- 1Business School, Beijing Normal University, Beijing, China
- 2College of Business, University of Doha for Science and Technology, Doha, Qatar
- 3School of Business, The Hang Seng University of Hong Kong, Hong Kong, Hong Kong SAR, China
Information and communication technology (ICT) has great potential to propel economic development toward a low-carbon direction. This study aims to investigate the effect of ICT development on total-factor carbon emission efficiency (TFCEE), as well as its public health co-benefits. We use dynamic (threshold) models and a panel of 30 Chinese provinces from 2008 to 2019. The results suggest that ICT significantly and positively impacts the TFCEE. Specifically, for every 10 per cent increase in the internet development index, the TFCEE increases by 0.11 per cent. Moreover, we find that ICT development indirectly improves the TFCEE by promoting green innovation and energy structure optimization. Furthermore, when green innovation (energy structure represented by the share of coal) switches from below to above (above to below) its threshold value, the promotion effect of ICT development on the TFCEE increases. Additionally, the results show that improving the TFCEE can lead to co-benefits in strengthening China's public health. This study delivers novel insights on promoting the TFCEE through the ICT channel and highlights its positive health-related externalities. Furthermore, we offer policy recommendations to Chinese decision-makers, which can apply to other emerging economies battling similar issues.
1 Introduction
While China's economy has grown significantly during the past four decades, its carbon emissions have increased dramatically. Consequently, China is the world's leading greenhouse gas emitter (1). Ample empirical evidence indicates that carbon emissions are among the significant factors threatening public health (2–4). Thus, low-carbon transition has massive potential to bring public health co-benefits by directly reducing carbon dioxide inhalation, mitigating climate change, and reducing air pollutants that share emission sources with CO2 (2, 5–7). Against the above backdrop, Chinese policymakers pledged to peak total CO2 emissions by 2030 and to achieve carbon neutrality by 2060 (8) to maintain a balance between sustainability and carbon emissions. It is estimated that under the conditions of achieving the 1.5 C temperature goal and reaching the peak earlier than 2030, Chinese policymakers could prevent 118,000 (614,000) deaths by 2030 (2050) (9).
Notwithstanding, as an emerging economy, China needs to promote prolonged economic expansion. Thus, in the face of conflicting goals of carbon emissions reduction and continued economic growth, the key to resolving this dilemma is to improve the total-factor carbon emission efficiency (hereafter TFCEE) (10). TFCEE takes the GDP (carbon emissions) as the desired (undesired) output while considering the factor inputs of capital, labor and energy. As such, the TFCEE can effectively measure whether and to what extent regional development models meet the requirements of low-carbon transformation (11). Given the above backdrop, how to effectively improve the TFCEE has become a topical research question in recent environmental studies (10, 12).
Since the 21st century, the integration of information and communication technology (hereafter ICT) with various sectors of the economy has shown an unstoppable trend. Countries worldwide endeavor to develop and harness ICT, with internet technology being an essential part of it. China's public internet access started in 1994, while in August 2013, the State Council issued the Broadband China policy vigorously promoting ICT infrastructure construction. The recent 50th Statistical Report released by the China Internet Network Information Center (13) shows that, between 2008 and 2022, internet penetration in China has more than tripled from 22.6 to 75.6 per cent (see Figure 1).
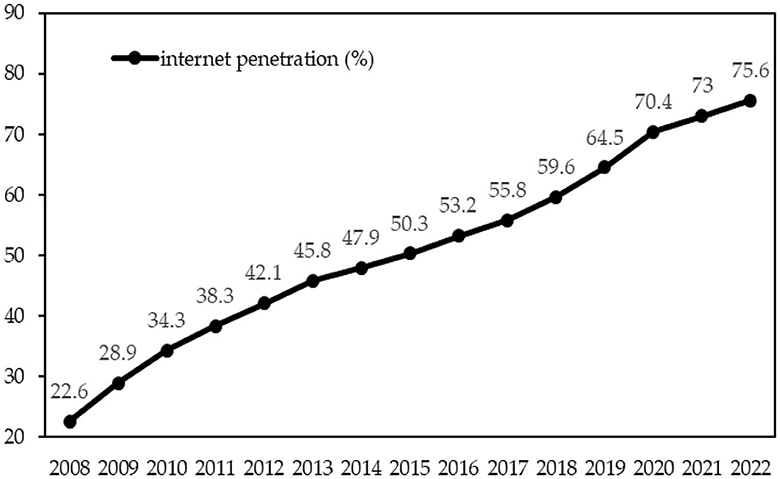
Figure 1. China's internet penetration growth in percentage terms from 2008 to 2022. Source: CNNIC, 2008-2022.
Many studies investigate the effect of ICT or internet development on variables related to energy or carbon emissions, but the results remain inconclusive. Some scholars argue that ICT-related factors reduce carbon intensity (14). Others find that internet development increases enterprise energy efficiency (15) and improves the TFCEE (11, 16). On the contrary (17, 18), document that demand for ICT products increases carbon emissions and energy intensity. Overall, the existing literature has four important shortcomings. First, current studies ignore the suspected path dependence of TFCEE and use static regression models, which may yield estimation bias (11, 16). Second, the mediation and threshold effects between ICT development and TFCEE have not received adequate attention. Third, while limited studies show a positive (negative) association between ICT (carbon emissions) and public health in China (2, 9, 19), the health co-benefits from improving TFCEE remain unexplored.
Given the pivotal role of ICT in propelling China's economic development toward a low-carbon direction and the above-listed gaps in empirical research, our study has four main objectives. First, to investigate the relationship between ICT and TFCEE using a dynamic model. Second, to unveil how ICT impacts TFCEE through the mediation effect of green innovation and energy structure optimization and the threshold effect of ICT at different values of the two mediator variables. Finally, the study directly examines whether and how TFCEE improvement affects public health across 30 Chinese provinces.
