- 1School of Biological and Agricultural Engineering, Jilin University, Changchun, China
- 2Key Laboratory of Bionic Engineering, Ministry of Education, Jilin University, Changchun, China
- 3School of Business and Management, Jilin University, Changchun, China
Background: Dietary behavior is a pivotal modifiable determinant in reducing the occurrence of obesity/overweight and chronic non-communicable diseases. Improving the dietary behavior of rural residents in China is imminent due to the poor performance of their dietary behavior. Nutrition knowledge and health literacy are considered as elements that are linked intimately to healthy dietary behaviors but lack research in the Chinese setting.
Purpose: The study is designed to explore the relationship between nutritional knowledge, health literacy and dietary behaviors and to analyze the performance under different demographic characteristics.
Methods: A face-to-face survey of 400 rural residents on their nutrition knowledge, functional health literacy and dietary intake of five food categories consisting of 32 items was conducted based on a validated questionnaire. Descriptive analysis, difference test including ANOVA, t-test and non-parametric test, and multivariate linear regression were used for data analysis.
Results: The results indicate that declarative nutrition knowledge, individuals’ information application capacity, and dietary behaviors, especially the intake of fruits, dairy and beans, and vegetable are not ideal and requires improvement. Male, elder, low-income, unmarried, and low-education populations performed significantly worse and were the high-risk group. Procedural nutrition knowledge, information access capacity, information understanding capacity, and information application capacity have remarkable effects on better dietary behavior.
Conclusion: This study provides evidence-based guidance for prioritizing information and populations for healthy dietary interventions.
1 Introduction
The Chinese population is switching from a plant-based diet to western-style diets high in fat and animal-based foods (1), and malnutrition has significantly decreased. But, the proportion of residents with an imbalanced diet and overnutrition has gradually increased, and there is a significant expansion in the prevalence of overweight, obesity and patients with chronic non-communicable diseases (2). The proportion of adults with a BMI ≥ 25 kg/m2 have increased from 20% (about 150 million) in 1992 to nearly 50% (about 550 million) in the most recent national survey (3). Dietary behavior is one of the key modifiable determinants for reducing obesity/overweight and chronic disease prevalence in China (4, 5). The Chinese government pays significant attention to improving the residents’ dietary and successively formulated the Outline of the Health China 2030 Plan (6) and the National Nutrition Plan (2017–2030) (7).
Currently, the incidence of chronic diseases is higher in urban areas than in rural areas in China, and the growth rate of rural areas is higher than that in urban areas, which is related to poor dietary behavior in rural residents (8). China, however, is a developing country with a rural population of over 500 million, and policy implementation capacity in rural areas is extremely limited (9). It is necessary to identify priority intervention contents and groups in order to conduct interventions more effectively.
To prioritize the intervention information, two domains that are closely associated to dietary behavior, nutritional knowledge and health literacy, were considered. The prevailing research state that nutrition knowledge has an important role in health behavior (10, 11), including dietary behavior (12, 13). But some intervention experiments based on knowledge courses have yielded mixed results, and such differences may be due to interventions targeting different types of knowledge. Some studies have indicated that knowledge is not a simple structure, but multiple types (14). In cognitive psychology, knowledge can be classified as declarative knowledge or procedural knowledge. Declarative knowledge is knowing about facts and objects, while procedural knowledge is pertaining to the execution of behavior (15). Several scholars have analyzed the relationship with dietary behavior using procedural nutrition knowledge (PNK) and declarative nutrition knowledge (DNK), respectively (16, 17), but limited studies have considered both types of knowledge, especially for Chinese populations.
Health literacy has become increasingly important in recent years because evidence supports its association with healthy behaviors (18). Health literacy also plays an important role in health education and promotion (19) and is negatively correlated with national healthcare utilization and expenditure (20). The U.S. Department of Health and Human Services has defined health literacy as the ability of individuals to access, process, and understand health information to make decisions about treatment and their health in general (21). Regarding dietary behaviors, some studies have found that health literacy motivates individuals to make healthier dietary food choices (22, 23), but others have demonstrated the reverse effect (18). The role of health literacy in the promotion of dietary behavior of the Chinese residents remains to be clarified. Functional health literacy (FHL) is the most common type of health literacy assessment, and this study is based on the FHL scale to evaluate individuals’ capacity to access, understand, and apply health information (24–26).
Priority intervention populations were identified by examining differences in nutrition knowledge, health literacy, and dietary behavior between groups. Previous studies have indicated that FHL, nutrition knowledge, and dietary behavior are affected by demographic characteristics. Characteristics such as gender (27), age (28), region (urban or rural) (29), occupation (30), education level, household income, and self-reported health status (31) were found to affect health literacy. Xu et al. (32) found that significant differences in nutritional knowledge across demographic characteristics, which in turn influenced their dietary habits and health. Education level, gender, BMI, and exposure to chronic disease were found to influence individuals’ nutritional knowledge and dietary behavior (32–35). To identify priority intervention populations in a multi-dimensional way, a wide range of demographic variables, including gender, age, education, marital status, income, body mass index (BMI), and chronic disease status, were selected to identify high-risk groups.
In summary, this study collected data on the nutrition knowledge, FHL, and dietary behavior of rural residents in China to serve three objectives: (1) to assess the current dietary behavior, FHL, and nutrition knowledge of rural residents in China; (2) to examine the differences in dietary behavior, FHL, and nutrition knowledge between different populations; and (3) to analyze the relationship between health literacy and nutrition knowledge and dietary behavior. This study provides guidelines for determining the priority intervention information and populations for healthy dietary intervention programs in rural China. Figure 1 shows the research framework.
2 Materials and methods
2.1 Questionnaire design
To investigate the nutrition knowledge, FHL, and dietary behavior, we designed a questionnaire for the target population. The questionnaire in this study had four parts: (1) demographic characteristics; (2) nutrition knowledge; (3) FHL; and (4) a food frequency questionnaire (FFQ). Demographic characteristics included gender, age, marital status, education level, annual household income, BMI, and chronic disease status. Nutrition knowledge included the PNK and DNK, and their items are from the studies of Dickson-Spillmann et al. (36) and Ju (37), respectively. The form of categorical judgment was chosen as the response to the nutrition knowledge, and all nutrition knowledge questions had only one correct answer. FHL included information access capacity (IACC), information understanding capacity (IUC), and information application capacity (IAPC). We selected the FHL questions from the health literacy questionnaire proposed by Osborne et al. (38) and FHL was measured using a five-point Likert scale. The original PNK and FHL questions were written in English. The questions were translated into Chinese, and two bilingual experts on dietary behavior corrected the semantics of the questionnaire and ensured translation equivalence. An initial set of items was formed consisting of seven items for measuring PNK, six items for measuring DNK, and nine items for measuring FHL.
