- 1Medical Psychological Center, The Second Xiangya Hospital, Central South University, Changsha, China
- 2Medical Psychological Institute of Central South University, Central South University, Changsha, China
- 3National Clinical Research Center on Mental Disorders (Xiangya), Changsha, China
Background: Cognitive decline and negative emotions are common in aging, especially decline in cold cognition which often co-occurred with depression in middle-aged and older adults. This study analyzed the interactions between cold cognition and depression in the middle-aged and elder populations using network analysis and explored the effects of grandparenting on the cold cognition-depression network.
Methods: The data of 6,900 individuals (≥ 45 years) from the China Health and Retirement Longitudinal Study (CHARLS) were used. The Minimum Mental State Examination (MMSE) and the Epidemiology Research Center Depression Scale-10 (CESD-10) were used to assess cold cognition and depressive symptoms, respectively. Centrality indices and bridge centrality indices were used to identify central nodes and bridge nodes, respectively.
Results: Network analysis showed that nodes “language ability” and “depressed mood” were more central nodes in the network of cold cognition and depression in all participants. Meantime, nodes “attention,” “language ability” and “hopeless” were three key bridge nodes connecting cold cognition and depressive symptoms. Additionally, the global connectivity of the cold cognition and depression network was stronger in the non-grandparenting than the grandparenting.
Conclusion: The findings shed a light on the complex interactions between cold cognition and depression in the middle-aged and elder populations. Decline in language ability and depressed mood can serve as predictors for the emergence of cold cognitive dysfunction and depression in individuals during aging. Attention, language ability and hopelessness are potential targets for psychosocial interventions. Furthermore, grandparenting is effective in alleviating cold cognitive dysfunction and depression that occur during individual aging.
Introduction
Aging is increasing seriously globally as the decline in birth rate and increases in life expectancy (1). The statistical data from the National Bureau of Statistics of China show that the number of populations aged 60 and older was 253 million in 2019 (2). Moreover, it is estimated that the number of middle-aged and older adults will reach 400 million in China by 2050, which will bring significant challenges in healthcare and socio-economic (3).
Previous studies have demonstrated that cognitive decline and negative emotions are common in aging, especially decline in cold cognition which often co-occurred with depression in middle-aged and older adults (4, 5). Cold cognition refers to the process of information with less emotional influence, such as working memory, attention, executive function and language (6). Studies have demonstrated that cold cognitive dysfunction is a risk factor for emotional disorders including depression (7, 8). Individuals with cold cognition decline are more vulnerable to suffering from depression than health ones (9). Meanwhile, the meta-analysis has also revealed that some cold cognition dysfunctions are present in the first episode and persist after the depression having been remitted (10–12). These findings suggest that cold cognition dysfunction can be used as a good marker for depression susceptibility (13), prognosis (14) and functional recovery (15). Moreover, a previous study reported that the co-occurrence of cold cognition dysfunction with depression has a negative impact on daily life significantly (16). Although it is well documented that cold cognition impairment and depression often co-exist, they only focused on the relationship between cold cognition and depression at the overall level, ignoring the effects of specific cold cognitive functions on different depressive symptoms. Furthermore, the study on the connection between specific cold cognition dysfunctions and depressive symptoms in middle-aged and older adults is rare.
Recently, increasing numbers of research have found that traditional conceptualization studies of psychopathology obscured meaningful associations between symptoms (17). With the development of cybermetrics, Borsboom (18) has proposed the network theory of psychiatric disorders based on the network analysis approach, which suggests that psychiatric disorders are conceptualized as representations of a symptom network. In the network, nodes are symptoms, the connectivity between nodes represents inter-symptomatic relationships, and the dynamic changes in the symptom network triggered by the interaction of symptoms represent the occurrence and development of psychiatric disorders. Network analysis has emerged as a new approach to investigate complex, dynamic relationships between individual psychiatric symptoms (19). In contrast to traditional perspectives, network analysis treats a particular psychiatric disorder as an interacting cluster of symptoms (18, 20), whereas the emergence and development of disorders are thought to be caused by strong causal interactions between symptoms (18, 21). Thus, an important aim of the network analysis approach is to identify the core symptoms (high centrality nodes) and the bridging symptoms that connect the symptom networks between different psychiatric disorders in the symptom network. Furthermore, core and key bridge symptoms can be more likely to activate other symptoms in the network, thereby driving the development of psychiatric disorders (20, 22). Clinically, core symptoms of psychiatric disorders identified by network analysis can be used as a target of interventions, which may be more effective in preventing the occurrence and promoting regression of psychiatric disorder. Recently, several studies have been conducted to explore psychiatric disorders based on network analysis. For example, Briganti et al. (23) proposed network analysis is a useful tool to explore depression symptoms and offers new insight as to how they interact. A symptom network analysis of PTSD found that fear circuitry and dysphoric PTSD symptoms appear to emerge as connected networks as time elapses after trauma, while intrusive memories and reactivity are centrally associated with other symptoms in the acute phase (22). These findings not only indicate that the relationship between psychiatric symptoms is a network of direct interactions, but also reveal that the clinical symptoms network of psychiatric disorders prominently highlights meaningful associations of symptoms within and between disorders (24). However, network analysis of cold cognition dysfunction and depressive symptoms in middle-aged and older adults is still lack.
In addition, previous studies have also shown that the heterogeneity of depressive symptoms and cold cognitive impairment are largely influenced by individual differences. For example, Sneed and Schulz (25) study in adults found a non-linear decline in cold cognition function with age, while older adults with grandparenting had a slower rate of cognition deterioration than the non-grandparenting. Grandparenting refers to grandparents living with their grandchildren assuming some or all of the responsibility for raising and educating their grandchildren (26), and is very common in China. There is evidence to support the benefits of grandparenting on human well-being and mental health. For example, it was found that older adults who lived with grandchildren had lower levels of depression compared to those without (27). Arpino and Bordone (28) study also found that grandparenting positively affects the cognition function in older adults. Collectively, grandparenting may have important effects on cold cognition and emotion in older adults. However, whether grandparenting plays an important role in cognitive function-depressive networks in middle-aged and older adults needs further confirmation.
In this study, network analysis was used to clarify the complex relationship between cold cognition and depression to identify the core symptoms and the mechanisms of co-occurrence between cold cognition and depression in middle-aged and older adults. Furthermore, we also compared cold cognition-depression networks between the grandparenting and non-grandparenting to explore the effect of grandparenting on the cold cognition-depression network. These may help to explain the negative impacts of cold cognition decline on the emotions of the middle-aged and elder populations, even facilitating timely treatments of core symptoms to prevent and alleviate depression for them.
Methods
Participants and procedure
The present study used the China Health and Retirement Longitudinal Survey (CHARLS) in 2018 follow-up data to explore the network structure of cold cognition and depression among middle-aged and older adults in China. The CHARLS is a nationally representative data with a large sample and high data quality regarding basic information, mental health and physical health of Chinese aged 45 years or older.
The inclusion and exclusion criteria for the subjects in this study were (1) having demographic information such as age, gender, whether or not they were raised in intergenerational relationships, and years of education and being older than 45 years old (2); completing the Center for Epidemiologic Studies Depression Scale (CESD-10) and having no missing values; and (3) completing Mini-Mental State Examination (MMSE) assessment with no missing values. There are a total of 19,816 subjects in the 2018 survey. According to the inclusion criteria, 1,107 cases with missing age, gender or age less than 45 years old, and 2,819 cases missing grandparenting status and years of education, 3,109 cases with incomplete CESD scores, and 5,881 cases with incomplete MMSE assessments were excluded, and a final valid sample of 6,900 cases was obtained in present study.