Accordingly, the contributions of this study are as follows. We are the first to employ the dynamic model to identify the role of ICT in promoting TFCEE. Specifically, we use the two-step system generalized method of moments (hereafter 2SGMM), which accounts for the suspected path dependence (autoregressive process) of the TFCEE variable and mitigates the endogeneity problem. Moreover, we document nascent empirical evidence of the significantly beneficial effect of ICT on the TFCEE via its positive influence on green innovation and energy structure optimization. Additionally, we are the first to quantify the significantly different impact of ICT on the TFCEE concerning threshold levels of green innovation and energy structure. Finally, we deliver a novel contribution by documenting positive public health-related externalities associated with policies that improve the TFCEE through ICT development.
The remainder of this article is as follows: Section 2 presents a literature review. Section 3 develops the hypotheses, while Section 4 offers data and research methods. In Section 5, we outline and discuss the empirical results. We conclude the study and provide policy suggestions in Section 6.
2 Literature review
2.1 TFCEE measurements
One can measure carbon emission efficiency by single-factor indicators, which usually reflect the interaction between carbon emissions and GDP. For example, CO2 emissions intensity is the ratio of carbon emissions to GDP (1). However, single-factor indicators do not consider all the key inputs in the production process, as well as the substitution effect among inputs. Thus, single-factor indicators are not capable of fully measuring CO2 emission efficiency (10). Because of this limitation, some studies add capital and labor inputs into the analysis of carbon emission efficiency and propose the TFCEE as an alternative superior indicator (20).
Using TFCEE, we can estimate more accurately whether the development pattern meets the sustainable requirement of economic growth and carbon abatement. Data envelopment analysis methods are widely used to calculate the TFCEE (10). For instance, the directional distance function (hereafter DDF) captures the production process, including the undesired output of carbon emissions (21). However, the original DDF model is limited to increasing (reducing) desired (undesired) outputs (and/or inputs) with the same ratio, thereby leading to an overestimation of efficiency when non-zero slacks exist (22). Therefore, scholars propose the non-radial directional distance function (hereafter NDDF) based on slack variables to solve such an acute problem (23).
2.2 ICT development and TFCEE nexus
Scholars examining the multifaceted effects of ICT/internet advancements on energy usage or carbon emissions deliver conflicting evidence. On the one hand, some researchers find that ICT/internet development reduces carbon emissions (24), CO2 per capita (25), carbon intensity (14), and energy consumption (26) while improving energy efficiency (27). On the other hand, others argue that the ICT/internet threatens the sustainability of resources and the environment by increasing CO2 emissions (17) and energy intensity (18). Moreover (28), point out that the impact of ICT on carbon emissions per capita is uncertain by documenting a positive (negative) relationship in the short (long) term. It is worth noting that the studies mentioned above use static models. However, the present value of the TFCEE is significantly influenced by its earlier values, which gives rise to a robust cumulative circularity (12, 29).
Two recent studies exploring the ICT and TFCEE nexus in China use static models and reveal a positive relationship (11, 16). Notwithstanding, some research suggests that there may be a threshold effect concerning the relationship between ICT progress and the environment or energy (30, 31). However, to our knowledge, no studies have investigated the threshold effects in the context of ICT effects on the TFCEE.1 Instead, researchers primarily use simple multivariate linear models, neglecting the jumping character or structural breaks in the relationship between ICT development and the TFCEE caused by changes in other economic variables (11, 16).
Moreover, the relationship between the environment and health has long been one of the research hotspots (9, 32, 33). Studies examining China (1), the United States (2), and OECD countries (3) document the adverse effects of carbon dioxide on residents' health. However, there is a considerable lack of research on the impact of TFCEE on health. Additionally, pregnant women are an environmentally sensitive group (34), and health literature has found a strong correlation between air pollution and maternal complications and mortality risk factors (35). However, the maternal group is often overlooked in environmentally related studies.
2.3 Research gaps
Summing up, the following deficiencies are found in the relevant empirical literature: (1) Existing research has not considered that the TFCEE is usually path-dependent (autoregressive process) and has used static models for estimation. (2) Few studies focus on the mediation and threshold effects between ICT development and the TFCEE. (3) Health benefits of the TFCEE improvement remain under-explored. Our study attempts to fill the above research gaps.
3 Hypotheses development
3.1 The direct effect of ICT development on the TFCEE
ICT relies on electricity, and thus, its development may also decrease the TFCEE. With the operation of technologies, e.g., 5G, cloud computing, and data centers, there is a need for a growing number of energy-intensive infrastructures (17). Furthermore, applying ICT in various fields may create more demand for ICT-related products and services, exerting pressure on energy demand and increasing carbon emissions (24).
Notwithstanding, ICT advancement directly improves the TFCEE in two ways. First, ICT promotes the low-carbon transition of people's work and lifestyles. For instance, paperless transmission, e-commerce, remote offices, and the sharing economy are gaining ground along with the development of ICT. These approaches achieve more effective utilization of resources and reduce emissions of CO2 caused by logistics, transportation, and the functioning of building space (36), thereby directly improving the TFCEE. Second, ICT improvement substantially boosts the level of informatization in other industries. Regarding production, ICT plays a fundamental role in the practical implementation of an energy management system (EMS) (37). The EMS, in turn, automatically controls, adapts, and optimizes the energy networks and orderly configures and schedules the multiple energy systems, thereby increasing the efficiency of the energy system.
Summing up, most studies suggest a generally beneficial influence of ICT development on the TFCEE. In other words, extant empirical evidence indicates that the positive impact of ICT tends to outweigh its adverse side effects on the TFCEE (24). Accordingly, we propose hypothesis 1, stated below.
Hypothesis 1. ICT development positively affects the TFCEE.
