- 1Department of Nursing, Qilu Hospital, Cheeloo College of Medicine, Shandong University, Jinan, China
- 2Nursing Theory and Practice Innovation Research Center, Cheeloo College of Medicine, Shandong University, Jinan, Shandong, China
- 3Department of Neonatology, Shandong Provincial Hospital, Jinan, China
- 4School of Nursing and Rehabilitation, Cheeloo College of Medicine, Shandong University, Jinan, China
Objective: Premenstrual syndrome (PMS) stands as a significant concern within the realm gynecological disorders, profoundly impacting women of childbearing age in China. However, the elusive nature of its risk factors necessitates investigation. This study, therefore, is dedicated to unraveling the intricacies of PMS by focusing on nurses, a cohort with unique occupational stressors, to develop and validate a predictive model for assessing the risk of PMS.
Methods: This investigation employed a multi-center cross-sectional analysis drawing upon data from the TARGET Nurses’ health cohort. Utilizing online survey versions of the Premenstrual Syndrome Scale (PMSS), a comprehensive dataset encompassing physiological, social, psychological, occupational, and behavioral variables was collected from 18,645 participants. A stepwise multivariate logistic regression analysis was conducted to identify independent risk factors for PMS. Furthermore, a refined variable selection process was executed, combining the Least Absolute Shrinkage and Selection Operator (LASSO) method with 10-fold cross-validation. The visualization of the risk prediction model was achieved through a nomogram, and its performance was evaluated using the C index, receiver operating characteristic (ROC) curves, and the calibration curves.
Results: Among the diverse variables explored, this study identified several noteworthy predictors of PMS in nurses, including tea or coffee consumption, sleep quality, menstrual cycle regularity, intermenstrual bleeding episodes, dysmenorrhea severity, experiences of workplace bullying, trait coping style, anxiety, depression and perceived stress levels. The prediction model exhibited robust discriminatory power, with an area under the curve of 0.765 for the training set and 0.769 for the test set. Furthermore, the calibration curve underscored the model’s high degree of alignment with observed outcomes.
Conclusion: The developed model showcases exceptional accuracy in identifying nurses at risk of PMS. This early alert system holds potential to significantly enhance nurses’ well-being and underscore the importance of professional support.
1. Introduction
Premenstrual syndrome (PMS) constitutes a constellation of emotional, behavioral, and physical symptoms that typically emerge during the luteal phase of the female menstrual cycle, manifesting 7–14 days before menstruation and subsequently abating. These symptoms encompass emotional manifestations such as irritability, anxiety, and depression, behavioral symptoms like sleep disorders and reduced attention, as well as physical complaints like headaches and diarrhea (1). Traditionally acknowledged as a concern among women of childbearing age (2), PMS is fast becoming a common mental disease, substantially impairing the ability of quite a few women to daily function. Meanwhile, increased incidence of some symptoms on PMS in the context of the COVID-19 pandemic (3). An examination of gynecological disease burden data in China underscores PMS as one of the top three gynecological diseases affecting women aged 15 to 49 (4, 5).
A comprehensive review of literature spanning the past five years reveals that PMS affects 25 to 70% of women across all age groups (2, 6–8). This is a matter of profound concern, given the substantial impact of PMS on both physical and mental well-being, as well as its disruptive influence on daily life and work (9). Moreover, PMS significantly increases suicidal ideation in patients, which is a significant risk factor for depression, postpartum depression, frequent work absenteeism and reduced work productivity (10–12). Therefore, the evaluation and effective management of PMS constitute crucial clinical imperatives, given its far-reaching implications.
Remarkably, prior investigations into the prevalence and associated factors of PMS have primarily focused on adult students (3, 13–15), with relatively limited attention directed toward specific occupational groups, including nurses. Clinical nurses, as the custodians of patient well-being, play pivotal roles in healthcare delivery. Owing to the demanding nature of their work and the associated pressures, nurses may be particularly susceptible to PMS, which can reduce their quality of life (16). It is important to note that PMS may be influenced by a variety of factors, encompassing personal characteristics, lifestyle habits, occupational attributes, physiological variables, psychological variables and social determinants. Several studies have linked factors such as underweight, obesity (17, 18), smoking (19), and consumption of coffee and tea (20) to an increased risk of PMS. Additionally, factors inherent to nursing professions, including shift work and sleep patterns, have been identified as risk factors for PMS (21, 22). Physiological variables, such as the intensity of menstrual pain, irregular menstrual cycles, early menarche, prolonged menstruation, and the use of excessive menstrual pads, have also shown significant associations with PMS (15). Other factors impacting PMS include physical activity (23), stress levels (18), personality traits (24), burnout and social support (25). However, PMS often goes unnoticed due to its absence of a clear developmental process. Given the multitude of related risk factors, accurate prediction and early intervention are effective strategies for reducing the incidence of PMS.
In summary, there exists a notable gap in research pertaining to the development of predictive models for assessing PMS risk in nurses, and lack of risk assessment tools for early individualized assessment by nurses themselves. Our study endeavors to fill this void by establishing a nomogram capable of predicting the risk factors for PMS among nurses. Which can early recognize of PMS and could mostly benefit nurses with targeted risk factors. This tool seeks to enable the early recognition of PMS and offer targeted support to nurses with identifiable risk factors. Through an exhaustive analysis and integration of risk factors that exert influence over the onset, development, and prognosis of PMS, our research aims to empower head nurses and nurses to identify PMS early and prompt them to actively seek professional assistance.
2. Materials and methods
2.1. Study population
The TARGET Nurses’ health cohort study (26), launched in December 2020, is a prospective cohort study that focuses on nurses in China. For our study, we harnessed data from the first survey, which recruited participants aged 18–65 with nurse qualification certificates. Participants across Shandong Province were recruited from 46 public hospitals of different grades. In total, 32,222 participants agreed to take part in the study until November 2022. Among these participants, 22,924 were dedicated nurses who diligently completed the comprehensive questionnaire. This nurse cohort was composed of 22,083 females and 211 males. To refine our study cohort further, we meticulously applied exclusion criteria to the subset of 20,798 women aged between 20 and 50 years. Exclusion criteria are set below: (a) nurses who had not experienced menstruation for the past six months due to breastfeeding, pregnancy, menopause, etc. (n = 1,170); (b) those with a prior diagnosis of polycystic ovarian syndrome, or severe mental illness, or those who had used psychopharmacological medicines within six months preceding the completion of the women’s questionnaire (n = 119). Additionally, nurses whose questionnaires exhibited missing data values or outliers across all variables were also excluded from the final analytical dataset (n = 864) (Figure 1).
All participants provided their informed consent prior to their involvement in the study. The study protocol secured approval from the Ethics Committee and was duly registered in the clinical trial registry.
