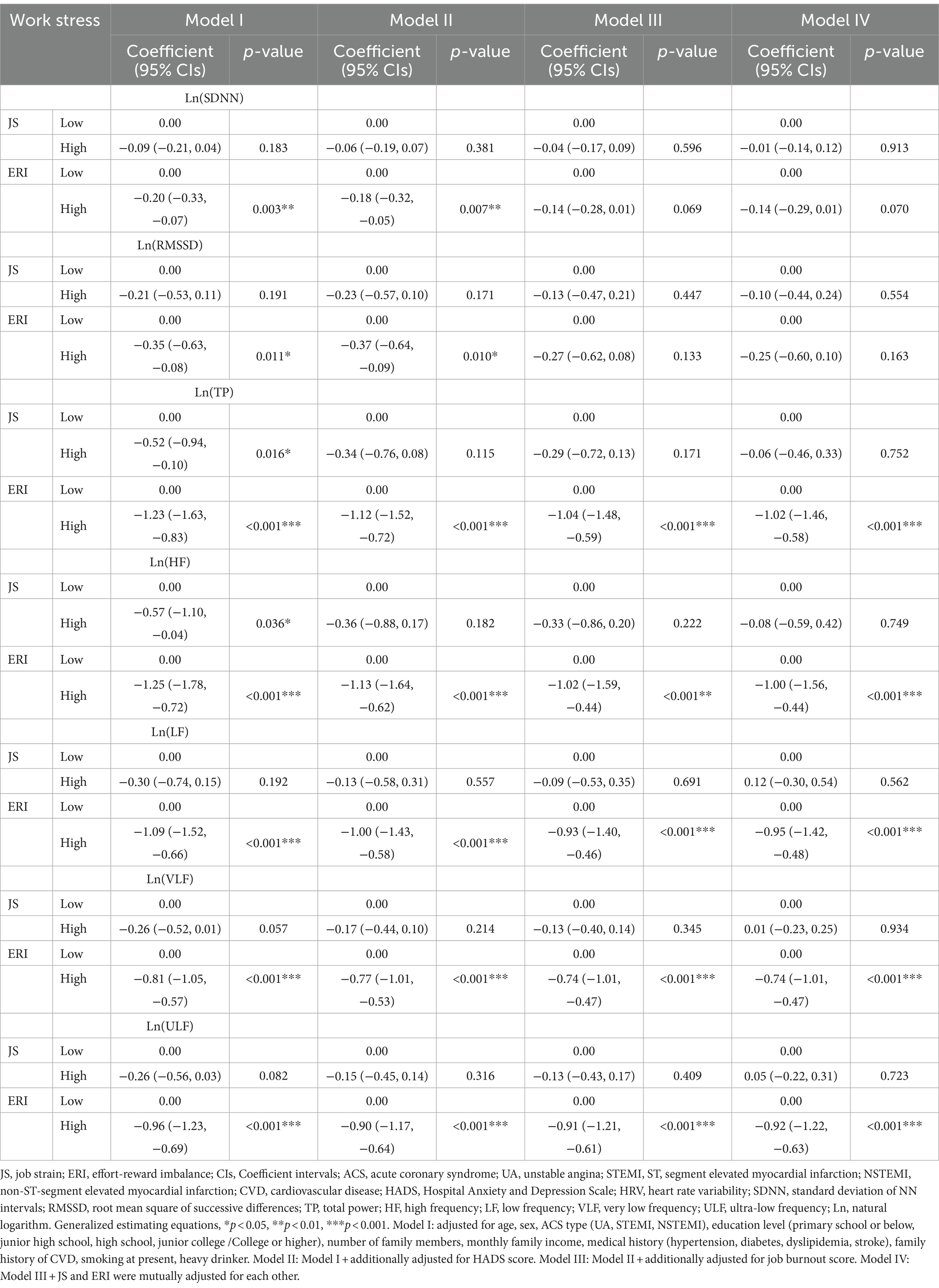
Table 3. The coefficients and 95% CIs of repeated measures of HRV parameters during 1-year follow-up by work stress at baseline according to JS and ERI models.
