- 1Department of Cardiology, The First Affiliated Hospital of Shandong First Medical University and Shandong Provincial Qianfoshan Hospital, Shandong Medicine and Health Key Laboratory of Cardiac Electrophysiology and Arrhythmia, Jinan, China
- 2Shandong First Medical University and Shandong Academy of Medical Sciences, Jinan, China
Background: Previous observational studies have shown that the prevalence of cardiovascular diseases (CVDs) is related to particulate matter (PM). However, given the methodological limitations of conventional observational research, it is difficult to identify causality conclusively. To explore the causality of PM on CVDs and cardiovascular biomarkers, we conducted a Mendelian randomization (MR) analysis.
Method: In this study, we obtained summary-level data for CVDs and cardiovascular biomarkers including atrial fibrillation (AF), heart failure (HF), myocardial infarction (MI), ischemic stroke (IS), stroke subtypes, body mass index (BMI), lipid traits, fasting glucose, fasting insulin, and blood pressure from several large genome-wide association studies (GWASs). Then we used two-sample MR to assess the causality of PM on CVDs and cardiovascular biomarkers, 16 single nucleotide polymorphisms (SNPs) for PM2.5 and 6 SNPs for PM10 were obtained from UK Biobank participants. Inverse variance weighting (IVW) analyses under the fixed effects model were used as the main analytical method to calculate MR Estimates, followed by multiple sensitivity analyses to confirm the robustness of the results.
Results: Our study revealed increases in PM2.5 concentration were significantly related to a higher risk of MI (odds ratio (OR), 2.578; 95% confidence interval (CI), 1.611–4.127; p = 7.920 × 10−5). Suggestive evidence was found between PM10 concentration and HF (OR, 2.015; 95% CI, 1.082–3.753; p = 0.027) and IS (OR, 2.279; 95% CI,1.099–4.723; p = 0.027). There was no evidence for an effect of PM concentration on other CVDs. Furthermore, PM2.5 concentration increases were significantly associated with increases in triglyceride (TG) (OR, 1.426; 95% CI, 1.133–1.795; p = 2.469 × 10−3) and decreases in high-density lipoprotein cholesterol (HDL-C) (OR, 0.779; 95% CI, 0.615–0.986; p = 0.038). The PM10 concentration increases were also closely related to the decreases in HDL-C (OR, 0.563; 95% CI, 0.366–0.865; p = 8.756 × 10−3). We observed no causal effect of PM on other cardiovascular biomarkers.
Conclusion: At the genetic level, our study suggested the causality of PM2.5 on MI, TG, as well HDL-C, and revealed the causality of PM10 on HF, IS, and HDL-C. Our findings indicated the need for continued improvements in air pollution abatement for CVDs prevention.
1. Introduction
Globally, one in three deaths is caused by cardiovascular diseases (CVDs) which place a heavy burden on health systems (1). It is essential to find ways to prevent and treat CVDs to reduce their global burden. It’s been a hot topic lately the connection between air pollution and CVDs. The increasing urbanization of the world has led to the exposure of more than 90% of the world’s population to levels of air pollution that exceed the guidelines set by the World Health Organization. As a result of air pollution, both developed and developing countries are facing serious public health concerns. Exposure to outside air pollution is generally identified as a challenge for public health agencies and physicians, especially to fine particulate matter (PM). PM are made up of an intricate mixture of liquid and solid particles, as well as inorganic and organic compounds. Particles are generally classified by size into coarse (aerodynamic diameter < 10 μm; PM10), fine (diameter < 2.5 μm; PM2.5) fractions (2). Due to its high level of danger for human health, PM can be used as a reliable proxy for ambient air pollution related morbidity and mortality (3). During the past decade, researchers have examined the association between PM and human health using epidemiological methods (4–6). Furthermore, despite the fact that PM exposure used to be thought to pose a threat to health, especially the lungs (7), the comprehensive clue now shows the greatest inverse impact occurs in the cardiovascular system (8, 9). A cross-sectional study undertaken in Ahvaz megacity showed that exposure to air pollution is significantly associated with cardiovascular mortality (CM), hospital admissions for cardiovascular disease (HACD), and hospital admission for respiratory disease (HARD) (10). Besides, a study by Dastoorpoor et al. also showed that a higher concentration of PM10 is associated with an increased risk of hospitalization for CVDs (11). Similarly, Moradi and his colleagues noted that exposure to PM, even at low concentrations, is related to an increased risk of cardiovascular diseases hospitalizations (12). Furthermore, several studies also demonstrated that PM increases the risk of CVDs such as atrial fibrillation (AF), heart failure (HF), myocardial infarction (MI), and ischemic stroke (IS) (13–16). However, several studies reported inconclusive conclusions (17–21). In addition, most of the evidence is based on observational studies. Due to residual confounding and reverse causation, these studies have difficulty identifying causality conclusively.