3.2 The mediation effect of green innovation
ICT development is conducive to green innovation via the generation, acquisition, processing, and exchange of information (38). Specifically, ICT expansion drives the growth of information related to environmentally sound technologies while improving search efficiency and decreasing the acquisition cost. Moreover, ICT advances dramatically enhance the ability of individuals and organizations to process information and transform enterprises from experience- to data-driven (39). ICT also forms innovation clusters by linking various innovative entities (e.g., firms, universities, R&D institutions, and non-governmental organizations) together, thus facilitating communication, complementarity, and knowledge spillover among innovators (38). The mechanisms mentioned above increase the utilization of relevant information resources and the success rate of green innovation, thus promoting green innovation.
Green innovation, in turn, can significantly improve the TFCEE through the following mechanisms. First, some green technological advances (e.g., capturing and storing CO2) directly contribute to energy savings and carbon reduction (10). Likewise, air quality management and energy storage technologies enable the same output with less energy input and fewer emissions, leading to a higher TFCEE. Second, environmental innovations can help renovate and upgrade pollution treatment equipment (40). As such, assuming it is effectively adopted, green technology innovation optimizes production processes and promotes the low-carbon transformation of energy-intensive enterprises, thereby improving the TFCEE (41). Given the above backdrop, we propose a second hypothesis as follows.
Hypothesis 2. ICT development significantly increases the TFCEE by promoting green innovation.
3.3 The mediation effect of energy structure optimization
ICT has motivated the so-called energy internet development (42), which uses cyberspace to identify, collect, analyse, transmit, and manage energy information. Furthermore, the energy internet integrates internet technology, multi-energy networks and technologies for renewable energy generation, enhancing system resilience and energy utilization efficiency and promoting renewable energy penetration (43). Additionally, the energy internet can overcome barriers to extraction, transmission, and control of large-scale clean energy projects and promote the transformation of economic development from traditional fossil fuels to a clean and efficient energy source (42). This process, in turn, drives the sustainable utilization of energy resources and promotes energy structure optimization. Moreover, with the growing popularity of the energy internet, clean energy usage is continuously expanding (e.g., electric vehicles), which further optimizes the energy structure.
Summing up, energy structure optimization increases the proportion of clean energy sources. Therefore, energy structure optimization can achieve the same economic output with fewer carbon emissions, improving the TFCEE (44). Given the above, we put forth hypothesis 3.
Hypothesis 3. ICT development significantly increases the TFCEE by promoting energy structure optimization.
4 Dataset, variables, and models
4.1 Benchmark regression model
The relationship between ICT development and the TFCEE can be represented by the basic model as follows.
Where TFCEEi, t is the total-factor carbon emission efficiency in Chinese province i and year t. The ICT term represents ICT development, while the Control term represents a vector of five control variables, including provincial population (POP), science and technology expenditure intensity (TECH), economic development (GDP), degree of economic openness (OPEN) and industrial structure (IND). μi and δt are the province and time-fixed effects, respectively. εi, t is a random error term with zero mean, constant variance and is not serially correlated.
Given that the outcome variable TFCEE may be path-dependent (autoregressive process) (12, 29), we introduce a first lag of the dependent variable (TFCEEi, t−1) into the right-hand side of Equation (1). However, dynamic models tend to suffer from endogeneity issues. In contrast, the OLS and the Fixed Effects (FE) estimators would yield biased results (45). Therefore, we use 2SGMM2 (46) to estimate the dynamic model [see Equation (2)], which tests hypothesis 1.
4.2 Mediation effects
Besides its direct effect, ICT development may also have two indirect effects on TFCEE, generally referred to as the mediation effects. To empirically examine such effects, Equation (3) is constructed as follows (47) to test hypotheses 2 and 3.
Where MED represents two mediator variables: green innovation (GINNO) and energy structure (ENER). If the coefficient of λ1is significant, a mediation effect exists, and vice versa (47).
4.3 Dynamic threshold model
With changes in green innovation (GINNO) and energy structure (ENER), the impact mechanism between ICT development and the TFCEE may have a structural change—that is, there may be a threshold effect (30). To examine this issue, we construct a dynamic threshold model (30) and present it below in Equation (4).
Where qi, t is the threshold variable, including green innovation (GINNO) and energy structure (ENER). T represents the threshold value to be estimated. α2(α3) represents the effect of ICT development on the TFCEE when the threshold variable is below (above) the threshold value.
4.4 Data and variables
In line with prior studies (23, 48), we use the NDDF to estimate the TFCEE variable. We use a sample of 30 Chinese provinces (n = 1, 2, …, 30). Each province includes inputs of capital stock (K), calculated by the perpetual inventory method; labor (L), measured by the total number of employees; and energy (E), expressed as the total energy consumption. Desired output GDP (Y) is proxied by provincial real GDP. In contrast, the undesired output of carbon emissions (C) is calculated according to the 2006 Intergovernmental Panel on Climate Change guidelines for national greenhouse gas inventories. Assuming that the optimal solution of each input and output obtained through the NDDF linear programming is . The dependent variable TFCEE can be formulated in Equation (6) (48).
TFCEE ranges from 0 to 1, such that a higher value of the TFCEE indicates higher production efficiency of the province. Consequently, if provincial TFCEE were equal to 1 (0), it would mean that the corresponding province was fully efficient (inefficient).
We construct a comprehensive index to measure ICT advancement, in line with recent studies (49), as shown in Table 1. Additionally, we employ the entropy method to evaluate the ICT development index (ICT variable), which ranges from 0 to 1.
Descriptions of all the variables are presented in Table 2. All variables are at the provincial level. The selection and measurement of the variables align with the prior research (6, 11, 16, 50, 51).