4 Discussion
This study provides new research evidence about a potential bio-medical pathway explaining the observed association of work stress with increased risk of recurrent CHD among employees (16, 17). An imbalance between high effort and low reward at work, assessed at baseline predicted lower HRV 1 year after ACS. For the alternative work stress model (i.e., JS) this association was not observed. To the best of our knowledge, this is the first study to reveal longitudinal associations between work stress and HRV changes in ACS patients, and it is also the first study to compare two work stress models in this regard.
HRV has been well recognized in determining prognosis of post myocardial infarction, and as an established clinical marker of sudden cardiac death. Back to 1987, a study showed that the mortality in post-MI patients with SDNN less than 50 ms was 5.3 times higher than those with SDNN more than 100 ms (40). And a meta-analysis in 2009 demonstrated that post-MI patients with SDNN below 70 ms had a 4-fold risk of death in the next 3 years (41). Regarding the frequency domain HRV measures, recent research suggested that decreased frequency power would elevate risk of adverse clinical outcomes among cardiac patients (42, 43). In particular, studies have shown that the reductions of VLF and ULF were associated with an increased risk of all-cause death and cardiac death (44–47). Our study revealed significant associations between higher work stress at baseline and slower recovery of HRV during one-year follow-up period. In this context, it seems plausible to assume an explanatory contribution of stressful work to the association of altered HRV with poor CHD prognostic outcomes.
HRV is also recognized as a biological indicator for work-related stress (48). For instance, HRV can serve as a metric for evaluating psychological stress experienced by emergency physicians during their duties (49). Several studies documented associations of high work stress with reduced HRV (50–52). For instance, concerning the JS model, four studies showed reduced HRV (53–56), whereas two more investigations did not support this notion (57, 58). With regard to the ERI model, a couple of investigations supported a link with reduced HRV (50–52, 57, 59–61). These studies were conducted among healthy workers in various trades and professions. Yet, no study on work stress and HRV was reported in post-MI patients so far.
It is of interest to observe that work stress in terms of ERI, but not of JS, was predictive in the current study. A similar finding was apparent in two earlier investigations of Chinese participants (39, 62). One explanation points to the relevant role of status-related and financial rewards emphasized in the former model, that may have received a prominent role in the context of rapid and far-reaching changes of working and employment conditions in China (63, 64). Clearly, additional research is required to support this notion, and at a more general level, cultural differences in the comparability of findings relating these work stress models with health outcomes between Western societies and societies with an Asian sociocultural background need to be critically appraised (65).
This study has several strengths. First, this was the first longitudinal study to examine the associations of work stress with HRV in patients after the first ACS. As a particular advantage of this study design, HRV was assessed four times within 1 year. Second, both independent and dependent variables in our study were measured with standardized instruments, with established psychometric quality criteria. Third, several further psychosocial factors (including anxiety, depression, and job burnout) were considered as potential confounders of the reported associations, given their documented associations with CHD prognosis (26, 27, 66).
A number of limitations need to be addressed. First, the proportion of female participants was relatively low, accounting for only 15.6%. Since the subjects included in this study were all at a working age, were relatively young, and were suffering from ACS, fewer women met these inclusion criteria. Second, work stress was assessed at baseline only, and potential changes of work stress and employment status after ACS were not documented. As a result, we are not able to explore the interplay between work stress and HRV over time. Third, we did not collect data on the types of occupations of our subjects. Therefore, the role of occupation in explaining associations between work stress and HRV is not able to be carefully examined. Fourth, unmeasured factors may have affected our HRV measurements, such as the participant’s physical condition, exercise regime, sleep, and breathing, thus potentially limiting the significance of reported findings. The results of this study have clinical implications for secondary prevention of coronary heart disease, particularly in view of the fact that the occurrence of this disease among younger age working populations was growing in recent past (67). To date, specific tailored measures of improving the quality of working and employment conditions among people with CHD returning to work are warranted. These measures include organizational changes instructed by the work stress models mentioned, suggesting the reduction of workload and the strengthening of control and reward at work (68). In addition, programs of medical rehabilitation among cardiac patients are now available that improve health outcomes, such as evidence-based cognitive behavioral therapy and stress management (69).
