- 1Department of Management, Jamia Hamdard, New Delhi, India
- 2USN School of Business, University of South-Eastern Norway, Kongsberg, Norway
Introduction: This study empirically investigates the attitude of tobacco and alcohol consumers towards health insurance purchase in India. The study aims to determine the factors which plays a significant role in determining the purchase intention of health insurance among tobacco and alcohol consumers.
Methods: We propose an extended theory of planned behavior (TPB) model comprising factors like attitude, subjective norms, perceived behavior control, perceived usefulness, perceived product risk, and intention to purchase. We collected responses from 420 tobacco and alcohol consumers through a Google Form link shared via different social media platforms. SPSS has been used to perform exploratory factor analysis, whereas AMOS has been used to validate the constructs, confirm the relationships among the variables, and analyze the data.
Results: The analysis outcomes demonstrate that subjective norms, perceived product risk, and perceived behavioral control are the factors that have a positive and significant effect on health insurance purchase intention among consumers.
Discussion: This research offers valuable insights to the insurance sector, government officials, policymakers, and academicians. Insurance companies may consider the criteria analysed when creating policies to promote the expansion of the health insurance sector.
1 Introduction
There are around 1.3 Billion tobacco users globally; out of them, an estimated 80% of tobacco users reside in low-and middle-income nations (1). By spendthrift of income from essentials like food and shelter, tobacco and alcohol consumers are found to push families into greater debt. Additionally, it results in the early demise and disability of working-age individuals within homes, which lowers household income and raises healthcare expenses. In India, health insurance is rapidly rising as a prominent sector, following life and automobile insurance. The health insurance industry is a primary driver of the expansion of the general insurance sector. It contributes around 29% of India’s total general insurance premium income (2). India spends 3.01% of its gross domestic product (GDP) on health, but only a third of it comes from public funds, a proportion lower than that of other developing countries (3). In the advent of decreasing saving rates from 29.9% (2020) to 28.2% (2021) in India (4), people generally take loans from organized and unorganized resources when they get ill and repay the same when they get healthy again. Health insurance came into existence to support consumers and prevent such situations. The primary goal of health insurance policy is to reduce the risk people face in the event of unexpected healthcare costs, preventing them from being negatively impacted by financial burdens.
Health insurance denotes the source of funds for medical care financing, in which one decides to purchase healthcare insurance on a specified contract (5). Health insurance is cited as a crucial intervention in a recent government of India policy statement to give financial security and lower out-of-pocket (OOP) expenses. According to reports, 70% of the population might be covered by existing health insurance programs, including government-subsidized plans, social health insurance programs, and private voluntary health insurance (6). In terms of both employment and revenue, the healthcare industry has grown to be one of India’s biggest industries, and it is in the top three in terms of incremental growth. Rising income levels, increased health awareness, the prevalence of lifestyle diseases, and government initiatives like the Pradhan Mantri Jan Arogya Yojana (PM-JAY), National Digital Health Mission (NDHM), etc. are all expected to contribute to the healthcare market in India reaching an estimated $8.6 trillion in value by 2022 (7).
Health insurance is increasingly vital in health care services (8). In 2018, India launched one of the biggest government-funded health insurance schemes to protect the vulnerable population from financial hardship and encourage them to seek hassle-free health care (9). Health insurance programs play a significant role in fighting poverty as they also connect to other social supports such as food assistance. Children’s health insurance programs are linked to decreasing mortality, fewer chronic health conditions, better educational attainment, and less reliance on government support (10). A related study has shown that a mother’s health insurance subscription influences child stunting and underweight through maternal healthcare utilization and providing diversified diets to the children (11).
With 1.35 billion beneficiaries, India’s healthcare system is one of the world’s largest, but most citizens lack access to health coverage or quality care (12). In India, there are 267 million tobacco users, with one of the world’s highest rates of tobacco use. It has been reported that tobacco causes a substantial economic burden, totaling an estimated USD 22.4 billion annually (13). In 2018, the World Health Organization (WHO) estimated that tobacco use accounted for one million deaths, or 9.5% of all deaths in the country. Notably, more than 3,500,000 deaths annually are associated with smokeless tobacco use. Furthermore, alcohol consumption in India amounted to about five billion liters in 2020 and is estimated to reach about 6.21 billion liters by 2024. According to a study, around 88% of Indians aged under 25 purchase or consume alcohol (14). According to the National Crime Records Bureau (NCRB), 6,172 people died between 2016 and 2020 due to the consumption of illicit liquor in India (15). Such consumers cover a large portion of the young population and the nation’s future, making it necessary to cover them under health insurance study. The country’s healthcare system has not been able to respond enough to the challenge. In 2018, India spent roughly 1.3% of its GDP on health care, one of the lowest health expenditures globally (3).
Few studies on the Theory of Planned Behavior (TPB) have been undertaken in India, focusing on insurance sectors. Still, we could not find studies focusing on the tobacco and alcohol consumers of India. However, the literature addressing the purchase intention of health insurance is also limited. The present study aims to examine the factors affecting the purchase of health insurance among tobacco and alcohol consumers. The study empirically investigated how attitude toward behavior, subjective norms, perceived behavior control, perceived usefulness, perceived product risk, intention to purchase, and behavioral control may play a significant role in determining the purchase of health insurance among tobacco and alcohol consumers in the context of emerging countries like India.
