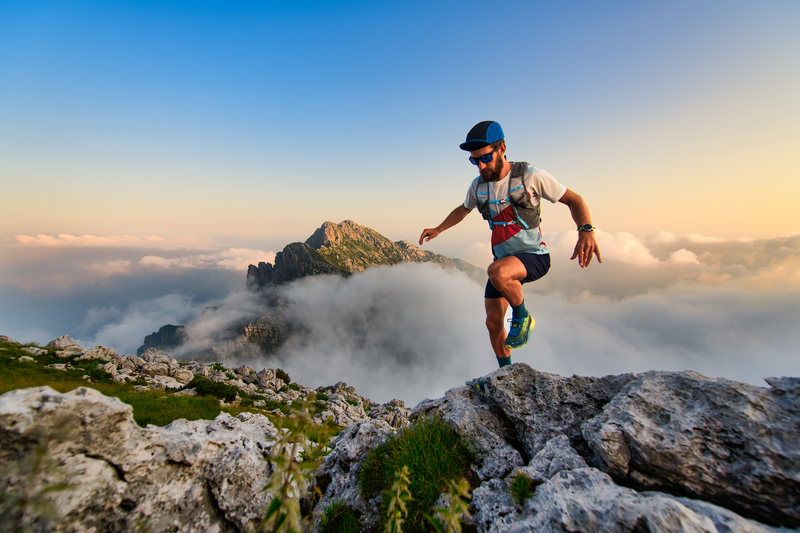
94% of researchers rate our articles as excellent or good
Learn more about the work of our research integrity team to safeguard the quality of each article we publish.
Find out more
ORIGINAL RESEARCH article
Front. Public Health , 27 July 2023
Sec. Environmental Health and Exposome
Volume 11 - 2023 | https://doi.org/10.3389/fpubh.2023.1212301
This article is part of the Research Topic Air Pollution as a Risk Factor Affecting Human Health and Economic Costs View all 18 articles
Purpose: The incidence of primary liver cancer is increasing year by year, with environmental factors playing a non-negligible role. At present, many studies are still disputing whether air pollution is associated with primary liver cancer incidence, and it is difficult to draw causal inferences. Therefore, in this study, we used two-sample Mendelian randomization (MR) to assess the causal relationship between air pollution (including PM2.5, PM2.5–10, PM10, nitrogen dioxide and nitrogen oxides) and primary liver cancer risk and its related biomarkers (Alpha-fetoprotein, Osteopontin, Glypican-3 and Arginase-1).
Patients and methods: We used large-scale publicly available genome-wide association studies (GWAS) summary data to conduct MR analyses of European and East Asian populations. Inverse variance weighted (IVW) method was used as the main analysis method, and weighted median model, MR-Egger, simple model and weighted model methods were selected for quality control. Heterogeneity was checked by the Cochran’s Q test. The MR-Egger regression and the MR-PRESSO global test detect pleiotropy. The sensitivity analysis was performed using the leave-one-out method.
Results: Between air pollution and primary liver cancer in either European (PM2.5: p = 0.993; PM2.5–10: p = 0.833; PM10: p = 0.257; nitrogen dioxide: p = 0.215; nitrogen oxides: p = 0.614) or East Asian (PM2.5: p = 0.718; PM2.5–10: p = 0.362; PM10: p = 0.720; nitrogen dioxide: p = 0.101; nitrogen oxides: p = 0.760) populations were found no statistical association. Notably, there was a causal relationship between nitrogen oxides and Arginase-1, a biomarker associated with hepatocellular differentiation, statistically significant associations remained after deletion for single nucleotide polymorphisms (SNPs) associated with alcohol intake frequency, Body mass index (BMI) and cancers (Beta: 4.46; 95%CI: 0.83–8.08; p = 0.015). There was no heterogeneity or pleiotropy in the results.
Conclusion: This MR study found no evidence to support a causality between air pollution and primary liver cancer in European and East Asian populations, but nitrogen oxides may affect hepatocellular differentiation.
Primary liver cancer is a malignant tumor originating from liver cells or intrahepatic bile duct epithelial cells, which is composed of hepatocellular carcinoma, cholangiocarcinoma, and mixed carcinoma (1). The estimated global cancer incidence rate for primary liver cancer in 2018 was 9.3 per 100,000 person-years, with a corresponding mortality rate of 8.5, making it the sixth most common cancer and the fourth leading cause of cancer death globally (2, 3). Currently, the region with the highest rates and prevalence of primary liver cancer is East Asia. However, from 1978 to 2012, the incidence of primary liver cancer has also increased year-on-year in regions with low incidence rates, such as most European countries, India and the United States, placing a huge burden on individuals, families and society (4). Diagnosis of primary liver cancer relies on pathological biopsies, and the detection of tumor biomarkers also plays an important role in early screening, diagnosis, treatment assessment, recurrence and prognosis prediction of tumors (1). Alpha-fetoprotein (AFP), Osteopontin (OPN), Glypican-3 (GPC-3) and Arginase-1 (Arg-1) are common primary liver cancer related biomarkers. AFP, derived from fetal hepatocytes and yolk sacs, has been widely used as a useful cancer biomarker in the diagnosis of liver cancers (5). OPN is a highly modified, phosphorylated and glycosylated extracellular matrix protein that binds to integrins and is expressed in a variety of cells. When combined with AFP, the sensitivity of OPN in the diagnosis of liver cancers increases to 65% (6). GPC-3, a membrane-bound heparin sulfate proteoglycan belonging to the glycoprotein family, is over-expressed in up to 80% of patients with hepatocellular carcinoma and can distinguish liver cancer from other malignancies (7). Arg-1, an enzyme associated with the hydrolysis of arginine to ornithine and urea, is highly sensitive and specific for the detection of malignant hepatocytes and is considered a useful biomarker of hepatocellular differentiation (8, 9). Hepatitis B virus (HBV) infection is the most important driver of primary liver cancer, but with the increase of HBV vaccination coverage, HBV infection has been effectively controlled, and the increase in incidence and mortality from primary liver cancer may be more attributable to other factors such as smoking, alcohol consumption and dietary habits (10, 11). In addition, the impact of environmental factors cannot be ignored as industrialization and associated pollution from burning fossil fuels, or coal, oil and gas, and vehicle emissions increase.
