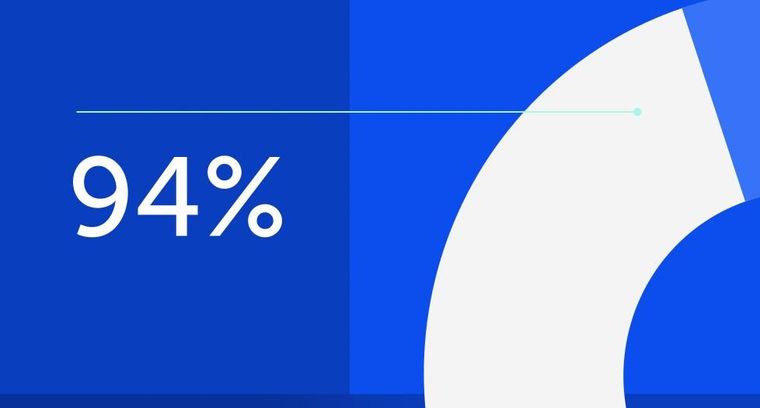
94% of researchers rate our articles as excellent or good
Learn more about the work of our research integrity team to safeguard the quality of each article we publish.
Find out more
ORIGINAL RESEARCH article
Front. Public Health, 03 August 2023
Sec. Infectious Diseases: Epidemiology and Prevention
Volume 11 - 2023 | https://doi.org/10.3389/fpubh.2023.1224175
Background: The impact of seasonal influenza vaccination (SIV) on mortality is still controversial; some studies have claimed that increasing vaccination coverage rates is beneficial, while others have found no significant association. This study aimed to construct a granular longitudinal dataset of local VCRs and assess their effect on pneumonia- and influenza-related (P&I) mortality among Italian adults aged ≥ 65 years.
Methods: NUTS-3 (nomenclature of territorial units for statistics) level data on SIV coverage were collected via a survey of local data holders. Fixed- and random-effects panel regression modeling, when adjusted for potential confounders, was performed to assess the association between local SIV coverage rates and P&I mortality in older adults.
Results: A total of 1,144 local VCRs from 2003 to 2019 were ascertained. In the fully adjusted fixed-effects model, each 1% increase in vaccination coverage was associated (P < 0.001) with a 0.6% (95% CI: 0.3–0.9%) average over-time decrease in P&I mortality. With an annual average of 9,293 P&I deaths in Italy, this model suggested that 56 deaths could have been avoided each year by increasing SIV coverage by 1%. The random-effects model produced similar results. The base-case results were robust in a sensitivity analysis.
Conclusion: Over the last two decades, Italian jurisdictions with higher SIV uptake had, on average, fewer P&I deaths among older adults. Local policy-makers should implement effective strategies to increase SIV coverage in the Italian senior population.
Compared with other age-classes, older adults display a disproportionately high risk of severe influenza outcomes (1). Annual vaccination is the best way to prevent seasonal influenza, and older adults are the primary target group for seasonal influenza vaccination (SIV) (2, 3).
The effects of SIV on influenza-related endpoints can be established by evaluating: (i) vaccine efficacy (risk reduction resulting from vaccination, as measured in randomized controlled trials); (ii) vaccine effectiveness (risk reduction resulting from vaccination, as measured in observational studies) and (iii) vaccine impact (reduction in the incidence of influenza-related outcomes in a population in which some subjects are vaccinated, as measured through modeling) (4). Although randomized studies are the gold standard technique for evaluating vaccine-induced protection, their external validity may be poor, since some population strata (e.g., the oldest old, subjects with multimorbidity or polypharmacy) are usually systematically excluded (5, 6). In this regard, well-designed observational studies can address the issue of inclusiveness, thereby complementing experimental evidence (5). On the other hand, both experimental and observational studies are usually conducted within a limited time-span (typically 1–3 seasons) that is characterized by specific patterns of viral circulation and degrees of vaccine match. For instance, across the last 18 seasons, overall SIV effectiveness in the United States (US) ranged from 10% (7) to 60% (8). From the policy-makers' perspective, it should be stressed that VE estimates alone are not a measure of the full population benefit of SIV and should be interpreted contextually (9). In this regard, modeling studies on vaccine impact may further support policy decisions.
