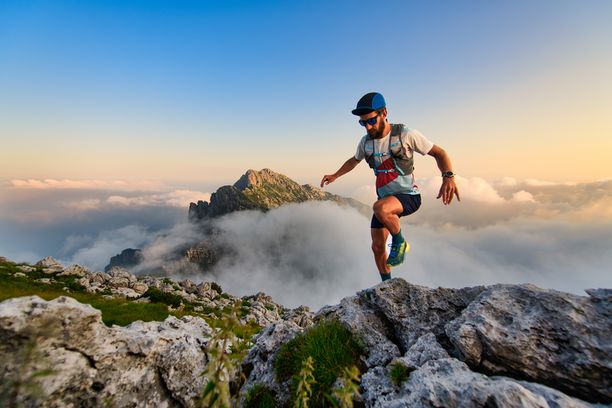
94% of researchers rate our articles as excellent or good
Learn more about the work of our research integrity team to safeguard the quality of each article we publish.
Find out more
MINI REVIEW article
Front. Public Health, 27 November 2023
Sec. Digital Public Health
Volume 11 - 2023 | https://doi.org/10.3389/fpubh.2023.1328918
This article is part of the Research TopicArtificial Intelligence Solutions for Global Health and Disaster Response: Challenges and OpportunitiesView all 26 articles
Millions of people’s health is at risk because of several factors and multiple overlapping crises, all of which hit the vulnerable the most. These challenges are dynamic and evolve in response to emerging health challenges and concerns, which need effective collaboration among countries working toward achieving Sustainable Development Goals (SDGs) and securing global health. Mental Health, the Impact of climate change, cardiovascular diseases (CVDs), diabetes, Infectious diseases, health system, and population aging are examples of challenges known to pose a vast burden worldwide. We are at a point known as the “digital revolution,” characterized by the expansion of artificial intelligence (AI) and a fusion of technology types. AI has emerged as a powerful tool for addressing various health challenges, and the last ten years have been influential due to the rapid expansion in the production and accessibility of health-related data. The computational models and algorithms can understand complicated health and medical data to perform various functions and deep-learning strategies. This narrative mini-review summarizes the most current AI applications to address the leading global health challenges. Harnessing its capabilities can ultimately mitigate the Impact of these challenges and revolutionize the field. It has the ability to strengthen global health through personalized health care and improved preparedness and response to future challenges. However, ethical and legal concerns about individual or community privacy and autonomy must be addressed for effective implementation.
Millions of people’s health and well-being are at risk of several factors and multiple overlapping crises, including but not limited to infectious disease outbreaks, rising malnutrition rates, and lack of sufficient medical access; all hit the vulnerable the most (1). As we are heading toward the end of 2023, a record 339 million people globally need urgent aid. Several critical issues need to be addressed urgently to improve health globally and build resilience against future threats (1); the recent COVID-19 has shown that each country’s security and prosperity depend on creating a healthier, safer, more resilient world.
Global public health priorities play a crucial role in addressing the most pressing health challenges faced by populations worldwide. They are dynamic and evolve in response to emerging health challenges and crises, which need effective collaboration among countries to secure global health. Mental Health, the Impact of climate change, cardiovascular diseases (CVDs), diabetes, Infectious diseases, health system, and population aging are examples of challenges that are examples of these challenges that are known to pose a vast burden worldwide (Figure 1) (2, 3).
We are at a point known as the “digital revolution,” characterized by the expansion of artificial intelligence (AI) and a fusion of technology types; the rapid and transformative changes brought about by these advances in digital technology led to increased connectivity and accessibility of vast amounts of information (4). AI has emerged as a powerful tool for addressing various challenges, and the last ten years have been influential in the digital health (5, 6). AI and its subfields or techniques, such as deep learning (DL), natural language processing (NLP), and machine learning (ML), have prospects to benefit healthcare, including public health, because of the rapid expansion in the production and accessibility of health-related data (7–9). The computational models and algorithms can understand complicated health and medical care data to perform various functions and deep-learning strategies. AI in health care improves disease surveillance, diagnosis, treatment selection, and clinical laboratory testing (10, 11). Harnessing its capabilities can ultimately mitigate the impact of global public health issues and revolutionize the field.
However, the burgeoning interest was accompanied by caution over using it, especially in health-related fields. Crucial ethics, privacy, and bias issues were raised to ensure AI’s responsible and equitable integration in the global public health landscape. Additionally, the human inability to see how ML systems make their decisions “black box,” brought uncertainty and threatened trust among the users regarding its application (12). This review aims to explore the current state of AI in those mentioned global public health challenges and provide insights about its current application in disease diagnosis, medicinal product development, and medical intervention. This step will provide more comprehension, and interpretability for most of AI’s leading evidence-based applications and contribute to a better understanding of AI in dealing with the increasing scale and complexity of challenges to global health.
The global development over the last years was also associated with the change in disease burden, and NCDs have almost become the leading cause of mortality, resulting in 200 million premature deaths and expectation of another 150 million people deaths during the next ten years; most of them in low and middle-income countries (13).
NCDs are usually multifactorial diseases, and several factors are associated with their development, including genetic and environmental factors, which make them challenging to prevent and treat effectively. They are exacerbated by four key modifiable risk factors, namely tobacco use, harmful use of alcohol, physical inactivity, and unhealthy diets (14). It was estimated that behavioral factors and genetic factors are the main contributors to preterm death in the USA with (40%), and (30%) respectively (15), and the role of public health awareness and intervention have a vast impact (16). AI can offer the potential to analyze large and complex datasets obtained from lifestyle, clinical, and biological data in a way that exceeds the human ability to make sense of it. ML, NLP, Robotic, virtual agents (chatbots), and speech analysis are just a few examples of the available AI applications that are used to improve public health (17). For example, Florence, which is the WHO’s first virtual health worker, is designed to help the world’s 1.3 billion tobacco users quit smoking (18); STop obesity Platform that can offer personalized support to people with obesity (19), and chatbots to personalized fitness strategies.
If we discuss kidney disease progression as a case illustration, AI has its application in pre/post-diagnosis, which can ultimately lead to improved outcomes in a timely and accurate manner (20). During the analysis phase of patient data, AI can identify the early signs of the diseases from the lab results, medical history, and images (21, 22), it can also help in the diagnosis of kidney disease from a kidney biopsy through deep learning-based approaches (23), and to improve outcome and early detection of other comorbidity in renal patients (24). The flowing part discusses AI’s applications in the major of NCDs.
Cardiovascular diseases (CVDs) are the leading cause of global mortality, taking an estimated 17.9 million lives in 2019. Strategies to tackle this considerable burden are directed toward reducing risk factors, enhancing the health system, and monitoring disease patterns and trends to inform national and global actions. The application of AI can identify, process, integrate, and analyze massive amounts of data, including but not limited to medical records, ultrasounds, medications, and experimental results. The gold standard in diagnosing most CVDs is an echocardiogram (ECG) and cardiac magnetic resonance (CMR). However, the clinician’s interpretation of ECG depends on their experience. Hence, ECG information might be missed due to clinicians’ difficulty analyzing them (25, 26).
Additionally, most ECGs are done on symptomatic patients, even though many CVDs, such as valvular heart diseases, involve long asymptomatic periods (27). However, the AI algorithm interpretation of ECG can diagnose heart failure, atrial fibrillation, hypertrophic cardiomyopathy, pulmonary hypertension, aortic valve stenosis, and anemia (28–36). An approach that reduced time and the physician’s cognitive burden by offering pre-diagnosis, correcting clinician errors and preventing the occurrence of misdiagnosis. Moreover, AI enhances the prompt efficiency of medical tools such as computed tomography (CT), echocardiography, magnetic resonance imaging (MRI), and Coronary computed tomography angiography (CCTA) (37–39). In addition to its application in CVD prediction and predictive modeling, such as mortality prediction, vascular aging, and predicting major adverse cardiovascular events in asymptomatic subjects (40–43).
Cancer is a large group of diseases and is considered the second leading cause of global mortality; the estimated mortality in 2020 was 10 million deaths. Despite the several accomplishments that have been made in the field of cancer diagnosis, prognosis, and treatment, individualized and data-driven care remains challenging. The challenge lies in the specific characteristics of distinct molecular, genetic, and tumor-based features (44). However, using machine learning and AI positively supports cancer prevention and management, as it is reshaping the existing picture, and is developing rapidly (45–47). AI has become pivotal as it can provide patients with forecasting and prediction and improved risk stratification according to specific criteria, such as in the cases of some breast, colon, ovarian, lung, and skin cancers (48–54). Further, it can detect hidden patterns from several sources such as molecular profiling, pathology, and medical imaging, and integration of-omics data to provide a more comprehensive understanding of cancer and improve the precision oncology (55). During a surgical procedure, AI can provide real-time detection and diagnosis of some cancers; through its ability to differentiate between cancerous and normal tissues (56, 57). Recently, mounting evidence indicated the potential role of microRNAs (miRNA) in cancer diagnosis and prognosis. MiRNAs, a small, single-stranded, non-coding ribonucleic acids (RNAs), are essential for all biological functions including cancer development. ML provides an opportunity to explore miRNA’s ability to serve as a reliable biomarker targeting drugs and improve cancer clinical classification (58, 59).