As a long-standing and widespread industrial pollutant, air pollution poses a worrying health hazard. Air pollutants are usually classified as particulate matter (PM), or as gases such as nitrogen dioxide (NO2), nitrogen oxides (NOx), sulfur dioxide (SO2), carbon monoxide (CO), and ozone (O3), etc. (12). PM2.5 is the most frequently inspected pollutant, followed by NO2 and NOx, with few studies focusing on other pollutants. From 1990 to 2015, the death rate attributable to PM2.5 increased from 3.5 to 4.2 million, accounting for 7.6 percent of the total global deaths (13). The high health hazard of PM2.5 is the main reason for its widespread concern. The International Agency for Research on Cancer (IARC) classifies air pollutants as Group I human carcinogens, and numerous studies have shown a positive association between air pollution and some cancers, such as lung, kidney and breast cancer (14–16).
There is no consensus on the association between air pollution and the risk of primary liver cancer. Although a study involving 20,221 participants in the United States found a positive association between PM2.5 and liver cancer mortality (HR was 1.18 by 5 μg/m3 increase in PM2.5; 95% CI: 1.16–1.20) (17), among the 8 studies on PM2.5 and the risk of primary liver cancer, only three studies (Coleman et al., Pan et al. and Vo Pham et al.) showed significant association between PM2.5 and primary liver cancer risk, while the other five studies did not (18–22). However, in five studies on the association between NO2 and liver cancer incidence, only So et al. found that an increase of NO2 concentration of 10 μg/m3 would affect the risk of liver cancer (HR = 1.17, 95%CI:1.02–1.35) (16, 20, 21). Therefore, to further explore whether there is an association between air pollution and the risk of primary liver cancer, we conducted a Mendelian randomization (MR) study using large-scale publicly available genome-wide association studies (GWAS) data with PM2.5, PM2.5–10, PM10, nitrogen dioxide and nitrogen oxides as exposures and primary liver cancer and its related biomarkers (Alpha-fetoprotein, Osteopontin, Glypican-3, and Arginase-1) as outcome to assess the causal relationship between air pollution and the risk of primary liver cancer.
Our study is based on the Mendelian randomization design, which depends on three core assumptions that instrumental variants (1) are associated with the exposure, (2) are not associated with the outcome via a confounding pathway, and (3) do not affect the outcome directly, only possibly indirectly via the exposure. In this study, air pollution (PM2.5, PM2.5–10, PM10, nitrogen dioxide and nitrogen oxides) was used as the exposure factor, single nucleotide polymorphisms (SNPs) significantly related to air pollution were used as instrumental variables (IVs), and primary liver cancer and its related biomarkers (Alpha-fetoprotein, Osteopontin, Glypican-3, and Arginase-1) were the outcome variable. Here, we conducted a two-sample MR analysis to estimate the causal effects of air pollution and primary liver cancer. The flowchart of this Mendelian randomization study is presented in Supplementary Figure 1.
The data sources are detailed in Table 1. We selected air pollution (including PM2.5, PM2.5–10, PM10, nitrogen dioxide and nitrogen oxides) as exposures, with data on all air pollution obtained from UK Biobank, a large prospective study with more than half a million United Kingdom participants for which data on phenotypes, genetic details, and genome-wide genotyping have been published (23, 24). We used the GWAS summary databases of air pollution for populations in Europe and East Asia. In European populations, the PM2.5 (GWAS ID: ukb-b-10,817), PM2.5–10 (GWAS ID: ukb-b-12,963), PM10 (GWAS ID: ukb-b-589), nitrogen dioxide (GWAS ID: ukb-b-2,618) and nitrogen oxides (GWAS ID: ukb-b-12,417) GWAS summary datasets included 423,796, 423,796, 455,314, 456,380, 456,380 participants, respectively. Among East Asian populations, the PM2.5 (GWAS ID: ukb-e-24006_EAS), PM2.5–10 (GWAS ID: ukb-e-24008_EAS), PM10 (GWAS ID: ukb-e-24005_EAS), nitrogen dioxide (GWAS ID: ukb-e-24016_EAS) and nitrogen oxides (GWAS ID: ukb-e-24004_EAS) GWAS summary datasets included 2,505, 2,505, 2,505, 2,625, 2,625 participants, respectively. Air pollution-related indicators were measured by land use regression (LUR) models (25).
Table 1. Summary of the genome-wide association studies (GWAS) included in this two-sample MR study.
We used primary liver cancer and its related biomarkers (Alpha-fetoprotein, Osteopontin, Glypican-3 and Arginase-1) as the outcome, and all GWAS data for primary liver cancer were obtained from FinnGen (European population) and Biobank Japan (East Asian population). The four biomarkers (only European population) Alpha-fetoprotein, Osteopontin, Glypican-3 and Arginase-1 were derived from the prot-a-53 (26), ebi-a-GCST90010244 (27), prot-c-4842_62_2 (28) and ebi-a-GCST90010286 (27) GWAS summary data. FinnGen is a large public-private research project that combines imputed genotype data generated from newly collected and legacy samples from the Finnish biobank and digital health record data from the Finnish Health Registry1 to provide new insights into disease genetics. As of August 2020, samples from 412,000 people have been collected and 224,737 have been analyzed, with 500,000 participants expected by the end of 20,232 (29, 30). Biobank Japan is a large patient-based biobank consisting of 200,000 patients. As a basic biobank for common disease gene research, the project has conducted genome-wide association studies for various diseases and identified many genetic variants associated with disease susceptibility and drug response. All publications are in this project from the project web site2 and open to the public (31). GWAS summary dataset of primary liver cancer in European populations (GWAS ID: finn-b-C3_LIVER_INTRAHEPATIC_BILE_DUCTS) contained 218,752 individuals (including 304 cases and 218,448controls). GWAS summary dataset of primary liver cancer in East Asian populations (GWAS ID: bbj-a-158) contained 197,611 individuals (including 1,866 cases and 195,745controls). Primary liver cancer cases were identified according to clinical diagnosis and conformed to the International Classification of Diseases, 8th Revision and 10th Revision codes.