2.2. Measures
The dataset employed in this study encompassed four distinct sections, comprising one dependent variable and 28 independent variables. These variables included the following categories: general information of participants (age, highest educational level, monthly income, marital status, and body mass index), physiology factors (menarcheal age, length of the menstrual cycle, regularity of the menstrual cycle, menstrual period, menstrual blood flow, number of intermenstrual bleedings, the degree of dysmenorrhea, and number of pregnancies), occupational and behavioral factors (nap frequency, smoking, drinking, milk, tea or coffee, physical activity, sleep quality, shifts, and work experience), psychological and social factors, were measured as described below (trait coping style, experiences of workplace bullying, social support, stress, anxiety, and depression).
2.2.1. General information of participants
This study collected data encompassing key demographic attributes of participants, including age, highest educational level achieved, monthly income, marital status, and body mass index, which were classified as underweight (< 18.5 kg/m2), normal weight (18.5–23.9 kg/m2), and overweight (24–27.9 kg/m2), obesity (> 27.9 g/m2), were collected from each participant.
2.2.2. Premenstrual syndrome scale
To assess the severity of PMS symptoms, we harnessed the widely employed Premenstrual Symptoms Scale (PMSS), which is renowned for its simplicity, expeditiousness, and effectiveness. In this study, we adopted the Chinese version (27) of PMSS (28) to measure the extent of PMS symptoms experienced by participants. The scale has 12 items, encompassing psychological symptoms (irritability, depression, anxiety, inattention, nervousness, fidgeting, neuroticism) and physical symptoms (bloating and diarrhea, sleepiness, migraine, insomnia, swelling of hands and feet). Participants were required to indicate whether these symptoms manifested within 14 days before the last menstrual period. Each symptom was graded on a scale of 0–3, with no symptoms (0); mild symptoms (1); deemed tolerable while affecting daily life and work (2), and symptoms seriously impeding daily life and work, necessitating treatment (3). The scale is calculated as the sum of the scores of each item (range 0–36 points). In our study, individuals scoring above 5 were categorized as symptomatic, whereas those scoring below 5 were considered asymptomatic. The Cronbach’s alpha coefficient for the scale was 0.930 in this study.
2.2.3. Physiology factors
Participants were prompted to provide information pertaining to menstrual characteristics and any alterations observed in the past six months, including menarcheal age (< 12 years, 12–14 years, 15–17 years, > 17 years), length of the menstrual cycle (< 25 days, 26–31 days, 32–39 days, > 39 days), regularity of the menstrual cycle, defined by the number of days between two menstrual cycles (within 1–2 days, within 3–4 days, within 5–7 days, more than 7 days, irregular); menstrual period (3–7 days, < 3 days, > 7 days, irregular), menstrual blood flow, quantified by the number of sanitary napkins used during each menstrual period (less than 1 pack of sanitary napkins, 1 or 2 packs of sanitary napkins, more than 2 packs of sanitary napkins), number of intermenstrual bleedings (< 1 time, 1–3 times, > 3 times) and the degree of dysmenorrhea (none, mild, moderate, severe). Additionally, reproductive factors included the number of pregnancies, categorized as 0, 1, 2, and ≥ 3 times.
2.2.4. Occupational and behavioral factors
In the realm of occupational considerations, our study delved into the nurses’ work patterns and their cumulative work experience. To further delineate their behavioral attributes, we employed the following inquiries: (a) How many naps do you take each week? (b) How often do you smoke or drink? And (c) How often do you consume milk, tea or coffee?
2.2.4.1. International physical activity questionnaire
For the assessment of physical activity levels, we harnessed the International Physical Activity Questionnaire (IPAQ). Originating from the World Health Organization in 1998 (29), this instrument has been adeptly adapted into the Chinese context by Qu Ningning in 2004 and has found widespread utility (30). The IPAQ prompts participants to recall their physical activities over the preceding week, including 4 types of physical activities: occupational, household, transportation, and leisure, with 24 entries. For each item denoting a specific physical activity, participants meticulously recorded the duration of engagement. Finally, the types and durations of activities were converted into metabolic equivalents (MET) to measure physical activity levels, classified as low, medium, or high.
2.2.4.2. The Pittsburgh sleep quality index
The Pittsburgh Sleep Quality Index (PSQI) scale was provided to all participants to assess their sleep quality (31). We used the Chinese scale to evaluate nurses’ sleep quality and time. The scale has been used widely and has good reliability and validity (32). The scale consists of 18 self-evaluation items and five other-evaluation items. The latter part is not scored. The former part is composed of seven dimensions, including sleep quality, sleep time, sleep efficiency, sleep disorder, hypnotic drugs and daytime dysfunction factors. The total score ranges from 0 to 21. The higher the score, the worse the sleep quality. In this study, a PSQI score of <6 was indicative of favorable sleep quality, and a score of≥6 indicated a deterioration in sleep quality. The Cronbach’s alpha coefficient for the scale was 0.798 in this study.
2.2.5. Psychological and social factors
2.2.5.1. Negative acts questionnaire
The Negative Acts Questionnaire-Revised (NAQ-R) (33) was used to measure the workplace bullying directed toward nurses by their leaders and colleagues. The Chinese version of the NAQ-R, widely adopted (34), encompasses three dimensions featuring 23 items, including nine items for personal-related negative behaviors, nine items for work-related negative behaviors, and four items for organizational injustice. The initial 22 items were scored on a 5-point Likert scale, ranging from 1 (never) to 5 (every day), with the 23rd item prompting participants to self-assess the level of workplace bullying that they had experienced. Based on the total score, exposure to bullying was classified into three categories: no bullying (< 33), frequent bullying (33–45), and exposure to severe bullying exposure (> 45). The Cronbach’s alpha coefficient for the scale was 0.978 in this study.
2.2.5.2. Perceived social support scale
The assessment of social support levels drew upon the Perceived Social Support Scale (PSSS) (35). The Chinese version (36) of the PSSS used in this study has been proven reliable. It has 12 items and is divided into three dimensions: support from family, friends, and others, providing insights into support both within and beyond the family context. Responses for each item range from 1 to 7, with higher scores denoting elevated levels of social support. Categorized into three tiers, the scale delineates low support (12–36 points), intermediate support (37–60 points), and relatively high support (61–84 points). Widely employed in China, the Cronbach’s alpha coefficient for the scale was 0.989 in this study.