The sample ranges from 2008 to 2019. Due to limited data availability in Hong Kong, Macao, Taiwan, and Tibet, this study focuses on the other 30 provincial administrative regions as the sample. We obtained data from the National Bureau of Statistics, the China Statistical Yearbook, the Statistical Report on China's internet development, and the Chinese Research Data Services Platform. All the data are adjusted for inflation with 2008 as a base period to ensure comparability.
Table 3 presents descriptive statistics for variables. We can observe that the dependent variable TFCEE has the minimum (maximum) value of 0.177 (1), with a mean and standard deviation of 0.430 and 0.157, respectively. The leading explanatory variable, ICT, has a minimum (maximum) value of 0.018 (0.773), with a mean and standard deviation of 0.116 and 0.117. This result suggests substantial cross-provincial variability in the TFCEE and ICT during the sample period.
Figure 2 illustrates the trends of TFCEE (Figure 2A) and ICT (Figure 2B) in China from 2008 to 2019. We can observe that TFCEE and ICT follow a gradually increasing path over time.
5 Results and discussions
5.1 Benchmark regression results
Table 4 shows the estimated results of the dynamic panel regressions corresponding to the benchmark model in Equation (2). Columns (1) and (2) display the estimation results using the OLS and the FE methods. Column (3) shows the output from the 2SGMM estimation technique. The coefficient on the LTFCEE in column (3) is 0.9755 and larger (smaller) than the estimated coefficient from the FE (OLS) method. The results based on the 2SGMM model also pass the m1, m2, and Hansen tests. Due to the above, we can assume that our 2SGMM model is correctly specified. Furthermore, the coefficient on the LTFCEE term in column (3) is positive (within the range of zero to one) and statistically significant. This finding indicates that Chinese provincial-level TFCEE is path-dependent, in line with the recent study (12, 29). Such evidence could mean that because of the difficulty of changing the provincial-level production mode in the short term, the TFCEE changes slowly and exhibits path dependence.
Column (3) also shows that the ICT variable coefficient is statistically significant and positive. Specifically, the coefficient informs us that, on average, for every 10 per cent increase in the ICT development index, we expect the TFCEE to increase by 0.11 per cent, ceteris paribus.3 Therefore, Table 4 implies that ICT development significantly promotes TFCEE improvement, which supports hypothesis 1. This finding supports the conclusions of Hao et al. (30) and Wu et al. (31) about the role of ICT development in driving low-carbon development. The estimated results can be associated with ICT accelerating the intelligent and exemplary management of the whole production process, improving the efficiency of resource allocation, reducing inefficient energy consumption and carbon emissions, and thus improving the TFCEE. China has entered a phase where the pace of economic growth has slowed down. In contrast, the task of carbon emission reduction is urgent due to the associated environmental and health damage, as well as policy commitments, thereby causing the TFCEE improvement to encounter bottlenecks. As such, our findings could inspire strategic planning development in China and across other emerging economies with similar goals and problems.
5.2 The mediation effects of green innovation and energy structure
Environmental innovations promote continuous production process improvement, e.g., by avoiding high carbon emissions at source and fostering the development of end-of-pipe treatment technologies (carbon-storing and reusing) (41). Therefore, green innovation significantly reduces energy saving and carbon reduction costs, thus driving the TFCEE improvement.
Column (1) in Table 5 shows the estimated results from 2SGMM regression models testing for indirect ICT effects in the TFCEE using green innovation as a mediator variable from equation (3). The coefficient on the ICT variable is significant and carries a positive sign, meaning that ICT progress increases green innovation. This aligns with Tang et al. (38), who adopt China's broadband policy as a quasi-natural experiment. Columns (2) and (3) further distinguish between high-quality innovation represented by green inventions (GINV) and low-quality innovation represented by green utility models (GUTI), respectively. Estimated coefficients indicate that ICT advancement has a significantly stronger (statistically and economically) effect on promoting high-quality rather than low-quality green innovation. Such results can be associated with the ICT promoting digital ecology, thereby increasing research and development (38). This comparative advantage, in turn, makes it more likely for enterprises to receive long-term external financial support when conducting quality green innovation research characterized by higher difficulty levels, greater failure rates, and more extended periods (53). Such incentives motivate Chinese enterprises and significantly increase high-quality green innovation.
Because ICT affects green innovation, which, in turn, affects the TFCEE, we can assume that ICT development indirectly affects the TFCEE, which supports hypothesis 2. ICT is deeply integrated into enterprises' production and operation activities, promoting their forward-looking technology research and significantly enhancing green innovation capabilities (54). At the same time, ICT development can enable firms to obtain knowledge and information at a lower marginal cost, break through barriers between industries, and further promote green innovation via the knowledge spillover effects (38). Green innovation, in turn, effectively increases energy efficiency and improves production processes (41), enhancing the TFCEE.
The optimization of the energy structure implies an increase (decrease) in the proportion of renewable energy (fossil fuels) in energy consumption. This can reduce carbon emissions, driving the TFCEE's improvement. The testing results for indirect ICT effects in the TFCEE using energy structure as a mediator variable from equation (3) are displayed in column (4) in Table 5. We can observe that the estimated coefficient on the ICT variable is negative and significant, which means that ICT development reduces coal's share in total energy consumption, i.e., optimizes the energy structure. This corroborates Xu et al. (55), who found that improving digitalization improves energy composition in a global study of 109 countries.
Again, because the ICT affects energy structure, which, in turn, promotes an improvement in the TFCEE, we can assume that the former indirectly affects TFCEE, in line with hypothesis 3. Specifically, ICT changes energy production and consumption patterns through knowledgeable information-based systems while accelerating energy transformation (27). Additionally, the ICT provides technological support for renewable energy development (43), promoting global energy internet construction (42). This helps reduce the use of traditional high-carbon energy sources, fosters the growth of clean energy sources and optimizes the energy structure. Increasing the proportion of clean and renewable energy helps cut carbon emissions, improving the TFCEE.