The primary purpose of this research is to explore and determine the behavioral intentions of alcohol and tobacco consumers toward purchasing health insurance in India. Understanding the behavioral intention of Indians may provide a useful framework for future marketing communications to reach the target population and determine ways to encourage more Indians to consider buying health insurance. The findings will likely help health insurance marketers form their strategic focus in developing the health insurance market in India. Applying planned behavior to health insurance services in India will contribute to the current literature by analyzing the underlying constructs of the behavioral intention toward purchasing health insurance in a developing country.
2 Theoretical background and hypotheses development
The effectiveness of the TPB in predicting the intention to purchase various products and services has been well-established by several researchers worldwide. The theory connects beliefs and behavior. This model was postulated by Ajzen (16), which states that an individual’s behavior in purchasing a product or service is determined by their intention. The intention to purchase a product/service depends upon the readiness of a consumer and how well they perceive the product. According to (17), purchase intention can be described as the consumer disposition to buy a product. Previous studies have stated that purchase intention is a base for actual purchase behavior (17–19). Fishbein and Ajzen (20) state that actual purchase behavior is influenced by the desire to purchase that product.
Considering the TPB’s robustness, several researchers have used the model in different contexts (18, 21, 22). In the present study, we have used an extension of the TPB model (Figure 1) to predict alcohol and tobacco consumers’ behavior toward buying health insurance. In this paper, the relationship among Attitude toward behavior (ATB), Subjective norms (SN), Perceived behavior control (PBC), Perceived usefulness (PU), Perceived product risk (PPR), with Intention to purchase has been explored.
2.1 Attitude toward behavior and intention to purchase
The term “attitude” refers to a psychological inclination expressed by evaluating something with either some degree of favor or disapproval (23). Attitude is how an individual experiences things and makes conceptions that influence their behavior (24). According to Fishbein and Ajzen (20), an attitude toward behavior indicates whether an individual likes or dislikes a particular thing. The intention to participate in an activity is stronger for individuals with a positive attitude toward it (25). A consumer is far more likely to purchase an insurance product to satisfy their consumption demands if their attitude is positive. Guan et al. (26) reported that attitude is an important factor influencing consumer buying decisions toward insurance products. The TPB has been used in several types of research to evaluate the relationship between consumers’ attitudes and intentions to buy goods and services (23, 27–29). Based on the above literature, the following hypothesis is framed:
H1: Attitude has a positive effect on the purchase intention of health insurance among tobacco and alcohol consumers.
2.2 Subjective norms and intention to purchase
Ajzen (16) describes subjective norms as being related to a person’s perception of social pressure to engage in a particular action. According to Lujja et al. (30), a person’s perception of subjective norms depends on whether they feel that others who are significant to them should engage in a particular conduct. The opinion of one’s friends, family, and peers might impact people’s purchase intention. Subjective norms play an important role and positively influence consumption patterns (31). In social science research, it has been widely accepted that subjective norms are a predictor of intention (29, 32). Researchers like Jain (22) have suggested that subjective norms are important in determining consumers’ purchase intention. Several researchers have suggested a positive and significant relationship between subjective norms and intent to purchase (19, 33, 34). Based on the above literature, the following hypothesis is framed:
H2: Subjective norms positively impact the purchase intention of health insurance among tobacco and alcohol consumers.
2.3 Perceived behavior control and intention to purchase
The perspective of an individual toward the ease or difficulty of carrying out any behavior is known as perceived behavioral control (34, 35). Perceived behavior control, directly and indirectly, impacts consumer behavior through intention, assuming that this component has motivational consequences for behavioral intention (32). Moreover, the consumer perception and their ability to engage in a specific activity directly influence their purchase intention. If consumers feel they have the necessary tools and the confidence to carry out a behavior, they are more inclined to engage in that behavior. Husin and Ab Rahman (35) stated that perceived behavioral control plays a significant role in the consumer buying decision and positively relates to their intention to purchase. Furthermore, several researchers have shown the significant effect of perceived behavior control on purchase intention in their studies (19, 36). Based on the above literature following hypothesis is framed:
H3: Perceived behavior control has a positive effect on the purchase intention of health insurance among tobacco and alcohol consumers.
2.4 Perceived usefulness and intention to purchase
It is stated that increasing a specific utility in people’s perceptions will make them feel more favorable about buying a particular product or service (37). The perceived usefulness of any insurance impacts a person’s decision to buy that particular insurance (23). Perceived usefulness significantly impacts how people feel about buying health insurance (38). In their study, Aziz et al. (17) highlighted a positive correlation between the purchase of health insurance and consumer intention. Moreover, they have suggested that insurance firms should consider and communicate its usefulness to motivate consumers to get health insurance. Several studies have advocated the significant impact of perceived usefulness on purchase intention (21, 39). Thus, the following hypothesis is framed:
H4: Perceived usefulness has a positive effect on the purchase intention of health insurance among tobacco and alcohol consumers.
2.5 Perceived product risk and intention to purchase
Perceived risk is defined as the expectation of unfavorable consequences made by the customer (40, 41). Product risk refers to the probability that a purchased product will not offer the intended advantages or function properly (42, 43). Product risk involves a potential loss if the product does not meet consumer expectations regarding product standards and quality (44, 45). Perceived risk plays a significant role in identifying consumer perception and intention to purchase (44). Consumer intention toward purchasing health insurance depends greatly on the perceived risk (Liebenberg et al., 2012). Perceived risk has been suggested to be an important factor in determining the consumers’ behavioral intention. Several researchers have found a significant impact of perceived risk on the behavioral patterns of consumers (44–46). Based on the above literature, the following hypothesis is framed:
H5: Perceived product risk has a positive effect on the purchase intention of health insurance among tobacco and alcohol consumers.