The FFQ was used as the dietary intake questionnaire to investigate food consumption frequency and intake in the past week in the subject. Food was divided into five categories (i.e., meat, poultry, fish, and eggs; dairy and beans; grain; fruit; and vegetable), and specific food names were listed in every category as a reference for the subject to fill in the food consumption frequency and food intake. To increase the suitability of the questionnaire, the types of food consumed with high frequency in the survey area were pre-survey by a group of 30 rural residents. The 24 most commonly consumed foods were listed in the food frequency questionnaire, and a blank area was present for the subject to add in foods that were not mentioned. Two forms were used for food consumption frequency. Foods with a high consumption frequency were written as “X times/day,” and foods with a low consumption frequency were written as “W times/week (7 days).” The question was not filled in if the food was not consumed in the past week. When filling in the food intake, the schematic diagram of standard food weight provided by the investigator was used to estimate the weight of the food consumed each time. See Appendix for schematic diagram of standard food weight (Supplementary Figure S1) and dietary intake questionnaire (Supplementary Table S1).
Three instruments, i.e., content validity, internal consistency, and test–retest reliability, were applied to evaluate the reliability of the questionnaire. First, an expert group was organized to assess the content validity of the questionnaire. The group is composed of two professors, one assistant professor, and three doctoral candidates, whose research areas are focused on nutrition and consumer behavior. Explain to the expert group the study objectives, questionnaire content, and assessment procedure via email or WeChat (a kind of instant messaging social software), and invite participation after receiving consent (39). The assessment included question clarity and understanding, relationship to the study objective, overlap between questions, answer rationality, and conformance to China’s context. In the light of the expert group’s comments, we deleted some questions that were not clearly stated. For example, one item which asked whether it was unhealthy to eat an excessive proportion of fruits and vegetables in the daily diet was deleted because it mentioned the intake of both fruits and vegetables, which is a double-barrelled question, and this may have confused respondents. Some questions were optimized, i.e., the item “A balanced diet is one that has the same intake of all nutrients” was modified to “A balanced diet is one that has the same intake of all types of food,” as nutrients are academic jargon that are difficult to understand for rural residents. Subsequently, a preliminary study was carried out on 30 rural residents. Each respondent completed the questionnaire from 30 to 45 min. We used Cronbach’s α coefficient to assess the internal consistency. Cronbach’s α coefficient of FHL (including information access capacity, information understanding capacity, and information application capacity) was above 0.8, and the Cronbach’s α coefficients of PNK and DNK were 0.597 and 0.538, respectively. In some studies involving the internal consistency of the items, especially for knowledge items, it was considered acceptable if it was close to the threshold (α = 0.6) (40, 41). Nunnally (42) first version of the introduction to Cronbach’s α coefficients suggested that the minimum accepted ranges in preliminary studies were 0.5 to 0.6. Thus, the internal consistency of items measuring the FHL, PNK, and DNK is acceptable. To further evaluate the stability of the questionnaire over time, 20 subjects were randomly selected for a telephone interview 3 days after the first investigation, and 10 questions in the questionnaire were randomly selected for the interviewee to answer. The two investigations were conducted 3 days apart to decrease memory bias, and 10 questions were randomly selected to reduce sequence bias. The Pearson correlation coefficient of the two scores was 0.86 (p < 0.01), showing that the questionnaire has high test–retest reliability.
2.2 Data collection
2.2.1 Survey site
Three provinces in northeastern China, which are Heilongjiang, Jilin, and Liaoning, were selected as survey areas. Northeastern China has the highest latitude and cold climate, and these three provinces are the main grain-producing regions of China and are major agricultural provinces, which have shaped unique dietary patterns. The daily diet of residents in northeastern China contained excess salt, and long-term high salt intake is an important factor causing chronic diseases (43, 44). Moreover, due to the long and cold winters in northeastern China, residents usually consume grains and meat with high calorific value to withstand the cold weather, leading to a lower proportion of fresh fruits and vegetables in their diet. Hu et al. (45) found that increasing fruit and vegetable intake in northeastern China could decrease the risk of lung cancer. Considering the high health risks associated with this unique dietary pattern, there is a great tendency to conduct healthy eating interventions in these regions.
2.2.2 Questionnaire survey
Ten investigators were recruited to conduct the survey. The survey was carried out in the form of face-to-face interviews. To ensure the credibility and reliability of the survey, training for investigators was implemented. The training included an introduction to the purpose of the survey, an explanation of the meaning of each question and how it should be answered, procedures for conducting the survey, and tips for dealing with unexpected situations that they may encounter during face-to-face interviews. The survey was conducted in the homes of the respondents. To ensure the representativeness of the sample, ten villages in three provinces were selected as survey locations by stratified random sampling. The sampling pattern is to select one city in each province and the corresponding village in each city. As Liaoning Province has the largest number of residents living in rural areas, four villages were selected in Liaoning Province and three villages were selected in the other two provinces. Forty residents in each village were recruited to conduct the survey. To increase randomness, one family was chosen to visit in every geographically separated 3 households. The questionnaire survey was completed from June to August 2019.
Based on the Bartlett et al. (46) formula shown below, the required sample size was estimated to be 323. Given the non-response rate, a total of 400 questionnaires were distributed (Eq. 1).
where n denotes the required sample size, d denotes a 5% margin with error (the standard value = 0.05), Z denotes a 95% confidence interval , p denotes the proportion of the target population (p = 0.7), and q denotes 1 − p (q = 0.3). N represents the population size, which, according to the 2018 statistics, is 34.84 million rural residents in the three provinces of Northeast China, i.e., N = 348,400,000.
Three hundred and seventy questionnaires were returned, of which 344 were valid questionnaires with no missing values, with a response rate of 92.5% (370/400) and a validity rate of 93.0% (344/370). Three hundred and forty-four valid samples are above the threshold number of required sample size (n = 323).
2.3 Data analysis
Amos (version 22.0) and SPSS (version 23.0) were applied for statistical analysis. The frequency (count and percentage) of every option was calculated, and the mean score and standard deviation (S.D.) of every question was calculated. Kurtosis and skewness were used to test the normality of the variables. It is considered that the variables are normally distributed when the absolute values of kurtosis index and skewness index are less than 7 and 2, respectively (47). ANOVA and t-test was used to compare the differences when data were normally distributed and variance was homogeneous. In other situations, nonparametric tests (Mann–Whitney U test or Kruskal–Wallis test) were used. Ordinary least squares (OLS) were used to analyze the relationship between nutrition knowledge, FHL, and dietary behavior. Variance inflation factor (VIF) was applied to check the multicollinearity of each fitted model (48).
For knowledge questions, 1 point and 0 point were given for a correct and incorrect answer, respectively. The PNK score was from 0 to 7 and the DNK score was from 0 to 6, and total nutrition knowledge was from 0 to 13. The five-point Likert scale was scored ranging from one to five. The FHL scale contains 9 items with scores ranging from 9 to 45. The three subscales, IACC, IUC, and IAPC, each contained three items scored from 3 to 15. Referring to Nakayama et al. (49), four levels of health literacy were defined based on average FHL scores: 9–23 as inadequate, 24–31 as problematic, 32–38 as sufficient, and 39–45 as excellent.