5 Conclusion
Our study found that work stress, as characterized by ERI, predicts a reduction in HRV over a one-year follow-up period after ACS onset. This underscores the significant potential role of work stress in the secondary prevention of ACS, as well as other types of CVD. Despite these promising results, it’s crucial to note that further studies are needed to confirm these findings and explore alternative mechanisms. Importantly, our study adds a valuable piece of evidence to understand the role of work stress in CVD secondary prevention, opening up new potential avenues for intervention, such as workplace-oriented and multidisciplinary programs (including cognitive behavioral therapy and coaching) which might be effective to job retention and disease management (17).
Data availability statement
The original contributions presented in the study are included in the article/Supplementary material, further inquiries can be directed to the corresponding authors.
Ethics statement
The studies involving humans were approved by The Ethics Committee of Kunming Medical University (Kunming, China). The studies were conducted in accordance with the local legislation and institutional requirements. The participants provided their written informed consent to participate in this study.
Author contributions
ZH: Data curation, Funding acquisition, Investigation, Writing – original draft. XC: Investigation, Writing – original draft. PJ: Data curation, Investigation, Writing – original draft. BZ: Data curation, Investigation, Writing – original draft. YS: Data curation, Formal analysis, Validation, Writing – original draft. JS: Writing – review & editing. JL: Conceptualization, Methodology, Writing – review & editing. MZ: Conceptualization, Funding acquisition, Methodology, Project administration, Supervision, Writing – review & editing.
Funding
The author(s) declare that financial support was received for the research, authorship, and/or publication of this article. This work was supported by research grants of the Yunnan Health Training Project of High Level Talents (No. L-2018014 and No. H-2019052), People’s Republic of China. JL was partially supported by the Targeted Research Training Program and Epidemiology Program of the Southern California NIOSH Education and Research Center (SCERC), Grant Agreement No. T42 OH008412 from the Centers for U.S. Disease Control and Prevention (CDC). Its contents are solely the responsibility of the authors and do not necessarily represent the official view of the U.S. CDC.
Acknowledgments
The authors thank the study participants.
Conflict of interest
The authors declare that the research was conducted in the absence of any commercial or financial relationships that could be construed as a potential conflict of interest.
Publisher’s note
All claims expressed in this article are solely those of the authors and do not necessarily represent those of their affiliated organizations, or those of the publisher, the editors and the reviewers. Any product that may be evaluated in this article, or claim that may be made by its manufacturer, is not guaranteed or endorsed by the publisher.
Supplementary material
The Supplementary material for this article can be found online at: https://www.frontiersin.org/articles/10.3389/fpubh.2024.1336065/full#supplementary-material
References
1. Kivimaki, M, Nyberg, ST, Batty, GD, Fransson, EI, Heikkila, K, Alfredsson, L, et al. Job strain as a risk factor for coronary heart disease: a collaborative meta-analysis of individual participant data. Lancet. (2012) 380:1491–7. doi: 10.1016/S0140-6736(12)60994-5
2. Dragano, N, Siegrist, J, Nyberg, ST, Lunau, T, Fransson, EI, Alfredsson, L, et al. Effort-reward imbalance at work and incident coronary heart disease: a multicohort study of 90,164 individuals. Epidemiology. (2017) 28:619–26. doi: 10.1097/EDE.0000000000000666
3. Siegrist, J, and Li, J. Effort-reward imbalance and occupational health In: T Theorell, editor. Socioeconomic determinants of occupational health – From macro-level to Micro-level evidence. Handbook series in occupational health sciences. Cham, Switzerland: Springer (2020).
4. Karasek, R. Job demands, job decision latitude, and mental strain: implications for job redesign. Administrative Science Quarterly. (1979) 24:285–308.