3 Research methodology
3.1 Stage 1: scale development
This study is an extension of the TPB model to investigate the intention to purchase health insurance among tobacco and alcohol consumers in India. Taking a cue from the extant literature, a model comprising of factors like attitude toward behavior (ATB), subjective norms (SNorm), perceived behavior control (PERBC), perceived usefulness (PU), perceived product risk (PPRsk), and intention to purchase health insurance (INHI) was proposed. The study scale comprised 30 items, a six-factor scale (Table 1). The items used in the study were adapted from the work of Mamun et al. (23) and Brahmana et al. (21). The questionnaire items measuring all the constructs were based on a 5-point Likert scale (1 = strongly disagree; 2 = disagree; 3 = neither agree nor disagree; 4 = agree; 5 = strongly agree).
3.2 Stage 2: pilot study
To check the one-dimensionality of the scale, researchers have suggested going for a pilot survey before the actual data collection (47). In the present study, we carried out a pilot survey on the proposed model to check the one-dimensionality of the used scale. The initial data was collected from 85 respondents and further analyzed using exploratory factor analysis (EFA) on SPSS 22. The Bartlett’s Test of Sphericity (BTS) was found to be significant. The Kaiser-Meyer-Olkin (KMO) value was also found to be satisfactory (0.939; >0.6) (48). The total variance was found to be 77.46.
Considering the previous research suggestions, the current study also looked at factor loadings and communalities to decide which items should be kept and which should be deleted. Some items were removed due to cross-loading or low factor loadings of less than 0.5. Because of low loadings (<0.5) and cross-loadings, certain items of the proposed scale had to be dropped. The iterative process of scale refinement resulted in a shorter four-factor (19-item) scale comprising subjective norms, perceived behavior control, perceived product risk, and intention to purchase health insurance (Table 1). It can be surmised from the analysis that the process resulted in the dropping of all the items of Attitude and Perceived Usefulness because of low as well as cross-loadings. Further, the hypotheses H1 and H4 had to be dropped. Also, one item of subjective norm had to be dropped because of low loading. The factors of the scale were found to reliable, with the alpha value was observed to be in the acceptable range, i.e., SN- 0.936, PBC- 0.892, PPR- 0.935, and IHI- 0.899 (48).
3.3 Data collection and ethical considerations
It has been recommended that the sample size of a study must be 10 times the number of items used in a scale (49). This means that 19 * 10 = 190 was the appropriate sample size for the present work. We gathered participant feedback using digital (via a link to a Google Form) and traditional methods (using paper and pencil). As per the suggestion of Bertilsson (50), if the respondents are offered anonymity in a study, no informed consent is required from them. In the present study, we have stood up to the ethical guidelines by obtaining prior consent from the respondents and maintaining the respondents’ anonymity in the research.
With the constraints of having no exact data on the tobacco and alcohol consumers, convenience sampling has been used for the study. The convenience sampling method is adopted because it is a type of nonprobability or non-random sampling in which study participants are selected based on practical considerations such as proximity, ease of accessibility, availability at a particular time, or willingness to participate. This sampling method is affordable and accessible, and the respondents are readily available (51). The study survey has been conducted in the Delhi NCR region. Delhi is also teeming with diverse socioeconomic classes (52). The largest metropolitan region in the nation is also located in the study area. It draws a sizable number of migrants from different states (53, 54). Over a three-month period, 420 replies were received in total, which was more than the minimal number of suggested responses needed. Out of the 420 responses, 389 were deemed suitable for subsequent analysis. The researchers excluded responses with irregular answering patterns or incomplete data on the variables in question. The sample individuals were between the age group of 18–50 who, according to researchers, have been found to consume more tobacco and alcohol compared to others (55, 56). Over 85% of the responses came from a younger demographic, specifically individuals aged between 18 and 30 years. The questionnaires focused on tobacco and alcohol use; therefore, respondents under the age of 18 were excluded from the study in compliance with Indian government regulations that categorize them as minors COPTA (57). The demographic profile of the respondents is presented below in Table 2.
4 Data analysis
Confirmatory factor analysis has been performed using AMOS on the four factors, i.e., perceived behavior control (PERBC), intention to purchase health insurance (INHI), perceived product risk (PPR), and subjective norms (SN). To consider the specification of the items loading on their respective variables, the measurement model (Figure 2) was evaluated for model fit. The loadings of the items with their corresponding variables were found to be within the acceptable range. The measurement model’s fit indices indicated a good model fit with comparative fit index (CFI) = 0.942, the goodness of fit index (GFI) = 0.886, adjusted goodness of fit index (AGFI) = 0.852 and root mean square error of approximation (RMSEA) = 0.81. Although the value of GFI and AGFI is less than 0.90 researchers have stated that these standards can be regarded as acceptable (58, 59). Thus, the overall model was found to be satisfactory.