The scientific community has given diverse instruments for diet assessment from different perspectives. The Dietary Quality Index-International (DQI-I), one of the composite measures that enable a more precise identification of the relationship between overall diet quality scores and the risk of diet-related diseases, was adopted because it is considered a valid tool to make comparisons across countries and regions (50–52). Notably, the DQI-I in the study was calculated depending on the intake of the five food groups in accordance with the recommended values without reference to micronutrients and macro-nutrients. The reason for this calculation format is that the dietary pattern recommended by the Chinese Dietary Guidelines mainly focuses on the intake of these five food groups, and that the adequate intake of these five food groups may, to a certain extent, meet the body’s needs for various types of nutrients and reduce chronic diseases (53). The first category (meat, poultry, fish, and eggs) included eight types of foods; the second category (dairy and beans) included five types of foods; the third category (grain) included seven types of foods; the fourth category (fruit) included six types of foods; and the fifth category (vegetable) included six types of foods. The daily intake for each food category ( ) was equal to the sum of all food intake under that category. Refer to Eqs. (2–6). For high consumption frequency food, the respondent would write the number of times the food was consumed each day ( ) and the amount of each intake ( ). For less consumption frequency food, the respondent would write the number of times the food was consumed each week ( ) and the amount of each intake ( ), yi is the daily intake of each category of food:
The 2022 Chinese Dietary Guideline recommends that the recommended intake for five food categories (i.e., meat, poultry, fish, and eggs; dairy and beans; grain; fruit; and vegetable) is 150 g/day, 300 g/day, 250 g/day, 200 g/day, and 300 g/day, respectively. If the intake of a category was lower than the recommended intake; it was scored as 0; otherwise, it was scored as 3. The range of DQI-I is from 0 to 15.
3 Results
3.1 Profile of samples
The sample characteristics of the survey is shown in Table 1. There were slightly more female respondents (57%) in the sample than male respondents (43%), 59% of respondents were between the ages of 35 and 54 years old, nearly 50% of the respondents having an annual household income range from RMB 36,000–84,000, equivalent to US$ 4,956–11,565.1 The proportion of married respondents (73%) in the sample is substantial, and more than 50% of the respondents have a junior high school education and below, which is consistent with the lack of young and well-educated people in rural China (54). A significant proportion of the sample, about 36%, were suffering from chronic diseases, and more than 50% of the respondents were not at a healthy weight, which is consistent with the results of previous studies that suggest that the health status of rural residents in Northeast China is a matter of concern (55).
3.2 Descriptive data analysis results
As shown in Table 2, the mean PNK score was 4.66 (range from 0 to 7), and the question with the lowest accuracy rate was meat should be the basis of our daily diet (45.9%), which the highest accuracy rate was fat is always bad for your health, so you should avoid it as much as possible (70.6%). The mean DNK score was 2.53 points (range from 0 to 6), and the question with the lowest accuracy rate was daily salt intake should not exceed (27.6%), which the question with the highest accuracy rate was which of the following food groups contains the most protein (65.7%). The mean score of total nutritional knowledge was 7.19 (range from 1 to 13).
As shown in Table 3 and Figure 2, the average FHL score was 26.52 (range from 9 to 45, S.D. = 6.49), with 80% of respondents (n = 275) having a health literacy status of inadequate/problematic, and only 3% of respondents (n = 9) having excellent health literacy. For the three sub-structures of the FHL, the highest mean score was IACC (9.78 ± 3.55), followed by the IUC (9.19 ± 3.05), with the lowest mean score for the IAPC (7.55 ± 2.81). The question with the highest mean score was I can obtain the healthy diet information that I need from text search (3.35 ± 1.27), and the question with the lowest mean score was I have sufficient healthy diet information to manage my diet (2.49 ± 1.13).
As shown in Table 4, the mean DQI-I score was 10.37 (range from 0 to 15). In particular, the proportion of respondents whose average daily intake of food categories met the recommended values at least four or more 50.87%. The percent of respondents whose average daily grain intake meet the recommendations was the highest, at 87.8%. While, only 55% of respondents ate the recommended amount of fruits and 62.8 and 67.7% of respondents ate the recommended amount of dairy and beans, and vegetable, respectively.
3.3 Difference analysis under different demographic variables
As shown in Table 5, the difference analysis of dietary knowledge of rural residents under different populations revealed that the overall nutrition knowledge level differed significantly by gender, annual household income, and education level. The PNK level of the residents varies as a function of the annual household income and education level. Three demographic dimensions, age, annual household income and education level, are important indicators for determining the different DNK levels of the residents.
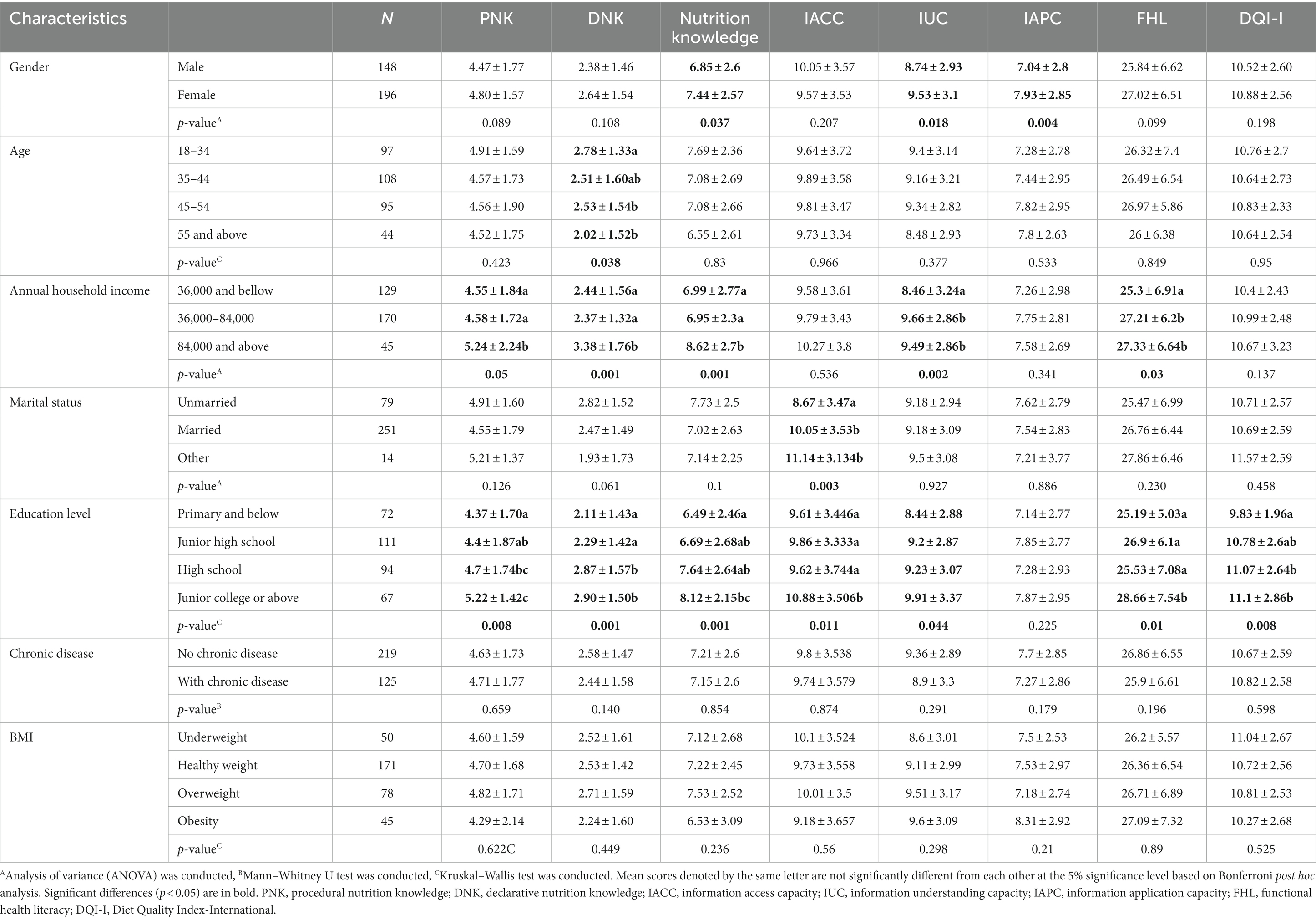
Table 5. Relationship between demographic characteristics and nutrition knowledge, functional health literacy, and dietary behavior.