The benefits of SIV on mortality in older adults is an ongoing controversy (10). While several time-series analyses, which attributed ~5% of all winter deaths to influenza, failed to demonstrate a decline in influenza-related mortality on increasing the vaccination coverage rate (VCR), some cohort studies have reported a paradoxically high (~50%) relative reduction in the total risk of winter mortality. Overestimation of the effect of SIV may be driven by both the frailty selection bias and the use of non-specific outcomes, such as all-cause (AC) mortality (10). Similarly, previous Italian modeling studies (11, 12) have reached different conclusions. Rizzo et al. (11) did not find a significant decrease in pneumonia- and influenza-related (P&I) and AC excess mortality following an increase in SIV coverage rates over 31 influenza seasons. Despite its long period of observation, that study (11) did not consider large between-region differences in both socio-structural dynamics and SIV policies. A later study by Fallani et al. (12) attempted to overcome the above-mentioned shortcomings by analyzing a panel dataset in which 21 Italian regions were monitored over 7 seasons. The authors concluded that each 1% increase in SIV uptake by older adults was associated (P < 0.001) with a 1.6–1.9% decrease in P&I mortality. However, while panel data are better able to capture unobserved effects than the analysis of historical trends (13), the study by Fallani et al. (12) was limited by its low number of observations in both time-series and cross-sectional components of the panel.
The ecological and evolutionary dynamics of infections like influenza play out over a broad range of interconnected temporal, spatial and organizational dimensions (14). Influenza modeling and trend assessment can further integrate the individual-level experimental and observational evidence by assessing the average impact of SIV campaigns on the incidence of influenza-related outcomes when complex spatio-temporal, socio-economic and policy-related factors are taken into account (14, 15). By establishing a granular space-time dataset, the present study aimed to assess the locally varying dynamic association between SIV uptake and influenza-related mortality in the Italian older population.
In this ecological study, we adopted an econometric panel data approach, in which each cross-sectional spatial unit was tracked over time. The study period was determined by the availability of outcome data, and covered 17 consecutive years (from 2003 to 2019). Years from 2020 onwards were not considered, owing to the impact of COVID-19 on both P&I mortality and influenza virus circulation. The spatial unit considered in the study was a single Province/Metropolitan City (N = 103/110), which is equivalent to the lowest Eurostat NUTS-3 (nomenclature of territorial units for statistics) level (16). Indeed, modeling at a higher level of spatial granularity allows to capture previously unseen associations or to uncover finer details of the known relationships. By incorporating time trend and sufficiently granular spatial components, panel datasets are able to dramatically increase the sample size and to identify and measure effects that are not detectable in pure time-series or cross-sectional data (13).
Data on P&I mortality and potential confounders were taken from publicly available sources (17–20), while NUTS-3 level VCRs among individuals aged ≥ 65 years (VCR65+) were established ad hoc (see below). NUTS-3 data for both outcomes and confounders were available for all study years. If NUTS-3 VCR65+ data were not available in a region or not routinely collected, the previous NUTS-2 level data on VCR65+ (21), outcomes and confounders were used. Indeed, imputing missing NUTS-3 VCR65+ data (both missing years and missing provinces) was judged unfeasible.
The study population was composed of Italian adults aged ≥ 65 years. During the whole study period, SIV was recommended and fully reimbursed for all subjects aged ≥ 65 years (22).
The study endpoint was the P&I mortality rate (per 10,000) among adults aged ≥ 65 years (P&I65+) observed in a NUTS-3 location i during the year t and derived from death certificates codified according to the international classification of diseases (ICD) (ICD-8: 470–474 and 480–486; ICD-9: 487 and 480–486; ICD-10: J09–J11 and J12–J18 for influenza and pneumonia, respectively) (17).
The Italian Ministry of Health routinely collects and reports (21) VCR65+ on the NUTS-2 level in 19 Regions and two Autonomous Provinces of Trento and Bolzano only. To obtain the more granular NUTS-3 VCR65+ (% of older adults vaccinated), a search of local datasets, statistical compendiums and other relevant documents was first conducted. Potential local data holders were then contacted and asked to provide these statistics for the longest possible time-span.
The list of potential confounders and effect modifiers was determined through an internal discussion and previous research (11, 12). These included independent variables related to socio-economic, environmental, healthcare and virological domains (Table 1) (17–20).