Measures
Cold cognition
The cold cognitive function was calculated by a Minimum Mental State Examination (MMSE) questionnaire in the CHARLS (29). The MMSE scale contains 30 items to cover time and place orientation, immediate memory, attention and calculation, recall, and language ability. The correct answer for each item is recorded as one score. High scores are associated with better cognitive function. While scores below the cut-off values can be considered as an impaired cognition. Specifically, the MMSE cutoff was set at 16/17 for uneducated persons (0 years of education), 19/20 for those with 1–6 years of education, and 23/24 for those with 7 or more years of education (30). The Cronbach’s α coefficient of the MMSE in this study was 0.813.
Depression
Center for Epidemiologic Studies Depression-10 (CESD-10) was used to assess depressive symptoms and levels in middle-aged and elder persons (31, 32). The CESD-10 is a self-reported questionnaire to assess depressive symptoms in the past week on a 4-point scale with a total score range of 0 to 30, more severe depression is indicated by a higher score, i.e., the CESD score ≥ 20 was regarded as depressed, ≥ 10 considered to have depressive symptoms and < 10 means no significant depressive symptoms (33). The scale Cronbach’s α coefficient in this study was 0.809.
Statistical analysis
The data analysis was performed with SPSS and R version 4.1.2.
Network estimation
The network model of 15 indicators was estimated using the EBIC glasso function in the q graph package of network tools R package (34). Specifically, a Gaussian graphical model was first estimated, which estimated the pairwise correlation parameters among all nodes. Meanwhile, the LASSO regularization technique was employed to cautiously identify correlated edges, and accurately identify the underlying network structure (35). Epskamp and Fried (34) study reported a detailed information on the estimation of such regularized biased correlation networks, and how to estimate such models in R.
For the network with 15 nodes, 105 parameters [15 × (15-1)/2] need to be estimated (34). According to at least 3–5 individuals per parameter, the sample size in this study is sufficient for conducting network analysis (N = 6,900). In the network, 15 indicators were depicted as nodes, while the correlations between symptoms were described as edges. The mgm package was used to assess the predictability of each node. A node with a high value of predictability indicated that it is explained by its neighboring nodes (36). Additionally, edges can be positive (green lines) or negative (red lines) and stronger connections were represented by thicker and more saturated edges.
Centrality and bridge centrality estimation
Three indices of centrality were calculated to assess the importance of each node in the network via the centrality Plot function in the graph package (37) (1). The overall importance of a symptom in the network is indicated by the strength centrality (38) (2); The closeness centrality indicated that the impact of one symptom rapidly spreads to other symptoms (39) (3), The betweenness centrality indicated a bridge symptom connecting with other symptoms and potential target symptoms for interventions (21). Given that the stability of closeness and betweenness centrality are usually low (40), the standardized strength centrality (z scores) was mainly reported in this study, and the results of closeness and betweenness centrality were presented in the Supplementary material.
The network tools R package was used to calculate bridge centrality statistics, including bridge strength, bridge betweenness, and bridge closeness. Similarly, only strength bridge centrality was mainly reported and the top 20% scoring nodes were selected as predicted bridge nodes based on the bridge strength values of the network (41).
Network accuracy and stability estimation
The accuracy and stability of the network were calculated using bootstrapping methods in the ‘bootnet’ package (34). First, the 95% confidence intervals (CIs) of edge-weights accuracy were computed via bootstrapping procedures (bootstrapped samples = 1,000). Secondly, the subset program (subsetting bootstrap; i.e., removing a certain percentage of the participants and re-estimating the network) was used to test the stability of the node centrality estimates. The centrality estimate was considered stable if the centrality order of the network constructed after removing many samples was the same as the centrality order of the original network, the centrality order of the original network was the same as that of the original network. The stability of the centrality indices was then summarized using CS-coefficients (correlation stability). The CS-coefficient should be ideally above 0.5 but at least above 0.25 (42).
Network comparison test
The comparison of networks between grandparenting and non-grandparenting was calculated using a permutation-based test with the ‘Network Comparison Test’ package (43), which tests differences between two networks based on global strength, network structure, and edge strength. The thresholds to the results of the two networks comparison were adjusted by applying False Discovery Rate (FDR) correction. In addition, centrality estimates were computed using the “test centrality” command, which statistically assesses the centrality of symptoms across the two networks. The magnitude of the node difference was compared using standardized data (z-scores) and evaluated using the effect size (Cohen’s d). Small, medium and high effects are represented by Cohen’s d values of 0.2, 0.4 and 0.8, respectively (44).
Results
Participant characteristics
The valid sample size was 6,900 including 3,360 grandparenting (48.70%) and 3,540 non-grandparenting (51.30%), 3,309 males (48.00%) and 3,591 females (52.00%), with the mean age of (63.31 ± 7.07) years and (6.64 ± 3.81) years of education. 1,192 participants did not receive education, 3,024 participants received 1–6 years of education and 2,681 participants received 7 or more years of education; 5,214 participants lived with a spouse (75.57%) and 1,686 participants lived without a spouse (24.43%); 1876 participants were urban residence (27.32%) while 4,991 participants were rural residence (72.68%). Meanwhile, 448 participants had no significant depressive symptoms (6.50%, the score of <10 on the CESD-10), 3,524 participants reported the presence of significant depressive symptoms (51.07%, with a score of ≥10 on the CESD-10). Of these, 2,928 participants were depressed (42.43%, with a score of ≥20 on the CESD-10). 968 paticipants with illiterats showed cognitive impairment (81.21%). 2,205 participants (72.92%) with 1–6 years and 2,360 participants (88.03%) with seven or more years of education exhibited cognition decline, see Appendix 1 for details.
Moreover, Table 1 showed the basic characteristics of the grandparenting and non-grandparenting samples. No significant difference in age, education years and gender between the two groups. The middle-aged and older adults in the grandparenting group had significantly higher levels of cold cognitive function and lower levels of depression than those in the non-grandparenting group. Moreover, scores of five cold cognitive functions (orientation, immediate memory, attention and calculation, recall, language ability) of the grandparenting group were all significantly higher than that of the non-grandparenting group. The grandparenting group also scored significantly lower on all depressive symptoms than the non-grandparenting group. The mean scores, standard deviations, predictability and abbreviation for each node of the MMSE and CESD-10 were presented in Table 2.
Network estimation for total samples
Network structure
The network structure of cold cognition and depression in middle-aged and older adults was depicted in Figure 1. The ring-shaped pie charts of the network were used to indicate the predictability of nodes, i.e., the mean predictability was 0.35 (range from 0.05 to 0.72, Table 2). Within the cold cognition community, node “memory” had the most directly positive connection with node “language ability,” followed by the positive connection between nodes “memory” and “recall.” Moreover, “memory,” “recall” and “language ability” were more closely linked to each other in the cold cognitive function cluster. Among the depressive symptom community, node “unhappy” had the most directly positive with node “hopeless,” followed by the positive connection between nodes “lonely” and “cannot continue.” Furthermore, “upset,” “mind distraction,” “depressed mood” and “exhaust” were more closely linked to each other, followed by the degree of the closeness among “fear,” “lonely” and “cannot continue.” Additionally, there were many associations among the items across the communities. For example, node “hopeless” was most strongly negatively associated with node “attention,” followed by connections between nodes “attention,” “exhaust,” and “cannot continue.” Simultaneously, not all nodes in the two sub-networks of cold cognition and depression were directly connected, e.g., nodes “lonely” and “depressed mood” were not significantly directly connected to the cluster of cold cognition nodes.