5.3 Dynamic threshold model results
In this section, we test whether the threshold effect exists in the ICT-TFCEE nexus. The threshold variables include green innovation (GINNO) and energy structure (ENER). In the first step, we conduct 1,000 repetitions of bootstrap self-sampling. Table 6 displays the self-sampling test results of the threshold value and a corresponding confidence interval. The Wald statistics and p-values all pass the significance test, informing that our model is suitable for further analysis.
Having estimated the threshold values for GINNO and ENER variables in Table 6, we run 2SGMM dynamic threshold panel regressions corresponding to Equation (4). The estimated results appear in Table 7, where qit ≤ C (qit > C) indicates that the threshold variable is below or equal to (above) the threshold value. Column (1) shows the results with green innovation (GINNO) as the threshold variable. We can observe that when green innovation exceeds the threshold value, the estimated coefficient on ICT changes from 0.1064 to 0.1737 and remains statistically significant. For instance, at qit ≤ C (qit > C), for every 10 per cent increase in ICT, we would expect the TFCEE to increase on average by 0.29 (0.47) per cent, holding all else constant. This result can be associated with the evidence that at the early stage of green innovation development, enterprises' research costs are high, and green patent application in production is still being explored. Because of that, the potential for TFCEE improvement through ICT development is somewhat limited. However, once the green innovation development exceeds a certain threshold, key technical challenges to improve the TFCEE no longer hold (38). This, in turn, decreases carbon emissions reduction costs and provides new impetus for ICT development to promote the TFCEE.
Column (2) of Table 7 displays estimated results with the energy structure (ENER) as the threshold term. Since ENER is represented by the share of coal in total energy consumption, a decrease in ENER implies optimization of the energy structure. We can observe that as ENER changes from above to below its threshold value, the coefficient on the ICT increases from 0.0932 to 0.1434 and remains statistically significant. The fossil fuel-based energy structure is associated with substantial carbon emissions, which impedes the TFCEE improvement. For instance, some underdeveloped regions in northwest China are highly dependent on fossil fuels, which will weaken their capacity to improve the TFCEE (56). As the cost of renewable energy decreases and the proportion of fossil fuels falls below a certain threshold, a cleaner energy structure can strengthen the relationship between ICT and TFCEE (44).
5.4 The health co-benefits of the TFCEE improvement
Excessive inhalation of carbon dioxide harms the respiratory system and reduces higher-level cognitive abilities (7), directly leading to adverse health effects. CO2 can also indirectly have negative effects on health. For example, carbon dioxide decreases the content of essential nutrients for humans in crops (57); global warming exacerbates air pollution, increases the frequency of extreme weather events, and damages ecosystems (6). Increasing the TFCEE leads to lower carbon emissions for the same output, potentially decreasing adverse health effects on Chinese residents. However, whether the improvement in TFCEE directly influences public health in China has not been empirically tested.
Columns (1–3) of Table 8 display the output from 2SGMM regression models testing for the impact of TFCEE on the three indicators of public health: the ratio of inpatients to total population (INP), the mortality rate per 1,000 persons (MOR), and maternal mortality rate per 1,000 births (MMOR).4 Higher values of these indicators indicate lower levels of public health and vice versa. We can observe that the coefficients of the TFCEE variable in all columns are significantly negative, indicating that TFCEE improves health levels. Accordingly, our analysis offers nascent robust empirical evidence supporting the health co-benefits of ICT driving TFCEE improvement.
5.5 Robustness test
To confirm the reliability of the estimated results presented in Table 4 and the associated research findings, we test their robustness concerning the following four aspects. First, we recalculate the TFCEE using the slack-based measure model (58). Second, we replace the core explanatory variable (ICT). Specifically, we adopt the principal component analysis (PCA) to recalculate the new ICT development index (ICT_PCA). Third, this study re-estimates the benchmark model using the difference GMM estimator. Finally, we rerun the benchmark model after shortening the sample period by 1 year, from 2008–2019 to 2009–2019, to account for the potential impact of the 2008 great financial crisis.
Results corresponding to the four robustness procedures are shown in Table 9. The coefficients on the TFCEE remain statistically significant, carry positive signs, and range from 0.6740 to 0.9512 in columns (1) and (2), respectively. Furthermore, the estimated coefficients on the ICT development variable in all four columns remain statistically significant and positive. Overall, Table 9 confirms that the results and research conclusions from Section 5.1 are robust and further corroborate hypothesis 1.
6 Conclusions and policy implications
Considering the ICT's rapid advancements and the urgency of meeting goals for carbon peaking and net zero, the study takes data from 30 Chinese provinces during the 2008–2019 period. We use the 2SGMM dynamic models to empirically test the impact of ICT development on the TFCEE and the mediation, threshold, and moderating effects, as well as the health co-benefits. This study delivers several significant findings. First, ICT development significantly increases the TFCEE. Second, ICT can indirectly improve TFCEE through green innovation and energy structure optimization. Moreover, when green innovation (energy structure) switches from below to above (above to below) its threshold value, the promotional effect of ICT development on the TFCEE increases substantially in magnitude. Third, TFCEE improvement has significant public health-related co-benefits by significantly reducing the inpatient and mortality ratios.
Three policy implications can be derived from our findings. First, from the perspective of TFCEE improvement, the results suggest policies promoting the cross-industry application of ICT. Primarily, policymakers should focus on the digital and intelligent transformation of energy- and environment-related fields to realize the normalization of ICT applications, decoupling economic growth from carbon emissions and creating new impetus for the promotion of TFCEE. Second, concerning the mediating effects of ICT development on the TFCEE, Chinese decision-makers should foster favorable conditions for realizing the positive ICT effect on green innovation. For instance, they could consider upgrading the intellectual property protection system for green innovation. Moreover, the development of the energy internet should be regarded as an essential direction for energy structure optimization. It is also vital to promote intelligent upgrading of the energy system. Third, from the public health co-benefits perspective, the significant role of TFCEE in decreasing the inpatient and mortality ratios should be emphasized to enhance the motivation of relevant stakeholders in the field of public health toward improving the TFCEE.