When evaluating the health risks of air pollutant exposure, individual health risks may be better comprehended by considering genetic diversity. It is becoming clearer that the impacts of air pollution vary by individual, with some populations being more vulnerable to its adverse effects. Genetic predisposition appears to play a crucial role in response to air pollution (22). Previous studies have revealed that exposure to PM2.5 causes negative health consequences driven by alteration of gene expression (23, 24). A study conducted by Yao et al. showed that participants with 2 alleles of SIRT1_391 can counteract the adverse effect of PM2.5 and reduce the 26.1% risk of premature mortality (25). Poursafa et al. revealed that synergistic effect of the TM-33G / A polymorphism and air pollutants on factors associated with the onset of atherosclerosis (26). To analyze the effect of genetic polymorphisms and PM on CVDs and cardiovascular biomarkers, we conduct Mendelian randomization (MR) analysis to examine the relationship between them by using data from the publicly available genome-wide association study (GWAS) and UK Biobank.
2. Method
2.1. Study design
MR analysis is applied to investigate the relationship between exposure and outcome, which can provide robust causality by utilizing one or multiple genetic variants, such as single nucleotide polymorphisms (SNPs) (27, 28). The MR study was built on the Mendelian inheritance rule, which states that the parents’ genetic alleles are randomly dispersed to the descendants during the process of meiosis, which is supposed to be equivalent to RCT. As a result, the MR design can be used to account for some intractable questions of causality due to high costs or ethical issues, avoiding the biases of observational studies. Therefore, this study intends to examine the causal relationships of PM with CVDs and cardiovascular biomarkers by MR analysis. CVDs included AF, HF, MI, IS, and stroke subtypes. Cardiovascular biomarkers contained body mass index (BMI), triglyceride (TG), high-density lipoprotein cholesterol (HDL-C), low-density lipoprotein cholesterol (LDL-C), fasting glucose, fasting insulin, diastolic blood pressure (DBP), and systolic blood pressure (SBP). MR analysis must satisfy three assumptions. First, IVs must be closely related to exposure. Second, the SNPs have no relationship to potential confounders. Third, IVs can only cause outcomes through exposure (29) (Figure 1).
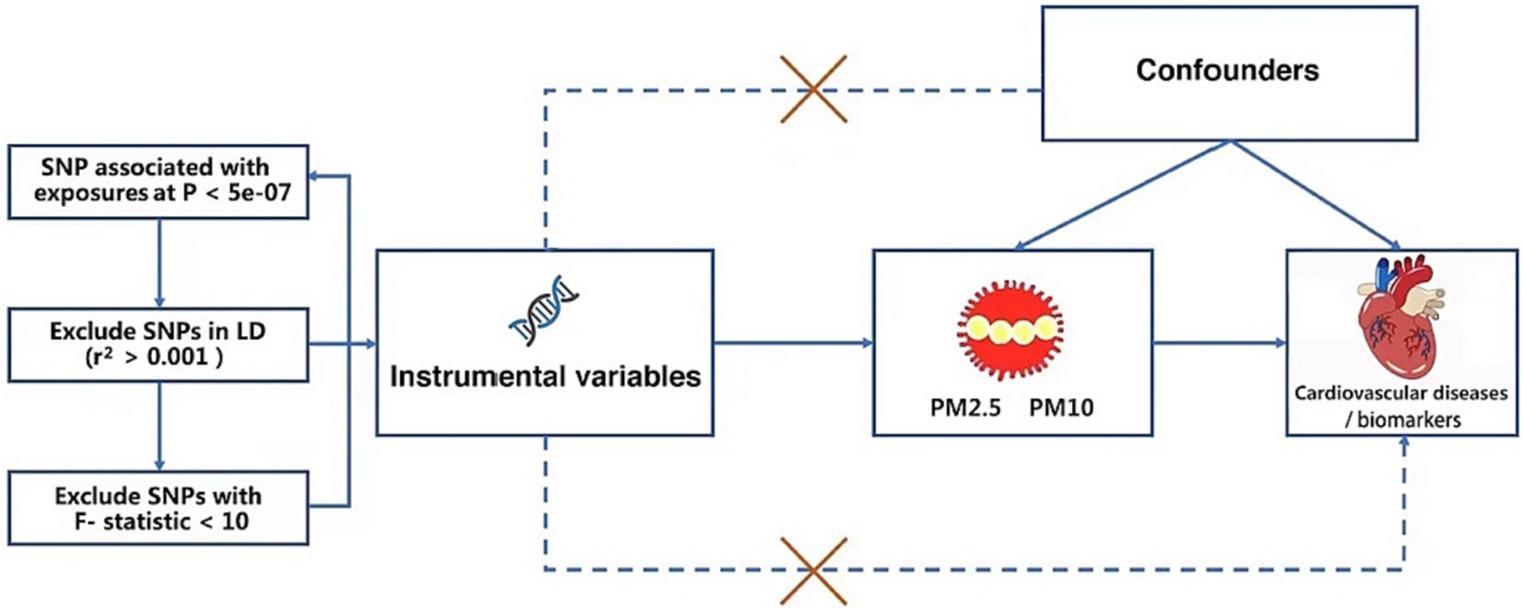
Figure 1. The specific steps for selecting SNPs and three crucial hypotheses of the Mendelian randomization study. PM, particulate matter. SNP, single nucleotide polymorphism.