5. Siegrist, J. Adverse health effects of high-effort/low-reward conditions. J Occup Health Psychol. (1996) 1:27–41. doi: 10.1037/1076-8998.1.1.27
6. Chandola, T, Britton, A, Brunner, E, Hemingway, H, Malik, M, Kumari, M, et al. Work stress and coronary heart disease: what are the mechanisms? Eur Heart J. (2008) 29:640–8. doi: 10.1093/eurheartj/ehm584
7. Chandola, T, Heraclides, A, and Kumari, M. Psychophysiological biomarkers of workplace stressors. Neurosci Biobehav Rev. (2010) 35:51–7. doi: 10.1016/j.neubiorev.2009.11.005
8. Jarczok, MN, Weimer, K, Braun, C, Williams, DP, Thayer, JF, Gundel, HO, et al. Heart rate variability in the prediction of mortality: a systematic review and meta-analysis of healthy and patient populations. Neurosci Biobehav Rev. (2022) 143:104907. doi: 10.1016/j.neubiorev.2022.104907
9. Heart rate variability: standards of measurement, physiological interpretation and clinical use. Task Force of the European Society of Cardiology and the north American Society of Pacing and Electrophysiology. Circulation. (1996) 93:1043–65. doi: 10.1161/01.CIR.93.5.1043
10. Thayer, JF, Ahs, F, Fredrikson, M, Sollers, JJ 3rd, and Wager, TD. A meta-analysis of heart rate variability and neuroimaging studies: implications for heart rate variability as a marker of stress and health. Neurosci Biobehav Rev. (2012) 36:747–56. doi: 10.1016/j.neubiorev.2011.11.009
11. Thayer, JF, Mather, M, and Koenig, J. Stress and aging: a neurovisceral integration perspective. Psychophysiology. (2021) 58:e13804. doi: 10.1111/psyp.13804
12. Jarczok, MN, Jarczok, M, Mauss, D, Koenig, J, Li, J, Herr, RM, et al. Autonomic nervous system activity and workplace stressors—a systematic review. Neurosci Biobehav Rev. (2013) 37:1810–23. doi: 10.1016/j.neubiorev.2013.07.004
13. de Looff, PC, Cornet, LJM, Embregts, P, Nijman, HLI, and Didden, HCM. Associations of sympathetic and parasympathetic activity in job stress and burnout: a systematic review. PLoS One. (2018) 13:e0205741. doi: 10.1371/journal.pone.0205741
14. Puymirat, E, Simon, T, Cayla, G, Cottin, Y, Elbaz, M, Coste, P, et al. Acute myocardial infarction: changes in patient characteristics, management, and 6-month outcomes over a period of 20 years in the FAST-MI program (French registry of acute ST-elevation or non-ST-elevation myocardial infarction) 1995 to 2015. Circulation. (2017) 136:1908–19. doi: 10.1161/CIRCULATIONAHA.117.030798
15. Kaasenbrood, L, Boekholdt, SM, van der Graaf, Y, Ray, KK, Peters, RJ, Kastelein, JJ, et al. Distribution of estimated 10-year risk of recurrent vascular events and residual risk in a secondary prevention population. Circulation. (2016) 134:1419–29. doi: 10.1161/CIRCULATIONAHA.116.021314
16. Li, J, Zhang, M, Loerbroks, A, Angerer, P, and Siegrist, J. Work stress and the risk of recurrent coronary heart disease events: a systematic review and meta-analysis. Int J Occup Med Environ Health. (2015) 28:8–19. doi: 10.2478/s13382-014-0303-7
17. Li, J, and Siegrist, J. Occupational risks of recurrent coronary heart disease. J Am Coll Cardiol. (2021) 77:1626–8. doi: 10.1016/j.jacc.2021.02.020
18. Thayer, JF, and Lane, RD. The role of vagal function in the risk for cardiovascular disease and mortality. Biol Psychol. (2007) 74:224–42. doi: 10.1016/j.biopsycho.2005.11.013