As shown in Supplementary Figure 1, in order to satisfy assumption 1, p < 5 × 10−8 was used as the genome-wide significance threshold for exposure, but only PM2.5 (European, ukb-b-10,817), PM10 (European, ukb-b-589), nitrogen dioxide (European, ukb-b-2,618) and nitrogen oxides (European, ukb-b-12,417) were able to pick out enough SNPs. Previous studies have shown that linear regression of each genetic variant on the risk factor with p < 5 × 10−6 as the screening criterion results in a low probability of weak instrumental variable bias in the MR Analysis (32, 33), so we lowered the genome-wide significance threshold of the remaining exposure (ukb-b-12,963, ukb-e-24006_EAS, ukb-e-24008_EAS, ukb-e-24005_EAS, ukb-e-24016_EAS and ukb-e-24004_EAS) to p < 5 × 10−6 to select enough SNPs as IVs associated with this significance level.
In order to remove SNPs with linkage disequilibrium (LD), r2 < 0.001 and kb > 10,000 was set when extracting IVs. If the selected SNP was not collected in the resulting GWAS, the proxy SNP in linkage disequilibrium (r2 > 0.8) was used. Palindromic SNPs were then removed to ensure that the effect of these SNPs on exposure corresponded to the same allele as the effect on outcome. Finally, we calculated the R2 (R2 = 2◊EAF◊(1–EAF)◊β2) (34) and F-statistic (F = β2/SE2) (35) for each SNP. R2 is the percentage of iron status variability explained by each SNP and F statistic to assess the presence of a weak IV bias. The F-statistic of each SNP we selected was > 10, suggesting that the genetic instruments selected strongly predicted the exposure (36). For specific SNP information and corresponding R2 and F-statistic, shown in Supplementary Tables 1–7.
To assess the causal relationship between air pollution and primary liver cancer and its related biomarkers, we used the inverse variance weighted (IVW) method to predict the genetic predictive value of the exposure factor for the outcome variable with an effect value of β. IVW can obtain an estimate of causal effect based on a single genetic IV through Wald ratio, and then select a fixed effect model to perform a meta-analysis of multiple estimates of causal effect based on a single gene IV, which can provide a reliable estimate of causal effect and is widely used in MR Analysis (32, 37). In order to further improve the reliability and accuracy of the study results, weighted median model, MR-Egger, simple model and weighted model methods were further used to verify the causal relationship between exposure factors and results, and were verified in both European and Asian populations (38). Biomarkers were only analyzed in European populations due to lack of GWAS data in East Asian populations.
First of all, we used leave-one-out method to test the sensitivity of the remaining SNPs after deleting SNPs one by one. If the results changed significantly, it indicated that the removed SNPs might be directly related to the results, which violated assumption 3 (39). Then, for the IVW method, Cochran’s Q test was used to evaluate the heterogeneity, and p > 0.05 indicated that there was no significant heterogeneity in the selected IVs (40). Finally, we need to perform pleiotropic tests using MR-Egger regression and MR-PRESSO global testing to ensure that IV does not influence the risk of primary liver cancer through other confounding factors or other biological pathways unrelated to air pollution exposure. The MR Egger regression effect model allows for causal estimation of pleiotropic effect corrections, evaluating instrumental intensity under the direct effect assumption independently of the null causality assumption, and MR-PRESSO enables a systematic assessment of the role of pleiotropy (41). The statistical threshold for IVs without pleiotropy was p > 0.05.
All analyses were performed using the packages “TwoSampleMR” (42) and “MR-PRESSO” (41) in R version 4.2.2. The threshold of statistical significance for evidence is p < 0.05.
The MR results are shown in Table 2 (European population) and Table 3 (Asian population), as well as in Figure 1 (Scatter plots, European population), Figure 2 (Scatter plots, Asian population), Figure 3 (Forest plots, European population) and Figure 4 (Forest plots, Asian population).
Table 2. Mendelian randomization (MR) analysis of air pollution (particulate matter, nitrogen dioxide and nitrogen oxides, exposure) with primary liver cancer outcome in European population.
Table 3. Mendelian randomization (MR) analysis of air pollution (particulate matter, nitrogen dioxide and nitrogen oxides, exposure) with primary liver cancer outcome in Asian population (East Asia).
Figure 1. Scatter plots for causal single nucleotide polymorphism (SNP) effect of air pollution (particulate matter, nitrogen dioxide and nitrogen oxides) on primary liver cancer in European population. Each black point representing each SNP on the exposure (horizontal-axis) and on the outcome (vertical-axis) is plotted with error bars corresponding to each standard error (SE). The slope of each line corresponds to the combined estimate using each method of the inverse variance weighted (light blue line), the MR-Egger (blue line), the simple mode (light green line), the weighted median (green line), and the weighted mode (pink line). (A) PM2.5; (B) PM2.5–10; (C) PM10; (D) Nitrogen dioxide; (E) Nitrogen oxides.
Figure 2. Scatter plots for causal SNP effect of air pollution (particulate matter, nitrogen dioxide and nitrogen oxides) on primary liver cancer in Asian population. Each black point representing each SNP on the exposure (horizontal-axis) and on the outcome (vertical-axis) is plotted with error bars corresponding to each standard error (SE). The slope of each line corresponds to the combined estimate using each method of the inverse variance weighted (light blue line), the MR-Egger (blue line), the simple mode (light green line), the weighted median (green line), and the weighted mode (pink line). (A) PM2.5; (B) PM2.5–10; (C) PM10; (D) Nitrogen dioxide; (E) Nitrogen oxides.
Figure 3. Forest plots of Leave-one-out analyses for causal SNP effect of air pollution (particulate matter, nitrogen dioxide and nitrogen oxides) on primary liver cancer in European population. The error bars indicate the 95% confidence interval (CI). (A) PM2.5; (B) PM2.5–10; (C) PM10; (D) Nitrogen dioxide; (E) Nitrogen oxides.
Figure 4. Forest plots of Leave-one-out analyses for causal SNP effect of air pollution (particulate matter, nitrogen dioxide and nitrogen oxides) on primary liver cancer in Asian population. The error bars indicate the 95% confidence interval (CI). (A) PM2.5; (B) PM2.5–10; (C) PM10; (D) Nitrogen dioxide; (E) Nitrogen oxides.