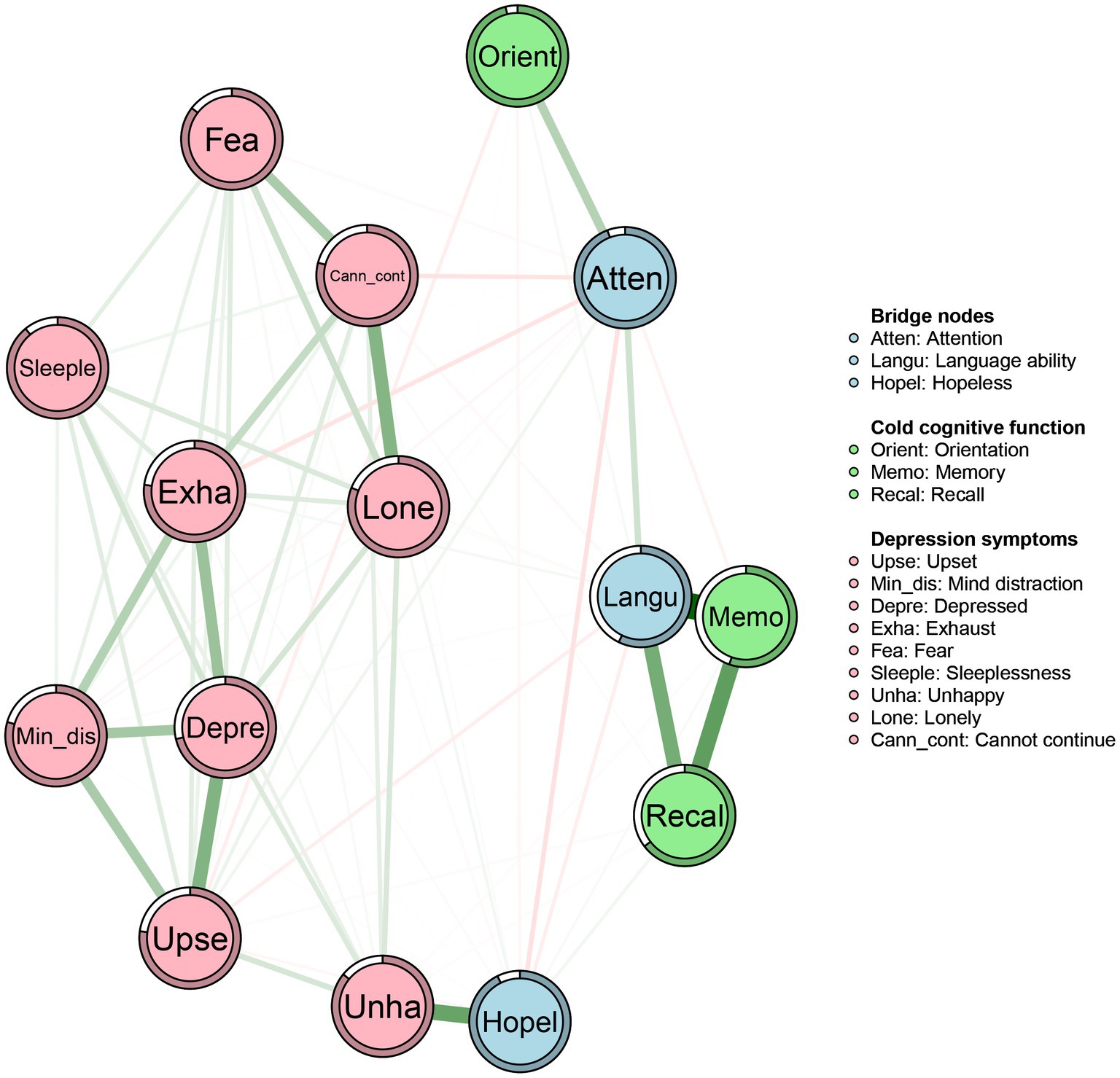
Figure 1. Networks of cold cognition and depressive symptoms in middle-aged and elder population. Nodes represent cold cognition and depressive symptoms and edges represent partial correlations between symptoms. Edge thickness and darkness indicate the association strength (minimum and maximum edge values were set to be equal across networks), and edge color indicates the correlation valence (green = positive; red = negative). Bridge nodes indicated the connection of cold cognition and depressive symptoms. Orient, Orientation; Memo, Memory; Atten, Attention; Recal, Recall; Langu, Language ability; Upse, Upset; Min_dis, Mind distraction; Depre, Depressed mood; Exha, Exhaust; Hopel, Hopeless; Fea, Fear; Sleeple, Sleeplessness; Unha, Unhappy; Lone, Lonely; Cann_cont, Cannot continue.
Centrality and bridge centrality
The centrality and bridge strength of each node among middle-aged and older adults were presented in Figure 2 (results of other centrality indicators and the estimation of node strength difference are in Appendix S2). Node “language ability” had the highest strength. Nodes “depressed mood,” “memory,” and “upset” were also statistically stronger than most other nodes in the network. The bridge strength in nodes “attention,” “language ability” and “hopeless” were stronger than most other nodes.
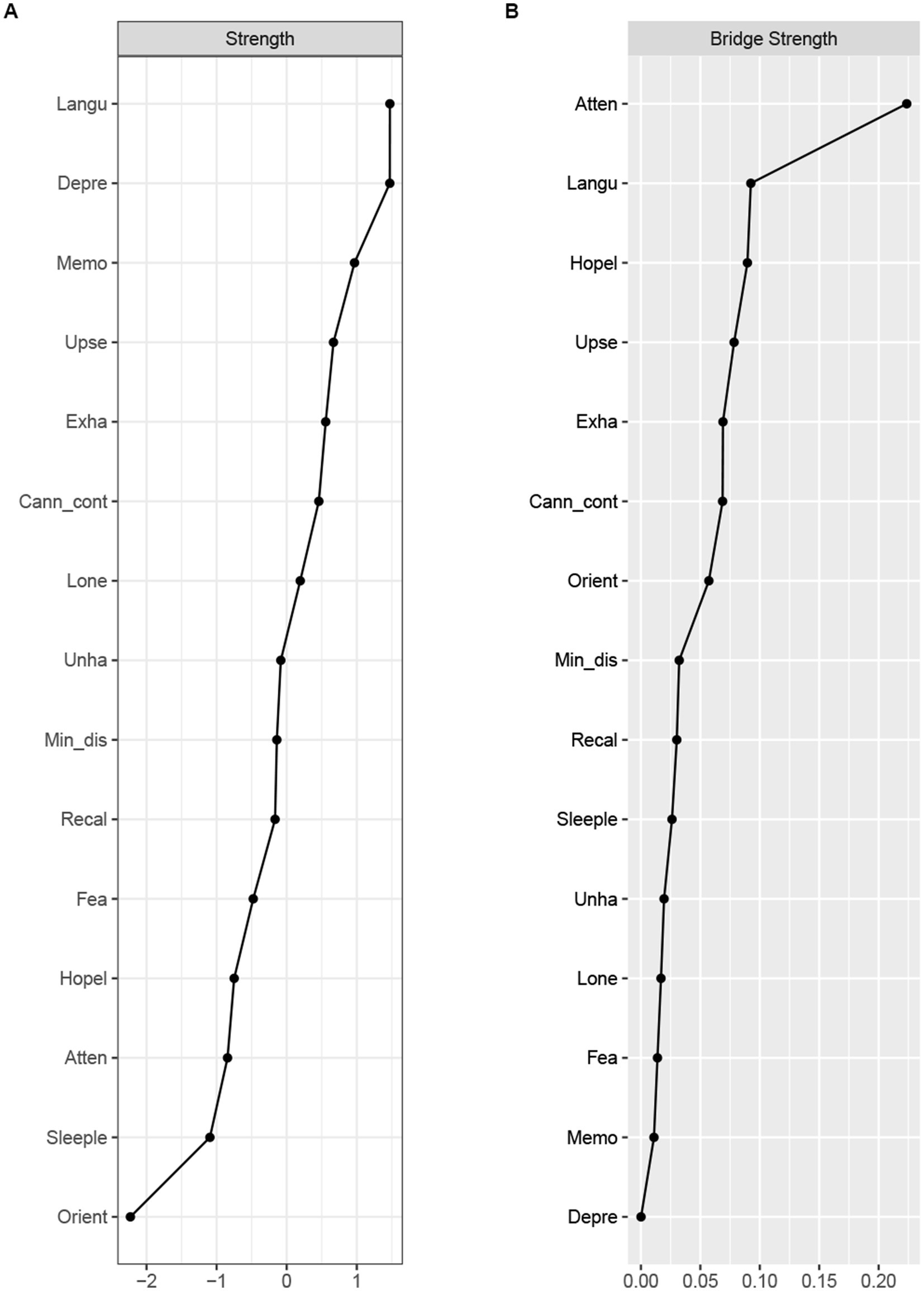
Figure 2. Node strength (A) and bridge strength (B) centrality estimates for the cold cognition and depressive symptoms network in middle-aged and elder population. Standardized z-scores are plotted for ease of interpretation. Higher scores represent higher centrality estimates (i.e., the symptom has greater influence in the network). Orient, Orientation; Memo, Memory; Atten, Attention; Recal, Recall; Langu, Language ability; Upse, Upset; Min_dis, Mind distraction; Depre, Depressed mood; Exha, Exhaust; Hopel, Hopeless; Fea, Fear; Sleeple, Sleeplessness; Unha, Unhappy; Lone, Lonely; Cann_cont, Cannot continue.