19. La Rovere, MT, Pinna, GD, Hohnloser, SH, Marcus, FI, Mortara, A, Nohara, R, et al. Baroreflex sensitivity and heart rate variability in the identification of patients at risk for life-threatening arrhythmias: implications for clinical trials. Circulation. (2001) 103:2072–7. doi: 10.1161/01.CIR.103.16.2072
20. Ibanez, B, James, S, Agewall, S, Antunes, MJ, Bucciarelli-Ducci, C, Bueno, H, et al. 2017 ESC guidelines for the management of acute myocardial infarction in patients presenting with ST-segment elevation: the task force for the management of acute myocardial infarction in patients presenting with ST-segment elevation of the European Society of Cardiology (ESC). Eur Heart J. (2018) 39:119–77. doi: 10.1093/eurheartj/ehx393
21. Roffi, M, Patrono, C, Collet, JP, Mueller, C, Valgimigli, M, Andreotti, F, et al. 2015 ESC guidelines for the management of acute coronary syndromes in patients presenting without persistent ST-segment elevation: task force for the Management of Acute Coronary Syndromes in patients presenting without persistent ST-segment elevation of the European Society of Cardiology (ESC). Eur Heart J. (2016) 37:267–315. doi: 10.1093/eurheartj/ehv320
22. Li, J, Yang, W, Liu, P, Xu, Z, and Cho, SI. Psychometric evaluation of the Chinese (mainland) version of job content questionnaire: a study in university hospitals. Ind Health. (2004) 42:260–7. doi: 10.2486/indhealth.42.260
23. Fransson, EI, Nyberg, ST, Heikkila, K, Alfredsson, L, Bacquer de, D, Batty, GD, et al. Comparison of alternative versions of the job demand-control scales in 17 European cohort studies: the IPD-work consortium. BMC Public Health. (2012) 12:62. doi: 10.1186/1471-2458-12-62
24. Li, J, Loerbroks, A, Shang, L, Wege, N, Wahrendorf, M, and Siegrist, J. Validation of a short measure of effort-reward imbalance in the workplace: evidence from China. J Occup Health. (2012) 54:427–33. doi: 10.1539/joh.12-0106-BR
25. Siegrist, J, Dragano, N, Nyberg, ST, Lunau, T, Alfredsson, L, Erbel, R, et al. Validating abbreviated measures of effort-reward imbalance at work in European cohort studies: the IPD-work consortium. Int Arch Occup Environ Health. (2014) 87:249–56. doi: 10.1007/s00420-013-0855-z
26. Meijer, A, Conradi, HJ, Bos, EH, Anselmino, M, Carney, RM, Denollet, J, et al. Adjusted prognostic association of depression following myocardial infarction with mortality and cardiovascular events: individual patient data meta-analysis. Br J Psychiatry. (2013) 203:90–102. doi: 10.1192/bjp.bp.112.111195
27. Celano, CM, Millstein, RA, Bedoya, CA, Healy, BC, Roest, AM, and Huffman, JC. Association between anxiety and mortality in patients with coronary artery disease: a meta-analysis. Am Heart J. (2015) 170:1105–15. doi: 10.1016/j.ahj.2015.09.013
28. Shi, Y, Jiang, R, Zhu, C, Zhang, M, Cai, H, Hu, Z, et al. High job burnout predicts low heart rate variability in the working population after a first episode of acute coronary syndrome. Int J Environ Res Public Health. (2021) 18:3431. doi: 10.3390/ijerph18073431
29. Shi, Y, Zhu, C, Jiang, R, Zhang, M, Cai, H, Hu, Z, et al. Job burnout is associated with slow improvement of quality of life in the employees after a first episode of acute coronary syndrome: a hospital-based longitudinal study in China. J Psychosom Res. (2021) 152:110690. doi: 10.1016/j.jpsychores.2021.110690
30. Zhang, M, Loerbroks, A, and Li, J. Job burnout predicts decline of health-related quality of life among employees with cardiovascular disease: a one-year follow-up study in female nurses. Gen Hosp Psychiatry. (2018) 50:51–3. doi: 10.1016/j.genhosppsych.2017.10.004