A recent achievement is the genetics-based classification and treatment response of Cancer of unknown primary (CUP); this type of cancer usually leads to poor outcomes because primary cancer is unknown (60). The study used ML to classify the cancer based on its genetic profile. This model identifies the likely prior site and predicts the best treatment option (60). Another example is pancreatic cancer, one of the most challenging cancers to diagnose as it is often asymptomatic until it metastasises, causing poor and ineffective treatment. AI modeling enables the detection of individuals at high risk of developing pancreatic cancer; the detection was up to 3 years earlier than currently by using medical records (61).
A global crisis that is increasing exponentially and is considered a significant cause of blindness, kidney failure, heart attacks, stroke, and lower limb amputation. It was estimated that the prevalence was 529 million in 2021 and is projected to reach 1·31 billion in 2050 (62), a burden that has a global agreement to halt before. According to several recommendations, the starting point is prevention by screening, especially for obese or overweight adults (63, 64). However, a significant number of cases were missed with these approaches. The current clinical application of AI in diabetes diagnosis and management is categorized into four domains: (1) automatic retinal screening, (2) clinical diagnosis support, (3) patient self-management tools, and (4) risk stratification (65). AI for automatic retinal screening enables early diagnosis with high specificity and sensitivity (66). Several studies have evaluated the prediction of new-onset diabetes mellitus by AI and ML models, and it was recommended to include data as an omics database (e.g., genomics) (67). Recently, a new model based on AI was developed to detect diabetes warning signs, even in patients who did not meet the guidelines for diabetes elevated risk. This model can enhance type 2 diabetes (T2D) detection; it uses the patient’s X-ray image collected during routine medical care and their medical records to detect T2D (68).
In diabetes management, AI devices can help patients monitor their glucose levels in real-time and predict spikes or drops in healthcare. AI-based medical devices, such as the Guardian Connect System by Medtronic and the DreaMed Diabetes system (DreaMed Diabetes Ltd), have been approved to help control diabetes (69, 70). Another mobile application (GoCARB) is used to estimate the carbohydrate content in meals, which can help enhance the patients’ skills in managing diabetic disease through diet management (71, 72). The future application of AI will introduce a paradigm shift in Diabetes care from conventional management to more personalized and data-driven precision care.
As a result of significant increases in life expectancy, the global population of people aged 60 years and older is increasing (73). One billion was the estimated number in 2019, rising to 1.4 billion by 2030, accounting for 16.7% of the global population, and projected to reach 2.1 billion by 2050; around 80% will live in low and middle-income countries (74, 75). The increase in age is associated with common health conditions, leading to several complex health states due to multiple underlying factors, such as disability. However, there is no linearity or consistency in developing these changes, and individual characteristics have a vast impact. They will continuously demand primary and long-term care, a more trained workforce, and physical and social environments for social support. Around 92% of global older adults have at least one chronic disease, and more than 81% of those aged ≥85 years suffer from two chronic diseases or more (76–78). Additionally, disability and its consequences also have a huge burden on the aging population. Globally, 1.3 billion people (16% of the world’s population) suffer from a physical or cognitive disability; although these estimates cover all age groups, starting from 18 years old (79). However, AI can provide intelligent solutions for longer lives, satisfy the growing unmet healthcare needs, and overcome the limited number of insufficient healthcare resources. Currently, AI technologies for aging population are used in the robotic intervention (80, 81), applications on smartphones or computers (82, 83), social interaction and support, such as improved mental well-being and quality of life (84–86); rehabilitation therapy, such as its application in the recovery of upper and lower extremity functions, gait robotic rehabilitation, or improve sleep quality and daily living activities (87–90), and wearables, voice-activated (91–94). It can create more advanced algorithms to provide more precise holistic interventions tailored to address the elders’ multiple needs in a safer and more friendly manner (78, 95, 96). Ambient-assisted working and ambient-assisted living are examples of smart systems that can adapt themselves to older adult needs by exploiting ambient intelligence solutions. These systems focus on using technology to support and enhance the quality of life of the older adult population, either in work, or indoor and outdoor environments (97, 98). Recently, a group of researchers have helped develop drugs that might potentially delay the effects of aging by eliminating senescent cells (96, 99).
The global estimate of mental health disorders is one billion individuals (100). Since the beginning of the COVID-19 pandemic, the rates of anxiety, depression, and substance use disorder have increased (101, 102). This situation is worse in low-and middle-income countries, where the estimated number of people with limited access and no treatment is around 75% of people with mental, neurological and substance use disorders (100, 102).
Despite the significant advantages of using AI in healthcare, mental health has been slower to adopt AI since the primary factor contributing to successful psychiatric diagnosis and treatment is the interaction with the patients’ (103–105). However, AI applications have great potential in diagnosing different kinds of mental illness. This is a great advantage given the available heterogeneity in the pathophysiology of mental illness. AI can access and analyze relevant information about a patient’s unique bio-psycho-social characteristics and identify pertinent data patterns that might help provide more objective, improved definitions of these illnesses (106). Further, AI can be used in biomarkers identification, develop better diagnoses and formulate risk models to predict individual risk (105, 107). Moreover, it can be used for some cases, such as depression or autism, where face-to-face interaction might be challenging. In autism, for example, AI could be a more useful tool than a psychotherapy session with a human doctor; it can provide tailored, personalized interventions or bridge the communication gap they may experience (105). Nevertheless, the variation of AI applications is persistent in dealing with sensitive issues like mental health.
As the world becomes increasingly globalized, health and illnesses have no borders. Concerns about One Health have gained prominence recently, which is justified as the world emerges from the most significant global emergency and the increasing number of infectious pathogens that spread from humans, animals, or the environment. COVID-19 has highlighted the high spreading rate with which infections can devastate the world’s health and economy. Results in more investment and investigation into the occurrence, prevalence, prevention, control, and treatment of infectious diseases to strengthen the epidemic response and mobilize quickly for public health priority.
Globally, the leading communicable diseases associated with high mortalities are HIV/AIDS, tuberculosis (TB), malaria, viral hepatitis, sexually transmitted infections, and neglected tropical diseases (NTDs). HIV continues to be a major global issue, claiming the lives of 36.3 million so far, and TB-associated mortalities reached 1.5 million annually, making it the world’s second top infectious killer after COVID-19 (108). Tackling AI applications in HIV will yield several examples in HIV prevention, testing, and treatment to achieve sustained viral suppression (109–111). It was used also for rapid detection and response through monitoring clusters of vulnerable groups to reduce HIV transmission (111). Another example is Syphilis, which is a sexually transmitted disease (STD). To eliminate congenital syphilis (CS), the WHO launched an initiative in Latin America and the Caribbean (112). However, as the syphilis epidemic increased in Brazil, the government of Brazil developed a national project, the “Syphilis No!” Project (SNP), for implementing and integrating a syphilis response into healthcare networks, (113, 114). This project encompasses four dimensions: (a) management and governance, (b) surveillance, (c) comprehensive care, and (d) strengthening of the educommunication (113, 115). The application of AI such as data mining and NLP in these strategies augments the country’s capabilities in combating syphilis (113).
It is essential to analyze global infectious disease cases regularly. However, some countries’ investment in contagious disease identification was typically based on the identification of presenting symptoms and the likelihood of exposure due to the high cost and feasibility of the primary approach of detection (116, 117). However, using big data, AI and ML algorithms can contribute to global infection control and help with the spatial and temporal prediction of the evolution and spread of infectious diseases (118). Their advanced capabilities can analyze several factors: population demographics, environmental conditions, and individual behaviors, all of which can be used simultaneously (119). Such as case prediction according to historical data (120), predicting the likelihood of an individual contracting an infectious disease according to personal and behavioral characteristics, using pathogen genetic makeup to identify the most likely sources of an outbreak, identifying or anticipating an epidemic by analyzing massive data; it can be used for early warning systems, hot spot detection, forecasting, and improving the recourses allocation at a country and a global level (68–72). After the exposure or presence of a potential outbreak, AI can advance in diagnostic approaches and differentiate various pathogens by using the pathogen genetic makeup, such as its ability to distinguish between COVID-19 and other circulating respiratory viruses with COVID-like symptoms (121, 122).