The association between P&I65+ and VCR65+ was examined by applying both fixed-effects (FE) and Swamy-Arora random-effects (RE) models for unbalanced panels implemented in the plm R package (23). The model structure may be presented as follows:
for i = 1…110 and t = 2003…2019, where β1 and βx are regression coefficients; “Confounders” is a set of potential confounders presented in Table 1; α is the unobserved time-invariant local effect (in the FE model) or constant intercept (in the RE model); i is a location; t is a year; ε is the error term.
The degree of imbalance, which was driven by some missing VCR65+, in the base-case multivariable model was low (γ = 0.92; ν = 0.93). The main advantage of the FE models is that they take into account potential bias from omitted time-invariant variables by eliminating between variation from the estimation. On the other hand, RE models may be more efficient; however, if individual effects are correlated with regressors, the RE estimation would be inconsistent (13, 24, 25). Hausman's specification test was used to select between the models (23). For all models, rates that were not percentages were loge-transformed and interpreted as elasticities (e.g., the regression coefficient for VCR65+ is a percent change in P&I65+ associated with a 1% change in VCR65+) (26). Considering the presence of cross-sectional dependence (Pesaran's test: P < 0.001) and serial correlation (Breusch-Godfrey/Wooldridge's test: P < 0.001), the Driscoll-Kraay robust covariance matrix estimator was used to establish heteroskedasticity and autocorrelation consistent (HAC) standard errors (SEs) and associated p-values. Finally, a sensitivity analysis was conducted in which all NUTS-2 units (N = 379) were excluded.
All analyses were performed in R stat packages v.4.2.2 (R Foundation for Statistical Computing; Vienna, Austria).
NUTS-3 VCR65+ data were obtained for 14 out of 20 Italian regions, and were available for 7–17 seasons. In summary, a total of 1,144 local VCR65+ were included in the analysis (Supplementary Table 1).
Table 2 summarizes the distribution patterns of both the study outcome and independent variables. On univariate FE analysis, each 1% increase in VCR65+ was associated with a 1.7% (95% CI: 1.5–2.0%) average over-time decrease in P&I65+. The unadjusted RE coefficient was the same. Other statistically significant crude associations included proportions of ≥ 75-year-olds and children, baseline mortality, gross domestic product (GDP), average January temperature, and patterns of circulating viruses (Table 2).
Table 2. Summary statistics of the study variables and unadjusted fixed- and random-effects models on the association between pneumonia- and influenza-related mortality among Italian older adults and potential predictors.
In the fully adjusted analysis (Table 3), the parameter estimates of both the FE and RE models were similar, although Hausman's test suggested (P = 0.001) that the RE model was inconsistent. The FE model showed that each 1% increase in VCR65+ was associated with a 0.6% (95% CI: 0.3–0.9%) average over-time decrease in P&I65+. GDP, baseline mortality and proportion of children were positive predictors of P&I65+. Analogously, as compared with seasonal pre-2009 H1N1s subtype, seasons (co)dominated by A(H3N2) and B strains were associated with higher P&I65+. By contrast, each 1°C increase in the average January temperature was associated (P = 0.001) with a 1.3% decrease in P&I65+. The FE and RE models explained 48.2 and 53.4% of variance, respectively (Table 3).
Table 3. Multivariable fixed- and random-effects models on the association between pneumonia- and influenza-related mortality and Influenza vaccination coverage rates among Italian older adults.
In the sensitivity analysis (Supplementary Table 2), the regression output was similar, suggesting the robustness of the base-case model.
Models are undoubtedly useful tools for structured decision-making and enable the principal stakeholders to consider the potential impact of VCRs on pre-defined population health outcomes. Importantly, models are more useful as tools for forecasting the likely pattern of disease-specific endpoints that might emerge under various circumstances over time (27). In this study, we collected a large amount of local SIV VCR65+ and used these to populate an econometric model of the impact of SIV over 17 years. Our model showed that each 1% increase in VCR65+ was associated with a 0.6% (95% CI: 0.3–0.9%) average over-time decrease in P&I65+. During the study period, an annual average of 9,293 P&I deaths in older adults were reported in Italy (17). Translated into absolute numbers, our model suggests that an average of 56 P&I deaths could have been avoided each year by increasing VCR by only 1%.