To assess the causal effect of air pollution (including PM2.5, PM2.5–10, PM10, nitrogen dioxide, and nitrogen oxides) on primary liver cancer, we first performed MR Analysis in a European population. 8, 23, 22, 4, and 8 SNPs for PM2.5, PM2.5–10, PM10, nitrogen dioxide, and nitrogen oxides were identified after removal of chained unbalanced IVs (Supplementary Table 1). Using IVW, weighted median model, MR-Egger, simple model and weighted model methods, we found no evidence of a causal relationship between air pollution and primary liver cancer risk (IVW method, PM2.5: p = 0.993; PM2.5–10: p = 0.833; PM10: p = 0.257; nitrogen dioxide: p = 0.215; nitrogen oxides: p = 0.614), and there was no evidence of significant heterogeneity or horizontal pleiotropy (Table 2). The scatter plots of the causal relationships between air pollution and the risk of primary liver cancer are shown in Figure 1. Leave-one-out analyses (Figure 3) showed that removing each SNP in turn had little effect on the results, suggesting that no single SNP had a significant effect on the overall causal effect estimates.
The MR analysis was repeated in the East Asian population to enhance the confidence of the above results. 4, 3, 5, 6, and 4 SNPs for PM2.5, PM2.5–10, PM10, nitrogen dioxide, and nitrogen oxides were identified after removal of chained unbalanced IVs (Supplementary Table 2). Consistent with the findings in the European population, we found no causal relationship between air pollution and primary liver cancer risk in the East Asian population using the above five methods (IVW method, PM2.5: p = 0.718; PM2.5–10: p = 0.362; PM10: p = 0.720; nitrogen dioxide: p = 0.101; nitrogen oxides: p = 0.760), and no significant heterogeneity and pleiotropy were found (Table 3). The scatter plots are shown in Figure 2. Leave-one-out analyses also did not identify abnormal SNPs (Figure 4).
The MR results are shown in Table 4, as well as in Supplementary Figures 2–5 (Scatter plots), and Supplementary Figures 6–9 (Forest plots).
Table 4. Mendelian randomization (MR) analysis of air pollution (particulate matter, nitrogen dioxide and nitrogen oxides, exposure) with biomarkers in primary liver cancer in European population (IVW method).
In order to further verify the causal relationship between air pollution and primary liver cancer, we selected four biomarkers (Alpha-fetoprotein, Osteopontin, Glypican-3 and Arginase-1) which are closely related to primary liver cancer as the outcome and conducted MR Analysis again. Consistent with the above results, we did not find any causal association between air pollution and Alpha-fetoprotein (PM2.5: p = 0.370; PM2.5–10: p = 0.405; PM10: p = 0.842; nitrogen dioxide: p = 0.585; nitrogen oxides: p = 0.652), Osteopontin (PM2.5: p = 0.695; PM2.5–10: p = 0.780; PM10: p = 0.517; nitrogen dioxide: p = 0.615; nitrogen oxides: p = 0.271) and Glypican-3 (PM2.5: p = 0.228; PM2.5–10: p = 0.058; PM10: p = 0.814; nitrogen dioxide: p = 0.388; nitrogen oxides: p = 0.405) through IVW method. The results showed no heterogeneity or pleiotropy (Table 4).
It is worth mentioning that we initially extracted 8 SNPs (rs1217106, rs12203592, rs1318845, rs6749467, rs72808024, rs7514956, rs77205736, and rs77255816) that were strongly associated with nitrogen oxides as instrumental variables and found a significant association between nitrogen oxides and Arginase-1 using IVW method (Beta: 3.56; 95%CI: 0.63–6.49; p = 0.017). Subsequently, we searched these 8 SNPs for possible confounding related to primary liver cancer through the PhenoScanner database (http://www.Phenoscanner.medschl.Cam.ac.uk/ accessed on March 12, 2023) one by one, and found 3 SNPs (rs1217106, rs12203592 and rs77205736) related to alcohol intake frequency, cancers (breast cancer, nonmelanoma skin cancer, cutaneous squamous cell carcinoma, and basal cell carcinoma) and Body mass index (BMI). After deleting them, we analyzed again and found that the results were still statistically significant (Beta: 4.46; 95%CI: 0.83–8.08; p = 0.015), and there was no heterogeneity or pleiotropy. The specific SNP information, corresponding R2 and F-statistic are shown in Supplementary Tables 3–7.
This study is the first to evaluate the causal relationship between air pollution (including PM2.5, PM2.5–10, PM10, nitrogen dioxide and nitrogen oxides) and primary liver cancer using MR methods. We found no genetic evidence of an association between air pollution and primary liver cancer risk in either the European or East Asian populations. However, in a further MR analysis of air pollution and biomarkers associated with primary liver cancer (Alpha-fetoprotein, Osteopontin, Glypican-3 and Arginase-1), we found a significant association between nitrogen oxides and biomarker Arginase-1 related to hepatocellular differentiation (Beta: 3.56; 95%CI: 0.63–6.49; p = 0.017), which remained statistically significant after adjustment (Beta: 4.46; 95%CI: 0.83–8.08; p = 0.015) for possible confounding alcohol intake frequency, body mass index (BMI) and cancers (breast cancer, nonmelanoma skin cancer, cutaneous squamous cell carcinoma, and basal cell carcinoma).