31. Zigmond, AS, and Snaith, RP. The hospital anxiety and depression scale. Acta Psychiatr Scand. (1983) 67:361–70. doi: 10.1111/j.1600-0447.1983.tb09716.x
32. Watson, R. A psychometric evaluation of the Chinese version of the hospital anxiety and depression scale in patients with coronary heart disease. J Clin Nurs. (2009) 18:3068. doi: 10.1111/j.1365-2702.2009.03043.x
33. Bjelland, I, Dahl, AA, Haug, TT, and Neckelmann, D. The validity of the hospital anxiety and depression scale. An updated literature review. J Psychosom Res. (2002) 52:69–77. doi: 10.1016/S0022-3999(01)00296-3
34. Kristensen, TS, Borritz, M, Villadsen, E, and Christensen, KB. The Copenhagen burnout inventory: a new tool for the assessment of burnout. Work Stress. (2005) 19:192–207. doi: 10.1080/02678370500297720
35. Yeh, WY, Cheng, Y, Chen, CJ, Hu, PY, and Kristensen, TS. Psychometric properties of the Chinese version of Copenhagen burnout inventory among employees in two companies in Taiwan. Int J Behav Med. (2007) 14:126–33. doi: 10.1007/BF03000183
36. Quintana, DS, Alvares, GA, and Heathers, JA. Guidelines for reporting articles on psychiatry and heart rate variability (GRAPH): recommendations to advance research communication. Transl Psychiatry. (2016) 6:e803. doi: 10.1038/tp.2016.73
37. Zeger, SL, Liang, KY, and Albert, PS. Models for longitudinal data: a generalized estimating equation approach. Biometrics. (1988) 44:1049–60. doi: 10.2307/2531734
38. Ziegler, A, and Vens, M. Generalized estimating equations. Notes on the choice of the working correlation matrix. Methods Inf Med. (2010) 49:421–5. doi: 10.3414/ME10-01-0026
39. Li, J, Yang, W, and Cho, SI. Gender differences in job strain, effort-reward imbalance, and health functioning among Chinese physicians. Soc Sci Med. (2006) 62:1066–77. doi: 10.1016/j.socscimed.2005.07.011
40. Kleiger, RE, Miller, JP, Bigger, JT Jr, and Moss, AJ. Decreased heart rate variability and its association with increased mortality after acute myocardial infarction. Am J Cardiol. (1987) 59:256–62. doi: 10.1016/0002-9149(87)90795-8
41. Buccelletti, E, Gilardi, E, Scaini, E, Galiuto, L, Persiani, R, Biondi, A, et al. Heart rate variability and myocardial infarction: systematic literature review and metanalysis. Eur Rev Med Pharmacol Sci. (2009) 13:299–307.
42. Huikuri, HV, and Stein, PK. Heart rate variability in risk stratification of cardiac patients. Prog Cardiovasc Dis. (2013) 56:153–9. doi: 10.1016/j.pcad.2013.07.003
43. Wu, L, Jiang, Z, Li, C, and Shu, M. Prediction of heart rate variability on cardiac sudden death in heart failure patients: a systematic review. Int J Cardiol. (2014) 174:857–60. doi: 10.1016/j.ijcard.2014.04.176
44. Bigger, JT Jr, Fleiss, JL, Steinman, RC, Rolnitzky, LM, Kleiger, RE, and Rottman, JN. Frequency domain measures of heart period variability and mortality after myocardial infarction. Circulation. (1992) 85:164–71. doi: 10.1161/01.CIR.85.1.164
45. Bigger, JT Jr, Fleiss, JL, Rolnitzky, LM, and Steinman, RC. Frequency domain measures of heart period variability to assess risk late after myocardial infarction. J Am Coll Cardiol. (1993) 21:729–36. doi: 10.1016/0735-1097(93)90106-B
46. Tapanainen, JM, Thomsen, PE, Kober, L, Torp-Pedersen, C, Makikallio, TH, Still, AM, et al. Fractal analysis of heart rate variability and mortality after an acute myocardial infarction. Am J Cardiol. (2002) 90:347–52. doi: 10.1016/S0002-9149(02)02488-8