2.2.5.3. Trait coping style questionnaire
To delve into personality traits and their reflection in coping strategies, we deployed the Trait Coping Style Questionnaire (TCSQ) (37). The questionnaire consists of 20 items, partitioned into two dimensions: positive coping and negative coping, each of which contains 10 items. Employing a 5-point Likert scoring method (1–5), the TCSQ ascertains respondents’ tendencies toward positive or negative attitudes and behavioral characteristics when confronting stressors. Higher scores within each dimension correspond to more pronounced positive or negative coping strategies. The Cronbach’s alpha coefficient for the scale was 0.907 in this study.
2.2.5.4. Perceived stress scale
To gauge the level of perceived stress among participants in their daily lives over the past month, we employed the Perceived Stress Scale (PSS), grounded in psychological stress theory and developed in 1983 (38). Introduced into China in 2003 (39), this scale boasts wide utilization and robust reliability and validity. The scale consists of 10 items on a five-point scale of 0–4, representing “never,” “occasionally,” “sometimes,” “often,” and “always” respectively. The total score ranges from 0 to 40. In this study, the level of perceived stress was classified as low stress (<14), medium stress (14–26), and high stress (>26). The Cronbach’s alpha coefficient for the scale in this study was 0.934.
2.2.5.5. Anxiety severity
The Generalized Anxiety Disorder 7-Item Scale (GAD-7) (40) developed by Spitzer et al. has been widely used to screen for anxiety and assess its severity. The reliability and validity of the Chinese version of the GAD-7 have been established by He et al. who found the test–retest reliability to be 0.830 and a Cronbach’s α of 0.920 indicating good reliability and validity (41). The total score ranges from 0 to 21, with each of the seven items scoring from zero (not at all) to three (almost every day), so that scores of 0–4, 5–9, 10–14, and 15–21 represent normal, mild, moderate, and severe anxiety, respectively. The Cronbach’s α of the GAD-7 was 0.963 in this study.
2.2.5.6. Depressed severity
The 9-item Patient Health Questionnaire (PHQ-9) was developed by Kroenke et al. and has been widely used to assess depressive symptoms (42). The PHQ-9 was translated into Chinese by Wang et al., has demonstrated sound psychometric properties, boasting a test–retest reliability of 0.860 and a Cronbach’s alpha of 0.860 (43). The total score ranges from 0 to 27 points, with each of the nine items scoring from zero (not at all) to three (nearly every day), so scores of 0–4, 5–9, 10–14, 15–19, and 20–27 represent normal, mild, moderate, moderately severe, and severe depression, respectively. The Cronbach’s α of the PHQ-9 was 0.955 in this study.
2.3. Data collection and quality control
The data for this study were collected from the baseline data of TARGET, accessible through electronic questionnaires made available on the official TARGET WeChat platform (26). These questionnaires have been validated in Chinese. All participants granted electronic informed consent via the WeChat platform, affirming their independent and anonymous completion of the questionnaire. This informed consent emphasized the voluntary nature of participation, affirming the unequivocal right of individuals to opt out if they so choose.
To ensure the quality and validity of the data, we adopted a comprehensive approach to quality control. A second reminder was given to all non-participants via WeChat one week after the initial questionnaire distribution, with a subsequent reminder issued after an equivalent interval. The electronic questionnaire was pre-set to enforce the completion of mandatory questions and integrate skip logic for certain items. This careful questionnaire formatting ensured the standardization and completeness of responses. Additionally, participants were allocated a specific response time window, ranging from 15 to 20 min, further enhancing data quality. Liaison officers at each participating hospital supervised the completion of the questionnaires, facilitating communication with researchers in cases where issues required resolution beyond routine channels.
2.4. Statistical analysis
All analyses were conducted using R software version 4.0.3 (R Development Core Team, Vienna, Austria). This study included one dependent variable and 28 independent variables in this study, all of which classified variables, expressed as frequencies and percentages. The characteristic distribution between nurses with PMS and non-PMS was evaluated by chi-square test or Fisher’s exact classification variable test. The analytical course followed this trajectory: First, the Least Absolute Shrinkage and Selection Operator (LASSO) method was used to sift through the variables of interest, pinpointing pivotal variables by the glmnet and caret packages. Subsequently, a multivariate logistic regression analysis was embarked upon for the selected variables, streamlining the variables and culminating in the construction of the risk prediction model. The visual representation of this model materialized through a nomogram, skillfully generated via the rms and regplot packages, with the principal aim of providing a readily interpretable snapshot of the predictive model. Decision curve analysis and ROC curves determined sensitivity and specificity, while the discrimination and calibration of the model were subject to scrutiny via ROC and calibration curves, fashioned with the assistance of the pROC and ggplot2 packages. The comprehensive validation spanned internal and external aspects, leveraging the power of 1,000 Bootstrap self-sampling iterations. Statistical significance hinged on a two-tailed p value threshold of less than 0.05.
3. Results
3.1. Sample and demographic characteristics
A total of 18,645 participants were included in this study for analysis (Figure 1). Among these participants, 8,216 were nurses diagnosed with PMS, while 10,429 were without PMS. The participants had a mean age of 33.50 years with a standard deviation of 5.89 years and 10.65 ± 6.40 for working years. The average BMI was 22.30 ± 3.11 kg/m2, and about 76.6% of the participants were married. Table 1 summarizes the clinical characteristics, encompassing physiological, occupational and behavioral, psychological, and social factors for the participants with PMS and non-PMS. In addition, for model validation, the data were divided into a training set and a test set in a 7: 3 ratio. Table 2 outlines the clinical characteristics of the participants in both sets. There were no significant differences among these characteristics, indicating the absence of bias arising from random grouping.
3.2. Variable selection
We used LASSO regression to select predictive factors associated with PMS. This method brings the advantage of variable selection, adjusts complexity while fitting generalized linear models, and effectively handles collinear data—a pivotal consideration given the substantial number of predictive factors in our study. LASSO regression for internal cross-validation pinpointed 10 variables, as shown in Figure 2, These variables included tea or coffee, sleep quality, regularity of the menstrual cycle, number of bleeding between menstruation, the degree of dysmenorrhea, level of bullying, trait coping style, anxiety, depression and perceived stress.
3.3. Construction of predictive models
The 10 variables selected underwent a multivariate logistic regression analysis with backward stepwise regression. The results underscored the significance of tea or coffee, sleep quality, regularity of the menstrual cycle, number of bleeding between menstruation, the degree of dysmenorrhea, level of bullying, trait coping style, anxiety levels, depression and perceived stress in relation to the occurrence of PMS among nurses (Table 3). Subsequently, a nomogram was constructed based on the results of a multivariate logistic regression model (Figure 3). The nomogram offered an intuitive representation of risk factors for PMS in nurses and facilitated predictions regarding PMS risk. Notably, the severity of dysmenorrhea was assigned the highest risk score, totaling 100 points. To calculate an individual’s risk score, each unique PMS risk factor was traced upwards to the corresponding row on the scale, and the scores for all 10 factors were summated, yielding a total score. Higher total scores indicated an elevated risk of PMS for nurses.