Network accuracy and stability
The edge stability estimation showed moderate stability of the estimated networks: although there were considerable overlaps among the 95% CIs of edge weights, nonoverlapped CIs also existed (Appendix S3) and estimation of edge weight difference indicated edges of higher stability were significantly different with other edges in the network (Appendix S4). Meanwhile, stability estimates of the centrality index showed that the centrality strength stability coefficient (CS-coefficients) was 0.750 (Appendix S5).
Cold cognition-depression networks in grandparenting and non-grandparenting
Network structure
The network structure of cold cognition and depression among middle-aged and older adults with and without grandparenting was depicted in Figure 3. Although the overall structure is similar, there were significant differences in the pattern of nodes’ connections and the strength of the edges between the two networks. Additionally, the ring-shaped pie charts of the grandparenting and non-grandparenting networks were used to indicate the predictability of nodes, i.e., the mean predictability was 0.32 (range from 0.03 to 0.75) and 0.36 (range from 0.08 to 0.69), respectively (Table 2).
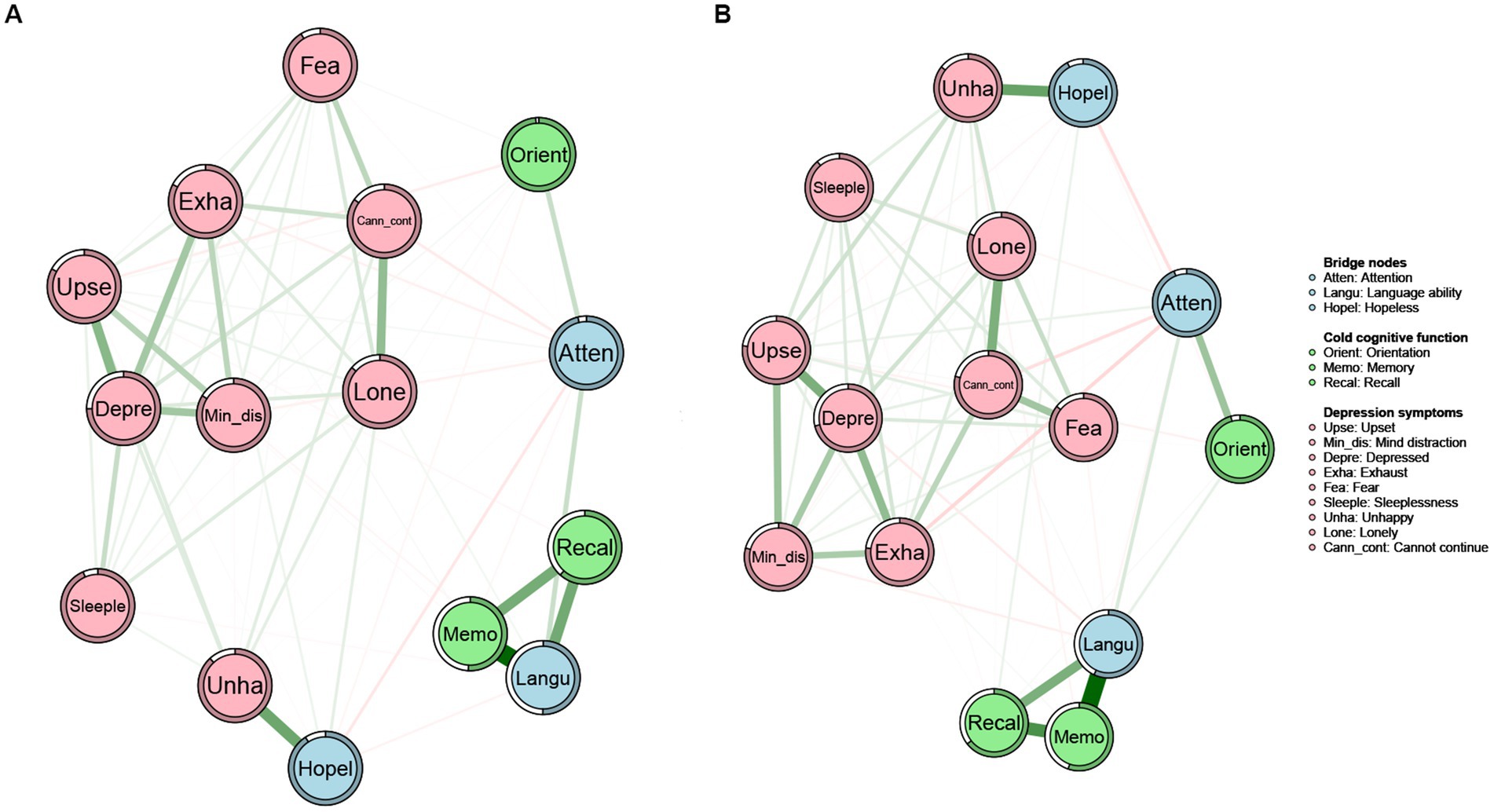
Figure 3. Networks of cold cognition and depressive symptoms in the grandparenting (A) and non-grandparenting (B). Nodes represent cold cognition and depressive symptoms and edges represent partial correlations between symptoms. Edge thickness and darkness indicate the association strength (minimum and maximum edge values were set to be equal across networks), and edge color indicates the correlation valence (green = positive; red = negative). Bridge nodes indicated the connection of cold cognition and depressive symptoms. Orient, Orientation; Memo, Memory; Atten, Attention; Recal, Recall; Langu, Language ability; Upse, Upset; Min_dis, Mind distraction; Depre, Depressed mood; Exha, Exhaust; Hopel, Hopeless; Fea, Fear; Sleeple, Sleeplessness; Unha, Unhappy; Lone, Lonely; Cann_cont, Cannot continue.
Centrality and bridge centrality
The centrality and bridge strength of each node in grandparenting and non-grandparenting networks were shown in Figure 4 (results of other centrality indicators and the estimation of node strength difference were presented in Appendix S6). Node “language ability” had the highest strength, while nodes “depressed mood,” “memory,” and “upset” were also statistically stronger than most other nodes in the grandparenting network. In the non-grandparenting network, the strength of node “depressed mood” was highest, followed by nodes “language ability,” “memory,” and “upset.” In addition, nodes “attention,” “hopeless” and “language ability” were stronger than most other nodes for the bridge strength of the two networks, which is consistent with the bridge nodes of the cold cognition and depression network in the total sample.