Another example is the possible application to the rising incidence of antimicrobial resistance (AMR), which has become a significant challenge. For this purpose, a group of researchers were able to develop a mobile application to classify bacterial susceptibility to various antibiotics, especially in resource-limited settings (123). Further, reducing transmission is essential to control global widespread infections such as those that occur in pandemics. The application of AI for screening technologies targeting infections and integrating them into data visualization has been introduced broadly, especially during the COVID-19 pandemic (117). This improved the surveillance and generated meaningful insights from multidimensional data, which can be widely used for public health practice.
In addition to surveillance, early detection, and diagnosis, AI is used to develop anti-infective therapies, although it became challenging with the spread of drug resistance (124). ML models can help explore the pathway of pathogen’s interaction with host cells and immune responses, facilitating antigen determination, vaccine design, and treatment strategies (124–127). Finally, the WHO global report on infection prevention and control estimated that implementing infection prevention and control (IPC) can reduce healthcare-associated infections (HAIs) by 70% (128). Using AI can improve current and past processes to speed infection prevention and control response, such as identifying the correlations associated with medically relevant conditions, identifying potential risk factors, and surveillance of emerging infectious diseases (129–131), improving hand hygiene compliance (132), and in-hospital analysis of transmission, and outbreak events identification and investigation (133).
The impact of environmental health on human lives and health are interconnected in various ways. The Global Health Observatory estimated that 24% of all estimated global deaths are linked to the environment. Between 2030 and 2050, climate change is expected to cause approximately 250,000 additional deaths per year, mainly from undernutrition, malaria, diarrhea and heat stress (134). Because of the adynamic of the environment, AI applications in this field are immense; its deployment will provide a better capacity to deal with the growing climate exigency and related challenges. In exposure assessment, AI can use satellite observations, meteorological variables, land use, and traffic data to predict the spatiotemporal patterns and concentrations of pollutants (135–138). AI were used in monitoring, such as its application during COVID-19, for airport security checks and patient tracking (139), or to improve the prediction of harmful algal blooms (140).
Additionally, it can predict diseases based on environmental factors, such as its application to predict the spread of Zika virus and Dengue fever (141, 142). In waste management, AI reduces fuel consumption and emissions, increases recycling rates, and reduces landfill waste (143). GeoAI is one of the emerging AI tools that can handle complex spatial and temporal data to adjust algorithms and workflows according to the specific characteristics of spatial processes (136, 144). It can develop various environmental exposure models across different geographical regions in prospective and retrospective approaches (136). In 2022, the United Nations launched The World Environment Situation Room, a new digital platform that can provide real-time analysis, track air quality, measure environmental footprint, and monitor (145).
Through good stewardship, resource development, funding and services, health systems support initiatives to prevent, promote, and provide for more health and well-being (146). They are complex and are in a constant state of flux; according to the World Health Organization (WHO), “A well-functioning health system working in harmony is built on having trained and motivated health workers, a well-maintained infrastructure, and a reliable supply of medicines and technologies backed by adequate funding, strong health plans and evidence-based policies” (147). At the global level, it should be able to control and address global health challenges and severe events (147). However, several myriads of difficulties impede their ability to provide these services. This includes but is not limited to the sudden onset or the slowly growing crises, such as the COVID-19 pandemic, the natural disasters the world is encountering, or the slow time impact of climate change (148–150), the rising number of older people, and the associated complex chronic medical illness. To overcome these difficulties and achieve effective and lasting change, four factors were proposed: (a) the acorn-to-oak tree principle (small initiative), (b) the data-to-information-to-intelligence principle (information technology (IT) and data), (c) the many-hands principle (stakeholders); and (d) the patients-the-preeminent-player principle (individuals) (151). These factors were established across 60 health systems; the role of data and technology cannot be missed (5, 151). AI applications are steadily entering novel domains previously governed solely by human experts. They can improve health financing, make public health more effective, and reach underserved populations by making health care more efficient and effective through more personal health services (152).
Further, the Primary health care system (PHC) is vital to addressing health issues effectively; they are considered the front door of the health care system. Using AI will enhance the holistic approach of PHC in outcome prediction, data mining, and personalized treatment (153–155). The current tools in PHC have several applications, including the risk prediction (156–158), workforce assessment (159), record data extraction (160, 161), control of healthcare-associated infections (162), and performing medical tasks remotely that contribute to public health domain (154, 163, 164).
AI integration and application to global health challenges have immense potential to overcome them efficiently and effectively. Disease prevention, detection, and response can quickly mobilize and yield medicinal products. As mentioned earlier, around 40% of preterm death was associated with behavioral factors. With AI advancement, data analysis and segmentation can be done for several characteristics such as behavior, opinion, and attitude. Using these data, the ML can analyze the online health information and provide personalized massaging to influence individuals’ health behaviors with high quality and clarity, amplifying their influence and effectiveness (165–167). This health communication can also inform AI technology in developing effective communication systems with patients and their healthcare providers. The health communication theories and models can highlight the available barriers to behavioral change and the available limitations of technology-driven health interventions. Which can help improve the efficacy of AI-supported systems or intervention designs (167–169).
AI in health care is expected to grow from nearly US $15 billion to $103 billion between 2023 and 2028 (170). However, incongruent with AI’s benefits, exacerbation of inequities was accompanied, and ethical and legal concerns about individual or community privacy and autonomy were raised (118, 171). The EU AI Act is nearing implementation, and it will be the first comprehensive regulation that addresses the risks of artificial intelligence; European Parliament proposed it to ensure better conditions for developing and using this innovative technology (172). Further, to avoid the risk of hindering AI applications in healthcare due to lack of sufficient transparency “black box,” researchers were urged to provide more research and explanation for AI; explainable AI (xAI), as an approach to more understandable and human-interpretable AI-based applications (173, 174).
However, AI stands as a cornerstone of the upcoming digital revolution. Despite the moral dilemmas in AI application in health care, it is likely to meager, co-exist or replace current systems and assets as a potent amplifier of human potential. It has the ability to strengthen global health through personalized health care and improved preparedness and response to future challenges.
AZ: Conceptualization, Writing – original draft.
The author declares that no financial support was received for the research, authorship, and/or publication of this article.
The author declares that the research was conducted in the absence of any commercial or financial relationships that could be construed as a potential conflict of interest.
All claims expressed in this article are solely those of the authors and do not necessarily represent those of their affiliated organizations, or those of the publisher, the editors and the reviewers. Any product that may be evaluated in this article, or claim that may be made by its manufacturer, is not guaranteed or endorsed by the publisher.
1. World Health Organization . WHO's health emergency appeal 2023. Geneva: World Health Organization (2023a).
2. Chan, A Eleven global health issues to watch in 2023, according to IHME experts: the Institute for Health Metrics and Evaluation. (2022). Available at: https://www.healthdata.org/news-events/insights-blog/acting-data/11-global-health-issues-watch-2023-according-ihme-experts.
3. Lucero-Prisno, DE, Shomuyiwa, DO, Kouwenhoven, MBN, Dorji, T, Odey, GO, Miranda, AV, et al. Top 10 public health challenges to track in 2023: shifting focus beyond a global pandemic. Public Health Challenges. (2023) 2:e86. doi: 10.1002/puh2.86
4. Schwab, K The fourth industrial revolution: what it means, how to respond New York. (2016). Available at: https://www.weforum.org/agenda/2016/01/the-fourth-industrial-revolution-what-it-means-and-how-to-respond/.
5. World Bank . Digital-in-health: Unlocking the value for everyone. Washington DC: World Bank (2023).
6. U.S. Agency for International Development . Artificial intelligence in Global Health: Defining a collective path forward. Washington: U.S. Agency for International Development (2019).
7. Suleimenov, IE, Vitulyova, YS, Bakirov, AS, and Gabrielyan, OA. Artificial intelligence: What is it? Proceedings of the 2020 6th International Conference on Computer and Technology Applications Antalya, Turkey: Association for Computing Machinery (2020).
8. Davenport, T, and Kalakota, R. The potential for artificial intelligence in healthcare. Future Healthcare J. (2019) 6:94–8. doi: 10.7861/futurehosp.6-2-94
9. Kubat, M . A simple machine-learning task. An introduction to machine learning. Cham: Springer International Publishing (2017).