3.4. Validation of predictive models
ROC curves were used to evaluate the accuracy of the prediction models. The results revealed that the area under the ROC curve was 0.765 (95% CI, 0.757 ~ 0.773) for the training set and 0.769 (95% CI, 0.756 ~ 0.781) for the test set, respectively (Figures 4A,B). Correspondingly, the C-index was 0.765 and 0.769 for the training and test sets, respectively. The above results affirmed the robust discriminative ability and moderate accuracy of the prediction model. Next, we used calibration curves to evaluate the deviation between the predicted results of the nomogram and the actual values. The prediction results showed a good agreement between the training and test sets (Figures 5A,B). The calibration curves closely paralleled the ideal predictions, yielding mean absolute errors of 0.006 and 0.003 after 1,000 bootstrap repetitions. These outcomes not only establish the model’s dependable calibration but also underscore its reliability.
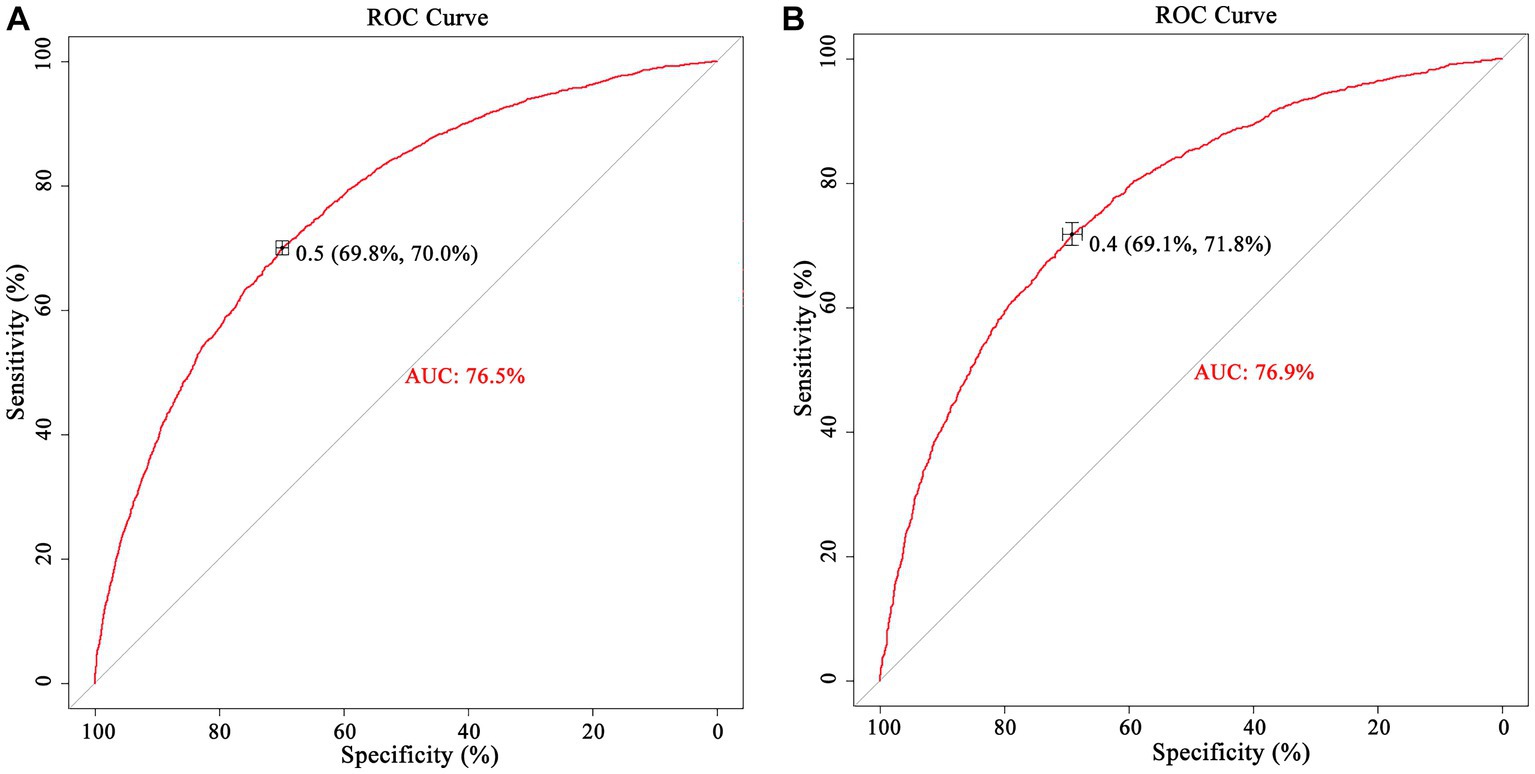
Figure 4. The nomogram model predicts the receiver operating characteristic curve of the PMS in nurse. [(A) training set and (B) test set].