Our findings further confirm several population-based cohort studies. Marie Pedersen et al. (20) pooled data from 174,770 participants in four cohorts [Diet, Cancer and Health study (43), Vorarlberg Health Monitoring and Promotion Program (44), European Prospective Investigation into Cancer and Nutrition (EPIC)-Varese (45) and EPIC-Turin (46)] from European Study of Cohorts for Air Pollution Effects (ESCAPE) in Denmark, Austria, and Italy to examine the association between air pollution (including PM2.5, PM2.5–10, PM10, nitrogen dioxide and nitrogen oxides) and the risk of primary liver cancer, and land-use regression models were used to measure PM2.5, PM2.5–10, PM10, nitrogen dioxide and nitrogen oxides. During an average follow-up period of 17 years, 279 patients with liver cancer were diagnosed, and the meta-analysis found that PM2.5, PM2.5–10, PM10, nitrogen dioxide and nitrogen oxides were all associated with an increased incidence of primary liver cancer, with Hazard ratios (HRs) greater than 1 for all exposures, but none of the associations were statistically significant. After adjusting for age, sex, smoking, drinking, high-risk occupations and other confounding factors that might be associated with primary liver cancer, no statistical association was found between air pollution and primary liver cancer. Unlike our findings, another prospective cohort study in Taiwan (19) followed 23,820 participants without a history of liver cancer for an average of 16.9 years suggested that every 0.73 μg/m3 increase in PM 2.5 increased the risk of hepatocellular carcinoma incidence by 22% in Penghu Islets (HR = 1.22, 95%CI: 1.02–1.47). However, PM2.5 levels in Taiwan and the Main Island of Taiwan (per 1 and 13.1 μg/m3) were not statistically associated with the risk of liver cancer. The reasons for the two conclusions may be related to the different levels of air pollution in different areas or the different susceptibility of people in different areas to air pollution, and genetic factors may also play a role. Therefore, we selected SNPs strongly associated with air pollution as instrumental variables, used two-sample Mendelian randomization to conduct causal analysis on air pollution and primary liver cancer at the genetic level, and verified them separately on European and East Asian populations, so as to improve the reliability and credibility of our research conclusions. Our study demonstrates the lack of statistical causality between air pollution and primary liver cancer, reduces the possibility of their clinical relevance, refutes the role of air pollution in the etiology of primary liver cancer, and complements and updates the methodology of several cohort studies that have reached similar conclusions as our study.
Alpha-fetoprotein (AFP), Osteopontin (OPN) and Glypican-3 (GPC-3) are commonly used as tumor markers for primary liver cancer. AFP, a glycoprotein derived from embryonic endodermal cells, is an important cytokine closely related to the malignant growth of tumors, which can promote the malignant transformation of hepatocytes and the occurrence and development of liver cancer and up to 70% of patients with liver cancer have elevated serum AFP levels (47). AFP has been reported to be 52% sensitive to tumors larger than 3 cm in diameter in hepatocellular carcinoma patients, and is the most widely used tumor marker in clinical practice (48). OPN, a highly modified extracellular matrix protein, is found in 0% of serum in healthy people and increases in hepatitis, cirrhosis and liver cancer patients. OPN even outperforms AFP in distinguishing cirrhosis from liver cancer. When OPN and AFP are combined in the diagnosis of hepatocellular carcinoma, the sensitivity can increase to 65% (6, 49). GPC-3 is a hepatocellular carcinoma related biomarker with a specificity of up to 97%, which can detect hepatocellular carcinoma at an earlier stage than AFP and one study showed that serum GPC-3 levels in 50% of patients with early hepatocellular carcinoma were > 300 ng/L, despite their serum AFP levels < 100 μg/L (50). MR Analysis of air pollution and primary liver cancer related tumor markers AFP, OPN and GPC-3 found no causal association, further confirming our previous conclusion that there was no statistical association between air pollution and primary liver cancer risk.
Arginase-1 (Arg-1), an enzyme that catalyzes the hydrolysis of arginine to ornithine and urea in the urea cycle, is mainly expressed in the cytoplasm of hepatocytes and is not expressed in bile duct epithelial cells, Kupffer cells, or vascular endothelial cells, and thus can be used in the differential diagnosis between hepatocellular carcinoma and other potentially confounding malignancies. Arg-1 is reported to be a highly specific biomarker for hepatocellular differentiation, with sensitivities of 100, 96.2 and 85.7% in highly differentiated, moderately differentiated and poorly differentiated hepatocellular carcinoma, respectively (51, 52). Our results found a significant association between nitrogen oxides and Arginase-1, a biomarker highly associated with hepatocellular differentiation, which remained statistically significant after adjusting for possible confounding factors such as alcohol intake frequency, body mass index (BMI) and cancers (breast cancer, nonmelanoma skin cancer, cutaneous squamous cell carcinoma, and basal cell carcinoma; Beta: 4.46; 95%CI: 0.83–8.08; p = 0.015). Currently, there is a lack of relevant research on the effects of nitrogen oxides on Arg-1, but it has been shown that nitric oxide can affect liver cell differentiation by affecting the tumor microenvironment. On the one hand, nitric oxide can play a role in tumor differentiation, growth progression and metastasis by modulating the expression of multiple inflammatory factors. On the other hand, it can affect and regulate anabolism and catabolism, including sugar, fatty acid and amino acid metabolism, to affect the tumor microenvironment, and which plays a very important role in the hepatocellular differentiation and the conversion of normal cells into tumor cells, and even determines to some extent the direction and type of differentiation of liver cancer (53–56). To some extent, this may explain our results that nitrogen oxides air pollution may affect hepatocellular differentiation by altering the hepatocyte microenvironment, but further in vivo and in vitro experiments are needed to confirm this hypothesis.
There were several limitations to our study. To begin with, although we conducted the MR Analysis on the causal relationship between air pollution and primary liver cancer in both European and East Asian populations, due to the limitation of data, we only analyzed the European population when we analyzed the tumor markers (Alpha-fetoprotein, Osteopontin, Glypican-3 and Arginase-1) of liver cancer, and whether this relationship is also present in other populations needs more verification. Additionally, our results in East Asian populations were based on a 5 × 10−6 significance level, as there were not enough SNPs associated with a 5 × 10−8 genome-wide significance threshold, and this may require an expanded sample size to further validate our conclusions.
In conclusion, our results suggested that there is no causal association between air pollution (including PM2.5, PM2.5–10, PM10, nitrogen dioxide, and nitrogen oxides) and primary liver cancer. However, there was a statistical association between nitrogen oxides and Arg-1, further experimental and mechanistic studies are needed to verify the validity of the findings obtained in this study.
Publicly available datasets were analyzed in this study. This data can be found at: https://gwas.mrcieu.ac.uk/datasets/.
MS and MG: designing, carrying out the study, analyzing the data, and writing the article. ML, TW, and TZ: carrying out the study, and revising. JQ: designing, revising, and financial support. All authors contributed to the article and approved the submitted version.
This work was supported by the National Natural Science Foundation Program of China (82073653 and 81803313), Hunan Outstanding Youth Fund Project (2022JJ10087), National Key Research and Development Project (2018YFE0114500), China Postdoctoral Science Foundation (2020M682644), Hunan Provincial Science and Technology Talent Support Project (2020TJ-N07), Hunan Provincial Key Research and Development Program (2018SK2063), Open Project from NHC Key Laboratory of Birth Defect for Research and Prevention (KF2020006), Natural Science Foundation of Hunan Province (2018JJ2551), Natural Science Foundation of Hunan Province of China (2022JJ40207), and Changsha Municipal Natural Science Foundation (kq2202470).