As mentioned earlier, previous Italian modeling studies (11, 12, 28) on the population-level benefits of increasing SIV VCRs have reached somewhat contrasting conclusions. A cyclic Serfling-type model on 31-year monthly data (1970–2001) implemented by Rizzo et al. (11) failed to demonstrate any marked association between VCRs and P&I/AC excess mortality in adults aged 65–84 years. Specifically, in the period (1970–1986) of low SIV VCR (< 8%) a significant (P ≤ 0.002) declining trend in both P&I and AC excess mortality rates over time was discerned. By contrast, in the period (1987–2001) of rapidly increasing SIV uptake, the authors found no evidence of any trend in either P&I (P = 0.36) or AC (P = 0.75) excess mortality (11). On the other hand, a correlational 12-season (from 2005/06 to 2016/17) study by Manzoli et al. (28) reported a significant (P = 0.036) linear increase in the annual incidence of influenza-like illness (ILI) following the registered decline in VCR65+. Each one-point increase in VCR65+ was associated with an 8.6% (95%: 1.0–16.7%) reduction in the ILI attack rate. In other words, at least 2,690 ILI cases in the Italian senior population could have been prevented by increasing VCR65+ by 1% (28). Owing to some methodological similarities, our results are more similar to the longitudinal FE model by Fallani et al. (12), who reported a significant inverse relationship between P&I65+ and VCR65+. However, the adjusted regression coefficient for VCR65+ in our study was about three times lower (−0.006 vs. −0.019), while the unadjusted (−0.017) regression coefficient approached that of Fallani's study (12). This difference is probably ascribable to the fact that the previous model was not corrected for the baseline mortality rate, which alone explained 37.1% of variance in our study. Contrary to our approach, which was based on 1,144 space-time observations, the study by Fallani et al. (12) included only 147 regression points and was limited to the post-2009 pandemic period. In sum, the inconsistencies in both the present and past research (11, 12, 28) are apparently driven by the modeling approach, time period and influenza-related proxy measures used. First, by incorporating intra-country dynamics, panel models have advantages over time-series, as they allow for heterogeneity across spatial units, thereby increasing the reliability of results, may be more efficient, and suffer less from deficient statistical power (13). Second, the relative frequency of influenza-related population-wide indicators may have changed over recent decades. Indeed, evidence from the US (29) has highlighted that, unlike pneumonia caused by Streptococcus pneumoniae and Haemophilus influenzae, the rates of both hospitalization and case-fatality due to pneumonia caused by influenza virus increased from 2002 to 2011 by 132% (P < 0.001) and 67% (P < 0.001), respectively. Similarly, during the current study period of 2003–2019, an increasing trend (Mann-Kendall trend test: P < 0.001; results not shown) in P&I65+ was reported in Italy (17). These observations contrast with those from the earlier time period (1970–2001) investigated in the study by Rizzo et al. (11). Finally, the sensitivity and specificity of influenza-related proxies may have a dramatic impact on SIV effectiveness (4). Indeed, it has been noted (30) that evaluating the benefits of SIV at the population level is challenging, since some disease proxies may lack statistically significant findings.
The main limitation of the present study is that several NUTS-3 observations were missing, especially in southern regions. Ideally, the 17-year dataset of 103/110 provinces would include 1,751/1,870 space-time records. Moreover, the availability of more granular VCR data depends on the digitalization of immunization registries, which has been implemented asymmetrically across Italian regions. Second, while the FE model controls for time-invariant unobserved variables (e.g., different local policies), we cannot exclude the presence of time-varying omitted variables. For instance, Fallani et al. (12) concluded that in addition to the overall benefit of increasing VCR65+, Italian regions with a higher market share of the MF59-adjuvanted trivalent SIV showed a further decrease in P&I65+. However, data on the procurement of single SIV types are typically available for NUTS-2 level only and these data cover only a limited time-span. Finally, P&I65+ is not a laboratory-confirmed endpoint, while laboratory confirmation is the most specific outcome and is currently considered to be the gold standard in SIV efficacy and effectiveness studies (4). On the other hand, among other registry-based influenza-related outcomes [e.g., AC mortality, which is not recommended for SIV effectiveness research (4)], endpoints related to influenza and/or pneumonia are among the most specific and, at the same time, more sensitive than laboratory-confirmed influenza (31). This means that the P&I65+ outcome would additionally cover cases that are not tested for influenza and cases of pneumonia initially triggered by influenza virus, which is the most common complication (32). On the assumption that 10% of pneumonia deaths are initially caused by influenza, and that the true effectiveness of SIV is 50%, the expected effectiveness against mortality due to pneumonia would be only 5% (4). We must therefore acknowledge that the observed effect estimate is probably underestimated.