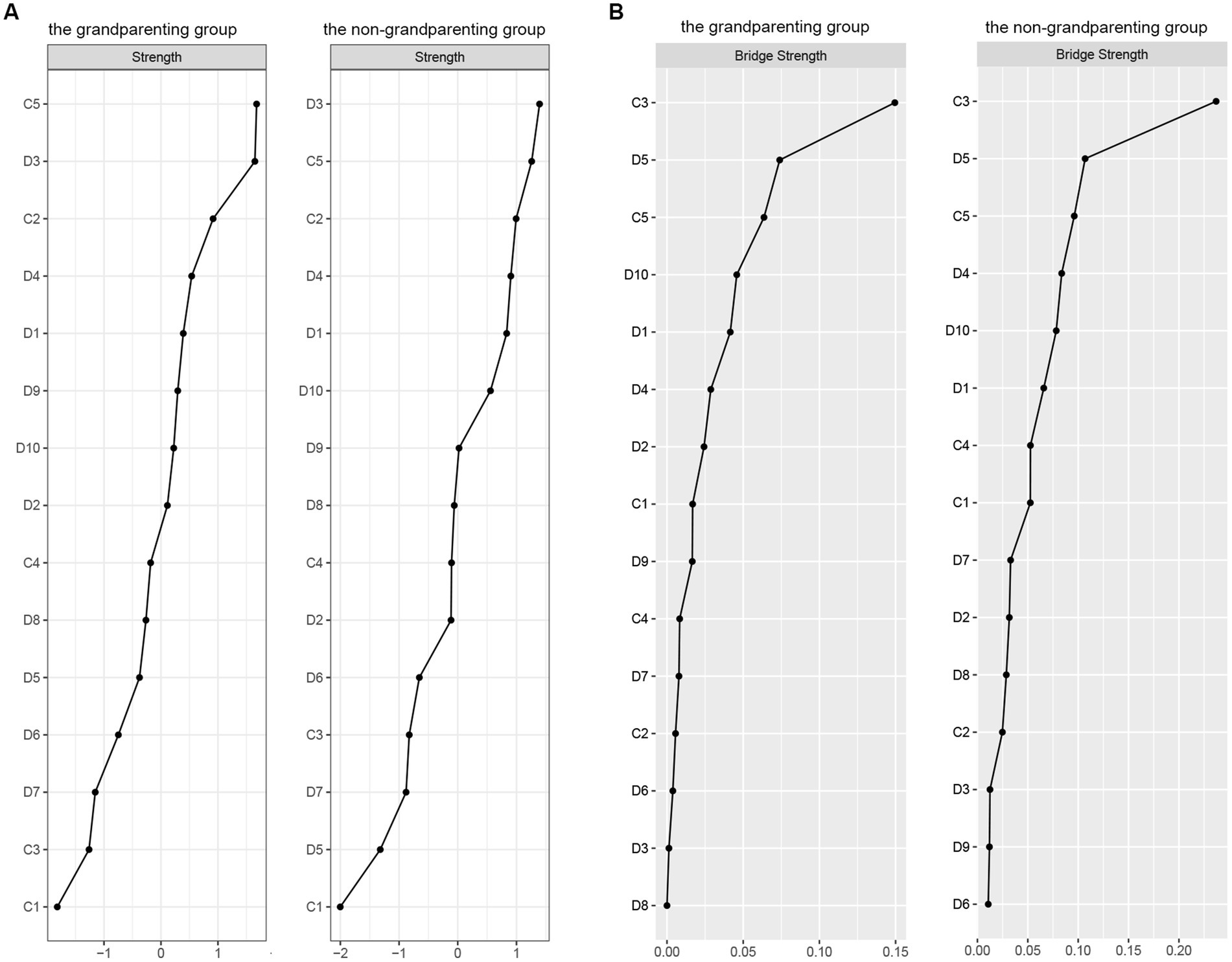
Figure 4. Node strength (A) and bridge strength (B) centrality estimates for the cold cognition and depressive symptoms network in the grandparenting and non-grandparenting. Standardized z-scores are plotted for ease of interpretation. Higher scores represent higher centrality estimates (i.e., the symptom has greater influence in the network). Orient, Orientation; Memo, Memory; Atten, Attention; Recal, Recall; Langu, Language ability; Upse, Upset; Min_dis, Mind distraction; Depre, Depressed mood; Exha, Exhaust; Hopel, Hopeless; Fea, Fear; Sleeple, Sleeplessness; Unha, Unhappy; Lone, Lonely; Cann_cont, Cannot continue.
Network accuracy and stability
The edge stability estimation showed moderate stability of the grandparenting and non-grandparenting networks (Appendix S7) and the estimation of edge weight difference showed that edges of higher stability of these two networks were significantly different from other edges (Appendix S8). Meanwhile, stability estimates of the centrality index showed that the centrality strength stability coefficients (CS-coefficients) were 0.75 in the two networks (Appendix S9).
Network comparison
The results of the network comparison showed that the grandparenting and non-grandparenting networks differed significantly in overall structure (M = 0.09, p = 0.02) and connectivity (S = 0.61, p < 0.01). The non-grandparenting network had higher global connectivity than the grandparenting network (6.03 vs. 5.41). The comparison of mean node strength centrality was presented in Table 3. The nodes in the non-grandparenting network had significantly higher centrality indices than nodes in other networks on “orientation,” “attention,” “recall,” “upset,” “exhaust,” “sleeplessness,” “unhappy,” “lonely” and “cannot continue” with Cohen’s d values above 0.8 (Table 3).
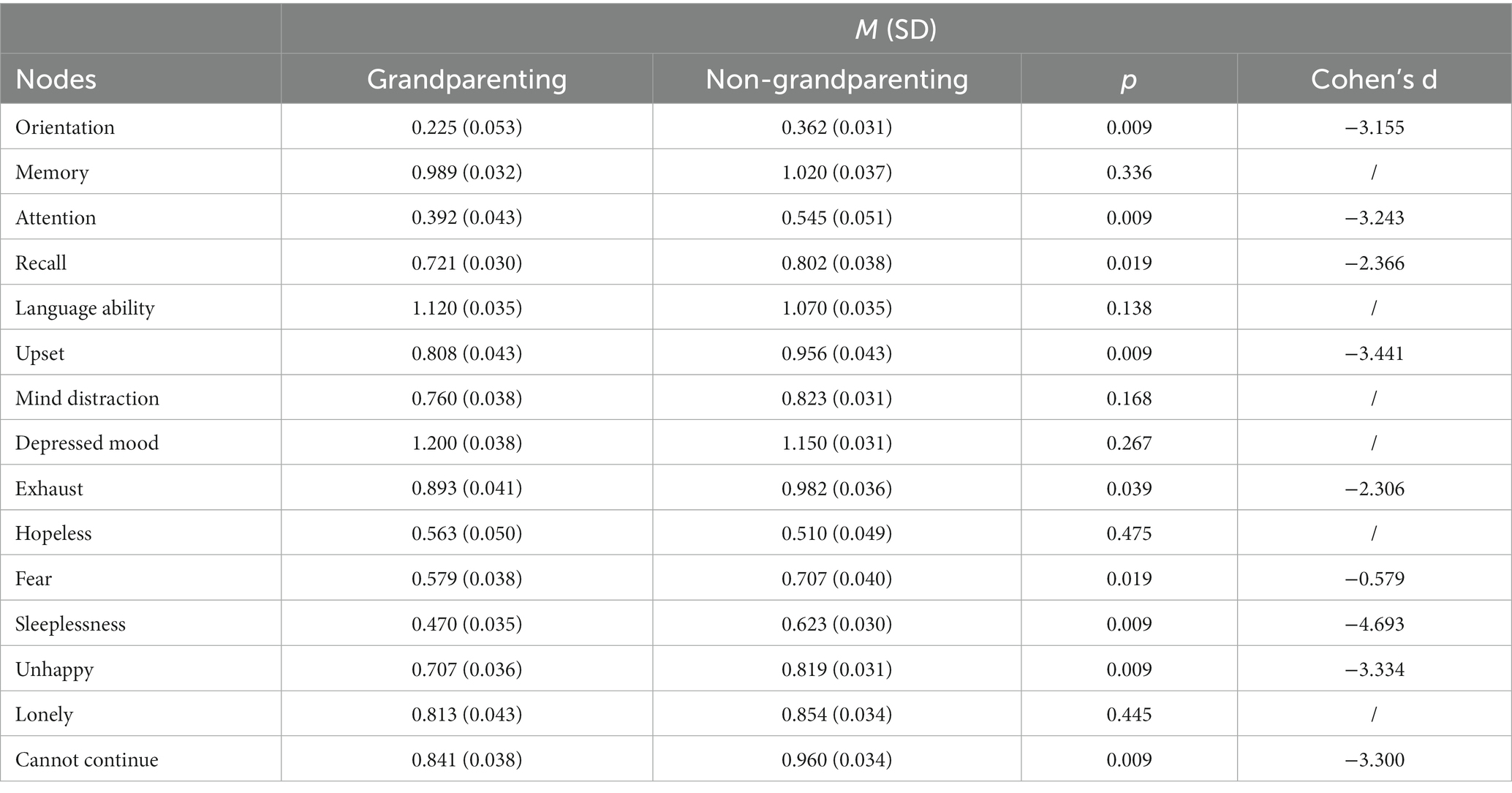
Table 3. Comparison of mean node strength centrality between the grandparenting and non-grandparenting.