10. Alowais, SA, Alghamdi, SS, Alsuhebany, N, Alqahtani, T, Alshaya, AI, Almohareb, SN, et al. Revolutionizing healthcare: the role of artificial intelligence in clinical practice. BMC Med Educ. (2023) 23:689. doi: 10.1186/s12909-023-04698-z
11. Rubens, M, Ramamoorthy, V, Saxena, A, and Shehadeh, N. Public health in the twenty-first century: the role of advanced technologies. Front Media. (2014) 2:224. doi: 10.3389/fpubh.2014.00224
12. Steerling, E, Siira, E, Nilsen, P, Svedberg, P, and Nygren, J. Implementing AI in healthcare—the relevance of trust: a scoping review. Front Health Serv. (2023) 3:150. doi: 10.3389/frhs.2023.1211150
14. World Health Organization . Noncommunicable diseases: Key facts. Geneva: World Health Organization (2023b).
15. Schroeder, SA . We can do better — improving the health of the American people. N Engl J Med. (2007) 357:1221–8. doi: 10.1056/NEJMsa073350
16. Frieden, TR . The future of public health. N Engl J Med. (2015) 373:1748–54. doi: 10.1056/NEJMsa1511248
17. Pan American Health Organization . Artificial intelligence in public health. Washington: Pan American Health Organization (2021).
18. World Health Organization . Using AI to lead a healthier lifestyle: How Florence can help you. Geneva: World Health Organization (2022a).
19. European Commission . STop obesity platform. (2019). Available at: https://cordis.europa.eu/project/id/823978.
20. Bellocchio, F, and Zhang, H. Editorial: artificial intelligence in nephrology. Front. Nephrol. (2023) 3:769. doi: 10.3389/fneph.2023.1270769
21. Fayos De Arizón, L, Viera, ER, Pilco, M, Perera, A, De Maeztu, G, Nicolau, A, et al. Artificial intelligence: a new field of knowledge for nephrologists? Clin Kidney J. (2023). doi: 10.1093/ckj/sfad182
22. Nong, Y, Wei, X, Qiu, H, Yang, H, Yang, J, Lu, J, et al. Analysis of risk factors for severe acute kidney injury in patients with acute myocardial infarction: a retrospective study. Front Nephrology. (2023) 3:249. doi: 10.3389/fneph.2023.1047249
23. Basso, MN, Barua, M, Meyer, J, John, R, and Khademi, A. Machine learning in renal pathology. Front Nephrology. (2022) 2:7002. doi: 10.3389/fneph.2022.1007002
24. Canaud, B, Kooman, J, Davenport, A, Campo, D, Carreel, E, Morena-Carrere, M, et al. Digital health technology to support care and improve outcomes of chronic kidney disease patients: as a case illustration, the Withings toolkit health sensing tools. Front Nephrol. (2023) 3:565. doi: 10.3389/fneph.2023.1148565
25. Elias, P, Poterucha, TJ, Rajaram, V, Moller, LM, Rodriguez, V, Bhave, S, et al. Deep learning electrocardiographic analysis for detection of left-sided Valvular heart disease. J Am Coll Cardiol. (2022) 80:613–26. doi: 10.1016/j.jacc.2022.05.029
26. Siontis, KC, Noseworthy, PA, Attia, ZI, and Friedman, PA. Artificial intelligence-enhanced electrocardiography in cardiovascular disease management. Nat Rev Cardiol. (2021) 18:465–78. doi: 10.1038/s41569-020-00503-2
27. Lancellotti, P, Magne, J, Dulgheru, R, Clavel, MA, Donal, E, Vannan, MA, et al. Outcomes of patients with asymptomatic aortic stenosis followed up in heart valve clinics. JAMA Cardiol. (2018) 3:1060–8. doi: 10.1001/jamacardio.2018.3152
28. Attia, ZI, Kapa, S, Lopez-Jimenez, F, McKie, PM, Ladewig, DJ, Satam, G, et al. Screening for cardiac contractile dysfunction using an artificial intelligence-enabled electrocardiogram. Nat Med. (2019) 25:70–4. doi: 10.1038/s41591-018-0240-2
29. Attia, ZI, Kapa, S, Noseworthy, PA, Lopez-Jimenez, F, and Friedman, PA. Artificial intelligence ECG to detect left ventricular dysfunction in COVID-19: a case series. Mayo Clin Proc. (2020) 95:2464–6. doi: 10.1016/j.mayocp.2020.09.020
30. Attia, ZI, Noseworthy, PA, Lopez-Jimenez, F, Asirvatham, SJ, Deshmukh, AJ, Gersh, BJ, et al. An artificial intelligence-enabled ECG algorithm for the identification of patients with atrial fibrillation during sinus rhythm: a retrospective analysis of outcome prediction. Lancet. (2019) 394:861–7. doi: 10.1016/S0140-6736(19)31721-0
31. Yao, X, Rushlow, DR, Inselman, JW, McCoy, RG, Thacher, TD, Behnken, EM, et al. Artificial intelligence-enabled electrocardiograms for identification of patients with low ejection fraction: a pragmatic, randomized clinical trial. Nat Med. (2021) 27:815–9. doi: 10.1038/s41591-021-01335-4
32. Kwon, JM, Cho, Y, Jeon, KH, Cho, S, Kim, KH, Baek, SD, et al. A deep learning algorithm to detect anaemia with ECGs: a retrospective, multicentre study. Lancet Digit Health. (2020) 2:e358–67. doi: 10.1016/S2589-7500(20)30108-4
33. Kwon, JM, Kim, KH, Medina-Inojosa, J, Jeon, KH, Park, J, and Oh, BH. Artificial intelligence for early prediction of pulmonary hypertension using electrocardiography. J Heart Lung Transplant. (2020) 39:805–14. doi: 10.1016/j.healun.2020.04.009
34. Ko, WY, Siontis, KC, Attia, ZI, Carter, RE, Kapa, S, Ommen, SR, et al. Detection of hypertrophic cardiomyopathy using a convolutional neural network-enabled electrocardiogram. J Am Coll Cardiol. (2020) 75:722–33. doi: 10.1016/j.jacc.2019.12.030
35. Kwon, JM, Lee, SY, Jeon, KH, Lee, Y, Kim, KH, Park, J, et al. Deep learning-based algorithm for detecting aortic stenosis using electrocardiography. J Am Heart Assoc. (2020) 9:e014717. doi: 10.1161/JAHA.119.014717
36. Cohen-Shelly, M, Attia, ZI, Friedman, PA, Ito, S, Essayagh, BA, Ko, WY, et al. Electrocardiogram screening for aortic valve stenosis using artificial intelligence. Eur Heart J. (2021) 42:2885–96. doi: 10.1093/eurheartj/ehab153
37. Choi, AD, Marques, H, Kumar, V, Griffin, WF, Rahban, H, Karlsberg, RP, et al. CT evaluation by artificial intelligence for atherosclerosis, stenosis and vascular morphology (CLARIFY): a multi-center, international study. J Cardiovasc Comput Tomogr. (2021) 15:470–6. doi: 10.1016/j.jcct.2021.05.004
38. Lin, A, Manral, N, McElhinney, P, Killekar, A, Matsumoto, H, Kwiecinski, J, et al. Deep learning-enabled coronary CT angiography for plaque and stenosis quantification and cardiac risk prediction: an international multicentre study. Lancet Digit Health. (2022) 4:e256–65. doi: 10.1016/S2589-7500(22)00022-X
39. Knott, KD, Seraphim, A, Augusto, JB, Xue, H, Chacko, L, Aung, N, et al. The prognostic significance of quantitative myocardial perfusion: an artificial intelligence-based approach using perfusion mapping. Circulation. (2020) 141:1282–91. doi: 10.1161/CIRCULATIONAHA.119.044666
40. CGdS, E Silva, Buginga, GC, Eads, E Silva, Arena, R, Rouleau, CR, Aggarwal, S, et al., Prediction of mortality in coronary artery disease: role of machine learning and maximal exercise capacity. Mayo Clinic proceedings; (2022). Elsevier.