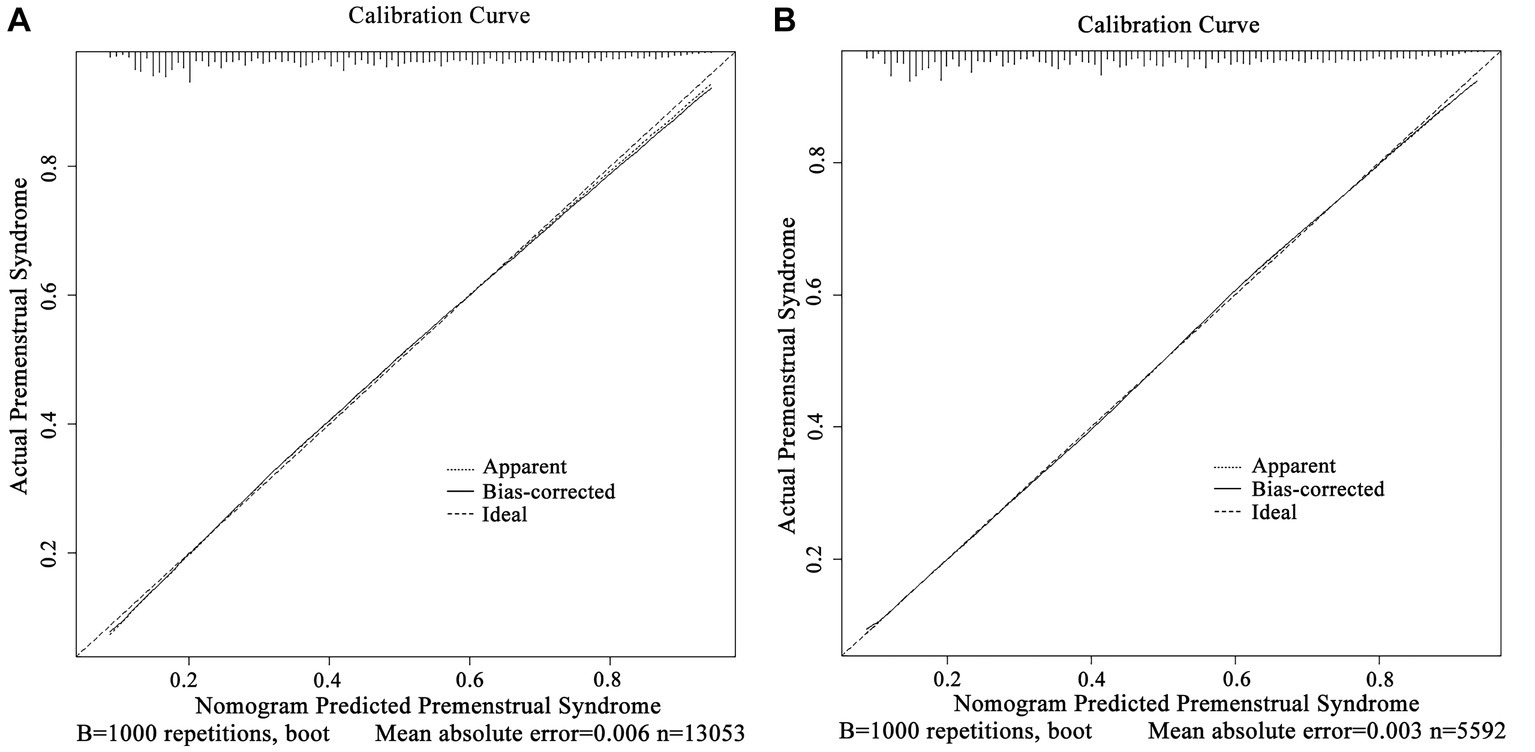
Figure 5. Calibration curve of the nomogram model for predicting PMS in nurses. [(A) training set and (B) test set].
4. Discussion
In this study, a risk prediction model for the PMS among nurses was constructed by multivariate logistic regression, and the nomogram was plotted to visualize the results. The model was validated by ROC curves and calibration curves, revealing close alignment between predicted and observed values and confirming its reliability. Through this modeling process, we identified ten influential risk factors for PMS in nurses, including tea or coffee consumption, sleep quality, regularity of the menstrual cycle, number of bleeding between menstruation, the degree of dysmenorrhea, level of bullying, trait coping style, anxiety, depression and perceived stress. According to the nomogram model, dysmenorrhea was the most significant factor among the ten factors, followed by depression, perceived stress and anxiety.
The results of our study indicated the significant influence of menstrual cycle regularity on PMS. This observation might be elucidated through hormonal mechanisms in the endocrine system. Hormones like progesterone, luteinizing hormone and prolactin play pivotal roles in maintaining normal menstruation in women, and their imbalances could lead to menstrual disorders, which might contribute to the development of PMS or mediate some of the neuropsychiatric symptoms associated with PMS (44–46). In addition, the conclusion of the menstrual cycle aligns with Eshetu’s (15) and Tarannum’s (47) argument that irregular menstrual cycles were a risk factor for PMS.
In terms of menstruation-related factors, our results also revealed a high prevalence of dysmenorrhea (79.2%) among participants with PMS, reaffirming the established association between dysmenorrhea and PMS, while emphasizing the role of dysmenorrhea as the most important risk factor in PMS (14, 48). We also found that the increased frequency of intermenstrual bleeding occurrences was also a risk factor for PMS. This observation may be linked to ovulation-related factors, as bleeding during ovulation often results from a short-term decline in estrogen levels that is insufficient to maintain endometrial stability, leading to small vaginal bleeds during ovulation. At present, its etiology was not clear, hypothalamic–pituitary-ovarian axis disruption may be implicated. However, menarche age was not identified as a PMS risk factor in our study, which is inconsistent with previous research results, which studies students (15, 49), suggesting potential recall bias among participants.
Our study further indicated that nurses who reported coffee or tea consumption were more likely to develop PMS than non-consumers, which also supported previous reports (48). At present, Existing research supports a link between coffee intake and adverse effects on female hormone levels, including lower estrogen and sex hormone binding globulin (SHBG) (50, 51). However, a longitudinal study has yielded opposite results (20), necessitating further investigation into this relationship.
An interesting finding in our study was that shift frequency among nurses was not associated with the occurrence of PMS. While a previous study found that PMS negatively affected work in nurses with three rotating shifts, it did not specifically attribute this to PMS (52). Furthermore, our results highlighted that poor sleep quality, often attributed to hormonal fluctuations and emotional disturbances in women’s menstrual cycles, was a significant PMS risk factor (21, 22, 53). Poor sleep quality leads to fatigue, drowsiness and irritability the next day, which will adversely affect nursing quality.
Our study also illuminated the predictive value of workplace bullying, personality traits, anxiety, depression and perceived stress in relation to PMS. Introverted personalities and occupational stress are more prone to PMS (22, 24, 54). Introverted individuals tend to experience negative emotions such as anxiety, depression, and anger, contributing to PMS development. Elevated levels of fatigue, depressed mood, anxiety and decreased interest in daily activities have been identified as strong PMS predictors (55). Additionally, the autonomic nervous system (ANS) may play a role, as high-intensity cognitive social stress can lead to ANS responses characterized by low arousal and delayed recovery in PMS-affected women. When faced with stress and adverse emotional experiences, their ANS response may render them unable to cope with stress stimuli and emotional experiences (56). However, scant research has explored the impact of workplace bullying on PMS in nurses. The noteworthy discovery that workplace bullying serves as a predictor of PMS diverges from findings in the general population. We hypothesize that association may be attributed to the adverse effects of workplace bullying, such as diminished job satisfaction and the increased possibility of experiencing negative consequences, including depression, stress, and other physical and psychological symptoms, which can contribute to the development of premenstrual syndrome (57, 58). Therefore, future research should delve deeper into the influence of social factors on nurses with PMS.