In conclusion, following the construction of a granular longitudinal dataset, this analysis revealed that increasing SIV VCRs yielded significant population-level benefits. National and local policy-makers and other stakeholders should implement effective strategies designed to enhance the community's demand for and access to SIV, with the final aim of achieving a minimum VCR of 75%.
The original contributions presented in the study are included in the article/Supplementary material, further inquiries can be directed to the corresponding author.
AD, AO, and GI conceptualized the study. AD, DP, MO, AB, FB, DC, MC, CC, DF, EP, CR, EV, and The FluCov Study Group collected data. AD performed the data analysis and wrote the first draft of the manuscript. All authors provided edits to the manuscript draft.
Daniela Amicizia and Federico Grammatico: Regional Health Agency of Liguria, Genoa, Italy and Department of Health Sciences, University of Genoa, Genoa, Italy; Catia Rosanna Borriello: Directorate General for Health, Lombardy Region, Milan, Italy; Francesco Chini: Directorate for Health and Social Policy, Lazio Region, Rome, Italy; Lorenza Ferrara: Regional Epidemiology Reference Service for the Surveillance, Prevention and Control of Infectious Diseases, Local Health Unit of Alessandria, Alessandria, Italy; Fabio Filippetti: Regional Health Agency, Ancona, Italy; Daniela Loconsole and Francesca Centrone: Department of Interdisciplinary Medicine, University of Bari, Aldo Moro Policlinico, Bari, Italy; Mario Palermo: Regional Epidemiological Observatory, Health Department, Sicily Region, Palermo, Italy; Simone Pizzini: Department of Public Health and Pediatrics, University of Turin, Turin, Italy.
The authors declare that the research was conducted in the absence of any commercial or financial relationships that could be construed as a potential conflict of interest.
All claims expressed in this article are solely those of the authors and do not necessarily represent those of their affiliated organizations, or those of the publisher, the editors and the reviewers. Any product that may be evaluated in this article, or claim that may be made by its manufacturer, is not guaranteed or endorsed by the publisher.
The Supplementary Material for this article can be found online at: https://www.frontiersin.org/articles/10.3389/fpubh.2023.1224175/full#supplementary-material
1. Paget J, Spreeuwenberg P, Charu V, Taylor RJ, Iuliano AD, Bresee J, et al. Global mortality associated with seasonal influenza epidemics: new burden estimates and predictors from the GLaMOR Project. J Glob Health. (2019) 9:020421. doi: 10.7189/jogh.09.020421
2. World Health Organization (WHO). Vaccines against influenza: WHO position paper–May 2022. Wkly Epidemiol Rec. (2022) 97:185–208.
3. World Health Organization (WHO). Seasonal Influenza Vaccines: An Overview for Decision-Makers. (2020). Available online at: https://apps.who.int/iris/bitstream/handle/10665/336951/9789240010154-eng.pdf
4. World Health Organization (WHO). Evaluation of Influenza Vaccine Effectiveness: A Guide to the Design and Interpretation of Observational Studies. (2017). Available online at: https://apps.who.int/iris/bitstream/handle/10665/255203/9789241512121-eng.pdf (accessed July 3, 2023).