Discussion
The present study used network analysis to construct the network of cold cognition and depression in middle-aged and elder adults. The key findings are that (1): the cold cognitive cluster and depressive symptoms cluster are relatively independent in the middle-aged and elder adults (2); language ability and depressed mood are the most central nodes in the cold cognitive cluster and depressive symptoms cluster, respectively, as well as in the cold cognition-depression network; while attention, language ability, and hopeless are the top-three key bridge nodes connecting cold cognition and depressive symptoms in the network (3); There are significant effects of grandparenting on the global structure and connectivity of the network, specifically, the connectivity of the cold cognition-depression network is significantly stronger in the non-grandparenting than that in the grandparenting; the non-grandparenting network nodes have higher centrality indices on orientation, attention, recall, upset, exhaust, sleeplessness, unhappy and lonely.
Brain organs gradually degenerate with aging, which leads to the decline of cognitive function, especially cold cognitive functions such as attention, working memory, and language ability. In this study, the language ability, assessed by the MMSE, is a comprehensive cold cognitive ability. Depressed mood, as a core symptom of depression (45), covers a series of negative emotional experiences, such as sadness and low mood (46). Due to the gradual decline of physical functions (including brain functions) and faded social roles, middle-aged and elder persons are prone to develop a depressed mood, even leading to depressive episodes (47). In this study, language ability is the core node in both the cold cognitive clusters and the cold cognition-depression network, while the cold cognitive ability shows an overall decline in middle-aged and elder population. Consistent with previous reports (36, 48), the depressed mood is another core symptom in both the depressive symptom cluster and cold cognition-depression network in middle-aged and older adults. In consideration of the vital roles of the highest centrality nodes in a network (48, 49), the language ability decline and depressed mood are the most likely candidates for triggering and maintaining the decline in cold cognition and depressive symptoms. According to the network theory of mental disorder (18), targeting central symptoms with biological or psychosocial interventions, rather than peripheral symptoms, can be highly effective. In present study, interventions for the two core symptoms (depressed mood and language skills) would alleviate depressed mood and slow down the decline of language skills in middle-aged and older adults, as well as facilitate the improvement of peripheral symptoms, such as decreased attention and hopelessness, which in turn would have a greater impact on the cold cognitive-depression network (20). However, we also found the lack of a significantly direct connection between depressed mood and cold cognition node clusters, which not only supports the hot and cold cognition model (50), but also hints that reducing core symptoms, such as depressed mood or enhancing language ability, alone only improves the internal symptom network of depression or cold cognition, respectively.
According to the network theory of psychiatric disorders (18), finding and targeting key bridge-connecting symptoms (nodes) is needed to treat with cold cognitive-depressive co-morbidities in middle-aged and older adults. In the present study, attention, language ability and hopelessness are the top-three key bridge nodes connecting cold cognition to depressive symptoms in the network, suggesting that bridge nodes contribute to comorbidity of cold cognitive dysfunction and depression. In clinically, targeting the three bridge nodes of attention, language skills, and hopelessness for interventions may be effective for both cold cognitive dysfunction and depressive symptoms (51). The hopelessness theory of depression (52) proposes that adverse life events are prone to induce despair, helplessness, and uselessness in individuals, resulting in depressed mood and thoughts. In this study, hopelessness is a stronger symptom linking cold cognition and depressive symptoms. In middle-aged and elder individuals, cold cognitions (such as working memory and attention) are markedly declined, and their social roles are gradually becoming less important until retirement, which may be a series of adverse stressors and lead to senses of uselessness and hopelessness, and in turn hopelessness trigger the most core symptoms of depression - depressed mood and eventually develop into depressive disorder. Thus, hopelessness in middle-aged and older adults is not only a symptom of depression but may also be a risk factor for developing into depressive disorder. The findings of this study combined with previous studies may have utility in tailoring and testing interventions to reduce the co-occurrence of cold cognitive dysfunctions and depression in the mild-aged and elder population. For example, it is recommended to maintain healthy lifestyle to slow down physiological aging (including cold cognition), while communication with good friends and family members is thought to alleviate negative mood symptoms (53).
In China, middle-aged and older adults often regard parenting their grandchildren as a responsibility and obligation. Previous studies suggested that intergenerational parenting may be a protective factor in cognitive impairment and depression (27, 28). This study also showed that the global connectivity of the cold cognition-depression network in the non-grandparenting is stronger than that in the grandparenting. Also, grandparenting failed to change the effect of cold cognition on depressive symptoms but had a moderating effect on the strength of the connection in the cold cognition – depressive symptom network. In addition, non-grandparenting group is more strongly connected to this network, which suggested that it is at higher risk for depression as cold cognitive functioning declines in mild-aged and elder adults without grandparenting.
Borsboom et al. (20) believed that high centrality symptoms increasingly activate other symptoms in the network and improve the development of psychiatric disorders. In this study, three-fifths of centrality nodes in the cold cognitive-depressive symptom network were much higher in the non-grandparenting group than in the grandparenting group, suggesting a protective effect of grandparenting on comorbidity of cold cognitive dysfunction and depression. Additionally, non-emotional cold cognitive impairment contributes to the development of hot cognition, and subsequently lead to psychiatric symptoms and further worsening cold cognitive impairment (12). Specifically, cold cognition decline such as attention and recall are prone to activate other emotional and somatic symptoms in the network among middle-aged and older adults without grandparenting, which would promote the development of co-occurrence in cold cognitive disorder and depression.
Finally, the present study also has some limitations: (1) although the effect of cold cognition on depression has received increasing attention from clinical studies, the role of hot cognition on depression should not be neglected. (2) The present study explored the relationship between cold cognition and depressive symptoms from a perspective network analysis, which has some clinical implications for improving cold cognition impairment and depression in the middle-aged and older adults. However, future studies should analyze their dynamic relationships based on longitudinal data to guide clinical practice with more robust and rigorous findings; (3) although the MMSE is a broad measure of cognitive ability and cold cognition is a complex ability, more precise and comprehensive neurofunctional tests are needed in the future to assess cold cognition and depressive network structure in middle-aged and older adults; (4) excepting for grandparenting, future research also needs to study the effects of other factors, such as socioeconomic status, physical and mental health status, on the association between cold cognitive and depressive symptoms in middle-aged and older adults.
In conclusion, there are complex interactions between cold cognition and depression in middle-aged and older adults. Grandparenting has effect on the cold cognitive-depression networks. Systemic multi-level interventions targeting central nodes and bridge nodes may be effective in alleviating the co-occurrence of cold cognitive cline and depressive symptoms in the mild-aged and older adult population.