41. Raghunath, S, Ulloa Cerna, AE, Jing, L, vanMaanen, DP, Stough, J, Hartzel, DN, et al. Prediction of mortality from 12-lead electrocardiogram voltage data using a deep neural network. Nat Med. (2020) 26:886–91. doi: 10.1038/s41591-020-0870-z
42. Toya, T, Ahmad, A, Attia, Z, Cohen-Shelly, M, Ozcan, I, Noseworthy, PA, et al. Vascular aging detected by peripheral endothelial dysfunction is associated with ECG-derived physiological aging. J Am Heart Assoc. (2021) 10:e018656. doi: 10.1161/JAHA.120.018656
43. Eisenberg, E, McElhinney, PA, Commandeur, F, Chen, X, Cadet, S, Goeller, M, et al. Deep learning-based quantification of Epicardial adipose tissue volume and attenuation predicts major adverse cardiovascular events in asymptomatic subjects. Circ Cardiovasc Imaging. (2020) 13:e009829. doi: 10.1161/CIRCIMAGING.119.009829
44. Bhinder, B, Gilvary, C, Madhukar, NS, and Elemento, O. Artificial intelligence in Cancer research and precision medicine. Cancer Discov. (2021) 11:900–15. doi: 10.1158/2159-8290.CD-21-0090
45. Gaur, K, and Jagtap, MM. Role of artificial intelligence and machine learning in prediction, diagnosis, and prognosis of Cancer. Cureus. (2022) 14:e31008. doi: 10.7759/cureus.31008
46. Huang, S, Yang, J, Fong, S, and Zhao, Q. Artificial intelligence in cancer diagnosis and prognosis: opportunities and challenges. Cancer Lett. (2020) 471:61–71. doi: 10.1016/j.canlet.2019.12.007
47. Wang, S-H, Chen, G, Zhong, X, Lin, T, Shen, Y, Fan, X, et al. Global development of artificial intelligence in cancer field: a bibliometric analysis range from 1983 to 2022. Front Oncol. (2023) 13:1215729. doi: 10.3389/fonc.2023.1215729
48. Enshaei, A, Robson, CN, and Edmondson, RJ. Artificial intelligence systems as prognostic and predictive tools in ovarian Cancer. Ann Surg Oncol. (2015) 22:3970–5. doi: 10.1245/s10434-015-4475-6
49. Nartowt, BJ, Hart, GR, Muhammad, W, Liang, Y, Stark, GF, and Deng, J. Robust machine learning for colorectal Cancer risk prediction and stratification. Front Big Data. (2020) 3:6. doi: 10.3389/fdata.2020.00006
50. Nartowt, BJ, Hart, GR, Roffman, DA, Llor, X, Ali, I, Muhammad, W, et al. Scoring colorectal cancer risk with an artificial neural network based on self-reportable personal health data. PLoS One. (2019) 14:e0221421. doi: 10.1371/journal.pone.0221421
51. Hart, GR, Roffman, DA, Decker, R, and Deng, J. A multi-parameterized artificial neural network for lung cancer risk prediction. PLoS One. (2018) 13:e0205264. doi: 10.1371/journal.pone.0205264
52. Stark, GF, Hart, GR, Nartowt, BJ, and Deng, J. Predicting breast cancer risk using personal health data and machine learning models. PLoS One. (2019) 14:e0226765. doi: 10.1371/journal.pone.0226765
53. Roffman, D, Hart, G, Girardi, M, Ko, CJ, and Deng, J. Predicting non-melanoma skin cancer via a multi-parameterized artificial neural network. Sci Rep. (2018) 8:1701. doi: 10.1038/s41598-018-19907-9
54. Hunter, B, Chen, M, Ratnakumar, P, Alemu, E, Logan, A, Linton-Reid, K, et al. A radiomics-based decision support tool improves lung cancer diagnosis in combination with the herder score in large lung nodules. EBioMedicine. (2022) 86:104344. doi: 10.1016/j.ebiom.2022.104344
55. Perez-Lopez, R, Reis-Filho, JS, and Kather, JN. A framework for artificial intelligence in cancer research and precision oncology. NPJ Prec Oncology. (2023) 7:43. doi: 10.1038/s41698-023-00383-y
56. Hollon, TC, Pandian, B, Adapa, AR, Urias, E, Save, AV, Khalsa, SSS, et al. Near real-time intraoperative brain tumor diagnosis using stimulated Raman histology and deep neural networks. Nat Med. (2020) 26:52–8. doi: 10.1038/s41591-019-0715-9
57. Zhang, B, Shi, H, and Wang, H. Machine learning and AI in Cancer prognosis, prediction, and treatment selection: a critical approach. J Multidiscip Healthc. (2023) 16:1779–91. doi: 10.2147/JMDH.S410301
58. Luo, Y, Peng, L, Shan, W, Sun, M, Luo, L, and Liang, W. Machine learning in the development of targeting microRNAs in human disease. Front Genet. (2023) 13:189. doi: 10.3389/fgene.2022.1088189
59. Azari, H, Nazari, E, Mohit, R, Asadnia, A, Maftooh, M, Nassiri, M, et al. Machine learning algorithms reveal potential miRNAs biomarkers in gastric cancer. Sci Rep. (2023) 13:6147. doi: 10.1038/s41598-023-32332-x
60. Moon, I, LoPiccolo, J, Baca, SC, Sholl, LM, Kehl, KL, Hassett, MJ, et al. Machine learning for genetics-based classification and treatment response prediction in cancer of unknown primary. Nat Med. (2023) 29:2057–67. doi: 10.1038/s41591-023-02482-6
61. Placido, D, Yuan, B, Hjaltelin, JX, Zheng, C, Haue, AD, Chmura, PJ, et al. A deep learning algorithm to predict risk of pancreatic cancer from disease trajectories. Nat Med. (2023) 29:1113–22. doi: 10.1038/s41591-023-02332-5
62. The Lancet . Diabetes: a defining disease of the 21st century. Lancet. (2023) 401:2087. doi: 10.1016/S0140-6736(23)01296-5
63. Duan, D, Kengne, AP, and Echouffo-Tcheugui, JB. Screening for diabetes and prediabetes. Endocrinol Metab Clin. (2021) 50:369–85. doi: 10.1016/j.ecl.2021.05.002
64. Davidson, KW, Barry, MJ, Mangione, CM, Cabana, M, Caughey, AB, Davis, EM, et al. Screening for prediabetes and type 2 diabetes: US preventive services task force recommendation statement. JAMA. (2021) 326:736–43. doi: 10.1001/jama.2021.12531
65. Ellahham, S . Artificial intelligence: The future for diabetes care. Am J Med. (2020) 133:895–00. doi: 10.1016/j.amjmed.2020.03.033
66. Huang, X, Wang, H, She, C, Feng, J, Liu, X, Hu, X, et al. Artificial intelligence promotes the diagnosis and screening of diabetic retinopathy. Front Endocrinol. (2022) 13:946915. doi: 10.3389/fendo.2022.946915
67. Nomura, A, Noguchi, M, Kometani, M, Furukawa, K, and Yoneda, T. Artificial intelligence in current diabetes management and prediction. Curr Diab Rep. (2021) 21:61. doi: 10.1007/s11892-021-01423-2
68. Pyrros, A, Borstelmann, SM, Mantravadi, R, Zaiman, Z, Thomas, K, Price, B, et al. Opportunistic detection of type 2 diabetes using deep learning from frontal chest radiographs. Nat Commun. (2023) 14:4039. doi: 10.1038/s41467-023-39631-x
69. Abraham, SB, Arunachalam, S, Zhong, A, Agrawal, P, Cohen, O, and McMahon, CM. Improved real-world glycemic control with continuous glucose monitoring system predictive alerts. (2021) 1932–2968 Contract No: 1
70. Benjamens, S, Dhunnoo, P, and Meskó, B. The state of artificial intelligence-based FDA-approved medical devices and algorithms: an online database. NPJ Digit Med. (2020) 3:118. doi: 10.1038/s41746-020-00324-0
71. Guan, Z, Li, H, Liu, R, Cai, C, Liu, Y, Li, J, et al. Artificial intelligence in diabetes management: advancements, opportunities, and challenges. Cell Rep Med. (2023) 4:101213. doi: 10.1016/j.xcrm.2023.101213
72. Vasiloglou, MF, Mougiakakou, S, Aubry, E, Bokelmann, A, Fricker, R, Gomes, F, et al. A comparative study on carbohydrate estimation: GoCARB vs. Diet Nutr. (2018) 10:741. doi: 10.3390/nu10060741
73. Beard, JR, Officer, A, De Carvalho, IA, Sadana, R, Pot, AM, Michel, J-P, et al. The world report on ageing and health: a policy framework for healthy ageing. Lancet. (2016) 387:2145–54. doi: 10.1016/S0140-6736(15)00516-4
74. World Health Organization . Ageing and health. (2022b). Available at: https://www.who.int/news-room/fact-sheets/detail/ageing-and-health.