We thank the IEU Open GWAS 340 project (https://gwas.mrcieu.ac.uk/datasets/) for providing summary results data for the analyses.
The authors declare that the study was conducted in the absence of any commercial or financial relationships that could be construed as a potential conflict of interest.
All claims expressed in this article are solely those of the authors and do not necessarily represent those of their affiliated organizations, or those of the publisher, the editors and the reviewers. Any product that may be evaluated in this article, or claim that may be made by its manufacturer, is not guaranteed or endorsed by the publisher.
The Supplementary material for this article can be found online at: https://www.frontiersin.org/articles/10.3389/fpubh.2023.1212301/full#supplementary-material
PM, particulate matter; NO2, nitrogen dioxide; NOx, nitrogen oxides; IVs, instrumental variables; MR, Mendelian randomization; GWAS, genome-wide association studies; IVW, inverse variance weighted; SNPs, single nucleotide polymorphisms; BMI, body mass index; AFP, alpha-fetoprotein; OPN, osteopontin; GPC-3, Glypican-3; Arg-1, Arginase-1; CI, confidence interval; HR, hazard ratio.
1. Gao, YX , Yang, TW , Yin, JM , Yang, PX , Kou, BX , Chai, MY, et al. Progress and prospects of biomarkers in primary liver cancer (review). Int J Oncol. (2020) 57:54–66. doi: 10.3892/ijo.2020.5035
2. Bray, F , Ferlay, J , Soerjomataram, I , Siegel, RL , Torre, LA , and Jemal, A . Global cancer statistics 2018: GLOBOCAN estimates of incidence and mortality worldwide for 36 cancers in 185 countries. CA Cancer J Clin. (2018) 68:394–424. doi: 10.3322/caac.21492
3. Gera, S , Ettel, M , Acosta-Gonzalez, G , and Xu, R . Clinical features, histology, and histogenesis of combined hepatocellular-cholangiocarcinoma. World J Hepatol. (2017) 9:300–9. doi: 10.4254/wjh.v9.i6.300
4. McGlynn, KA , Petrick, JL , and El-Serag, HB . Epidemiology of hepatocellular carcinoma. Hepatology. (2021) 73:4–13. doi: 10.1002/hep.31288
5. Yamashita, T , Forgues, M , Wang, W , Kim, JW , Ye, Q , Jia, H, et al. EpCAM and alpha-fetoprotein expression defines novel prognostic subtypes of hepatocellular carcinoma. Cancer Res. (2008) 68:1451–61. doi: 10.1158/0008-5472.CAN-07-6013
6. Ying, X , Zhao, Y , Wang, JL , Zhou, X , Zhao, J , He, CC, et al. Serum anti-osteopontin autoantibody as a novel diagnostic and prognostic biomarker in patients with hepatocellular carcinoma. Oncol Rep. (2014) 32:1550–6. doi: 10.3892/or.2014.3367
7. Shirakawa, H , Kuronuma, T , Nishimura, Y , Hasebe, T , Nakano, M , Gotohda, N, et al. Glypican-3 is a useful diagnostic marker for a component of hepatocellular carcinoma in human liver cancer. Int J Oncol. (2009) 34:649–56. doi: 10.3892/ijo_00000190
8. Timek, DT , Shi, J , Liu, H , and Lin, F . Arginase-1, HepPar-1, and Glypican-3 are the most effective panel of markers in distinguishing hepatocellular carcinoma from metastatic tumor on fine-needle aspiration specimens. Am J Clin Pathol. (2012) 138:203–10. doi: 10.1309/AJCPK1ZC9WNHCCMU
9. Pesce, JT , Ramalingam, TR , Mentink-Kane, MM , Wilson, MS , el Kasmi, KC , Smith, AM, et al. Arginase-1-expressing macrophages suppress Th2 cytokine-driven inflammation and fibrosis. PLoS Pathog. (2009) 5:e1000371. doi: 10.1371/journal.ppat.1000371
10. El-Serag, HB . Epidemiology of viral hepatitis and hepatocellular carcinoma. Gastroenterology. (2012) 142:1264–1273.e1. doi: 10.1053/j.gastro.2011.12.061
11. Bradbury, KE , Appleby, PN , and Key, TJ . Fruit, vegetable, and fiber intake in relation to cancer risk: findings from the European prospective investigation into Cancer and nutrition (EPIC). Am J Clin Nutr. (2014) 100:394S–8S. doi: 10.3945/ajcn.113.071357
12. Kim, HB , Shim, JY , Park, B , and Lee, YJ . Long-term exposure to air pollution and the risk of non-lung cancer: a meta-analysis of observational studies. Perspect Public Health. (2020) 140:222–31. doi: 10.1177/1757913919891751
13. Cohen, AJ , Brauer, M , Burnett, R , Anderson, HR , Frostad, J , Estep, K, et al. Estimates and 25-year trends of the global burden of disease attributable to ambient air pollution: an analysis of data from the global burden of diseases study 2015. Lancet. (2017) 389:1907–18. doi: 10.1016/S0140-6736(17)30505-6
14. White, AJ , Keller, JP , Zhao, S , Carroll, R , Kaufman, JD , and Sandler, DP . Air pollution, clustering of particulate matter components, and breast Cancer in the sister study: a U.S.-wide cohort. Environ Health Perspect. (2019) 127:107002. doi: 10.1289/EHP5131
15. Loomis, D , Grosse, Y , Lauby-Secretan, B , Ghissassi, FE , Bouvard, V , Benbrahim-Tallaa, L, et al. The carcinogenicity of outdoor air pollution. Lancet Oncol. (2013) 14:1262–3. doi: 10.1016/S1470-2045(13)70487-X
16. Raaschou-Nielsen, O , Andersen, ZJ , Hvidberg, M , Jensen, SS , Ketzel, M , Sørensen, M, et al. Air pollution from traffic and cancer incidence: a Danish cohort study. Environ Health. (2011) 10:67. doi: 10.1186/1476-069X-10-67
17. Deng, H , Eckel, SP , Liu, L , Lurmann, FW , Cockburn, MG , and Gilliland, FD . Particulate matter air pollution and liver cancer survival. Int J Cancer. (2017) 141:744–9. doi: 10.1002/ijc.30779