5. Tan YY, Papez V, Chang WH, Mueller SH, Denaxas S, Lai AG. Comparing clinical trial population representativeness to real-world populations: an external validity analysis encompassing 43 895 trials and 5 685 738 individuals across 989 unique drugs and 286 conditions in England. Lancet Healthy Longev. (2022) 3:e674–89. doi: 10.1016/S2666-7568(22)00186-6
6. Flores LE, Frontera WR, Andrasik MP, Del Rio C, Mondríguez-González A, Price SA, et al. Assessment of the inclusion of racial/ethnic minority, female, and older individuals in vaccine clinical trials. JAMA Netw Open. (2021) 4:e2037640. doi: 10.1001/jamanetworkopen.2020.37640
7. Belongia EA, Kieke BA, Donahue JG, Greenlee RT, Balish A, Foust A, et al. Effectiveness of inactivated influenza vaccines varied substantially with antigenic match from the 2004–2005 season to the 2006–2007 season. J Infect Dis. (2009) 199:159–67. doi: 10.1086/595861
8. Treanor JJ, Talbot HK, Ohmit SE, Coleman LA, Thompson MG, Cheng PY, et al. Effectiveness of seasonal influenza vaccines in the United States during a season with circulation of all three vaccine strains. Clin Infect Dis. (2012) 55:951–9. doi: 10.1093/cid/cis574
9. Hollingsworth R, El Guerche-Séblain C, Tsai T, Vasiliev Y, Lee S, Bright H, et al. Assessment of the benefits of seasonal influenza vaccination: elements of a framework to interpret estimates of vaccine effectiveness and support robust decision-making and communication. Influenza Other Respir Viruses. (2021) 15:164–74. doi: 10.1111/irv.12786
10. Simonsen L, Taylor RJ, Viboud C, Miller MA, Jackson LA. Mortality benefits of influenza vaccination in elderly people: an ongoing controversy. Lancet Infect Dis. (2007) 7:658–66. doi: 10.1016/S1473-3099(07)70236-0
11. Rizzo C, Viboud C, Montomoli E, Simonsen L, Miller MA. Influenza-related mortality in the Italian elderly: no decline associated with increasing vaccination coverage. Vaccine. (2006) 24:6468–75. doi: 10.1016/j.vaccine.2006.06.052
12. Fallani E, Orsi A, Signori A, Icardi G, Domnich A. An exploratory study to assess patterns of influenza- and pneumonia-related mortality among the Italian elderly. Hum Vaccin Immunother. (2021) 17:5514–21. doi: 10.1080/21645515.2021.2005381
13. Hsiao C. Panel data analysis—advantages and challenges. Test. (2007) 16:1–22. doi: 10.1007/s11749-007-0046-x
14. Heesterbeek H, Anderson RM, Andreasen V, Bansal S, De Angelis D, Dye C, et al. Modeling infectious disease dynamics in the complex landscape of global health. Science. (2015) 347:aaa4339. doi: 10.1126/science.aaa4339
15. Ding C, Liu X, Yang S. The value of infectious disease modeling and trend assessment: a public health perspective. Expert Rev Anti Infect Ther. (2021) 19:1135–45. doi: 10.1080/14787210.2021.1882850
16. Eurostat. NUTS-Nomenclature of territorial units for statistics. (2023). Available online at: https://ec.europa.eu/eurostat/web/nuts/background (accessed July 3, 2023).
17. Italian Institute of Statistics (ISTAT). Health for all. (2023). Available online at: https://www.istat.it/it/archivio/14562 (accessed July 3, 2023).
18. System of regional provincial social indicators (SISREG). Gross domestic product pro capita. (2023). Available online at: https://www.sisreg.it/index.php?option=com_content&view=article&id=102&Itemid=75 (accessed July 3, 2023).
19. Italian National Institute for the Environmental Protection Research (ISPRA). National system for the elaboration and diffusion of climatic data. (2023). Available online at: www.scia.isprambiente.it (accessed July 3, 2023).
20. Italian Institute of Health (ISS). InfluNet–Integrated surveillance system of influenza. (2023). Available online at: https://w3.iss.it/site/rmi/influnet/Default.aspx (accessed July 3, 2023).
21. Italian Ministry of Health. Influenza vaccination coverage. (2023). Available online at: https://www.salute.gov.it/imgs/C_17_tavole_19_3_1_file.pdf (accessed July 3, 2023).
22. Grossi A, Bella A, Caraglia A, Maraglino F, Rizzo C. La copertura vaccinale antinfluenzale in Italia: andamento nella popolazione generale e ultrasessantaquattrenne, dal 1999–2000 al 2019–2020. Boll Epidemiol Naz. (2021) 2:1–7.