75. World health Organization . (2023c). Ageing Geneva Available at: https://www.who.int/health-topics/ageing#tab=tab_1.
76. Tkatch, R, Musich, S, MacLeod, S, Alsgaard, K, Hawkins, K, and Yeh, CS. Population health management for older adults: review of interventions for promoting successful aging across the health continuum. Gerontol Geriatr Med. (2016) 2:787. doi: 10.1177/2333721416667877
77. Salive, ME . Multimorbidity in older adults. Epidemiol Rev. (2013) 35:75–83. doi: 10.1093/epirev/mxs009
78. Ma, B, Yang, J, Wong, FKY, Wong, AKC, Ma, T, Meng, J, et al. Artificial intelligence in elderly healthcare: a scoping review. Ageing Res Rev. (2023) 83:101808. doi: 10.1016/j.arr.2022.101808
80. Hu, X, Zeng, X, Xu, Y, Luo, C, Jia, L, Zhao, Z, et al. A soft robotic intervention for gait enhancement in older adults. IEEE Trans Neural Syst Rehabil Eng. (2021) 29:1838–47. doi: 10.1109/TNSRE.2021.3109729
81. Pu, L, Moyle, W, Jones, C, and Todorovic, M. The effect of a social robot intervention on sleep and motor activity of people living with dementia and chronic pain: a pilot randomized controlled trial. Maturitas. (2021) 144:16–22. doi: 10.1016/j.maturitas.2020.09.003
82. Valero, MÁ, Bravo, J, Chamizo, JMG, and López-de-Ipiña, D. Integration of multisensor hybrid reasoners to support personal autonomy in the smart home. Sensors. (2014) 14:17313–30. doi: 10.3390/s140917313
83. VandeWeerd, C, Yalcin, A, Aden-Buie, G, Wang, Y, Roberts, M, Mahser, N, et al. HomeSense: design of an ambient home health and wellness monitoring platform for older adults. Heal Technol. (2020) 10:1291–09. doi: 10.1007/s12553-019-00404-6
84. Follmann, A, Schollemann, F, Arnolds, A, Weismann, P, Laurentius, T, Rossaint, R, et al. Reducing loneliness in stationary geriatric care with robots and virtual encounters—a contribution to the COVID-19 pandemic. Int J Environ Res Public Health. (2021) 18:4846. doi: 10.3390/ijerph18094846
85. Pei-Ti, H, Chen-Shie, H, Ya-Fang, H, and Jeu-Jung, C. The effects of a social participation app on seniors. J Nurs Res. (2021) 29:e168. doi: 10.1097/JNR.0000000000000451
86. Aggar, C, Sorwar, G, Seton, C, Penman, O, and Ward, A. Smart home technology to support older people's quality of life: a longitudinal pilot study. Int J Older People Nursing. (2023) 18:e12489. doi: 10.1111/opn.12489
87. Park, J-H . The effects of robot-assisted left-hand training on hemispatial neglect in older patients with chronic stroke: a pilot and randomized controlled trial. Medicine. (2021) 100:e24781. doi: 10.1097/MD.0000000000024781
88. Calabrò, RS, De Luca, R, Leo, A, Balletta, T, Marra, A, and Bramanti, P. Lokomat training in vascular dementia: motor improvement and beyond! Aging Clin Exp Res. (2015) 27:935–7. doi: 10.1007/s40520-015-0343-2
89. Koumpouros, Y, Toulias, TL, Tzafestas, CS, and Moustris, G. Assessment of an intelligent robotic rollator implementing navigation assistance in frail seniors. Technol Disabil. (2020) 32:159–77. doi: 10.3233/TAD-200271
90. Mizuno, J, Saito, D, Sadohara, K, Nihei, M, Ohnaka, S, Suzurikawa, J, et al. Effect of the information support robot on the daily activity of older people living alone in actual living environment. Int J Environ Res Public Health. (2021) 18:2498. doi: 10.3390/ijerph18052498
91. Ahmed, MU . A personalized health-monitoring system for elderly by combining rules and case-based reasoning. pHealth. (2015) 1:249–54.
92. Tsamis, KI, Rigas, G, Nikolaos, K, Fotiadis, DI, and Konitsiotis, S. Accurate monitoring of Parkinson’s disease symptoms with a wearable device during COVID-19 pandemic. In Vivo. (2021) 35:2327–30. doi: 10.21873/invivo.12507
93. Wang, L-H, Hsiao, Y-M, Xie, X-Q, and Lee, S-Y. An outdoor intelligent healthcare monitoring device for the elderly. IEEE Trans Consum Electron. (2016) 62:128–35. doi: 10.1109/TCE.2016.7514671
94. Lazarou, I, Stavropoulos, TG, Meditskos, G, Andreadis, S, Kompatsiaris, IY, and Tsolaki, M. Long-term impact of intelligent monitoring technology on people with cognitive impairment: an observational study. J Alzheimers Dis. (2019) 70:757–92. doi: 10.3233/JAD-190423
95. Pu, L, Moyle, W, Jones, C, and Todorovic, M. The effectiveness of social robots for older adults: a systematic review and meta-analysis of randomized controlled studies. The Gerontologist. (2019) 59:e37–51. doi: 10.1093/geront/gny046
96. Marino, N, Putignano, G, Cappilli, S, Chersoni, E, Santuccione, A, Calabrese, G, et al. Towards AI-driven longevity research: an overview. Front Aging. (2023) 4:1057204. doi: 10.3389/fragi.2023.1057204
97. Guerra, BMV, Torti, E, Marenzi, E, Schmid, M, Ramat, S, Leporati, F, et al. Ambient assisted living for frail people through human activity recognition: state-of-the-art, challenges and future directions. Front Neurosci. (2023) 17:1256682. doi: 10.3389/fnins.2023.1256682
98. Spoladore, D, and Trombetta, A. Ambient assisted working solutions for the ageing workforce: a literature review. Electronics. (2023) 12:101. doi: 10.3390/electronics12010101
99. Smer-Barreto, V, Quintanilla, A, Elliott, RJR, Dawson, JC, Sun, J, Campa, VM, et al. Discovery of senolytics using machine learning. Nat Commun. (2023) 14:3445. doi: 10.1038/s41467-023-39120-1
100. World Health Organization (2020). World mental health day: an opportunity to kick-start a massive scale-up in investment in mental health. Geneva: World Health Organization.
101. National Institutes of Health (2023). Mental Health During the COVID-19 Pandemic: an urgent issue. Available at: https://covid19.nih.gov/covid-19-topics/mental-health#:~:text=Rates%20of%20anxiety%2C%20depression%2C%20and,have%20mental%20illnesses%20or%20disorders.
102. Gao, Y, Bagheri, N, and Furuya-Kanamori, L. Has the COVID-19 pandemic lockdown worsened eating disorders symptoms among patients with eating disorders? systematic review. A Z Gesundh Wiss. (2022) 30:2743–52. doi: 10.1007/s10389-022-01704-4
103. Fei, J, Yong, J, Hui, Z, Yi, D, Hao, L, Sufeng, M, et al. Artificial intelligence in healthcare: past, present and future. Stroke Vasc Neurol. (2017) 2:230–43. doi: 10.1136/svn-2017-000101
104. Miller, DD, and Brown, EW. Artificial intelligence in medical practice: the question to the answer? Am J Med. (2018) 131:129–033. doi: 10.1016/j.amjmed.2017.10.035
105. Minerva, F, and Giubilini, A. Is AI the future of mental healthcare? Topoi (Dordr). (2023) 42:1–9. doi: 10.1007/s11245-023-09932-3
106. Graham, S, Depp, C, Lee, EE, Nebeker, C, Tu, X, Kim, HC, et al. Artificial intelligence for mental health and mental illnesses: an overview. Curr Psychiatry Rep. (2019) 21:116. doi: 10.1007/s11920-019-1094-0
107. D’Alfonso, S . AI in mental health. Curr Opin Psychol. (2020) 36:112–7. doi: 10.1016/j.copsyc.2020.04.005
108. World Health Organization (n.d.). Communicable and noncommunicable diseases, and mental health. Available at: https://www.who.int/our-work/communicable-and-noncommunicable-diseases-and-mental-health
109. Feller, DJ, Zucker, J, Yin, MT, Gordon, P, and Elhadad, N. Using clinical notes and natural language processing for automated HIV risk assessment. J Acquir Immune Defic Syndr. (2018) 77:160–6. doi: 10.1097/QAI.0000000000001580
110. Kakalou, C, Lazarus, JV, and Koutkias, V. Mining social Media for Perceptions and Trends on HIV pre-exposure prophylaxis. Stud Health Technol Inform. (2019) 264:959–63. doi: 10.3233/SHTI190366
111. Xiang, Y, Du, J, Fujimoto, K, Li, F, Schneider, J, and Tao, C. Application of artificial intelligence and machine learning for HIV prevention interventions. Lancet HIV. (2022) 9:e54–62. doi: 10.1016/S2352-3018(21)00247-2
112. Pan American Health Organization . PAHO launches new initiative to eliminate mother-to-child transmission of four diseases. (2017) Available at: https://www3.paho.org/hq/index.php?option=com_content&view=article&id=13567:paho-launches-new-initiative-to-eliminate-mother-to-child-transmission-of-four-diseases&Itemid=0&lang=en#gsc.tab=0.