For the health literacy status of rural residents, the overall FHL varies mainly by annual household income and education level. Regarding the three sub-dimensions of FHL, there are also differences in their influencing factors. Gender was identified as shaping the differences in residents’ IUC and IAPC. The education level contributes to the different IACC and IUC. Moreover, residents’ IUC and IACC are influenced by annual household income and marital status, respectively.
We identified significant differences in dietary variety between different education levels, that is, the higher the education level, the healthier the dietary behavior. The average DQI-I score for individuals in high school and above was over 11, while the average score for individuals in primary school and below was less than 10.
The results of the above analysis showed that male, elder, low-income, unmarried, and low-education groups performed significantly worse than their counterparts on one or more dimensions of knowledge, health literacy, and dietary behavior. Of these, male is showing significantly worse performance than female in three dimensions, namely nutrition knowledge, IUC, and IAPC. The 55 and older group performs significantly worse than the younger group in one dimension, i.e., DNK. The high-income group performs significantly better than the low-income group in five dimensions, i.e., PNK, DNK, nutrition knowledge, IUC, and FHL. The married group performs significantly better than the unmarried group in one dimension, i.e., IACC. Except for IAPC, the low-educated group was significantly worse than the high-educated group in the remaining dimensions. Given these findings, male, older adult, low-income, unmarried, and low-education groups are considered high-risk groups in terms of healthy diet and warrant prioritization in the design of intervention programs.
3.4 Relationship between nutrition knowledge and health literacy with dietary variety
Table 6 shows the results of the regression analysis. The VIF values were all below 1.5, indicating that there was no multicollinearity. The regression results showed that nutrition knowledge and health literacy could explain 31.5% of variance in dietary behavior. IACC, IUC, IAPC, and PNK significantly and positively affected DQI-I. Of these, the effects of IACC (β = 0.291) and IAPC (β = 0.277) on dietary behavior were the most influential. The coefficient of DNK on DQI-I was positive but not significant. After adding age, marital status, gender, annual household income, and education level as control variables, the effect of the above variables on DQI-I remains significant, showing that the test results are robust. The effects of control variables on dietary behavior were not significant.
4 Discussion
In this study, a validated questionnaire was developed to measure the nutrition knowledge (including PNK and DNK), FHL, and DQI-I of rural residents in northeastern China. Based on survey data through face-to-face approach, we were informed about the baseline status of dietary knowledge, health literacy and dietary behavior of rural residents. By examining the differences in different demographic characteristics, high-risk groups with poorer performance in terms of knowledge, literacy, and behavior were identified. In addition, factors associated with dietary behavior were also uncovered by evaluating the effect of dietary knowledge and health literacy. Based on these results, we were able to give evidence-based guidance on the priority content and populations for healthy dietary interventions.
4.1 The picture of consumers’ nutrition knowledge, FHL, and dietary behavior
The study showed the average correct scores of PNK, DNK and overall knowledge scores of rural residents in the three northeastern provinces were 66.57% (4.66/7), 42.17% (2.53/7) and 55.31% (7.19/13), respectively. This indicates that the level of nutritional knowledge of the residents is not sufficient and needs to be further promoted. This is consistent with the outcomes of other studies on Chinese residents, e.g., Hou et al. (56) and Zhang et al. (57). The relationship analysis between dietary knowledge and behavior showed that PNK had a positive effect on dietary behavior, while DNK had a non-significant effect on dietary behavior, indicating that different types of knowledge showed different effects on behavior and that the priority of different types of knowledge should be weighed in designing intervention programs.
The results of this study suggest that improvements in behavior may be insignificant if the intervention is targeted to residents’ descriptive knowledge. Previous studies that found a weak relationship between dietary knowledge and behavior may also have resulted from a failure to distinguish between different types of knowledge (58–60). Accordingly, integrating content related to procedural knowledge in intervention information development is an effective way to bridge the knowledge–behavior gap in dietary interventions.
The FHL score was generally low, and only 3% of the respondents showed excellent health literacy. This result is significantly lower than the health literacy of the residents of Denmark (35.2 ± 4.0, rang from 9 to 40) (61), Australia (Mean = 30.31, range from 9 to 40) (62) and Netherlands (Mean = 30.06, range from 9 to 40) (63). Li et al. (64) surveyed the Chinese population and found that the proportion of urban residents with adequate health literacy was 24%, while the proportion of the rural population with adequate health literacy in this study was only 20% (17% sufficient and 3% excellent). These suggest that the health literacy of Chinese rural residents is poor and needs to be improved. Furthermore, the ability to apply information to manage dietary was the lowest. FHL was evidenced to be significantly associated with the residents’ dietary behavior. Specifically, residents with stronger IACC, IUC, and IAPC had higher DQI-I scores. This is consistent with the findings of a systematic review, which showed that FHL was the most important predictor variable of dietary behavior (65). Some studies also proved that health literacy was a predictor of fruit and vegetable intake (66, 67). This signifies that diet-related health literacy is the priority to be addressed when designing intervention programs, including improving individuals’ ability to access, understand, and apply health information.
The dietary behavior met the recommendations (score = 15) accounted for only 15.41% of the residents, and nearly 50% of the individuals were those who had two food categories with less than the recommended intake. Among them, there was a high proportion (87.8%) of participants that met the recommended grain intake value. The reason for this phenomenon is that northeastern China is a major food-producing region where farmers mainly grow rice. The cost for farmers to obtain grain through self-sufficiency is relatively low. Besides, the proportion of fruits, dairy products and beans, and vegetables consumed up to the recommended values is quite low. Previous studies have also found that Chinese consumers’ intake of these food groups is grossly inadequate (68, 69). Fruits and vegetables can provide dietary fiber, which is strongly associated with a low incidence of cardiovascular disease and obesity (70). This implies that there is a great urgency to improve the dietary patterns of the Chinese residents, and intervention information should focus on increasing the population’s intake of specific foods (e.g., fruits, vegetables, beans, and dairy products).