Data availability statement
The original contributions presented in the study are included in the article/Supplementary material, further inquiries can be directed to the corresponding author.
Ethics statement
Ethical review and approval was not required for the study on human participants in accordance with the local legislation and institutional requirements. Written informed consent for participation was not required for this study in accordance with the national legislation and the institutional requirements.
Author contributions
YZ and DY: conception and design. DY, JW, and SL: provision of study materials. DY, JW, RZ, and XZ: collection and assembly of data. DY and YZ: data analysis, interpretation, and manuscript writing. All authors contributed to the article and approved the submitted version.
Funding
This work was supported by Hunan Provincial Natural Science Foundation China (No. 2022JJ70146), the Hunan Provincial Innovation Foundation for Postgraduate (No. CX20230299) and the Fundamental Research Funds for the Central Universities of Central South University (No. 2023ZZTS0224).
Conflict of interest
The authors declare that the research was conducted in the absence of any commercial or financial relationships that could be construed as a potential conflict of interest.
Publisher’s note
All claims expressed in this article are solely those of the authors and do not necessarily represent those of their affiliated organizations, or those of the publisher, the editors and the reviewers. Any product that may be evaluated in this article, or claim that may be made by its manufacturer, is not guaranteed or endorsed by the publisher.
Supplementary material
The Supplementary material for this article can be found online at: https://www.frontiersin.org/articles/10.3389/fpubh.2023.1204977/full#supplementary-material
References
1. Naja, S, Din Makhlouf, MME, and Chehab, MAH. An ageing world of the 21st century: a literature review. Int J Community Med Public Health. (2017) 4:4363. doi: 10.18203/2394-6040.ijcmph20175306
2. Jing, Z, Wang, Y, Ding, L, Tang, X, Feng, Y, and Zhou, C. Effect of social integration on the establishment of health records among elderly migrants in China: a nationwide cross-sectional study. BMJ Open. (2019) 9:e034255. doi: 10.1136/bmjopen-2019-034255
3. Fang, EF, Scheibye-Knudsen, M, Jahn, HJ, Li, J, Ling, L, Guo, H, et al. A research agenda for aging in China in the 21st century. Ageing Res Rev. (2015) 24:197–205. doi: 10.1016/j.arr.2015.08.003
4. Mac Aulay, RK, Calamia, MR, Cohen, AS, Daigle, K, Foil, H, Brouillette, R, et al. Understanding heterogeneity in older adults: latent growth curve modeling of cognitive functioning. J Clin Exp Neuropsychol. (2018) 40:292–302. doi: 10.1080/13803395.2017.1342772
5. Sun, Z, Wang, Z, Xu, L, Lv, X, Li, Q, Wang, H, et al. Characteristics of cognitive deficit in amnestic mild cognitive impairment with subthreshold depression. J Geriatr Psychiatry Neurol. (2019) 32:344–53. doi: 10.1177/0891988719865943
6. Roiser, JP, and Sahakian, BJ. Hot and cold cognition in depression. CNS Spectr. (2013) 18:139–49. doi: 10.1017/S1092852913000072
7. Stern, Y. The concept of cognitive reserve: a catalyst for research. J Clin Exp Neuropsychol. (2003) 25:589–93. doi: 10.1076/jcen.25.5.589.14571
8. Hugo, J, and Ganguli, M. Dementia and cognitive impairment: epidemiology, diagnosis, and treatment. Clin Geriatr Med. (2014) 30:421–42. doi: 10.1016/j.cger.2014.04.001
9. Mirza, SS, Ikram, MA, Bos, D, Mihaescu, R, Hofman, A, and Tiemeier, H. Mild cognitive impairment and risk of depression and anxiety: a population-based study. Alzheimers Dement. (2017) 13:130–9. doi: 10.1016/j.jalz.2016.06.2361
10. Bora, E, Harrison, BJ, Yücel, M, and Pantelis, C. Cognitive impairment in euthymic major depressive disorder: a meta-analysis. Psychol Med. (2013) 43:2017–26. doi: 10.1017/S0033291712002085
11. Rock, PL, Roiser, JP, Riedel, WJ, and Blackwell, AD. Cognitive impairment in depression: a systematic review and meta-analysis. Psychol Med. (2014) 44:2029–40. doi: 10.1017/S0033291713002535
12. Ahern, E, and Semkovska, M. Cognitive functioning in the first-episode of major depressive disorder: a systematic review and meta-analysis. Neuropsychology. (2017) 31:52–72. doi: 10.1037/neu0000319
13. Allott, K, Fisher, CA, Amminger, GP, Goodall, J, and Hetrick, S. Characterizing neurocognitive impairment in young people with major depression: state, trait, or scar? Brain Behav. (2016) 6:e00527. doi: 10.1002/brb3.527
14. Groves, SJ, Douglas, KM, and Porter, RJ. A systematic review of cognitive predictors of treatment outcome in major depression. Front Psych. (2018) 9:382. doi: 10.3389/fpsyt.2018.00382
15. Bortolato, B, Miskowiak, KW, Köhler, CA, Maes, M, Fernandes, BS, Berk, M, et al. Cognitive remission: a novel objective for the treatment of major depression? BMC Med. (2016) 14:9. doi: 10.1186/s12916-016-0560-3
16. Buist-Bouwman, MA, Ormel, J, de Graaf, R, de Jonge, P, van Sonderen, E, Alonso, J, et al. ESEMeD/MHEDEA 2000 investigators. Mediators of the association between depression and role functioning. Acta Psychiatr Scand. (2008) 118:451–8. doi: 10.1111/j.1600-0447.2008.01285.x
17. Fried, EI, and Nesse, RM. The impact of individual depressive symptoms on impairment of psychosocial functioning. PLoS One. (2014) 9:e90311. doi: 10.1371/journal.pone.0090311
18. Borsboom, D. A network theory of mental disorders. World Psychiatry. (2017) 16:5–13. doi: 10.1002/wps.20375
19. Fisher, ML, Loukola, A, Kaprio, J, and Turner, JR. Role of the Neuregulin signaling pathway in nicotine dependence and co-morbid disorders. Int Rev Neurobiol. (2015) 124:113–31. doi: 10.1016/bs.irn.2015.07.006
20. Borsboom, D, and Cramer, AOJ. Network analysis: an integrative approach to the structure of psychopathology. Annu Rev Clin Psychol. (2013) 9:91–121. doi: 10.1146/annurev-clinpsy-050212-185608
21. McNally, RJ. Can network analysis transform psychopathology? Behav Res Ther. (2016) 86:95–104. doi: 10.1016/j.brat.2016.06.006
22. Bryant, RA, Creamer, M, O’Donnell, M, Forbes, D, McFarlane, AC, Silove, D, et al. Acute and chronic posttraumatic stress symptoms in the emergence of posttraumatic stress disorder: a network analysis. JAMA Psychiat. (2017) 74:135. doi: 10.1001/jamapsychiatry.2016.3470
23. Briganti, G, Scutari, M, and Linkowski, P. Network structures of symptoms from the Zung depression scale. Psychol Rep. (2021) 124:1897–911. doi: 10.1177/0033294120942116
24. Epskamp, S, Kruis, J, and Marsman, M. Estimating psychopathological networks: be careful what you wish for. PLoS One. (2017) 12:e0179891. doi: 10.1371/journal.pone.0179891
25. Sneed, RS, and Schulz, R. Grandparent caregiving, race, and cognitive functioning in a population-based sample of older adults. J Aging Health. (2019) 31:415–38. doi: 10.1177/0898264317733362