113. da Rocha, MA, dos Santos, MM, Fontes, RS, de Melo, ASP, Cunha-Oliveira, A, Miranda, AE, et al. The text mining technique applied to the analysis of health interventions to combat congenital syphilis in Brazil: The case of the “syphilis no!” Project. Front Public Health. (2022) 10:680. doi: 10.3389/fpubh.2022.855680
114. Pinto, R, Valentim, R, Fernandes da Silva, L, Fontoura de Souza, G, Farias, G, De Moura Santos Lima, T, et al. Use of interrupted time series analysis in understanding the course of the congenital syphilis epidemic in Brazil. The Lancet regional health. Americas. (2022) 7:100163. doi: 10.1016/j.lana.2021.100163
115. Caitano, AR, Gusmão, CMG, Dias-Trindade, S, Barbalho, IMP, Morais, PSG, Caldeira-Silva, GJP, et al. Massive health education through technological mediation: analyses and impacts on the syphilis epidemic in Brazil. Front Public Health. (2022) 10:944213. doi: 10.3389/fpubh.2022.944213
116. Sisimayi, C, Harley, C, Nyabadza, F, and Visaya, MV. AI-enabled case detection model for infectious disease outbreaks in resource-limited settings. Front Appl Math Statis. (2023) 9:9. doi: 10.3389/fams.2023.1133349
117. Brownstein, JS, Rader, B, Astley, CM, and Tian, H. Advances in artificial intelligence for infectious-disease surveillance. N Engl J Med. (2023) 388:1597–607. doi: 10.1056/NEJMra2119215
118. World Health Organization . Ethics and governance of artificial intelligence for health: WHO guidance. Geneva: World Health Organization (2021).
119. Santangelo, OE, Gentile, V, Pizzo, S, Giordano, D, and Cedrone, F. Machine learning and prediction of infectious diseases: a systematic review. Mach Learn Knowl Extract. (2023) 5:175–98. doi: 10.3390/make5010013
120. Palaniappan, S, and David, B. Prediction of epidemic disease dynamics on the infection risk using machine learning algorithms. SN Comp Sci. (2021) 3:97. doi: 10.1007/s42979-021-00902-3
121. Maharaj, AS, Parker, J, Hopkins, JP, Gournis, E, Bogoch, II, Rader, B, et al. The effect of seasonal respiratory virus transmission on syndromic surveillance for COVID-19 in Ontario Canada. Lancet Infect Dis. (2021) 21:593–4. doi: 10.1016/S1473-3099(21)00151-1
122. Roy, S, Biswas, P, and Ghosh, P. Spatiotemporal tracing of pandemic spread from infection data. Sci Rep. (2021) 11:17689. doi: 10.1038/s41598-021-97207-5
123. Pascucci, M, Royer, G, Adamek, J, Asmar, MA, Aristizabal, D, Blanche, L, et al. AI-based mobile application to fight antibiotic resistance. Nat Commun. (2021) 12:1173. doi: 10.1038/s41467-021-21187-3
124. Wong, F, de la Fuente-Nunez, C, and Collins, JJ. Leveraging artificial intelligence in the fight against infectious diseases. Science. (2023) 381:164–70. doi: 10.1126/science.adh1114
125. Bojar, D, Powers, RK, Camacho, DM, and Collins, JJ. Deep-learning resources for studying glycan-mediated host-microbe interactions. Cell Host Microbe. (2021) 29:132–44.e3. doi: 10.1016/j.chom.2020.10.004
126. Hie, B, Zhong, ED, Berger, B, and Bryson, B. Learning the language of viral evolution and escape. Science. (2021) 371:284–8. doi: 10.1126/science.abd7331
127. Wheeler, NE, Gardner, PP, and Barquist, L. Machine learning identifies signatures of host adaptation in the bacterial pathogen Salmonella enterica. PLoS Genet. (2018) 14:e1007333. doi: 10.1371/journal.pgen.1007333
128. World Health Organization . Global report on infection prevention and control. Geneva, World Health Organization: (2022c).
129. Fitzpatrick, F, Doherty, A, and Lacey, G. Using artificial intelligence in infection prevention. Curr Treat Options Infect Dis. (2020) 12:135–44. doi: 10.1007/s40506-020-00216-7
130. Bechmann, L, and Geginat, G. Semi-automated contact tracing and management of contact precautions during the COVID-19 pandemic within a tertiary hospital. Infect Prev Pract. (2023) 5:100266. doi: 10.1016/j.infpip.2022.100266
131. Milinovich, GJ, Williams, GM, Clements, AC, and Hu, W. Internet-based surveillance systems for monitoring emerging infectious diseases. Lancet Infect Dis. (2014) 14:160–8. doi: 10.1016/S1473-3099(13)70244-5
132. Worlikar, H, Vadhiraj, VV, Murray, A, O'Connell, J, Connolly, C, Walsh, JC, et al. Is it feasible to use a humanoid robot to promote hand hygiene adherence in a hospital setting? Infect Prev Pract. (2022) 4:100188. doi: 10.1016/j.infpip.2021.100188
133. Branch-Elliman, W, Sundermann, AJ, Wiens, J, and Shenoy, ES. The future of automated infection detection: innovation to transform practice (part III/III). Antimicrob Steward Healthc Epidemiol. (2023) 3:e26. doi: 10.1017/ash.2022.333
134. World Health Organization . Public health and environment. Geneva: World Health Organization (2023e).
135. Nti, EK, Cobbina, SJ, Attafuah, EA, Senanu, LD, Amenyeku, G, Gyan, MA, et al. Water pollution control and revitalization using advanced technologies: uncovering artificial intelligence options towards environmental health protection, sustainability and water security. Heliyon. (2023) 9:e18170. doi: 10.1016/j.heliyon.2023.e18170
136. VoPham, T, Hart, JE, Laden, F, and Chiang, Y-Y. Emerging trends in geospatial artificial intelligence (geoAI): potential applications for environmental epidemiology. Environ Health. (2018) 17:40. doi: 10.1186/s12940-018-0386-x
137. Chinnaswamy, A, and Walton, N. AI and environmental health: a platform ecosystem perspective In: HL Ong, R-a Doong, R Naguib, CP Lim, and AK Nagar, editors. Artificial intelligence and environmental sustainability: Challenges and solutions in the era of industry 40. Singapore: Springer Nature Singapore (2022)
138. Weichenthal, S, Hatzopoulou, M, and Brauer, M. A picture tells a thousand… exposures: opportunities and challenges of deep learning image analyses in exposure science and environmental epidemiology. Environ Int. (2019) 122:3–10. doi: 10.1016/j.envint.2018.11.042
139. Alafif, T, Tehame, AM, Bajaba, S, Barnawi, A, and Zia, S. Machine and deep learning towards COVID-19 diagnosis and treatment: survey, challenges, and future directions. Int J Environ Res Public Health. (2021) 18:1117. doi: 10.3390/ijerph18031117
140. Lee, S, and Lee, D. Improved prediction of harmful algal blooms in four Major South Korea’s Rivers using deep learning models. Int J Environ Res Public Health. (2018) 15:1322. doi: 10.3390/ijerph15071322
141. Gangula, R, Thirupathi, L, Parupati, R, Sreeveda, K, and Gattoju, S. Ensemble machine learning based prediction of dengue disease with performance and accuracy elevation patterns. Mater Today Proceed. (2023) 80:3458–63.