4.2 Conditions under different demographic characteristics
This study revealed significant differences in dietary knowledge, health literacy, and behavior across demographic variables, which provides the empirical evidence for identifying high-risk groups in relation to healthy diets. One of the significant factors contributing to the differences in nutritional knowledge and FHL was gender. Females have more nutritional knowledge, information understanding and information application capacity than males. Dickson-Spillmann et al. (36) also found that PNK was significantly higher in females than in males. However, some previous studies found no significant relationship between health literacy by gender (71, 72) or that males have higher health literacy than females (73). The reason for this different outcome may be that the dietary decision makers in Chinese households are generally females, who spend more time and energy on food choices and therefore generate more health literacy than males (74). This also suggests that Chinese males are poor performers in terms of health behaviors and are a priority group for dietary interventions.
Age affects DNK, i.e., the elder the respondent, the lower the DNK, which is consistent with previous studies concluding that age is negatively associated with nutritional knowledge (36), but this differs from Hendrie, Coveney and Cox (75). These differences may be due to the fact that the questionnaire items are according to the latest healthy dietary recommendation guidelines, which differ from the past versions. Access to up-to-date knowledge can be a challenge for the elder community (76). Accordingly, this points to the elder population as a priority group for dietary interventions.
Previous studies have shown that residents with higher incomes have access to health information and services (67, 77, 78). Our study also found significant differences in nutritional knowledge and information understanding capacity between income levels, that is, those with higher incomes generally had more nutritional knowledge, including PNK and DNK, and higher health literacy. While lower income groups are the ones that need more attention in dietary interventions owing to poor performance in terms of knowledge and health literacy.
It has been shown that higher education levels not only help individuals to acquire knowledge and skills, but also enable them to better translate this information into health literacy (79, 80). Our findings also validated this finding and uncovered that those with higher education levels showed better in terms of dietary behavior, which is consistent with the Yang et al. (81). Furthermore, Kristal et al. (82) pointed to a relationship between the effectiveness of the dietary intervention and the years of education of the subjects. The educational level has been confirmed in several studies to have a significant positive effect on health behaviors (83, 84). In light of this study’s findings, individuals with lower education levels as a high-risk group deserve priority treatment in dietary intervention.
Marital status would affect the residents’ health literacy, mainly in the sense that married individuals have better ability to receive health information than unmarried ones. The reason for this may be that married individuals invest more energy in their family life, including paying attention to their family’s health, which leads these individuals to be more willing to obtain health information. This also means that unmarried residents need increased focus in dietary interventions.
4.3 Implications for designing dietary intervention
4.3.1 Implications for designing intervention information
By investigating the nutrition knowledge, FHL, and dietary behavior of rural residents in northeastern China and analyzing the relationships between these factors, this study identifies priority intervention information and populations for health dietary promotion programs. We found that the nutrition knowledge, FHL, and dietary behavior is not well developed and needs to be improved. Among them, PNK and FHL have significant positive effects on dietary variety. In order to more effectively promote healthy dietary behaviors, the intervention information needs to involve knowledge of how to more efficiently and rationally implement healthy dietary and improve individual health literacy. Dietary intervention programs in China now mostly introduce residents to what constitutes a healthy diet, i.e., DNK, which helps improve DNK but has limited effect on behavior improvement. Developing Internet and mobile phone app–based dietary guidelines is also essential to decrease the difficulty of accessing, understanding, and applying information.
4.3.2 Implications for targeting high-risk population groups
Moreover, by understanding the performance of individuals under different demographic characteristics it is also possible to identify priority populations for intervention. Groups that are male, older adult, low-income, unmarried, and low education levels should be given more attention because they perform worse in terms of knowledge, health literacy, and dietary behaviors. The number of mobile Internet users in China is nearly one billion (85), which allows the use of big data technology to push healthy diet information to priority groups.
4.4 Limitations and future studies
Although we have made an in-depth consideration of the research design there are still some limitations in this study. First, we selected only DQI-I as a marker for dietary behavior assessment. Other markers could be added for dietary behavior assessment in future studies, such as the proportion of macro-energy supply and micronutrient intake. Second, this study focused only on nutritional knowledge and FHL as influencing factors of dietary behavior. However, in previous studies it has also been revealed that there are other factors that may have influenced dietary behaviors, such as social network type, self-efficacy, and food availability (86, 87). In coming studies, psychosocial models and environmental factors could be considered to evaluate dietary behavior. Additionally, this study used the FFQ as an instrument to investigate dietary behavior. Although this measurement method is low-cost and valid, its accuracy is slightly lacking. More accurate survey tools, such as logs or real-time images, can be chosen to record dietary behaviors. Finally, we only surveyed the northeastern region of China, and although the northeastern region is quite representative, a national survey could be considered in the future to get a clearer picture of the dietary situation of Chinese residents.
5 Conclusion
This study showed that the dietary behavior, nutrition knowledge, and health literacy of rural residents in China need to be improved. FHL is an important factor affecting dietary behavior, and more attention should be paid to this issue in dietary behavior interventions. As a different type of nutrition knowledge, procedural nutrition knowledge showed a significant positive influence on dietary behavior, whereas declarative nutrition knowledge was not significantly influenced with dietary behavior. The promotion of procedural nutrition knowledge should be reinforced in the promotion of healthy diets going forward. The results of differences analysis demonstrated that demographic variables affect individual nutritional knowledge, health literacy, and dietary behavior and identified males, older adult, unmarried, low-income, and low-education populations as high-risk group. This provides evidence-based guidance for prioritizing information and populations for healthy dietary interventions.
Data availability statement
The raw data supporting the conclusions of this article will be made available by the authors, without undue reservation.
Ethics statement
The studies involving humans were approved by School of Biological and Agricultural Engineering, Jilin University. The studies were conducted in accordance with the local legislation and institutional requirements. The participants provided their written informed consent to participate in this study.
Author contributions
LB: Conceptualization, Data curation, Formal analysis, Methodology, Validation, Writing – review & editing. HT: Resources, Formal analysis, Investigation, Writing – original draft. MW: Supervision, Conceptualization, Writing – review & editing, Funding acquisition. All authors contributed to the article and approved the submitted version.
Funding
This work was supported by the National Natural Science Foundation of China, China [grant numbers: 72203073], Humanities and Social Science Fund of Ministry of Education of China [grant numbers: 22YJCZH175; 23YJAZH003], and project funded by China Postdoctoral Science Foundation [grant numbers: 2022M711304; 2023T160267].
Conflict of interest
The authors declare that the research was conducted in the absence of any commercial or financial relationships that could be construed as a potential conflict of interest.
Publisher’s note
All claims expressed in this article are solely those of the authors and do not necessarily represent those of their affiliated organizations, or those of the publisher, the editors and the reviewers. Any product that may be evaluated in this article, or claim that may be made by its manufacturer, is not guaranteed or endorsed by the publisher.
Supplementary material
The Supplementary material for this article can be found online at: https://www.frontiersin.org/articles/10.3389/fpubh.2024.1239449/full#supplementary-material
Footnotes
1. ^On November 1, 2022, 1 U.S. dollar equals approximately 7.264 RMB.