5. Limitations and recommendations
Despite being one of the most extensive studies involving a substantial sample of Chinese nurses and pioneering the exploration of a risk prediction model for PMS in this population, there were some limitations that necessitate consideration. Firstly, our study’s cross-sectional nature precludes the establishment of causal relationships between the identified factors and PMS. To facilitate a more comprehensive understanding of these associations, we recommend future research to prioritize longitudinal investigations. Secondly, reliance on self-reported measurements in this study introduces the potential for recall bias due to the inherent subjectivity of such assessments. Thirdly, the utilization of the number of sanitary napkins as the method for measuring menstrual flow may introduce measurement bias. However, this study offers valuable insights with implications for clinical practice and preventative strategies. We aspire that the observed higher incidence of PMS serves as a catalyst for increased awareness among nurses and healthcare administrators. In addition, we strongly recommend that nurses with menstrual abnormalities can seek medical evaluation and intervention. Finally, we also highly endorse the establishment of nurse counseling centers, the implementation of staff wellness programs, or the integration of virtual reality technology to bolster nurses’ mental health and overall well-being. A healthier nursing workforce is pivotal for ensuring the safety and care quality of patients.
6. Conclusion
The prediction model developed in this research demonstrates its potential to effectively predict PMS risk in nurses. The identified risk factors include severe dysmenorrhea, intermenstrual bleeding, irregular menstrual cycle, severe depression and anxiety, high stress, negative coping style, workplace bullying, poorer sleep quality and tea or coffee consumption increased the risk of PMS in nurses. As we move forward, it is crucial to refine the risk prediction model, validate its effectiveness in clinical settings, and continue exploring innovative approaches to support nurses in managing and mitigating the impact of PMS.
Data availability statement
The data analyzed in this study is subject to the following licenses/restrictions: the data presented in this paper is not readily available. Because the data from the TARGET Nurses’ health cohort study needs time for data clearing and establishment of guidelines. We are planning to make this data available to the public in the future. Requests to obtain data should be sent directly to Y2FveWpAc2R1LmVkdS5jbg==. Requests to access these datasets should be directed to Y2FveWpAc2R1LmVkdS5jbg==.
Ethics statement
The studies involving humans were approved by the Qilu Hospital of Shandong province’s ethics committee (Approved No. of ethic committee: KYLL-202210-060-1). The studies were conducted in accordance with the local legislation and institutional requirements. The participants provided their written informed consent to participate in this study.
Author contributions
YC: conceptualization, resources, supervision, and project administration. LL, XL, and YL: methodology and writing-review and editing. XL and YL: project administration and data curation. LL and YL: software. XZ and ML: investigation. LL: writing-original draft preparation, formal analysis, and visualization. All authors have read and agreed to the published version of the manuscript.
Funding
The study was funded by the Key Projects of Shandong Medical System Staff Science and Technology Innovation Program (Grant number SDYWZGKCJH2023090).
Acknowledgments
The authors are grateful to the nurses who participated in the TARGET Nurses’ health cohort. The authors would like to thank TopEdit (www.topeditsci.com) for its linguistic assistance during the preparation of this manuscript.
Conflict of interest
The authors declare that the research was conducted in the absence of any commercial or financial relationships that could be construed as a potential conflict of interest.
Publisher’s note
All claims expressed in this article are solely those of the authors and do not necessarily represent those of their affiliated organizations, or those of the publisher, the editors and the reviewers. Any product that may be evaluated in this article, or claim that may be made by its manufacturer, is not guaranteed or endorsed by the publisher.
Supplementary material
The Supplementary material for this article can be found online at: https://www.frontiersin.org/articles/10.3389/fpubh.2023.1203280/full#supplementary-material
Abbreviations
PMS, Premenstrual Syndrome; PMSS, Premenstrual Syndrome Scale; IPAQ, International Physical Activity Questionnaire; PSQI, Pittsburgh Sleep Quality Index; NAQ-R, Negative Acts Questionnaire-revised; PSSS, Perceived Social Support Scale; PSS, Perceived Stress Scale; SHBG, Sex Hormone Binding Globulin; TCSQ, Trait Coping Style Questionnaire; ANS, Autonomic Nervous System.
References
1. Freeman, EW . Premenstrual syndrome and premenstrual dysphoric disorder: definitions and diagnosis. Psychoneuroendocrinology. (2003) 28:25–37. doi: 10.1016/s0306-4530(03)00099-4
2. Erbil, N, and Yücesoy, H. Premenstrual syndrome prevalence in Turkey: a systematic review and meta-analysis. Psychol Health Med. (2023) 28:1347–57. doi: 10.1080/13548506.2021.2013509
3. Aolymat, I, Khasawneh, AI, and Al-Tamimi, M. COVID-19-associated mental health impact on menstrual function aspects: dysmenorrhea and premenstrual syndrome, and genitourinary tract health: a cross sectional study among Jordanian medical students. Int J Environ Res Public Health. (2022) 19:1439. doi: 10.3390/ijerph19031439
4. Ji, N, Liu, SW, Zeng, XY, Dong, WL, Jiang, YY, and Zhou, MG. Disease burden for gynecological disease in China, 2016. Chin J Obstet Gynecol. (2018) 53:313–8. doi: 10.3760/cmaj.issn.0529-567x.2018.05.006
5. Li, H, Qi, MX, and Guan, XR. Trend of disease burden of gynecological diseases in China from 1990 to 2019. Modern. Prev Med. (2021) 48:3322–36.
6. Ranjbaran, M, Omani Samani, R, Almasi-Hashiani, A, Matourypour, P, and Moini, A. Prevalence of premenstrual syndrome in Iran: a systematic review and meta-analysis. Int J Reprod Biomed. (2017) 15:679–6. doi: 10.34172/hpp.2021.20
7. Chumpalova, P, Iakimova, R, Stoimenova-Popova, M, Aptalidis, D, Pandova, M, Stoyanova, M, et al. Prevalence and clinical picture of premenstrual syndrome in females from Bulgaria. Ann General Psychiatry. (2020) 19:3. doi: 10.1186/s12991-019-0255-1
8. Geta, TG, Woldeamanuel, GG, and Dassa, TT. Prevalence and associated factors of premenstrual syndrome among women of the reproductive age group in Ethiopia: systematic review and meta-analysis. PLoS One. (2020) 15:e0241702. doi: 10.1371/journal.pone.0241702
9. Hantsoo, L, Rangaswamy, S, Voegtline, K, Salimgaraev, R, Zhaunova, L, and Payne, JL. Premenstrual symptoms across the lifespan in an international sample: data from a mobile application. Arch Womens Ment Health. (2022) 25:903–16. doi: 10.1007/s00737-022-01261-5
10. Hou, L, Chen, L, and Zhang, W. The longitudinal predictive effect of self-reported frequency of premenstrual syndrome on depression: findings from the Australian longitudinal study on Women's health. Front Public Health. (2023) 11:1126190. doi: 10.3389/fpubh.2023.1126190
11. Prasad, D, Wollenhaupt-Aguiar, B, Kidd, KN, de Azevedo, CT, and Frey, BN. Suicidal risk in women with premenstrual syndrome and premenstrual dysphoric disorder: a systematic review and Meta-analysis. J Womens Health (Larchmt). (2021) 30:1693–07. doi: 10.1089/jwh.2021.0185
12. Heinemann, LA, Minh, TD, Heinemann, K, Lindemann, M, and Filonenko, A. Intercountry assessment of the impact of severe premenstrual disorders on work and daily activities. Health Care Women Int. (2012) 33:109–4. doi: 10.1080/07399332.2011.610530
13. Hou, L, and Zhou, R. Patterns of premenstrual syndrome and depression symptoms in Chinese female university students: results of a latent profile analysis. J Affect Disord. (2021) 293:64–70. doi: 10.1016/j.jad.2021.06.017
14. Mao, C . Premenstrual Syndrome and its Related Factors in Female Nursing Students: A Network Analysis. Master Thesis, Shandong University, Jinan, Shandong. (2021).