142. Dadheech, P, Mehbodniya, A, Tiwari, S, Kumar, S, Singh, P, Gupta, S, et al. Zika virus prediction using AI-driven technology and hybrid optimization algorithm in healthcare. J Healthcare Eng. (2022) 2022:1–13. doi: 10.1155/2022/2793850
143. Fayyazi, M, Sardar, P, Thomas, SI, Daghigh, R, Jamali, A, Esch, T, et al. Artificial intelligence/machine learning in energy management systems, control, and optimization of hydrogen fuel cell vehicles. Sustainability. (2023) 15:5249. doi: 10.3390/su15065249
144. Fan, Z, Yan, Z, and Wen, S. Deep learning and artificial intelligence in sustainability: a review of SDGs, renewable energy, and environmental health. Sustainability. (2023) 15:13493. doi: 10.3390/su151813493
145. United Nations . World environment situation room: data, information and knowledge on the environment. (n.d.). United Nation Environment Programme Available at: https://wesr.unep.org
146. Darrudi, A, Ketabchi Khoonsari, MH, and Tajvar, M. Challenges to achieving universal health coverage throughout the world: a systematic review. J Prev Med Public Health. (2022) 55:125–33. doi: 10.3961/jpmph.21.542
147. World Health Organization . Health systems strengthening. Geneva: World Health Organization (2023f).
148. Mora, C, McKenzie, T, Gaw, IM, Dean, JM, von Hammerstein, H, Knudson, TA, et al. Over half of known human pathogenic diseases can be aggravated by climate change. Nat Clim Chang. (2022) 12:869–75. doi: 10.1038/s41558-022-01426-1
149. World Health Organization . Climate action: fast facts [press release]. Geneva: World Health Organization (2022d).
150. Wilson, D, Sheikh, A, Görgens, M, and Ward, K. Technology and universal health coverage: examining the role of digital health. J Glob Health. (2021) 11:16006. doi: 10.7189/jogh.11.16006
151. Braithwaite, J, Mannion, R, Matsuyama, Y, Shekelle, P, Whittaker, S, Al-Adawi, S, et al. Accomplishing reform: successful case studies drawn from the health systems of 60 countries. Int J Qual Health Care. (2017) 29:880–6. doi: 10.1093/intqhc/mzx122
152. McKinsey & Company . How digital tools could boost efficiency in African health systems. Chicago: McKinsey & Company (2023).
153. Kueper, JK . Primer for artificial intelligence in primary care. Can Fam Physician. (2021) 67:889–93. doi: 10.46747/cfp.6712889
154. Lin, SY, Mahoney, MR, and Sinsky, CA. Ten ways artificial intelligence will transform primary care. J Gen Intern Med. (2019) 34:1626–30. doi: 10.1007/s11606-019-05035-1
155. Terry, AL, Kueper, JK, Beleno, R, Brown, JB, Cejic, S, Dang, J, et al. Is primary health care ready for artificial intelligence? What do primary health care stakeholders say? BMC Med Inform Decis Mak. (2022) 22:237. doi: 10.1186/s12911-022-01984-6
156. Amit, G, Girshovitz, I, Marcus, K, Zhang, Y, Pathak, J, Bar, V, et al. Estimation of postpartum depression risk from electronic health records using machine learning. BMC Pregnancy Childbirth. (2021) 21:630. doi: 10.1186/s12884-021-04087-8
157. Black, JE, Kueper, JK, Terry, AL, and Lizotte, DJ. Development of a prognostic prediction model to estimate the risk of multiple chronic diseases: constructing a copula-based model using Canadian primary care electronic medical record data. Int J Popul Data Sci. (2021) 6:1395. doi: 10.23889/ijpds.v5i1.1395
158. Pearce, C, McLeod, A, Rinehart, N, Whyte, R, Deveny, E, and Shearer, M. Artificial intelligence and the clinical world: a view from the front line. Med J Aust. (2019) 210:S38–s40. doi: 10.5694/mja2.50025
159. Wingrove, P, Liaw, W, Weiss, J, Petterson, S, Maier, J, and Bazemore, A. Using machine learning to predict primary care and advance workforce research. Ann Fam Med. (2020) 18:334–40. doi: 10.1370/afm.2550
160. MacRae, J, Darlow, B, McBain, L, Jones, O, Stubbe, M, Turner, N, et al. Accessing primary care big data: the development of a software algorithm to explore the rich content of consultation records. BMJ Open. (2015) 5:e008160. doi: 10.1136/bmjopen-2015-008160
161. Turner, NM, MacRae, J, Nowlan, ML, McBain, L, Stubbe, MH, and Dowell, A. Quantifying the incidence and burden of herpes zoster in New Zealand general practice: a retrospective cohort study using a natural language processing software inference algorithm. BMJ Open. (2018) 8:e021241. doi: 10.1136/bmjopen-2017-021241
162. Scardoni, A, Balzarini, F, Signorelli, C, Cabitza, F, and Odone, A. Artificial intelligence-based tools to control healthcare associated infections: a systematic review of the literature. J Infect Public Health. (2020) 13:1061–77. doi: 10.1016/j.jiph.2020.06.006
163. Patel, VL, Shortliffe, EH, Stefanelli, M, Szolovits, P, Berthold, MR, Bellazzi, R, et al. The coming of age of artificial intelligence in medicine. Artif Intell Med. (2009) 46:5–17. doi: 10.1016/j.artmed.2008.07.017
164. Morgenstern, JD, Rosella, LC, Daley, MJ, Goel, V, Schünemann, HJ, and Piggott, T. AI's gonna have an impact on everything in society, so it has to have an impact on public health: a fundamental qualitative descriptive study of the implications of artificial intelligence for public health. BMC Public Health. (2021) 21:40. doi: 10.1186/s12889-020-10030-x
165. Stalgaitis, CA, Jordan, JW, Djakaria, M, Saggese, DJ, and Bruce, HR. Psychographic segmentation to identify higher-risk teen peer crowds for health communications: validation of Virginia's Mindset Lens Survey. Front Public Health. (2022) 10:864. doi: 10.3389/fpubh.2022.871864
166. Hasikin, K, Lai, KW, Satapathy, SC, Sabanci, K, and Aslan, MF. Editorial: emerging applications of text analytics and natural language processing in healthcare. Front Digital Health. (2023) 5:948. doi: 10.3389/fdgth.2023.1227948
167. Lim, S, and Schmälzle, R. Artificial intelligence for health message generation: an empirical study using a large language model (LLM) and prompt engineering. Front Commun. (2023) 8:82. doi: 10.3389/fcomm.2023.1129082
168. Hudlicka, E . Virtual training and coaching of health behavior: example from mindfulness meditation training. Patient Educ Couns. (2013) 92:160–6. doi: 10.1016/j.pec.2013.05.007
169. Green, N, Rubinelli, S, Scott, D, and Visser, A. Health communication meets artificial intelligence. Patient Educ Couns. (2013) 92:139–41. doi: 10.1016/j.pec.2013.06.013
170. The Lancet . Can artificial intelligence improve cancer care? Lancet Oncol. (2023) 24:577. doi: 10.1016/S1470-2045(23)00240-1
171. Naik, N, Hameed, BMZ, Shetty, DK, Swain, D, Shah, M, Paul, R, et al. Legal and ethical consideration in artificial intelligence in healthcare: who takes responsibility? Front Surgery. (2022) 9:322. doi: 10.3389/fsurg.2022.862322
172. Europarleuropaeu . Report on the proposal for a regulation of the European Parliament and of the council on the protection of individuals with regard to the processing of personal data and on the free movement of such data (general data protection regulation). (2023) Available at: https://www.europarl.europa.eu/doceo/document/A-7-2013-0402_EN.html.
173. Spoladore, D, Sacco, M, and Trombetta, A. A review of domain ontologies for disability representation. Expert Syst Appl. (2023) 228:120467. doi: 10.1016/j.eswa.2023.120467
Keywords: global health, artificial intelligence, public health, global health challenges, machine learning, health care, mortality
Citation: Zaidan AM (2023) The leading global health challenges in the artificial intelligence era. Front. Public Health. 11:1328918. doi: 10.3389/fpubh.2023.1328918
Received: 27 October 2023; Accepted: 13 November 2023;
Published: 27 November 2023.
Edited by:
Dmytro Chumachenko, National Aerospace University – Kharkiv Aviation Institute, UkraineReviewed by:
Daniele Spoladore, Institute of Intelligent Industrial Technologies and Systems for Advanced Manufacturing, ItalyCopyright © 2023 Zaidan. This is an open-access article distributed under the terms of the Creative Commons Attribution License (CC BY). The use, distribution or reproduction in other forums is permitted, provided the original author(s) and the copyright owner(s) are credited and that the original publication in this journal is cited, in accordance with accepted academic practice. No use, distribution or reproduction is permitted which does not comply with these terms.
*Correspondence: Amal Mousa Zaidan, emFpZGFuYW1Aa3NhdS1ocy5lZHUuc2E=
Disclaimer: All claims expressed in this article are solely those of the authors and do not necessarily represent those of their affiliated organizations, or those of the publisher, the editors and the reviewers. Any product that may be evaluated in this article or claim that may be made by its manufacturer is not guaranteed or endorsed by the publisher.
Research integrity at Frontiers
Learn more about the work of our research integrity team to safeguard the quality of each article we publish.