Our examination of the ICT-TFCEE nexus is at the provincial level due to the availability of ICT development data. With continuous data enrichment, future research could be conducted at different spatial units, e.g., prefectural and enterprise levels. Moreover, once more data is available, it would be interesting to see whether the recent exogeneous macroeconomic shock of the COVID-19 pandemic and related policies (e.g., lockdowns) affect the documented results and findings. Finally, we advocate further research on the long-run convergence-divergence path in public health across the cities and provinces with different levels of ICT and the TFCEE by applying the distribution dynamics approach (59–61).
Data availability statement
The original contributions presented in the study are included in the article/supplementary material, further inquiries can be directed to the corresponding author.
Author contributions
JL: Conceptualization, Data curation, Software, Visualization, Methodology, Writing — original draft, Writing — review & editing. MW: Conceptualization, Data curation, Supervision, Methodology, Writing — original draft, Writing — review & editing, Project administration. TC: Conceptualization, Supervision, Validation, Writing — review & editing, Project administration.
Funding
The author(s) declare that no financial support was received for the research, authorship, and/or publication of this article.
Conflict of interest
The authors declare that the research was conducted in the absence of any commercial or financial relationships that could be construed as a potential conflict of interest.
Publisher's note
All claims expressed in this article are solely those of the authors and do not necessarily represent those of their affiliated organizations, or those of the publisher, the editors and the reviewers. Any product that may be evaluated in this article, or claim that may be made by its manufacturer, is not guaranteed or endorsed by the publisher.
Footnotes
1. ^Hao et al. (30) and Wu et al. (31) examine the threshold effects of ICT/internet development on electricity intensity or green total factor energy efficiency in China.
2. ^2SGMM is a highly efficient estimation method in tackling the issue of endogeneity (1).
3. ^Because the sample's mean values (see Table 3) of TFCEE (0.430) and ICT (0.116) and the coefficient on ICT (0.0404) in column (3) of Table 4, the average effect on the TFCCE associated with a 10 per cent increase in ICT variable is equal to 0.116*0.1*0.0404/0.430, or 0.11 per cent (52).
4. ^All three variables are at the provincial level and obtained from the China Health Statistical Yearbook.
References
1. Wojewodzki M, Wei Y, Cheong TS, Shi X. Urbanization, agriculture and convergence of carbon emissions nexus: global distribution dynamics analysis. J Clean Prod. (2023) 385:135697. doi: 10.1016/j.jclepro.2022.135697
2. Dong HM, Xue MG, Xiao YJ, Liu YS. Do carbon emissions impact the health of residents? Considering China's industrialization and urbanization. Sci Total Environ. (2021) 758:143688. doi: 10.1016/j.scitotenv.2020.143688
3. Apergis N, Gupta R, Lau CKM, Mukherjee Z. U.S. state-level carbon dioxide emissions: does it affect health care expenditure? Renew Sust Energ Rev. (2018) 91:521–30. doi: 10.1016/j.rser.2018.03.035
4. Kutlu G, Örün E. The effect of carbon dioxide emission, GDP per capita and urban population on health expenditure in OECD countries: a panel ARDL approach. Int J Environ Health Res. (2022) 33:1233–42. doi: 10.1080/09603123.2022.2083088
5. Scovronick N, Budolfson M, Dennig F, Errickson F, Fleurbaey M, Peng W, et al. The impact of human health co-benefits on evaluations of global climate policy. Nat Commun. (2019) 10:2095. doi: 10.1038/s41467-019-09499-x
6. Farooq MU, Shahzad U, Sarwar S, Li ZJ. The impact of carbon emission and forest activities on health outcomes: empirical evidence from China. Environ Sci Pollut Res. (2019) 26:12894–906. doi: 10.1007/s11356-019-04779-x
7. Jacobson TA, Kler JS, Hernke MT, Braun RK, Meyer KC, Funk WE. Direct human health risks of increased atmospheric carbon dioxide. Nat Sustain. (2019) 2:8. doi: 10.1038/s41893-019-0323-1
8. Liu X, Wojewodzki M, Cai Y, Sharma S. The dynamic relationships between carbon prices and policy uncertainties. Technol Forecast Soc Change. (2023) 188:122325. doi: 10.1016/j.techfore.2023.122325
9. Tang R, Zhao J, Liu Y, Huang X, Zhang Y, Zhou D, et al. Air quality and health co-benefits of China's carbon dioxide emissions peaking before 2030. Nat Commun. (2022) 13:1008. doi: 10.1038/s41467-022-28672-3
10. Xu L, Fan M, Yang L, Shao S. Heterogeneous green innovations and carbon emission performance: evidence at China's city level. Energ Econ. (2021) 99:105269. doi: 10.1016/j.eneco.2021.105269
11. Lin B, Zhou Y. Does the Internet development affect energy and carbon emission performance? Sustain Prod Consum. (2021) 28:1–10. doi: 10.1016/j.spc.2021.03.016
12. Ran QY, Liu L, Razzaq A, Meng YX, Yang XD, Attewell P. Does green finance improve carbon emission efficiency? Experimental evidence from China. Environ Sci Pollut Res. (2021) 74:252–9.
13. CNNIC. The 51st statistical report on China's internet. (2022). Available online at: https://www.cnnic.com.cn/IDR/ReportDownloads/202212/P020221209344717199824.pdf (accessed July 23, 2023).