15. Eshetu, N, Abebe, H, Fikadu, E, Getaye, S, Jemal, S, Geze, S, et al. Premenstrual syndrome, coping mechanisms and associated factors among Wolkite university female regular students, Ethiopia, 2021. BMC Womens Health. (2022) 22:88. doi: 10.1186/s12905-022-01658-5
16. Kahyaoglu Sut, H, and Mestogullari, E. Effect of premenstrual syndrome on work-related quality of life in Turkish nurses. Saf Health Work. (2016) 7:78–82. doi: 10.1016/j.shaw.2015.09.001
17. Thakur, H, Pareek, P, Sayyad, MG, and Otiv, S. Association of Premenstrual Syndrome with adiposity and nutrient intake among young Indian women. Int J Women's Health. (2022) 14:665–5. doi: 10.2147/IJWH.S359458
18. Park, J, Lee, JJ, Park, S, Lee, H, Nam, S, Lee, S, et al. Endocrine disrupting chemicals and premenstrual syndrome in female college students in East Asia: a multi-country study. Int J Women's Health. (2022) 14:167–7. doi: 10.2147/IJWH.S349172
19. Choi, SH, and Hamidovic, A. Association between smoking and premenstrual syndrome: a Meta-analysis. Front Psych. (2020) 11:575526. doi: 10.3389/fpsyt.2020.575526
20. Purdue-Smithe, AC, Manson, JE, Hankinson, SE, and Bertone-Johnson, ER. A prospective study of caffeine and coffee intake and premenstrual syndrome. Am J Clin Nutr. (2016) 104:499–7. doi: 10.3945/ajcn.115.127027
21. Erbil, N, and Yücesoy, H. Relationship between premenstrual syndrome and sleep quality among nursing and medical students. Perspect Psychiatr Care. (2022) 58:448–5. doi: 10.1111/ppc.12628
22. Baek, J, and Choi-Kwon, S. Secondary data analysis on the factors influencing premenstrual symptoms of shift work nurses: focused on the sleep and occupational stress. J Korean Acad Nurs. (2020) 50:631–16. doi: 10.4040/jkan.19230
23. Lee, W, Lee, S, Ahn, J, Lee, RS, and Kang, SK. Premenstrual syndrome incidence rate and risk factors among the working population in the Republic of Korea: a prospective cohort study. BMC Womens Health. (2022) 22:265. doi: 10.1186/s12905-022-01852-5
24. Arslantaş, H, Abacigil, F, and Çinakli, Ş. Relationship between premenstrual syndrome and basic personality traits: a cross-sectional study. São Paulo Med J. (2018) 136:339–5. doi: 10.1590/1516-3180.2018.0061240418
25. Chang, HY, and Park, S. Effects of exposure to endocrine disruptors, burnout, and social support from peers on premenstrual syndrome in nurses. Korean J Women Health Nurs. (2020) 26:171–9. doi: 10.4069/kjwhn.2020.06.18
26. Lv, X, Cao, Y, Li, Y, Liu, Y, Li, R, Guan, X, et al. The TARGET Nurses' health cohort study protocol towards a revolution in getting nurses' health ticked. J Adv Nurs. (2022) 78:1815–3. doi: 10.1111/jan.15204
27. Zhao, GL, Wang, LH, and Qu, CY. Prevalence of premenstrual syndrome in reproductive women and its influential factors. China. J Obstet Gynecol. (1998) 4:29–31. doi: 10.3760/j.issn:0529-567X.1998.04.009
28. Bancroft, J . The premenstrual syndrome--a reappraisal of the concept and the evidence. Psychol Med. (1993) 24:1–47. doi: 10.1017/s0264180100001272
29. Craig, CL, Marshall, AL, Sjöström, M, Bauman, AE, Booth, ML, Ainsworth, BE, et al. International physical activity questionnaire: 12-country reliability and validity. Med Sci Sports Exerc. (2003) 35:1381–95. doi: 10.1249/01.MSS.0000078924.61453.FB
30. Qu, NN, and Li, KJ. Study on the reliability and validity of international physical activity questionnaire (Chinese vision, IPAQ). Zhonghua Liu Xing Bing Xue Za Zhi. (2004) 25:265–8. doi: 10.3760/j.issn:0254-6450.2004.03.021
31. Buysse, DJ, Reynolds, CF 3rd, Monk, TH, Berman, SR, and Kupfer, DJ. The Pittsburgh sleep quality index: a new instrument for psychiatric practice and research. Psychiatry Res. (1989) 28:193–213. doi: 10.1016/0165-1781(89)90047-4
32. Lu, TY, Li, Y, Xia, P, Zhang, GQ, and Wu, DR. Analysis on reliability and validity of the Pittsburgh. Chongqing Med. (2014) 43:260–3. doi: 10.3969/j.issn.1671-8348.2014.03.002
33. Einarsen, S, Hoel, H, and Notelaers, G. Measuring exposure to bullying and harassment at work: validity, factor structure and psychometric properties of the negative acts questionnaire-revised. Work Stress. (2009) 23:24–44. doi: 10.1080/02678370902815673
34. Xun, LHX, and Tian, ZL. A preliminary reliability and validity study of the Chinese version of the negative. Chinese. Nurs Manag. (2012) 12:21–4. doi: 10.3969/j.issn.1672-1756.2012.06.006
35. Zimet, GD, Powell, SS, Farley, GK, Werkman, S, and Berkoff, KA. Psychometric characteristics of the multidimensional scale of perceived social support. J Pers Assess. (1990) 55:610–7. doi: 10.1080/00223891.1990.9674095
36. Zhong, X, Jiang, QJ, Wu, ZX, and Qian, LJ. Effect of life events, social support, stress response on coping style in medical personnel (in Chinese). Chin J Behav Med Sci. (2004) 13:560–2. doi: 10.3760/cma.j.issn.1674-6554.2004.05.044