23. Croissant Y, Millo G. Panel data econometrics in R: the plm package. J Stat Soft. (2008) 27:1–43. doi: 10.18637/jss.v027.i02
24. Clark TS, Linzer DA. Should I use fixed or random effects? Pol Sci Res Method. (2015) 3:399–408. doi: 10.1017/psrm.2014.32
25. Arellano M, Honoré B. Panel data models: some recent developments. In:Heckman JJ and Leamer E, , editors. Handbook of Econometrics. New York, NY: Elsevier. (2001). p. 3229–96. doi: 10.1016/S1573-4412(01)05006-1
26. Goldhaber-Fiebert JD, Lipsitch M, Mahal A, Zaslavsky AM, Salomon JA. Quantifying child mortality reductions related to measles vaccination. PLoS ONE. (2010) 5:e13842. doi: 10.1371/journal.pone.0013842
27. Thompson J, McClure R, Scott N, Hellard M, Abeysuriya R, Vidanaarachchi R, et al. A framework for considering the utility of models when facing tough decisions in public health: a guideline for policy-makers. Health Res Policy Syst. (2022) 20:107. doi: 10.1186/s12961-022-00902-6
28. Manzoli L, Gabutti G, Siliquini R, Flacco ME, Villari P, Ricciardi W. Association between vaccination coverage decline and influenza incidence rise among Italian elderly. Eur J Public Health. (2018) 28:740–2. doi: 10.1093/eurpub/cky053
29. Wuerth BA, Bonnewell JP, Wiemken TL, Arnold FW. Trends in pneumonia mortality rates and hospitalizations by organism, United States, 2002–2011. Emerg Infect Dis. (2016) 22:1624–7. doi: 10.3201/eid2209.150680
30. Yang L, Chan KP, Wong CM, Chiu SSS, Magalhaes RJS, Thach TQ, et al. Comparison of influenza disease burden in older populations of Hong Kong and Brisbane: the impact of influenza and pneumococcal vaccination. BMC Infect Dis. (2019) 19:162. doi: 10.1186/s12879-019-3735-7
31. Domnich A, de Waure C. Comparative effectiveness of adjuvanted versus high-dose seasonal influenza vaccines for older adults: a systematic review and meta-analysis. Int J Infect Dis. (2022) 122:855–63. doi: 10.1016/j.ijid.2022.07.048
32. Lapi F, Domnich A, Marconi E, Rossi A, Cricelli C. Adjuvanted versus non-adjuvanted standard-dose influenza vaccines in preventing all-cause hospitalizations in the elderly: a cohort study with nested case-control analyses over 18 influenza seasons. Expert Rev Vaccines. (2022) 21:1647–53. doi: 10.1080/14760584.2022.2115362
Keywords: influenza, vaccination, vaccine impact, older adults, modeling, Italy
Citation: Domnich A, Orsi A, Panatto D, Ogliastro M, Barca A, Bert F, Cereda D, Chironna M, Costantino C, Fiacchini D, Pariani E, Rizzo C, Volpe E, Icardi G and The FluCoV Study Group (2023) Population-level benefits of increasing influenza vaccination uptake among Italian older adults: results from a granular panel model. Front. Public Health 11:1224175. doi: 10.3389/fpubh.2023.1224175
Received: 17 May 2023; Accepted: 17 July 2023;
Published: 03 August 2023.
Edited by:
Lorenzo Giovanni Mantovani, University of Milano-Bicocca, ItalyReviewed by:
Pietro Ferrara, Italian Auxological Institute (IRCCS), ItalyCopyright © 2023 Domnich, Orsi, Panatto, Ogliastro, Barca, Bert, Cereda, Chironna, Costantino, Fiacchini, Pariani, Rizzo, Volpe, Icardi and The FluCoV Study Group. This is an open-access article distributed under the terms of the Creative Commons Attribution License (CC BY). The use, distribution or reproduction in other forums is permitted, provided the original author(s) and the copyright owner(s) are credited and that the original publication in this journal is cited, in accordance with accepted academic practice. No use, distribution or reproduction is permitted which does not comply with these terms.
*Correspondence: Alexander Domnich, YWxleGFuZGVyLmRvbW5pY2hAaHNhbm1hcnRpbm8uaXQ=
Disclaimer: All claims expressed in this article are solely those of the authors and do not necessarily represent those of their affiliated organizations, or those of the publisher, the editors and the reviewers. Any product that may be evaluated in this article or claim that may be made by its manufacturer is not guaranteed or endorsed by the publisher.
Research integrity at Frontiers
Learn more about the work of our research integrity team to safeguard the quality of each article we publish.