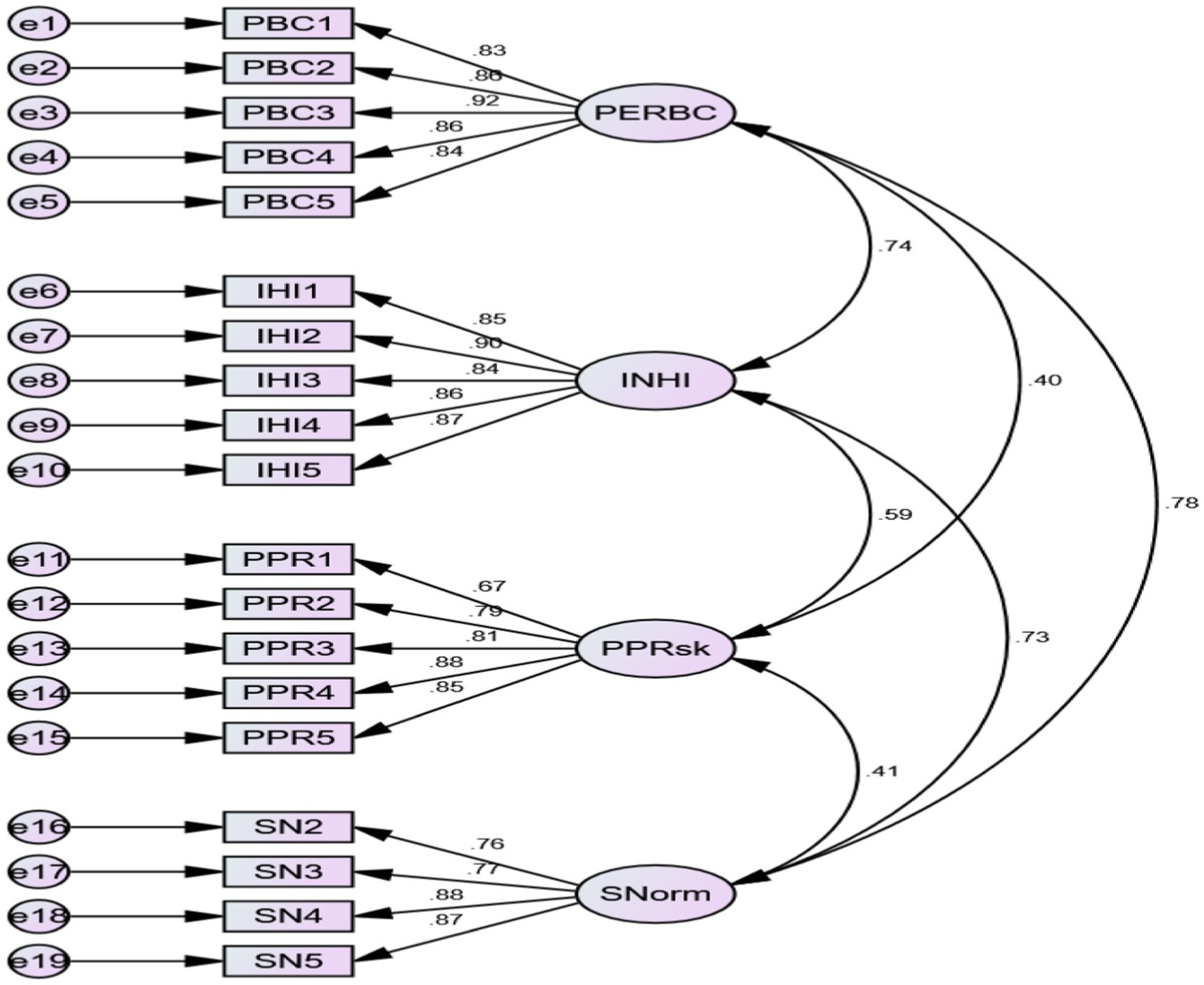
Figure 2. Measurement model. Perceived Behavior Control (PERBC), Intention to Purchase Health Insurance (INHI), Perceived Product Risk (PPRsk), Subjective Norms (SNorm).
Convergent and discriminant validity were examined using the average variance extracted (AVE) (60). The AVE for the current measurement model was determined to be above the benchmark value of 0.5 (Table 3), indicating good convergent validity (60, 61). Each construct’s square root of AVE (bold diagonal values in Table 3) was greater than the inter-construct correlations, showing that the scale’s discriminant validity was satisfactory (60, 61). Researchers like Hair et al. (60) and Malhotra and Dash (48), have suggested the reliability of the factors to be demonstrated by the composite reliability, which was significantly higher than the threshold value of 0.7 (Table 3). Thus, the values suggest the scale to be reliable and valid.
4.1 Structural model
Table 4 presents model fit indicators of the structural model (Figure 3). With CMIN/df = 4.194 and RMSEA = 0.81, the CFI value was found to be greater than that the recommended value of 0.90 The GFI and AGFI values were less than 0.90, but researchers have suggested values below that too can be regarded fine (64). Thus, the goodness-of-fit measures and overall model fit indices were within the acceptable parameters, signifying the model to be fit.
Subjective norm positively impacts the intention to buy health insurance, leading to support hypothesis H2 (ß = 0.360; sig < 0.05). Similarly, hypothesis H3, depicting a positive relationship between perceived behavioral control and intention to buy health insurance, was also supported (ß = 0.364; sig < 0.05). Perceived product risk was also found to positively impact the intention to buy health insurance; thus, hypothesis H5 was supported (ß = 0.378; sig < 0.05). The relevant indices have been depicted in Table 5.