18. Coleman, NC , Burnett, RT , Ezzati, M , Marshall, JD , Robinson, AL , and Pope, CA III. Fine particulate matter exposure and Cancer incidence: analysis of SEER Cancer registry data from 1992-2016. Environ Health Perspect. (2020) 128:107004. doi: 10.1289/EHP7246
19. Pan, WC , Wu, CD , Chen, MJ , Huang, YT , Chen, CJ , Su, HJ, et al. Fine particle pollution, alanine transaminase, and liver Cancer: a Taiwanese prospective cohort study (REVEAL-HBV). J Natl Cancer Inst. (2016) 108:djv341. doi: 10.1093/jnci/djv341
20. Pedersen, M , Andersen, ZJ , Stafoggia, M , Weinmayr, G , Galassi, C , Sørensen, M, et al. Ambient air pollution and primary liver cancer incidence in four European cohorts within the ESCAPE project. Environ Res. (2017) 154:226–33. doi: 10.1016/j.envres.2017.01.006
21. So, R , Chen, J , Mehta, AJ , Liu, S , Strak, M , Wolf, K, et al. Long-term exposure to air pollution and liver cancer incidence in six European cohorts. Int J Cancer. (2021) 149:1887–97. doi: 10.1002/ijc.33743
22. VoPham, T , Bertrand, KA , Tamimi, RM , Laden, F , and Hart, JE . Ambient PM(2.5) air pollution exposure and hepatocellular carcinoma incidence in the United States. Cancer Causes Control. (2018) 29:563–72. doi: 10.1007/s10552-018-1036-x
23. Sudlow, C , Gallacher, J , Allen, N , Beral, V , Burton, P , Danesh, J, et al. UK biobank: an open access resource for identifying the causes of a wide range of complex diseases of middle and old age. PLoS Med. (2015) 12:e1001779. doi: 10.1371/journal.pmed.1001779
24. Bycroft, C , Freeman, C , Petkova, D , Band, G , Elliott, LT , Sharp, K, et al. The UK biobank resource with deep phenotyping and genomic data. Nature. (2018) 562:203–9. doi: 10.1038/s41586-018-0579-z
25. Eeftens, M , Beelen, R , de Hoogh, K , Bellander, T , Cesaroni, G , Cirach, M, et al. Development of land use regression models for PM(2.5), PM(2.5) absorbance, PM(10) and PM(coarse) in 20 European study areas; results of the ESCAPE project. Environ Sci Technol. (2012) 46:11195–205. doi: 10.1021/es301948k
26. Sun, BB , Maranville, JC , Peters, JE , Stacey, D , Staley, JR , Blackshaw, J, et al. Genomic atlas of the human plasma proteome. Nature. (2018) 558:73–9. doi: 10.1038/s41586-018-0175-2
27. Gilly, A , Park, YC , Png, G , Barysenka, A , Fischer, I , Bjørnland, T, et al. Whole-genome sequencing analysis of the cardiometabolic proteome. Nat Commun. (2020) 11:6336. doi: 10.1038/s41467-020-20079-2
28. Suhre, K , Arnold, M , Bhagwat, AM , Cotton, RJ , Engelke, R , Raffler, J, et al. Connecting genetic risk to disease end points through the human blood plasma proteome. Nat Commun. (2017) 8:14357. doi: 10.1038/ncomms14357
29. Minton, K . The FinnGen study: disease insights from a 'bottlenecked' population. Nat Rev Genet. (2023) 24:207. doi: 10.1038/s41576-023-00584-y
30. Kurki, MI , Karjalainen, J , Palta, P , Sipilä, TP , Kristiansson, K , Donner, KM, et al. FinnGen provides genetic insights from a well-phenotyped isolated population. Nature. (2023) 613:508–18. doi: 10.1038/s41586-022-05473-8
31. Kubo, M , and Guest, E . BioBank Japan project: epidemiological study. J Epidemiol. (2017) 27:S1. doi: 10.1016/j.je.2016.11.001
32. Burgess, S , Butterworth, A , and Thompson, SG . Mendelian randomization analysis with multiple genetic variants using summarized data. Genet Epidemiol. (2013) 37:658–65. doi: 10.1002/gepi.21758
33. Zou, XL , Wang, S , Wang, LY , Xiao, LX , Yao, TX , Zeng, Y, et al. Childhood obesity and risk of stroke: a Mendelian randomisation analysis. Front Genet. (2021) 12:727475. doi: 10.3389/fgene.2021.727475
34. Zhou, J , Ye, Z , Wei, P , Yi, F , Ouyang, M , Xiong, S, et al. Effect of basal metabolic rate on osteoporosis: a Mendelian randomization study. Front Public Health. (2023) 11:1096519. doi: 10.3389/fpubh.2023.1096519
35. Bowden, J , del Greco, MF , Minelli, C , Davey Smith, G , Sheehan, NA , and Thompson, JR . Assessing the suitability of summary data for two-sample Mendelian randomization analyses using MR-egger regression: the role of the I2 statistic. Int J Epidemiol. (2016) 45:1961–74. doi: 10.1093/ije/dyw220
36. Burgess, S , and Thompson, SG, C.C.G. Collaboration . Avoiding bias from weak instruments in Mendelian randomization studies. Int J Epidemiol. (2011) 40:755–64. doi: 10.1093/ije/dyr036
37. Li, C , Ou, R , and Shang, H . Rheumatoid arthritis decreases risk for Parkinson's disease: a Mendelian randomization study. NPJ Parkinsons Dis. (2021) 7:17. doi: 10.1038/s41531-021-00166-x
38. Bowden, J , del Greco M, F , Minelli, C , Davey Smith, G , Sheehan, N , and Thompson, J . A framework for the investigation of pleiotropy in two-sample summary data Mendelian randomization. Stat Med. (2017) 36:1783–802. doi: 10.1002/sim.7221
39. Wang, Z , Chen, M , Wei, YZ , Zhuo, CG , Xu, HF , Li, WD, et al. The causal relationship between sleep traits and the risk of schizophrenia: a two-sample bidirectional Mendelian randomization study. BMC Psychiatry. (2022) 22:399. doi: 10.1186/s12888-022-03946-8
40. Bowden, J , del Greco M, F , Minelli, C , Zhao, Q , Lawlor, DA , Sheehan, NA, et al. Improving the accuracy of two-sample summary-data Mendelian randomization: moving beyond the NOME assumption. Int J Epidemiol. (2019) 48:728–42. doi: 10.1093/ije/dyy258