37. Wang, X, Wang, X, and Ma, H. Rating scales for mental health. China J Ment Health Press. (1999) 1:120.
38. Cohen, S, Kamarck, T, and Mermelstein, R. A global measure of perceived stress. J Health Soc Behav. (1983) 24:385–6. doi: 10.2307/2136404
39. Yang, TZ, and Huang, HT. An epidemiological study on stress among urban residents in social transition period. Zhonghua Liu Xing Bing Xue Za Zhi. (2003) 24:760–4. doi: 10.3760/j.issn:0254-6450.2003.09.004
40. Spitzer, RL, Kroenke, K, Williams, JB, and Löwe, B. A brief measure for assessing generalized anxiety disorder: the GAD-7. Arch Intern Med. (2006) 166:1092–7. doi: 10.1001/archinte.166.10.1092
41. He, XY, Li, CB, Qian, J, Cui, HS, and Wu, WY. Reliability and validity of a generalized anxiety disorder scale in general hospital outpatients. Arch Psych. (2010) 22:200–3. doi: 10.3969/j.issn.1002-0829.2010.04.002
42. Kroenke, K, Spitzer, RL, and Williams, JB. The PHQ-9: validity of a brief depression severity measure. J Gen Intern Med. (2001) 16:606–3. doi: 10.1046/j.1525-1497.2001.016009606.x
43. Wang, W, Bian, Q, Zhao, Y, Li, X, Wang, W, Du, J, et al. Reliability and validity of the Chinese version of the patient health questionnaire (PHQ-9) in the general population. Gen Hosp Psychiatry. (2014):36. doi: 10.1016/j.genhosppsych.2014.05.021
44. Smith, K, Archibong, AE, Ansah, TA, and Nayyar, T. Correlation between beta-arrestin1 and prolactin levels in women with depression during premenstrual dysphoric disorder. FASEB J. (2017) 31:1000.7. doi: 10.1096/fasebj.31.1_supplement.1000.7
45. Walsh, S, Ismaili, E, Naheed, B, and O"Brien, S. Diagnosis, pathophysiology and management of premenstrual syndrome. Obstet Gynaecol. (2015) 17:99–4. doi: 10.1111/tog.12180
46. Roomruangwong, C, Carvalho, AF, Comhaire, F, and Maes, M. Lowered plasma steady-state levels of progesterone combined with declining progesterone levels during the luteal phase predict Peri-menstrual syndrome and its major subdomains. Front Psychol. (2019) 10:2446. doi: 10.3389/fpsyg.2019.02446
47. Tarannum, F, Khalique, N, and Eram, U. Premenstrual syndrome: prevalence, symptoms, and associated risk factors among adolescent girls in Aligarh Uttar Pradesh. Indian J Public Health. (2021) 65:396–9. doi: 10.4103/ijph.ijph_985_21
48. Thakur, J, Goswami, M, and Roy, S. Do contrasting socio-ecological conditions bring difference in premenstrual syndrome and its concomitants? A sedente-migrant comparative study from eastern India. Am J Hum Biol. (2023) 5:e23955. doi: 10.1002/ajhb.23955
49. Bhuvaneswari, K, Rabindran, P, and Bharadwaj, B. Prevalence of premenstrual syndrome and its impact on quality of life among selected college students in Puducherry. Natl Med J India. (2019) 32:17–9. doi: 10.4103/0970-258X.272109
50. Hang, D, Kværner, AS, Ma, W, Hu, Y, Tabung, FK, Nan, H, et al. Coffee consumption and plasma biomarkers of metabolic and inflammatory pathways in US health professionals. Am J Clin Nutr. (2019) 109:635–7. doi: 10.1093/ajcn/nqy295
51. Jiang, Z, He, R, Wu, H, Yu, J, Zhu, K, Luo, Q, et al. The causal association between smoking initiation, alcohol and coffee consumption, and women's reproductive health: a two-sample Mendelian randomization analysis. Front Genet. (2023) 14:1098616. doi: 10.3389/fgene.2023.1098616
52. Mayama, M, Umazume, T, Watari, H, Nishiguchi, S, Moromizato, T, and Watari, T. Frequency of night shift and menstrual cycle characteristics in Japanese nurses working under two or three rotating shifts. J Occup Health. (2020) 62:e12180. doi: 10.1002/1348-9585.12180
53. Aşcı, Ö, Gökdemir, F, Kahyaoğlu Süt, H, and Payam, F. The relationship of premenstrual syndrome symptoms with menstrual attitude and sleep quality in Turkish nursing student. J Caring Sci. (2015) 4:179–7. doi: 10.15171/jcs.2015.018
54. Erenolu, R, and Szbir, EY. Are premenstrual syndrome and dysmenorrhea related to the personality structure of women? A descriptive relation-seeker type study. Perspect Psychiatr Care. (2020) 56:979–4. doi: 10.1111/ppc.12551
55. Śliwerski, A, and Koszałkowska, K. The influence of depression on biased diagnosis of premenstrual syndrome and premenstrual dysphoric disorder by the PSST inventory. Life (Basel). (2021) 11:1278. doi: 10.3390/life11111278
56. Meng, Y, Chang, L, Hou, L, and Zhou, R. Menstrual attitude and social cognitive stress influence autonomic nervous system in women with premenstrual syndrome. Stress. (2022) 25:87–96. doi: 10.1080/10253890.2021.2024163
57. Coetzee, M, and van Dyk, J. Workplace bullying and turnover intention: exploring work engagement as a potential mediator. Psychol Rep. (2018) 121:375–2. doi: 10.1177/0033294117725073
Keywords: premenstrual syndrome, nurses, risk factors, nomogram, model validation
Citation: Li L, Lv X, Li Y, Zhang X, Li M and Cao Y (2023) Development and validation of risk prediction model for premenstrual syndrome in nurses: results from the nurses-based the TARGET cohort study. Front. Public Health. 11:1203280. doi: 10.3389/fpubh.2023.1203280
Edited by:
Juan Gómez-Salgado, University of Huelva, SpainReviewed by:
Priyanka Pareek, Mahatma Gandhi Mission Institute of Health Sciences, IndiaAna Penjak, University of Split, Croatia
Copyright © 2023 Li, Lv, Li, Zhang, Li and Cao. This is an open-access article distributed under the terms of the Creative Commons Attribution License (CC BY). The use, distribution or reproduction in other forums is permitted, provided the original author(s) and the copyright owner(s) are credited and that the original publication in this journal is cited, in accordance with accepted academic practice. No use, distribution or reproduction is permitted which does not comply with these terms.
*Correspondence: Yingjuan Cao, Y2FveWpAc2R1LmVkdS5jbg==