5 Discussion
India has been seeing constant economic growth and is one of the biggest economies in the world. Nearly 514 million individuals in India had health insurance coverage for the fiscal year 2021 (65). Government-sponsored health insurance programs covered most of them, while individual insurance policies covered the minority. The study findings suggest that subjective norms, perceived behavioral control, and perceived product risk (PERBC, PPRsk, and SNorms) positively impact the purchase intention for health insurance. In this study, we extend the TPB model by adding a new factor, i.e., perceived product risk. Thus, the study findings significantly contribute to the existing literature on health insurance. We took a cue from the extant literature and proposed a six-factor model. The proposed model comprises five independent factors that help determine the purchase intention of tobacco and alcohol consumers toward health insurance. However, the pilot study yielded three independent factors (SNorms, PERBC, and PPRsk) significantly influencing purchase intention. Attitude and perceived usefulness were removed from the scale because of low factor loadings and cross-loadings.
The findings of the present study indicate that tobacco and alcohol consumers in India are more concerned with subjective norms, perceived behavior control, and perceived product risk in the context of purchasing health insurance. The findings of this paper suggest that the three factors are essential and play a significant role in building up a positive purchase intention among tobacco and alcohol consumers toward health insurance. Moreover, to enhance the interest in obtaining health insurance among users of alcohol and tobacco, insurance policymakers and marketing practitioners should focus on these three factors.
The study findings align with the results of several researchers who found the above factors to have a significant role in determining consumers’ purchase intention. Bainchi et al. (32) suggested that consumer purchasing behavior is influenced by family, friends, relatives, colleagues, and others perceived as important. If the consumers can engage in any activity, they are more likely to buy it. The significance of perceived behavior control on purchase intention has been suggested by several researchers, including Photcharorn et al. (19) and Husin and Ab Rahman (35). The findings of our study also highlight the significance of perceived behavior control on the purchase intention of health insurance among tobacco and alcohol consumers. The study findings indicate that perceived product risk has a significant role in determining consumer purchase intentions, which aligns with the results of other researchers. Perceived product risk refers to the potential for a product to perform unconventionally compared to its original specification (45). When customers are uncertain about their choice, they experience risk during the purchasing process (46). According to the theory of how consumers perceive risk, customers feel risk when faced with uncertainty and unfavorable outcomes from actions that do not meet their expectations (46, 66, 67).
5.1 Implications
The study has examined the correlation between TPB components and the purchase intention of tobacco and alcohol consumers, leading to health insurance purchases. A new independent factor, perceived product risk (PPR), is embedded into the TPB model, which appears crucial for tobacco and alcohol consumers to purchase health insurance. In the present study, we extended the TPB model by incorporating perceived product risk to determine the purchase intention of health insurance among tobacco and alcohol consumers in India.
We have tried to explore the intention of a cohort rarely tapped by the research community. The study’s findings can help the insurance industry create strategies and make policies for the growth of their health insurance sector. Insurance companies should emphasize the unpredictability of the future to boost demand for health insurance. The policymakers of the insurance industry can incorporate subjective norms, perceived behavior control, and perceived product risk in their strategies to accelerate more demand for their health insurance products. In addition to encouraging individuals to get health insurance, the government and insurance providers may take the initiative to educate people on the value of having health insurance. This research makes a significant contribution to the field of health insurance studies. This study provides insurance companies and policymakers with a better understanding of consumer purchase behavior and their perception, which can be capitalized to enable them to be in the right direction. Based on the study findings, insurance companies should be able to develop more effective marketing strategies by focusing on tobacco and alcohol consumers.
Several behavioral factors, including insurance literacy, perceived complexity, and regulation literacy, influence the purchase intention among tobacco and alcohol consumers. This suggests that insurance providers must offer training courses to enlighten the public about health insurance, disseminate knowledge, and develop easier processes. Consumers would become more motivated to buy health insurance-related items and services.
5.2 Limitations and future directions
It should be kept in mind that our study has certain limitations. First, we have used an extended version of the TPB model in the current study. Future research could extend the model by including other relevant factors. Second, this study is related to the purchase intention toward health insurance among tobacco and alcohol consumers only. Third, the data represent respondents from a single host country (India), so the study findings may not apply to other parts of the world. Our study results should be validated by including respondents worldwide in future work.
Data availability statement
The raw data supporting the conclusions of this article will be made available by the authors, without undue reservation.
Ethics statement
Ethical approval was not required for the study involving humans in accordance with the local legislation and institutional requirements. The participants provided their written informed consent to participate in this study.
Author contributions
AM: Conceptualization, Data curation, Formal analysis, Investigation, Methodology, Resources, Software, Visualization, Writing – original draft, Writing – review & editing. MJ: Conceptualization, Data curation, Formal analysis, Investigation, Methodology, Resources, Software, Validation, Writing – original draft, Writing – review & editing. AA: Conceptualization, Investigation, Methodology, Project administration, Resources, Software, Supervision, Validation, Writing – original draft, Writing – review & editing. SG: Investigation, Methodology, Resources, Validation, Writing – original draft, Writing – review & editing. DM: Project administration, Resources, Validation, Writing – review & editing.
Funding
The author(s) declare that no financial support was received for the research, authorship, and/or publication of this article.
Conflict of interest
The authors declare that the research was conducted in the absence of any commercial or financial relationships that could be construed as a potential conflict of interest.
Publisher’s note
All claims expressed in this article are solely those of the authors and do not necessarily represent those of their affiliated organizations, or those of the publisher, the editors and the reviewers. Any product that may be evaluated in this article, or claim that may be made by its manufacturer, is not guaranteed or endorsed by the publisher.