References
1. Bu, T, Tang, D, Liu, Y, and Chen, D. Trends in dietary patterns and diet-related behaviors in China. Am J Health Behav. (2021) 45:371–83. doi: 10.5993/AJHB.45.2.15
2. Wang, Y, Xu, L, Wang, N, Zhu, L, Zhao, F, Xu, K, et al. Associations of dietary patterns and incident type 2 diabetes in a community population cohort from Southwest China. Front Public Health. (2022) 10:22. doi: 10.3389/fpubh.2022.773172
3. Pan, XF, Wang, L, and Pan, A. Epidemiology and determinants of obesity in China. Lancet Diabetes Endocrinol. (2021) 9:373–92. doi: 10.1016/S2213-8587(21)00045-0
4. Huang, L, Wang, Z, Wang, H, Zhao, L, Jiang, H, Zhang, B, et al. Nutrition transition and related health challenges over decades in China. Eur J Clin Nutr. (2021) 75:247–52. doi: 10.1038/s41430-020-0674-8
5. Mu, T, Xu, R, Zhu, Q, Chen, L, Dong, D, Xu, J, et al. Diet-related knowledge, attitudes, and behaviors among young and middle-aged individuals with high-normal blood pressure: a cross-sectional study in China. Front Public Health. (2022) 10:898457. doi: 10.3389/fpubh.2022.898457
6. The State Council of China. (2016). Outline of the Plan for Health China 2030. Available at: http://www.gov.cn/gongbao/content/2016/content_5133024.htm (Accessed October 25, 2016)
7. The State Council of China. (2017). National Nutrition Program 2017–2030. Available at: http://www.gov.cn/zhengce/content/2017-07/13/content_5210134.htm (Accessed June 30, 2017)
8. Shilian, H, Jing, W, Cui, C, and Xinchun, W. Analysis of epidemiological trends in chronic diseases of Chinese residents. Aging Med. (2020) 3:226–33. doi: 10.1002/agm2.12134
9. Evans, M, Yu, S, Song, B, Deng, Q, Liu, J, and Delgado, A. Building energy efficiency in rural China. Energy Policy. (2014) 64:243–51. doi: 10.1016/j.enpol.2013.06.040
10. Cui, B, Wang, LDL, Wang, FR, Peng, J, Ma, JY, Chen, X, et al. Correlation between dietary information sources and knowledge of adequate diets in eastern China. Front Public Health. (2022) 10:955766. doi: 10.3389/fpubh.2022.955766
11. Wang, M, Huang, L, Liang, X, and Bai, L. Consumer knowledge, risk perception and food-handling behaviors–a national survey in China. Food Control. (2021) 122:107789. doi: 10.1016/j.foodcont.2020.107789
12. Alami, A, Tavakoly Sany, SB, Lael-Monfared, E, Ferns, GA, Tatari, M, Hosseini, Z, et al. Factors that influence dietary behavior toward iron and vitamin D consumption based on the theory of planned behavior in Iranian adolescent girls. Nutr J. (2019) 18:1–9. doi: 10.1186/s12937-019-0433-7
13. Roth, SE, Gill, M, Chan-Golston, AM, Crespi, CM, Albert, SL, Rice, LN, et al. Nutrition campaign knowledge and dietary behavior in middle school students. Californian J Health Promot. (2018) 16:1–10. doi: 10.32398/cjhp.v16i2.2086
16. Carbonneau, E, Lamarche, B, Provencher, V, Desroches, S, Robitaille, J, Vohl, MC, et al. Associations between nutrition knowledge and overall diet quality: the moderating role of sociodemographic characteristics—results from the PREDISE study. Am J Health Promot. (2021) 35:38–47. doi: 10.1177/0890117120928877
17. Dickson-Spillmann, M, and Siegrist, M. Consumers’ knowledge of healthy diets and its correlation with dietary behaviour. J Hum Nutr Diet. (2011) 24:54–60. doi: 10.1111/j.1365-277X.2010.01124.x
18. Buja, A, Grotto, G, Montecchio, L, De Battisti, E, Sperotto, M, Bertoncello, C, et al. Association between health literacy and dietary intake of sugar, fat and salt: a systematic review. Public Health Nutr. (2021) 24:2085–97. doi: 10.1017/S1368980020002311
19. Nutbeam, D. Health literacy as a public health goal: a challenge for contemporary health education and communication strategies into the 21st century. Health Promot Int. (2000) 15:259–67. doi: 10.1093/heapro/15.3.259
20. Rasu, RS, Bawa, WA, Suminski, R, Snella, K, and Warady, B. Health literacy impact on national healthcare utilization and expenditure. Int J Health Policy Manag. (2015) 4:747–55. doi: 10.15171/ijhpm.2015.151
21. Anne Katz, RN. Health literacy: what do you know? Oncol Nurs Forum. (2017) 44:521–2. doi: 10.1188/17.ONF.521-522
22. Cha, E, Kim, KH, Lerner, HM, Dawkins, CR, Bello, MK, Umpierrez, G, et al. Health literacy, self-efficacy, food label use, and diet in young adults. Am J Health Behav. (2014) 38:331–9. doi: 10.5993/AJHB.38.3.2
23. Oberne, A, Vamos, C, Wright, L, Wang, W, and Daley, E. Does health literacy affect fruit and vegetable consumption? An assessment of the relationship between health literacy and dietary practices among college students. J Am Coll Heal. (2022) 70:134–41. doi: 10.1080/07448481.2020.1727911
24. Baker, DW, Williams, MV, Parker, RM, Gazmararian, JA, and Nurss, J. Development of a brief test to measure functional health literacy. Patient Educ Couns. (1999) 38:33–42. doi: 10.1016/S0738-3991(98)00116-5
25. Murphy, PW, Davis, TC, Long, SW, Jackson, RH, and Decker, BC. Rapid estimate of adult literacy in medicine (REALM): a quick reading test for patients. J Read. (1993) 37:124–30.
26. Parker, RM, Baker, DW, Williams, MV, and Nurss, JR. The test of functional health literacy in adults. J Gen Intern Med. (1995) 10:537–41. doi: 10.1007/BF02640361
27. Amoah, PA, and Phillips, DR. Socio-demographic and behavioral correlates of health literacy: a gender perspective in Ghana. Women Health. (2020) 60:123–39. doi: 10.1080/03630242.2019.1613471
28. Xu, X, Parker, D, Shi, Z, Byles, J, Hall, J, and Hickman, L. Dietary pattern, hypertension and cognitive function in an older population: 10-year longitudinal survey. Front Public Health. (2018) 6:201. doi: 10.3389/fpubh.2018.00201
29. Nie, X, Li, Y, Li, LI, and Huang, X. A study on health information literacy among urban and suburban residents in six provinces in China. Zhonghua Yu Fang Yi Xue Za Zhi. (2014) 48:566–70. doi: 10.3760/cma.j.issn.0253-9624.2014.07.007
30. Wang, X, Guo, H, Wang, L, Li, X, Huang, M, Liu, Z, et al. Investigation of residents’ health literacy status and its risk factors in Jiangsu Province of China. Asia Pac J Public Health. (2015) 27:NP2764–72. doi: 10.1177/1010539513487012
31. Chen, W, Ren, H, Wang, N, Xiong, Y, and Xu, F. The relationship between socioeconomic position and health literacy among urban and rural adults in regional China. BMC Public Health. (2021) 21:1–10. doi: 10.1186/s12889-021-10600-7
32. Xu, Y, Zhu, S, Zhang, T, Wang, D, Hu, J, Gao, J, et al. Explaining income-related inequalities in dietary knowledge: evidence from the China health and nutrition survey. Int J Environ Res Public Health. (2020) 17:532. doi: 10.3390/ijerph17020532
33. Geaney, F, Fitzgerald, S, Harrington, JM, Kelly, C, Greiner, BA, and Perry, IJ. Nutrition knowledge, diet quality and hypertension in a working population. Prev Med Rep. (2015) 2:105–13. doi: 10.1016/j.pmedr.2014.11.008
34. O’brien, G, and Davies, M. Nutrition knowledge and body mass index. Health Educ Res. (2007) 22:571–5. doi: 10.1093/her/cyl119
35. Post, RE, Mainous, AG III, Diaz, VA, Matheson, EM, and Everett, CJ. Use of the nutrition facts label in chronic disease management: results from the national health and nutrition examination survey. J Am Diet Assoc. (2010) 110:628–32. doi: 10.1016/j.jada.2009.12.015
36. Dickson-Spillmann, M, Siegrist, M, and Keller, C. Development and validation of a short, consumer-oriented nutrition knowledge questionnaire. Appetite. (2011) 56:617–20. doi: 10.1016/j.appet.2011.01.034
37. Ju, Y. Investigation on knowledge, attitude and behavior among university students. Mod Prevent Med. (2012) 39:2502–3.