47. Guzzetti, S, La Rovere, MT, Pinna, GD, Maestri, R, Borroni, E, Porta, A, et al. Different spectral components of 24 h heart rate variability are related to different modes of death in chronic heart failure. Eur Heart J. (2005) 26:357–62. doi: 10.1093/eurheartj/ehi067
48. Waren, A, and Ilyas, M. Literature review: heart rate variability as a biomonitoring of occupational stress. Indonesian J Occup Safety Health. (2021) 10:273–9. doi: 10.20473/ijosh.v10i2.2021.273-279
49. Thielmann, B, Pohl, R, and Böckelmann, I. Heart rate variability as a strain indicator for psychological stress for emergency physicians during work and alert intervention: a systematic review. J Occup Med Toxicol. (2021) 16:24. doi: 10.1186/s12995-021-00313-3
50. Hintsanen, M, Elovainio, M, Puttonen, S, Kivimaki, M, Koskinen, T, Raitakari, OT, et al. Effort-reward imbalance, heart rate, and heart rate variability: the cardiovascular risk in young Finns study. Int J Behav Med. (2007) 14:202–12. doi: 10.1007/BF03002994
51. Falk, A, Kosse, F, Menrath, I, Verde, PE, and Siegel, L. Unfair pay and health. Manag Sci. (2018) 64:1477–88. doi: 10.1287/mnsc.2016.2630
52. Thielmann, B, Hartung, J, and Bockelmann, I. Objective assessment of mental stress in individuals with different levels of effort reward imbalance or overcommitment using heart rate variability: a systematic review. Syst Rev. (2022) 11:48. doi: 10.1186/s13643-022-01925-4
53. Borchini, R, Bertu, L, Ferrario, MM, Veronesi, G, Bonzini, M, Dorso, M, et al. Prolonged job strain reduces time-domain heart rate variability on both working and resting days among cardiovascular-susceptible nurses. Int J Occup Med Environ Health. (2015) 28:42–51. doi: 10.2478/s13382-014-0289-1
54. Collins, SM, Karasek, RA, and Costas, K. Job strain and autonomic indices of cardiovascular disease risk. Am J Ind Med. (2005) 48:182–93. doi: 10.1002/ajim.20204
55. Collins, S, and Karasek, R. Reduced vagal cardiac control variance in exhausted and high strain job subjects. Int J Occup Med Environ Health. (2010) 23:267–78. doi: 10.2478/v10001-010-0023-6
56. Hernandez-Gaytan, SI, Rothenberg, SJ, Landsbergis, P, Becerril, LC, De Leon-Leon, G, Collins, SM, et al. Job strain and heart rate variability in resident physicians within a general hospital. Am J Ind Med. (2013) 56:38–48. doi: 10.1002/ajim.22098
57. Loerbroks, A, Schilling, O, Haxsen, V, Jarczok, MN, Thayer, JF, and Fischer, JE. The fruits of ones labor: effort-reward imbalance but not job strain is related to heart rate variability across the day in 35-44-year-old workers. J Psychosom Res. (2010) 69:151–9. doi: 10.1016/j.jpsychores.2010.03.004
58. Lee, KH, Yoon, K, Ha, M, Park, J, Cho, SH, and Kang, D. Heart rate variability and urinary catecholamines from job stress in korean male manufacturing workers according to work seniority. Ind Health. (2010) 48:331–8. doi: 10.2486/indhealth.48.331
59. Jarczok, MN, Koenig, J, Li, J, Mauss, D, Hoffmann, K, Schmidt, B, et al. The Association of Work Stress and Glycemic Status is Partially Mediated by autonomic nervous system function: cross-sectional results from the Mannheim industrial cohort study (MICS). PLoS One. (2016) 11:e0160743. doi: 10.1371/journal.pone.0160743
60. Garza, JL, Cavallari, JM, Eijckelhof, BH, Huysmans, MA, Thamsuwan, O, Johnson, PW, et al. Office workers with high effort-reward imbalance and overcommitment have greater decreases in heart rate variability over a 2-h working period. Int Arch Occup Environ Health. (2015) 88:565–75. doi: 10.1007/s00420-014-0983-0