References
1. WHO. Tobacco. (2023). Available at: https://www.who.int/news-room/fact-sheets/detail/tobacco
2. Dutta, MM. Health insurance sector in India: an analysis of its performance. Vilakshan XIMB J Manag. (2020) 17:97–109. doi: 10.1108/XJM-07-2020-0021
3. The World Bank. Current health expenditure (% of GDP). (2023). Available at: https://data.worldbank.org/indicator/SH.XPD.CHEX.GD.ZS (Accessed March 29, 2023).
4. CEICdata.com. India gross savings rate. Economic indicators | CEIC. (2021). Available at: https://www.ceicdata.com/en/indicator/india/gross-savings-rate (accessed February 19, 2023
5. Anandalakshmy, A, and Brindha, K. Policy holders’ awareness and factors influencing purchase decision towards health insurance in Coimbatore District. Policy. (2017) 3:12–6.
6. Gupta, R, Makkar, JS, Sharma, SK, Agarwal, A, Sharma, KK, Bana, A, et al. Association of health insurance status with coronary risk factors, coronary artery disease, interventions and outcomes in India. Int J Cardiol Cardiovasc Risk Preven. (2022) 14:200146. doi: 10.1016/j.ijcrp.2022.200146
7. Kumar, R, and Duggirala, A. Health insurance as a healthcare financing mechanism in India: key strategic insights and a business model perspective. Vikalpa. (2021) 46:112–28. doi: 10.1177/02560909211027089
8. Sonymol, K, and Shankar, R. Healthcare cost reduction and health insurance policy improvement. Value Health Reg Issues. (2022) 29:93–9. doi: 10.1016/j.vhri.2021.10.001
9. Dubey, S, Deshpande, S, Krishna, L, and Zadey, S. Evolution of government-funded health insurance for universal health coverage in India. Lancet Reg Health Southeast Asia. (2023) 13:100180. doi: 10.1016/j.lansea.2023.100180
10. Wherry, LR, Kenney, GM, and Sommers, BD. The role of public health insurance in reducing child poverty. Acad Pediatr. (2016) 16:S98–S104. doi: 10.1016/j.acap.2015.12.011
11. Kofinti, RE, Koomson, I, Paintsil, JA, and Ameyaw, EK. Reducing children’s malnutrition by increasing mothers’ health insurance coverage: a focus on stunting and underweight across 32 sub-Saharan African countries. Econ Model. (2022) 117:106049. doi: 10.1016/j.econmod.2022.106049
12. Worldometer India Population. (2019). Available at: https://www.worldometers.info/world-population/india-population/ (Accessed March 29, 2023).
13. John, RM, Rout, SK, Kumar, BR, and Arora, M. Economic burden of tobacco related diseases in india, New Delhi: Ministry of Health and Family Welfare, Government of India. (2014).
14. Minhas, A.. Consumption of alcoholic beverages in India 2020–2024. (2023). Available at: https://www.statista.com/statistics/727026/consumption-of-alcoholic-beverages-india/#:~:text=Alcohol%20consumption%20in%20India%20amounted,growing%20urban%20population%20among%20others (Accessed on 5/10/2023).
15. Krishnan, M Why alcohol consumption is on the rise in India 2022. (2022). Available at: https://www.dw.com/en/why-alcohol-consumption-is-on-the-rise-in-india/a-63296901 (Accessed 5/10/2023).
16. Ajzen, I. The theory of planned behavior. Organ Behav Hum Decis Process. (1991) 50:179–211. doi: 10.1016/0749-5978(91)90020-T
17. Aziz, S, Husin, MM, Hussin, N, and Afaq, Z. Factors that influence individuals’ intentions to purchase family takaful mediating role of perceived trust. Asia Pac J Mark Logist. (2019) 31:81–104. doi: 10.1108/APJML-12-2017-0311
18. Hassan, HA, and Abbas, SK. Factors influencing the investors’ intention to adopt Takaful (Islamic insurance) products: a survey of Pakistan. J Islamic Market. (2020) 11:1–13. doi: 10.1108/JIMA-03-2018-0064
19. Photcharoen, C, Chung, R, and Sann, R. Modelling theory of planned behavior on health concern and health knowledge towards purchase intention on organic products. Int Bus Res. (2020) 13:100–16. doi: 10.5539/ibr.v13n8p100
20. Fishbein, M, and Ajzen, I. Belief, attitude, intention and behaviour: an introduction to theory and research. Boston, US: Addison-Wesley (1975).