38. Osborne, RH, Batterham, RW, Elsworth, GR, Hawkins, M, and Buchbinder, R. The grounded psychometric development and initial validation of the health literacy questionnaire (HLQ). BMC Public Health. (2013) 13:1–17. doi: 10.1186/1471-2458-13-658
39. DiIorio, CK. Measurement in Health Behavior: Methods for Research and Evaluation. New Jersey: John Wiley & Sons (2006).
40. Fornell, C, and Larcker, DF. Evaluating structural equation models with unobservable variables and measurement error. J Mark Res. (1981) 18:39–50. doi: 10.1177/002224378101800104
41. Uggioni, PL, and Salay, E. Reliability and validity of a questionnaire to measure consumer knowledge regarding safe practices to prevent microbiological contamination in restaurants. J Nutr Educ Behav. (2013) 45:250–7. doi: 10.1016/j.jneb.2011.09.007
43. Du, Z, Xing, L, Liu, S, Jing, L, Tian, Y, Zhang, B, et al. Prevalence and determinants of metabolic syndrome based on three definitions in rural Northeast China. Public Health Nutr. (2020) 23:3379–86. doi: 10.1017/S1368980019004166
44. Wang, X, Luan, D, Xin, S, Liu, Y, and Gao, Q. Association between individual components of metabolic syndrome and cognitive function in northeast rural China. Am J Alzheimers Dis Other Dement. (2019) 34:507–12. doi: 10.1177/1533317519865428
45. Hu, J, Johnson, KC, Mao, Y, Xu, T, Lin, Q, Wang, C, et al. A case-control study of diet and lung cancer in Northeast China. Int J Cancer. (1997) 71:924–31. doi: 10.1002/(SICI)1097-0215(19970611)71:6<924::AID-IJC2>3.0.CO;2-#
46. Barlett, JE, Kotrlik, JW, and Higgins, CC. Organizational research: determining appropriate sample size in survey research. Inf Technol Learn Perform J. (2001) 19:43.
47. Kline, RB. Principles and Practice of Structural Equation Modeling. New York: Guilford Publications (2015).
48. Agaku, I, Odani, S, and Nelson, J. Medical use and misuse of psychoactive prescription medications among US youth and young adults. Fam Med Commun Health. (2021) 9:e000374. doi: 10.1136/fmch-2020-000374
49. Nakayama, K, Osaka, W, Togari, T, Ishikawa, H, Yonekura, Y, Sekido, A, et al. Comprehensive health literacy in Japan is lower than in Europe: a validated Japanese-language assessment of health literacy. BMC Public Health. (2015) 15:1–12. doi: 10.1186/s12889-015-1835-x
50. Hann, CS, Rock, CL, King, I, and Drewnowski, A. Validation of the healthy eating index with use of plasma biomarkers in a clinical sample of women. Am J Clin Nutr. (2001) 74:479–86. doi: 10.1093/ajcn/74.4.479
51. Kant, AK. Indexes of overall diet quality: a review. J Am Diet Assoc. (1996) 96:785–91. doi: 10.1016/S0002-8223(96)00217-9
52. Kim, S, Haines, PS, Siega-Riz, AM, and Popkin, BM. The diet quality index-international (DQI-I) provides an effective tool for cross-national comparison of diet quality as illustrated by China and the United States. J Nutr. (2003) 133:3476–84. doi: 10.1093/jn/133.11.3476
53. Zhang, L, Zhou, Y, Wang, P, and Qin, L. Evaluation of Chinese food guide pagoda, 2022. J Environ Occup Med. (2023) 40:1074–8. doi: 10.11836/JEOM23013
54. Liao, L, Long, H, Gao, X, and Ma, E. Effects of land use transitions and rural aging on agricultural production in China’s farming area: a perspective from changing labor employing quantity in the planting industry. Land Use Policy. (2019) 88:104152. doi: 10.1016/j.landusepol.2019.104152
55. Li, Z, Guo, X, Zheng, L, Yang, H, and Sun, Y. Grim status of hypertension in rural China: results from Northeast China rural cardiovascular health study 2013. J Am Soc Hypertens. (2015) 9:358–64. doi: 10.1016/j.jash.2015.02.014
56. Hou, M, Qing, P, and Min, S. Multiple indicators of household dietary diversity in rural China: effects of income and dietary knowledge. Nutrition. (2021) 91–92:111406. doi: 10.1016/j.nut.2021.111406
57. Zhang, J, Xu, AQ, Ma, JX, Shi, XM, Guo, XL, Engelgau, M, et al. Dietary sodium intake: knowledge, attitudes and practices in Shandong Province, China, 2011. PLoS One. (2013) 8:e58973. doi: 10.1371/journal.pone.0058973
58. Rossi, MDSC, Stedefeldt, E, da Cunha, DT, and de Rosso, VV. Food safety knowledge, optimistic bias and risk perception among food handlers in institutional food services. Food Control. (2017) 73:681–8. doi: 10.1016/j.foodcont.2016.09.016
59. Shepherd, R, and Stockley, L. Nutrition knowledge, attitudes, and fat consumption. J Am Diet Assoc. (1987) 87:615–9. doi: 10.1016/S0002-8223(21)03164-3
60. Shepherd, R, and Towler, G. Nutrition knowledge, attitudes and fat intake: application of the theory of reasoned action. J Hum Nutr Diet. (1992) 20:159–69. doi: 10.1111/j.1365-277X.2007.00776.x
61. Riiser, K, Helseth, S, Haraldstad, K, Torbjørnsen, A, and Richardsen, KR. Adolescents’ health literacy, health protective measures, and health-related quality of life during the COVID-19 pandemic. PLoS One. (2020) 15:e0238161. doi: 10.1371/journal.pone.0238161
62. Beauchamp, A, Buchbinder, R, Dodson, S, Batterham, RW, Elsworth, GR, McPhee, C, et al. Distribution of health literacy strengths and weaknesses across socio-demographic groups: a cross-sectional survey using the health literacy questionnaire (HLQ). BMC Public Health. (2015) 15:1–13. doi: 10.1186/s12889-015-2056-z
63. Jansen, T, Rademakers, J, Waverijn, G, Verheij, R, Osborne, R, and Heijmans, M. The role of health literacy in explaining the association between educational attainment and the use of out-of-hours primary care services in chronically ill people: a survey study. BMC Health Serv Res. (2018) 18:1–13. doi: 10.1186/s12913-018-3197-4
64. Li, Z, Tian, Y, Gong, Z, and Qian, L. Health literacy and regional heterogeneities in China: a population-based study. Front Public Health. (2021) 9:603325. doi: 10.3389/fpubh.2021.603325