61. Uusitalo, A, Mets, T, Martinmaki, K, Mauno, S, Kinnunen, U, and Rusko, H. Heart rate variability related to effort at work. Appl Ergon. (2011) 42:830–8. doi: 10.1016/j.apergo.2011.01.005
62. Wang, L, Shi, Y, Hu, Z, Li, Y, Ang, Y, Jing, P, et al. Longitudinal associations of work stress with changes in quality of life among patients after acute coronary syndrome: a hospital-based study. Int J Environ Res Public Health. (2022) 19:17018. doi: 10.3390/ijerph192417018
63. Zhang, X, Wang, Z, and Li, T. The current status of occupational health in China. Environ Health Prev Med. (2010) 15:263–70. doi: 10.1007/s12199-010-0145-2
64. Li, J, Yin, P, Wang, H, Zeng, X, Zhang, X, Wang, L, et al. The disease burden attributable to 18 occupational risks in China: an analysis for the global burden of disease study 2017. Environ Health. (2020) 19:21. doi: 10.1186/s12940-020-00577-y
65. Mazzola, JJ, Schonfeld, IS, and Spector, PE. What qualitative research has taught us about occupational stress. Stress Health. (2011) 27:93–110. doi: 10.1002/smi.1386
66. Melamed, S, Shirom, A, Toker, S, Berliner, S, and Shapira, I. Burnout and risk of cardiovascular disease: evidence, possible causal paths, and promising research directions. Psychol Bull. (2006) 132:327–53. doi: 10.1037/0033-2909.132.3.327
67. Andersson, C, and Vasan, RS. Epidemiology of cardiovascular disease in young individuals. Nat Rev Cardiol. (2018) 15:230–40. doi: 10.1038/nrcardio.2017.154
68. Trudel, X, Gilbert-Ouimet, M, Vezina, M, Talbot, D, Masse, B, Milot, A, et al. Effectiveness of a workplace intervention reducing psychosocial stressors at work on blood pressure and hypertension. Occup Environ Med. (2021) 78:738–44. doi: 10.1136/oemed-2020-107293
69. Yonezawa, R, Masuda, T, Matsunaga, A, Takahashi, Y, Saitoh, M, Ishii, A, et al. Effects of phase II cardiac rehabilitation on job stress and health-related quality of life after return to work in middle-aged patients with acute myocardial infarction. Int Heart J. (2009) 50:279–90. doi: 10.1536/ihj.50.279
Keywords: acute coronary syndrome, effort-reward imbalance, heart rate variability, job strain, work stress
Citation: Hu Z, Cao X, Jing P, Zhang B, Shi Y, Siegrist J, Li J and Zhang M (2024) Work stress and changes in heart rate variability among employees after first acute coronary syndrome: a hospital-based longitudinal cohort study. Front. Public Health. 12:1336065. doi: 10.3389/fpubh.2024.1336065
Edited by:
Sohrab Amiri, Baqiyatallah University of Medical Sciences, IranReviewed by:
Elisabeth Maria Balint, Private Clinic Meiringen, SwitzerlandNicola Mucci, University of Florence, Italy
Copyright © 2024 Hu, Cao, Jing, Zhang, Shi, Siegrist, Li and Zhang. This is an open-access article distributed under the terms of the Creative Commons Attribution License (CC BY). The use, distribution or reproduction in other forums is permitted, provided the original author(s) and the copyright owner(s) are credited and that the original publication in this journal is cited, in accordance with accepted academic practice. No use, distribution or reproduction is permitted which does not comply with these terms.
*Correspondence: Min Zhang, zhangm@ydyy.cn; Jian Li, jianli2019@ucla.edu
†These authors have contributed equally to this work and share first authorship