21. Brahmana, R, Brahmana, RK, and Memarista, G. Planned behaviour in purchasing health insurance. South East Asian J Manag. (2018) 12:53–64. doi: 10.21002/seam.v12i1.7465
22. Jain, S. Assessing the moderating effect of subjective norm on luxury purchase intention: a study of gen Y consumers in India. Int J Retail Distrib Manag. (2020) 48:517–36. doi: 10.1108/IJRDM-02-2019-0042
23. Mamun, AA, Rahman, MK, Munikrishnan, UT, and Permarupan, PY. Predicting the intention and purchase of health insurance among Malaysian working adults. SAGE Open. (2021) 11:215824402110613. doi: 10.1177/21582440211061373
24. Bananuka, J, Kasera, M, Najjemba, GM, Musimenta, D, Ssekiziyivu, B, and Kimuli, SNL. Attitude: mediator of subjective norm, religiosity and intention to adopt Islamic banking. J Islamic Market. (2020) 11:81–96. doi: 10.1108/JIMA-02-2018-0025
25. Shih, HM, Chen, BH, Chen, MH, Wang, CH, and Wang, LF. A study of the financial behavior based on the theory of planned behavior. Int J Market Stud. (2022) 14:1. doi: 10.5539/ijms.v14n2p1
26. Guan, LP, Yusuf, DHM, and Ghani, MRA. Factors influencing customer purchase intention towards insurance products. Int J Bus Manag. (2020) 4:70–9. doi: 10.26666/rmp.ijbm.2020.5.9
27. Casidy, R, Phau, I, and Lwin, M. The role of religious leaders on digital piracy attitude and intention. J Retail Consum Serv. (2016) 32:244–52. doi: 10.1016/j.jretconser.2016.04.006
28. Chin, HC, Choong, WW, Alwi, SRW, and Mohammed, AH. Using theory of planned behaviour to explore oil palm smallholder planters’ intention to supply oil palm residues. J Clean Prod. (2016) 126:428–39. doi: 10.1016/j.jclepro.2016.03.042
29. Hsu, CL, Chang, CY, and Yansritakul, C. Exploring purchase intention of green skincare products using the theory of planned behavior: testing the moderating effects of country of origin and price sensitivity. J Retail Consum Serv. (2017) 34:145–52. doi: 10.1016/j.jretconser.2016.10.006
30. Lujja, S, Omar Mohammad, M, and Hassan, R. Modelling public behavioral intention to adopt Islamic banking in Uganda: the theory of reasoned action. Int J Islam Middle East Financ Manag. (2016) 9:583–600. doi: 10.1108/IMEFM-08-2015-0092
31. Basha, MB, and Lal, D. Indian consumers’ attitudes towards purchasing organically produced foods: an empirical study. J Clean Prod. (2019) 215:99–111. doi: 10.1016/j.jclepro.2018.12.098
32. Bianchi, M, Bagnasco, A, Aleo, G, Catania, G, Zanini, MP, Timmins, F, et al. Preparing healthcare students who participate in interprofessional education for interprofessional collaboration: a constructivist grounded theory study protocol. J Interprof Care. (2018) 32:367–9. doi: 10.1080/13561820.2017.1340877
33. Judge, M, Warren-Myers, G, and Paladino, A. Using the theory of planned behaviour to predict intentions to purchase sustainable housing. J Clean Prod. (2019) 215:259–67. doi: 10.1016/j.jclepro.2019.01.029
34. Raza, SA, Ahmed, R, Ali, M, and Qureshi, MA. Influential factors of Islamic insurance adoption: an extension of theory of planned behavior. J Islamic Market. (2020) 11:1497–515. doi: 10.1108/JIMA-03-2019-0047
35. Husin, MM, and Ab Rahman, A. Do Muslims intend to participate in Islamic insurance? Analysis from theory of planned behaviour. J Islamic Acc Bus Res. (2016) 7:42–58. doi: 10.1108/JIABR-03-2014-0012
36. Mas’ud, A. (2016). Integrating the moderating effect of individuals’ risk vulnerability into takaful acceptance model: evidence from a frontier market. In 11th International Conference of Islamic Economics and Finance.
37. Dzulkipli, MR, Zainuddin, NNN, Maon, SN, Jamal, A, and Omar, MK. Intention to purchase medical and health insurance: application of theory of planned behavior. Adv Sci Lett. (2017) 23:10515–8. doi: 10.1166/asl.2017.10092
38. Berkman, ND, Sheridan, SL, Donahue, KE, Halpern, DJ, and Crotty, K. Low health literacy and health outcomes: an updated systematic review. Ann Intern Med. (2011) 155:97–107. doi: 10.7326/0003-4819-155-2-201107190-00005
39. Tennyson, S. Consumers’ insurance literacy: evidence from survey data. Fin Serv Rev. (2011) 20:165.
40. Park, S, and Tussyadiah, IP. Multidimensional facets of perceived risk in mobile travel booking. J Travel Res. (2017) 56:854–67. doi: 10.1177/0047287516675062
41. Pelaez, A, Chen, CW, and Chen, YX. Effects of perceived risk on intention to purchase: a meta-analysis. J Comput Inf Syst. (2019) 59:73–84. doi: 10.1080/08874417.2017.1300514
42. Farivar, S, Turel, O, and Yuan, Y. A trust-risk perspective on social commerce use: an examination of the biasing role of habit. Internet Res. (2017) 27:586–607. doi: 10.1108/IntR-06-2016-0175
43. Ventre, I, and Kolbe, D. The impact of perceived usefulness of online reviews, trust and perceived risk on online purchase intention in emerging markets: a Mexican perspective. J Int Consum Mark. (2020) 32:287–99. doi: 10.1080/08961530.2020.1712293
44. Ariffin, SK, Mohan, T, and Goh, YN. Influence of consumers’ perceived risk on consumers’ online purchase intention. J Res Interact Mark. (2018) 12:309–27. doi: 10.1108/JRIM-11-2017-0100
45. Zheng, L, Favier, M, Huang, P, and Coat, F. Chinese consumer perceived risk and risk relievers in e-shopping for clothing. J Electron Commer Res. (2012) 13:255.