41. Verbanck, M , Chen, CY , Neale, B , and do, R . Detection of widespread horizontal pleiotropy in causal relationships inferred from Mendelian randomization between complex traits and diseases. Nat Genet. (2018) 50:693–8. doi: 10.1038/s41588-018-0099-7
42. Hemani, G , Zheng, J , Elsworth, B , Wade, KH , Haberland, V , Baird, D, et al. The MR-base platform supports systematic causal inference across the human phenome. elife. (2018) 7:7. doi: 10.7554/eLife.34408
43. Tjønneland, A , Olsen, A , Boll, K , Stripp, C , Christensen, J , Engholm, G, et al. Study design, exposure variables, and socioeconomic determinants of participation in diet, Cancer and health: a population-based prospective cohort study of 57,053 men and women in Denmark. Scand J Public Health. (2007) 35:432–41. doi: 10.1080/14034940601047986
44. Ulmer, H , Kelleher, CC , Fitz-Simon, N , Diem, G , and Concin, H . Secular trends in cardiovascular risk factors: an age-period cohort analysis of 698,954 health examinations in 181,350 Austrian men and women. J Intern Med. (2007) 261:566–76. doi: 10.1111/j.1365-2796.2007.01779.x
45. Riboli, E , Hunt, KJ , Slimani, N , Ferrari, P , Norat, T , Fahey, M, et al. European prospective investigation into Cancer and nutrition (EPIC): study populations and data collection. Public Health Nutr. (2002) 5:1113–24. doi: 10.1079/PHN2002394
46. Cesaroni, G , Badaloni, C , Porta, D , Forastiere, F , and Perucci, CA . Comparison between various indices of exposure to traffic-related air pollution and their impact on respiratory health in adults. Occup Environ Med. (2008) 65:683–90. doi: 10.1136/oem.2007.037846
47. Zheng, Y , Zhu, M , and Li, M . Effects of alpha-fetoprotein on the occurrence and progression of hepatocellular carcinoma. J Cancer Res Clin Oncol. (2020) 146:2439–46. doi: 10.1007/s00432-020-03331-6
48. Saffroy, R , Pham, P , Reffas, M , Takka, M , Lemoine, A , and Debuire, B . New perspectives and strategy research biomarkers for hepatocellular carcinoma. Clin Chem Lab Med. (2007) 45:1169–79. doi: 10.1515/CCLM.2007.262
49. Liu, K , Duan, J , Liu, H , Yang, X , Yang, J , Wu, M, et al. Precancer antiviral treatment reduces microvascular invasion of early-stage hepatitis B-related hepatocellular carcinoma. Sci Rep. (2019) 9:2220. doi: 10.1038/s41598-019-39440-7
50. Waidely, E , al-Youbi, AO , Bashammakh, AS , el-Shahawi, MS , and Leblanc, RM . Alpha-l-Fucosidase immunoassay for early detection of hepatocellular carcinoma. Anal Chem. (2017) 89:9459–66. doi: 10.1021/acs.analchem.7b02284
51. Morris, SM Jr . Recent advances in arginine metabolism: roles and regulation of the arginases. Br J Pharmacol. (2009) 157:922–30. doi: 10.1111/j.1476-5381.2009.00278.x
52. Ordonez, NG . Arginase-1 is a novel immunohistochemical marker of hepatocellular differentiation. Adv Anat Pathol. (2014) 21:285–90. doi: 10.1097/PAP.0000000000000022
53. Yongsanguanchai, N , Pongrakhananon, V , Mutirangura, A , Rojanasakul, Y , and Chanvorachote, P . Nitric oxide induces cancer stem cell-like phenotypes in human lung cancer cells. Am J Physiol Cell Physiol. (2015) 308:C89–C100. doi: 10.1152/ajpcell.00187.2014
54. Weidenbach, H , Nussler, AK , Shu, Z , Adler, G , and Beckh, K . Nitric oxide formation lowers norepinephrine-induced intrahepatic resistance without major effects on the metabolism in the perfused rat liver. Hepatology. (1997) 26:147–54. doi: 10.1002/hep.510260120
55. Dios-Barbeito, S , González, R , Cadenas, M , García, LF , Victor, VM , Padillo, FJ, et al. Impact of nitric oxide in liver cancer microenvironment. Nitric Oxide. (2022) 128:1–11. doi: 10.1016/j.niox.2022.07.006
Keywords: air pollution, primary liver cancer, particulate matter, nitrogen oxides, biomarkers
Citation: Sun M, Gao M, Luo M, Wang T, Zhong T and Qin J (2023) Association between air pollution and primary liver cancer in European and east Asian populations: a Mendelian randomization study. Front. Public Health. 11:1212301. doi: 10.3389/fpubh.2023.1212301
Received: 26 April 2023; Accepted: 12 July 2023;
Published: 27 July 2023.
Edited by:
Ghulam Mujtaba Kayani, Hubei University of Economics, ChinaReviewed by:
Ying Wu, Southern Medical University, ChinaCopyright © 2023 Sun, Gao, Luo, Wang, Zhong and Qin. This is an open-access article distributed under the terms of the Creative Commons Attribution License (CC BY). The use, distribution or reproduction in other forums is permitted, provided the original author(s) and the copyright owner(s) are credited and that the original publication in this journal is cited, in accordance with accepted academic practice. No use, distribution or reproduction is permitted which does not comply with these terms.
*Correspondence: Jiabi Qin, cWluamlhYmkxMjNAMTYzLmNvbQ==
Disclaimer: All claims expressed in this article are solely those of the authors and do not necessarily represent those of their affiliated organizations, or those of the publisher, the editors and the reviewers. Any product that may be evaluated in this article or claim that may be made by its manufacturer is not guaranteed or endorsed by the publisher.
Research integrity at Frontiers
Learn more about the work of our research integrity team to safeguard the quality of each article we publish.