26. Muller, Z, and Litwin, H. Grandparenting and psychological well-being: how important is grandparent role centrality? Eur J Ageing. (2011) 8:109–18. doi: 10.1007/s10433-011-0185-5
27. Tsai, F-J. The maintaining and improving effect of grandchild care provision on elders’ mental health-evidence from longitudinal study in Taiwan. Arch Gerontol Geriatr. (2016) 64:59–65. doi: 10.1016/j.archger.2016.01.009
28. Arpino, B, and Bordone, V. Does Grandparenting pay off? The effect of child care on grandparents’ cognitive functioning. J Marriage Fam. (2014) 76:337–51. doi: 10.1111/jomf.12096
29. Folstein, MF, Folstein, SE, and McHugh, PR. “Mini-mental state”. A practical method for grading the cognitive state of patients for the clinician. J Psychiatr Res. (1975) 12:189–98. doi: 10.1016/0022-3956(75)90026-6
30. Li, H, Jia, J, and Yang, Z. Mini-mental state examination in elderly Chinese: a population-based normative study. J Alzheimers Dis. (2016) 53:487–96. doi: 10.3233/JAD-160119
31. Cheng, HG, Chen, S, McBride, O, and Phillips, MR. Prospective relationship of depressive symptoms, drinking, and tobacco smoking among middle-aged and elderly community-dwelling adults: results from the China health and retirement longitudinal study (CHARLS). J Affect Disord. (2016) 195:136–43. doi: 10.1016/j.jad.2016.02.023
32. Boey, KW. Cross-validation of a short form of the CES-D in Chinese elderly. Int J Geriatr Psychiatry. (1999) 14:608–17. doi: 10.1002/(SICI)1099-1166(199908)14:8<608::AID-GPS991>3.0.CO;2-Z
33. Andresen, EM, Malmgren, JA, Carter, WB, and Patrick, DL. Screening for depression in well older adults: evaluation of a short form of the CES-D (Center for Epidemiologic Studies Depression Scale). Am J Prev Med. (1994) 10:77–84. doi: 10.1016/S0749-3797(18)30622-6
34. Epskamp, S, Borsboom, D, and Fried, EI. Estimating psychological networks and their accuracy: a tutorial paper. Behav Res Methods. (2018) 50:195–212. doi: 10.3758/s13428-017-0862-1
35. van Borkulo, CD, Borsboom, D, Epskamp, S, Blanken, TF, Boschloo, L, Schoevers, RA, et al. A new method for constructing networks from binary data. Sci Rep. (2014) 4:5918. doi: 10.1038/srep05918
36. Liu, R, Chen, X, Qi, H, Feng, Y, Su, Z, Cheung, T, et al. Network analysis of depressive and anxiety symptoms in adolescents during and after the COVID-19 outbreak peak. J Affect Disord. (2022) 301:463–71. doi: 10.1016/j.jad.2021.12.137
37. Opsahl, T, Agneessens, F, and Skvoretz, J. Node centrality in weighted networks: generalizing degree and shortest paths. Soc Netw. (2010) 32:245–51. doi: 10.1016/j.socnet.2010.03.006
38. McNally, RJ, Robinaugh, DJ, Wu, GWY, Wang, L, Deserno, MK, and Borsboom, D. Mental disorders as causal systems: a network approach to posttraumatic stress disorder. Clin Psychol Sci. (2015) 3:836–49. doi: 10.1177/2167702614553230
39. Borgatti, SP, Mehra, A, Brass, DJ, and Labianca, G. Network analysis in the social sciences. Science. (2009) 323:892–5. doi: 10.1126/science.1165821
40. Birkeland, MS, Greene, T, and Spiller, TR. The network approach to posttraumatic stress disorder: a systematic review. Eur J Psychotraumatol. (2020) 11:1700614. doi: 10.1080/20008198.2019.1700614
41. Jones, PJ, Ma, R, and McNally, RJ. Bridge centrality: a network approach to understanding comorbidity. Multivar Behav Res. (2021) 56:353–67. doi: 10.1080/00273171.2019.1614898
42. Armour, C, Fried, EI, Deserno, MK, Tsai, J, and Pietrzak, RH. A network analysis of DSM-5 posttraumatic stress disorder symptoms and correlates in U.S. military veterans. J Anxiety Disord. (2017) 45:49–59. doi: 10.1016/j.janxdis.2016.11.008
43. van Borkulo, CD, van Bork, R, Boschloo, L, Kossakowski, JJ, Tio, P, Schoevers, RA, et al. Comparing network structures on three aspects: a permutation test. Psychol Methods. (2022) 2022:476. doi: 10.1037/met0000476
44. Cohen, J. Statistical power analysis. Curr Dir Psychol Sci. (1992) 1:98–101. doi: 10.1111/1467-8721.ep10768783
45. Beard, C, Millner, AJ, Forgeard, MJC, Fried, EI, Hsu, KJ, Treadway, MT, et al. Network analysis of depression and anxiety symptom relationships in a psychiatric sample. Psychol Med. (2016) 46:3359–69. doi: 10.1017/S0033291716002300
46. Danhauer, SC, Legault, C, Bandos, H, Kidwell, K, Costantino, J, Vaughan, L, et al. Positive and negative affect, depression, and cognitive processes in the cognition in the study of tamoxifen and Raloxifene (co-STAR) trial. Neuropsychol Dev Cogn B Aging Neuropsychol Cogn. (2013) 20:532–52. doi: 10.1080/13825585.2012.747671
47. Alexopoulos, GS. Depression in the elderly. Lancet Lond Engl. (2005) 365:1961–70. doi: 10.1016/S0140-6736(05)66665-2
48. Hayes, AM, Yasinski, C, Ben Barnes, J, and Bockting, CLH. Network destabilization and transition in depression: new methods for studying the dynamics of therapeutic change. Clin Psychol Rev. (2015) 41:27–39. doi: 10.1016/j.cpr.2015.06.007
49. Levinson, CA, Zerwas, S, Calebs, B, Forbush, K, Kordy, H, Watson, H, et al. The core symptoms of bulimia nervosa, anxiety, and depression: a network analysis. J Abnorm Psychol. (2017) 126:340–54. doi: 10.1037/abn0000254
50. Stange, JP, Jenkins, LM, Hamlat, EJ, Bessette, KL, DelDonno, SR, Kling, LR, et al. Disrupted engagement of networks supporting hot and cold cognition in remitted major depressive disorder. J Affect Disord. (2018) 227:183–91. doi: 10.1016/j.jad.2017.10.018
51. Kaiser, T, Herzog, P, Voderholzer, U, and Brakemeier, E-L. Unraveling the comorbidity of depression and anxiety in a large inpatient sample: network analysis to examine bridge symptoms. Depress Anxiety. (2021) 38:307–17. doi: 10.1002/da.23136
52. Abramson, LY, Metalsky, GI, and Alloy, LB. Hopelessness depression: a theory-based subtype of depression. Psychol Rev. (1989) 96:358–72. doi: 10.1037/0033-295X.96.2.358
Keywords: network analysis, cold cognition, depression, grandparenting, the middle-aged and elder population
Citation: Yuan D, Wu J, Li S, Zhang R, Zhou X and Zhang Y (2023) Network analysis of cold cognition and depression in middle-aged and elder population: the moderation of grandparenting. Front. Public Health. 11:1204977. doi: 10.3389/fpubh.2023.1204977
Edited by:
Miriam Rodriguez-Monforte, Ramon Llull University, SpainReviewed by:
Taolin Chen, Sichuan University, ChinaMax Jacob Owens, University of South Florida St. Petersburg, United States
Copyright © 2023 Yuan, Wu, Li, Zhang, Zhou and Zhang. This is an open-access article distributed under the terms of the Creative Commons Attribution License (CC BY). The use, distribution or reproduction in other forums is permitted, provided the original author(s) and the copyright owner(s) are credited and that the original publication in this journal is cited, in accordance with accepted academic practice. No use, distribution or reproduction is permitted which does not comply with these terms.
*Correspondence: Yi Zhang, emhhbmd5aXBzeUBjc3UuZWR1LmNu