65. Fleary, SA, Joseph, P, and Pappagianopoulos, JE. Adolescent health literacy and health behaviors: a systematic review. J Adolesc. (2018) 62:116–27. doi: 10.1016/j.adolescence.2017.11.010
66. Guntzviller, LM, King, AJ, Jensen, JD, and Davis, LA. Self-efficacy, health literacy, and nutrition and exercise behaviors in a low-income, Hispanic population. J Immigr Minor Health. (2017) 19:489–93. doi: 10.1007/s10903-016-0384-4
67. Von Wagner, C, Knight, K, Steptoe, A, and Wardle, J. Functional health literacy and health-promoting behaviour in a national sample of British adults. J Epidemiol Community Health. (2007) 61:1086–90. doi: 10.1136/jech.2006.053967
68. Cai, Z, Xian, J, Xu, X, Zhang, Z, Araujo, C, Sharma, M, et al. Dietary behaviours among Han, Tujia and Miao primary school students: a cross-sectional survey in Chongqing, China. Risk Manage Healthc Policy. (2020) 13:1309–18. doi: 10.2147/RMHP.S249101
69. Cheng, G, Duan, R, Kranz, S, Libuda, L, and Zhang, L. Development of a dietary index to assess overall diet quality for Chinese school-aged children: the Chinese children dietary index. J Acad Nutr Diet. (2016) 116:608–17. doi: 10.1016/j.jand.2015.11.010
70. Slavin, JL, and Lloyd, B. Health benefits of fruits and vegetables. Adv Nutr. (2012) 3:506–16. doi: 10.3945/an.112.002154
71. Chang, FC, Chiu, CH, Chen, PH, Miao, NF, Lee, CM, Chiang, JT, et al. Relationship between parental and adolescent eHealth literacy and online health information seeking in Taiwan. Cyberpsychol Behav Soc Netw. (2015) 18:618–24. doi: 10.1089/cyber.2015.0110
72. Chisolm, DJ, Manganello, JA, Kelleher, KJ, and Marshal, MP. Health literacy, alcohol expectancies, and alcohol use behaviors in teens. Patient Educ Couns. (2014) 97:291–6. doi: 10.1016/j.pec.2014.07.019
73. Chang, LC. Health literacy, self-reported status and health promoting behaviours for adolescents in Taiwan. J Clin Nurs. (2011) 20:190–6. doi: 10.1111/j.1365-2702.2009.03181.x
74. Min, S, Peng, J, and Qing, P. Does internet use improve food safety behavior among rural residents? Food Control. (2022) 139:109060. doi: 10.1016/j.foodcont.2022.109060
75. Hendrie, GA, Coveney, J, and Cox, D. Exploring nutrition knowledge and the demographic variation in knowledge levels in an Australian community sample. Public Health Nutr. (2008) 11:1365–71. doi: 10.1017/S1368980008003042
76. Walter, P, Infanger, E, and Mühlemann, P. Food pyramid of the Swiss Society for Nutrition. Ann Nutr Metab. (2007) 51:15–20. doi: 10.1159/000103562
77. Bakkeli, NZ. Income inequality and health in China: a panel data analysis. Soc Sci Med. (2016) 157:39–47. doi: 10.1016/j.socscimed.2016.03.041
78. Wu, Y, Wang, L, Cai, Z, Bao, L, Ai, P, and Ai, Z. Prevalence and risk factors of low health literacy: a community-based study in Shanghai, China. Int J Environ Res Public Health. (2017) 14:628. doi: 10.3390/ijerph14060628
79. Albert, C, and Davia, MA. Education is a key determinant of health in Europe: a comparative analysis of 11 countries. Health Promot Int. (2011) 26:163–70. doi: 10.1093/heapro/daq059
80. Zhu, B, and Ye, Y. Gender disparities in the education gradient in self-reported health across birth cohorts in China. BMC Public Health. (2020) 20:375–11. doi: 10.1186/s12889-020-08520-z
81. Yang, Y, Wang, S, Chen, L, Luo, M, Xue, L, Cui, D, et al. Socioeconomic status, social capital, health risk behaviors, and health-related quality of life among Chinese older adults. Health Qual Life Outcomes. (2020) 18:1–8. doi: 10.1186/s12955-020-01540-8
82. Kristal, AR, Feng, Z, Coates, RJ, Oberman, A, and George, V. Associations of race/ethnicity, education, and dietary intervention with the validity and reliability of a food frequency questionnaire: the Women's health trial feasibility study in minority populations. Am J Epidemiol. (1997) 146:856–69. doi: 10.1093/oxfordjournals.aje.a009203
83. Andriani, H, Rachmadani, SD, Natasha, V, and Saptari, A. Continuity of maternal healthcare services utilisation in Indonesia: analysis of determinants from the Indonesia demographic and health survey. Fam Med Commun Health. (2021) 9:e001389. doi: 10.1136/fmch-2021-001389
84. Park, EY, Oliver, TR, Peppard, PE, and Malecki, KC. Sense of community and mental health: a cross-sectional analysis from a household survey in Wisconsin. Fam Med Commun Health. (2023) 11:e001971. doi: 10.1136/fmch-2022-001971
85. China Internet Network Information Center (CNNIC). (2021). The 47th China Statistical Report on Internet Development.
86. Mills, CM, Keller, HH, DePaul, VG, and Donnelly, C. Nutrition risk varies according to social network type: data from the Canadian longitudinal study on aging. Fam Med Commun Health. (2023) 11:e002112. doi: 10.1136/fmch-2022-002112
Keywords: dietary behavior, health literacy, nutrition knowledge, behavior intervention, health promotion
Citation: Bai L, Tang H and Wang M (2024) Dietary behaviors of rural residents in northeastern China: implications for designing intervention information and targeting high-risk population. Front. Public Health. 12:1239449. doi: 10.3389/fpubh.2024.1239449
Edited by:
Andrea K. Boggild, University of Toronto, CanadaReviewed by:
Jerrald Lau, National University of Singapore, SingaporeMateusz Krystian Grajek, Medical University of Silesia in Katowice, Poland
Copyright © 2024 Bai, Tang and Wang. This is an open-access article distributed under the terms of the Creative Commons Attribution License (CC BY). The use, distribution or reproduction in other forums is permitted, provided the original author(s) and the copyright owner(s) are credited and that the original publication in this journal is cited, in accordance with accepted academic practice. No use, distribution or reproduction is permitted which does not comply with these terms.
*Correspondence: Mingliang Wang, amx1X3dtbEAxNjMuY29t