14. Sun H, Kim G. The composite impact of ICT industry on lowering carbon intensity: from the perspective of regional heterogeneity. Technol Soc. (2022) 66:101661. doi: 10.1016/j.techsoc.2021.101661
15. Li M, Du W. Can internet development improve the energy efficiency of firms: empirical evidence from China. Energ. (2021) 237:121590. doi: 10.1016/j.energy.2021.121590
16. Wang J, Dong K, Sha Y, Yan C. Envisaging the carbon emissions efficiency of digitalisation: the case of the internet economy for China. Technol Forecast Soc Change. (2022) 184:121965. doi: 10.1016/j.techfore.2022.121965
17. Charfeddine L, Kahia M. Do information and communication technology and renewable energy use matter for carbon dioxide emissions reduction? Evidence from the middle east and North Africa region. J Clean Prod. (2021) 327:129410. doi: 10.1016/j.jclepro.2021.129410
18. Zhou X, Zhou D, Wang Q. How does information and communication technology affect China's energy intensity? A three-tier structural decomposition analysis. Energy. (2018) 151:748–59. doi: 10.1016/j.energy.2018.03.115
19. Zhang JP, Gong XM, Zhang H, ICT. diffusion and health outcome: effects and transmission channels. Telemat Inform. (2022) 67:101755. doi: 10.1016/j.tele.2021.101755
20. Wang L, Liu B, He Y, Dong Z, Wang S. Have public environmental appeals inspired green total factor productivity? Empirical evidence from Baidu environmental search index. Environ Sci Pollut Res. (2022) 30:30237–52. doi: 10.1007/s11356-022-23993-8
21. Chung YH, Färe R, Grosskopf S. Productivity and undesirable outputs: a directional distance function approach. J Environ Manage. (1997) 51:229–40. doi: 10.1006/jema.1997.0146
22. Fukuyama H, Weber WL, A. directional slacks-based measure of technical inefficiency. Socio-Econ Plan Sci. (2009) 43:274–87. doi: 10.1016/j.seps.2008.12.001
23. Zhou P, Ang BW, Wang H. Energy and CO2 emission performance in electricity generation: a non-radial directional distance function approach. Eur J Oper Res. (2012) 221:625–35. doi: 10.1016/j.ejor.2012.04.022
24. Wang J, Zhao J, Dong K, Dong X. How does the internet economy affect CO2 emissions? Evidence from China. Appl Econ. (2023) 55:447–66. doi: 10.1080/00036846.2022.2089623
25. Shobande OA, Asongu SA. The critical role of education and ICT in promoting environmental sustainability in eastern and southern Africa: a panel var approach. Technol Forecast Soc Chang. (2022) 176:121480. doi: 10.1016/j.techfore.2022.121480
26. Kouton J. Information communication technology development and energy demand in African countries. Energy. (2019) 189:116192. doi: 10.1016/j.energy.2019.116192
27. Zhao S, Hafeez M, Faisal CMN. Does ICT diffusion lead to energy efficiency and environmental sustainability in emerging Asian economies? Environ Sci Pollut Res. (2022) 29:12198–207. doi: 10.1007/s11356-021-16560-0
28. Shobande OA. Decomposing the persistent and transitory effect of information and communication technology on environmental impacts assessment in Africa: evidence from Mundlak specification. Sustainability. (2021) 13:9. doi: 10.3390/su13094683
29. Wang Q, Zhang C, Li R. Towards carbon neutrality by improving carbon efficiency - a system-GMM dynamic panel analysis for 131 countries' carbon efficiency. Energy. (2022) 258:124880. doi: 10.1016/j.energy.2022.124880
30. Hao Y, Li Y, Guo Y, Chai J, Yang C, Wu H. Digitalization and electricity consumption: does internet development contribute to the reduction in electricity intensity in China? Energ Policy. (2022) 164:112912. doi: 10.1016/j.enpol.2022.112912
31. Wu H, Hao Y, Ren S, Yang X, Xie G. Does internet development improve green total factor energy efficiency? Evidence from China. Energy Policy. (2021) 153:112247. doi: 10.1016/j.enpol.2021.112247
32. Prüss-Üstün A, Bonjour S, Corvalán C. The impact of the environment on health by country: a meta-synthesis. Environ Health. (2008) 7:7. doi: 10.1186/1476-069X-7-7
33. Manisalidis I, Stavropoulou E, Stavropoulos A, Bezirtzoglou E. Environmental and health impacts of air pollution: a review. Front Public Health. (2020) 8:14. doi: 10.3389/fpubh.2020.00014
34. Wang YJ, Chen XH, Ren SG. Clean energy adoption and maternal health: evidence from China. Energy Econ. (2020) 84:104517. doi: 10.1016/j.eneco.2019.104517
35. Yan ML, Liu NN, Fan YF, Ma LK, Guan TJ. Associations of pregnancy complications with ambient air pollution in China. Ecotox Environ Safe. (2022) 241:113727. doi: 10.1016/j.ecoenv.2022.113727
36. Romm J. The internet and the new energy economy. Resour Conserv Recycl. (2002) 36:197–210. doi: 10.1016/S0921-3449(02)00084-8
37. Rathor SK, Saxena D. Energy management system for smart grid: an overview and key issues. Int J Energ Res. (2020) 44:4067–109. doi: 10.1002/er.4883
38. Tang C, Xu Y, Hao Y, Wu H, Xue Y. What is the role of telecommunications infrastructure construction in green technology innovation? A firm-level analysis for China. Energy Econ. (2021) 103:105576. doi: 10.1016/j.eneco.2021.105576
39. Chatterjee S, Chaudhuri R, Vrontis D. Does data-driven culture impact innovation and performance of a firm? An empirical examination. Ann Oper Res. (2021) doi: 10.1007/s10479-020-03887-z