46. Nursiana, A, Budhijono, F, and Fuad, M. Critical factors affecting customers’ purchase intention of insurance policies in Indonesia. J Asian Fin Econ Bus. (2021) 8:123–33. doi: 10.13106/jafeb.2021.vol8.no2.0123
47. Ahmad, A, and Kirmani, MD. Investigating factors affecting intention to use government websites for COVID-19-related information: an empirical study. Int J Electr Govern Res. (2020) 16:60–74. doi: 10.4018/IJEGR.2020040104
48. Malhotra, NK, and Dash, S. Marketing research: an applied orientation. 6th ed. NJ: Pearson Education (2011).
49. Hair, JF, Anderson, RE, Tatham, RL, and Black, WC. Multivariate data analysis with readings. 5th ed. Englewood Cliffs, NJ: Prentice Hall (1998).
50. Bertilsson, J. The cynicism of consumer morality. Consum Mark Cult. (2015) 18:447–67. doi: 10.1080/10253866.2015.1038255
52. Agarwal, PK, and Gupta, VP. Determinants of purchase intention of private brands in India: a study conducted on hypermarkets of Delhi. Optimiz J Res Manag. (2017) 9:42–52.
53. Gautam, U, and Bajpai Tewari, D. Public knowledge, attitudes, and practices toward COVID-19 pandemic: a cross-sectional study in Delhi and National Capital Region (NCR), India. SAGE Open. (2023) 13:215824402311533. doi: 10.1177/21582440231153370
54. Pan, X, Ojcius, DM, Gao, T, Li, Z, Pan, C, and Pan, C. Lessons learned from the 2019-nCoV epidemic on prevention of future infectious diseases. Microbes Infect. (2020) 22:86–91. doi: 10.1016/j.micinf.2020.02.004
55. Goel, N, Khandelwal, V, Pandya, K, and Kotwal, A. Alcohol and tobacco use among undergraduate and postgraduate medical students in India: a multicentric cross-sectional study. Cent Asian J Glob Health. (2015) 4. doi: 10.5195/cajgh.2015.187
56. Jumrani, J, and Birthal, PS. Does consumption of tobacco and alcohol affect household food security? Evidence from rural India. Food Secur. (2017) 9:255–79. doi: 10.1007/s12571-017-0660-8
57. COPTA. The cigarettes and other tobacco products (prohibition of advertisement and regulation of trade and commerce, production, supply and distribution) act, 2003. (2003). Available at: https://legislative.gov.in/sites/default/files/A2003-34.pdf.
58. Ahmad, A. Predicting attitude of young Indian consumers toward brand pages over social media: a structural equation modeling approach. J Public Aff. (2020) 20:e2093. doi: 10.1002/pa.2093
59. Ahmad, A, Rahman, O, and Khan, MN. Exploring the role of website quality and hedonism in forming e-satisfaction and e-loyalty: evidence from internet users in India. J Res Interact Mark. (2017) 11:246–67. doi: 10.1108/JRIM-04-2017-0022
60. Hair, JF, Black, WC, Babin, BJ, and Anderson, RE. Multivariate data analysis. 7th ed. Upper Saddle River, NJ: Pearson Prentice Hall (2010).
61. Khan, MN, and Adil, M. Data analysis techniques in service quality literature: essentials and advances. Serbian J Manag. (2013) 8:95–112. doi: 10.5937/sjm8-3469
62. Hooper, D, Coughlan, J, and Mullen, M. Structural Equation Modelling: Guidelines for Determining Model Fit. EJBRM. (2008) 6:53–60. doi: 10.21427/D7CF7R
63. Hu, LT, and Bentler, PM. Fit indices in covariance structure modeling: sensitivity to underparameterized model misspecification. Psychol Methods. (1998) 3:424–53. doi: 10.1037/1082-989X.3.4.424
64. Etezadi-Amoli, J, and Farhoomand, AF. A structural model of end user computing satisfaction and user performance. Inf Manag. (1996) 30:65–73. doi: 10.1016/0378-7206(95)00052-6
65. Patidar, S., and Dudi, S. (2023) Estimating medical insurance cost using linear regression with HyperParameterization, decision tree and random forest models. In 2023 13th international conference on cloud computing, data science & engineering (confluence) (pp. 504–508). Noida, India: IEEE.
66. Masoud, EY. The effect of perceived risk on online shopping in Jordan. Eur J Busin Manag. (2013) 5:76–87. doi: 10.7176/EJBM
Keywords: consumer behavior, factor analysis, health insurance, theory of planned behavior, intention to purchase, India
Citation: Mishra A, Jamshed M, Ahmad A, Garg S and Madsen DØ (2024) Factors influencing the intention to purchase health insurance: a study of Indian tobacco and alcohol consumers. Front. Public Health. 12:1332511. doi: 10.3389/fpubh.2024.1332511
Edited by:
Zahiruddin Quazi Syed, Datta Meghe Institute of Higher Education and Research, IndiaReviewed by:
Rojan Gümüş, Dicle University, TürkiyeBogdan Narcis Firtescu, Alexandru Ioan Cuza University, Romania
Copyright © 2024 Mishra, Jamshed, Ahmad, Garg and Madsen. This is an open-access article distributed under the terms of the Creative Commons Attribution License (CC BY). The use, distribution or reproduction in other forums is permitted, provided the original author(s) and the copyright owner(s) are credited and that the original publication in this journal is cited, in accordance with accepted academic practice. No use, distribution or reproduction is permitted which does not comply with these terms.
*Correspondence: Asad Ahmad, YXNhZDdiYWJhckBnbWFpbC5jb20=; Dag Øivind Madsen, ZGFnLm9pdmluZC5tYWRzZW5AdXNuLm5v