40. Feng S, Chong Y, Yu H, Ye X, Li G. Digital financial development and ecological footprint: evidence from green-biased technology innovation and environmental inclusion. J Clean Prod. (2022) 380:135069. doi: 10.1016/j.jclepro.2022.135069
41. Du K, Li J. Towards a green world: how do green technology innovations affect total-factor carbon productivity? Energ Policy. (2019) 131:240–50. doi: 10.1016/j.enpol.2019.04.033
42. Wang K, Yu J, Yu Y, Qian Y, Zeng D, Guo S, et al. survey on energy internet: architecture, approach, and emerging technologies. IEEE Syst J. (2018) 12:2403–16. doi: 10.1109/JSYST.2016.2639820
43. Zheng J, Wang X. Can mobile information communication technologies (ICTs) promote the development of renewables? Evidence from seven countries. Energy Policy. (2021) 149:112041. doi: 10.1016/j.enpol.2020.112041
44. Liu Y, Zhao G, Zhao Y. An analysis of Chinese provincial carbon dioxide emission efficiencies based on energy consumption structure. Energy Policy. (2016) 96:524–33. doi: 10.1016/j.enpol.2016.06.028
45. Wojewodzki M, Poon WPH, Shen J. The role of credit ratings on capital structure and its speed of adjustment: an international study. Eur J Finance. (2018) 24:735–60. doi: 10.1080/1351847X.2017.1354900
46. Blundell R, Bond S. Initial conditions and moment restrictions in dynamic panel data models. J Econom. (1998) 87:115–43. doi: 10.1016/S0304-4076(98)00009-8
47. Dell M. The persistent effects of Peru's mining mita. Econometrica. (2010) 78:1863–903. doi: 10.3982/ECTA8121
48. Zhang N, Kong F, Choi Y, Zhou P. The effect of size-control policy on unified energy and carbon efficiency for Chinese fossil fuel power plants. Energ Policy. (2014) 70:193–200. doi: 10.1016/j.enpol.2014.03.031
49. Ren S, Hao Y, Xu L, Wu H, Ba N. Digitalization and energy: how does internet development affect China's energy consumption? Energ Econ. (2021) 98:105220. doi: 10.1016/j.eneco.2021.105220
50. Guo M, Kuai Y, Liu X. Stock market response to environmental policies: evidence from heavily polluting firms in China. Econ Model. (2020) 86:306–16. doi: 10.1016/j.econmod.2019.09.028
51. Wu HT, Ba N, Ren SY, Xu L, Chai JX, Irfan M, et al. The impact of internet development on the health of Chinese residents: Transmission mechanisms and empirical tests. Socio-Econ Plan Sci. (2022) 81:101178. doi: 10.1016/j.seps.2021.101178
52. Firth M, Malatesta PH, Xin Q, Xu L. Corporate investment, government control, and financing channels: evidence from China's listed companies. J Corp Finance. (2012) 18:433–50. doi: 10.1016/j.jcorpfin.2012.01.004
53. Huang H, Mbanyele W, Wang F, Song M, Wang Y. Climbing the quality ladder of green innovation: does green finance matter? Technol Forecast Soc Change. (2022) 184:122007. doi: 10.1016/j.techfore.2022.122007
54. Glavas C, Mathews S. How international entrepreneurship characteristics influence internet capabilities for the international business processes of the firm. Int Bus Rev. (2014) 23:228–45. doi: 10.1016/j.ibusrev.2013.04.001
55. Xu Q, Zhong M, Li X. How does digitalization affect energy? International evidence. Energ Econ. (2022) 107:105879. doi: 10.1016/j.eneco.2022.105879
56. Mi ZF, Sun XL. Provinces with transitions in industrial structure and energy mix performed best in climate change mitigation in China. Commun Earth Environ. (2021) 2:182. doi: 10.1038/s43247-021-00258-9
57. Zhu CW, Kobayashi K, Loladze I, Zhu JG, Jiang Q, Xu X, et al. Carbon dioxide (CO2) levels this century will alter the protein, micronutrients, and vitamin content of rice grains with potential health consequences for the poorest rice-dependent countries. Sci Adv. (2018) 4:5. doi: 10.1126/sciadv.aaq1012
58. Tone K. A slacks-based measure of efficiency in data envelopment analysis. Eur J Oper Res. (2001) 130:498–509. doi: 10.1016/S0377-2217(99)00407-5
59. Cheong TS, Wu Y, Wojewodzki M, Ma N. The impacts of globalization on inequality in the post-covid-19 world: evidence from China. Front Public Health. (2021) 9:790312. doi: 10.3389/fpubh.2021.790312
60. Williams AD, Cheong TS, Wojewodzki M. Transitional dynamics and the evolution of information transparency: a global analysis. Estud Econ. (2022) 49:31–62. doi: 10.4067/S0718-52862022000100031
Keywords: information and communication technology development, total-factor carbon emission efficiency, health, dynamic threshold model, moderating effect
Citation: Linghu J, Wojewodzki M and Cheong TS (2024) Effects of information and communication technology on total-factor carbon emission efficiency and the health co-benefits. Front. Public Health 11:1301627. doi: 10.3389/fpubh.2023.1301627
Received: 25 September 2023; Accepted: 11 December 2023;
Published: 05 January 2024.
Edited by:
Hai Fang, Peking University, ChinaReviewed by:
Marian Rizov, University of Lincoln, United KingdomWei Lang, Sun Yat-sen University, China
Copyright © 2024 Linghu, Wojewodzki and Cheong. This is an open-access article distributed under the terms of the Creative Commons Attribution License (CC BY). The use, distribution or reproduction in other forums is permitted, provided the original author(s) and the copyright owner(s) are credited and that the original publication in this journal is cited, in accordance with accepted academic practice. No use, distribution or reproduction is permitted which does not comply with these terms.
*Correspondence: Tsun Se Cheong, amFtZXNjaGVvbmdAaHN1LmVkdS5oaw==