2.2. Data sources
2.2.1. GWAS summary data for PM and CVDs
GWAS summary statistics for PM were obtained from the UK Biobank, including about 423,796 participants from Europe, both male and female, which assessed the relationship between PM and SNPs. Briefly, this GWAS examined two PM phenotypes, including PM10 (n = 423,796) and PM2.5 (n = 423,796). In the case of AF (n = 103,083,6), we acquired summary-level data from the GWAS meta-analysis reported by Nielsen et al. (30), which included 60,620 AF cases and 970,216 controls and revealed 142 independent risk variants at 111 loci and prioritized 151 functional candidate genes likely to be involved in atrial fibrillation. MI (n = 395,795) was obtained from the GWAS conducted by Hartiala et al., which contained 395,795 participants from Europe, both male and female (31). HF (n = 977,323) was gained from the Heart Failure Molecular Epidemiology for Therapeutic Targets consortium (HERMES) conducted by Shah et al. (32). The GWAS comprised 47,309 HF cases and 930,014 controls, showing 12 independent variants at 11 genomic loci were associated with HF. IS (n = 440,328), large artery stroke (n = 150,765), small vessel stroke (n = 1988,048) and cardioembolic stroke (n = 211,763) was had access to the results conducted by Malik et al. (33), who tested 8 million SNPs in up to 67,162 stroke cases and 454,450 controls and revealed twenty-one additional loci were associated with stroke in this study. Descriptions of the datasets used in the analysis can be found in Table 1.
2.2.2. GWAS summary data for cardiovascular biomarkers
The GWAS summary statistics of BMI were accessible to the study conducted by Wood et al., including 119,688 individuals from European (34). The GWAS summary statistics for lipid traits were available from the Within family GWAS consortium, containing TG, HDL-C, LDL-C, which included 78,700, 77,409, and 70,814 participants from Europe, both male and female. The GWAS summary statistics for fasting insulin and fasting glucose were obtained from the study conducted by Chen et al. (35). The GWAS summary statistics for blood pressure were accessible to the consortium of the MRC-IEU, including DBP and SBP. Descriptions of the datasets used in the analysis can be found in Table 1.
2.3. Selection and validation of SNPs
After we set the threshold of the p value as 5 × 10−8, we did not obtain any independent SNPs from the GWAS of PM10. In order to contain more SNPs that are concerned with PM10, we used a more lenient criterion (p < 5 × 10−7) which had been applied to previous MR research (36). Then we identified 16 SNPs connected with PM2.5 and 6 independent SNPs connected with PM10 at the genome-wide significance level (p < 5 × 10−7), showing the low likelihood of weak instrumental variable bias in MR analysis. No SNP was directly associated with CVDs or cardiovascular biomarkers. Using the TwoSampleMR R package, we conducted clumping functions in order to pick genetic variants without any linkage disequilibrium (LD) (r2 < 0.001 across a 10,000 kb window) (37). At last, 16 independent SNPs related to PM2.5 and 6 independent SNPs related to PM10 were determined; details can be found in the Supplementary Table. In addition, when the F-statistic is greater than 10, the SNPs were regarded as adequate to moderate the effect of potential bias, using the following formula: F = R2 × (N-2)/(1-R2) (38). No SNP was excluded from the MR analyses (The specific steps for selecting SNPs are shown in Figure 1).
2.4. MR analysis
This study used the two-sample MR method. As a primary analysis, we used inverse variance weighted (IVW) analyses under the fixed effects model as our main method because no heterogeneity was found in most analyses. Additionally, to ensure the results are robust, multiple complementary analyses were conducted like IVW under the random effects model, weighted median, and MR-egger. Sensitivity analyses like the MR-PRESSO test, Egger-intercept test, and the leave-one-out analysis are used to test whether the result of MR estimate is reliable. The Cochran Q test is mainly used to test and evaluate the heterogeneity of the selected IVs, which refers to the difference between the GWAS samples of exposure and outcome (39). We performed the MR-Egger intercept test in order to detect potential directional pleiotropy. It was determined that a significant pleiotropic bias existed when the intercept p-value <0.05 (40). We used the MR-PRESSO method to detect outliers before IVW methods were proceeded. MR-PRESSO eliminated abnormal SNPS (outliers) to detect potential horizontal pleiotropic and test whether there is a difference between the results before and after correction (41). The leave-one-out method was applied to analyze the sensitivity of the results by sequentially removing one SNP at a time to examine whether a single SNP with a large horizontal pleiotropy effect might affect the MR estimates. A total of MR analyses was performed using the R package “TwosampleMR.”
3. Result
3.1. Causal effect of PM and CVDs
According to the IVW analysis, PM2.5 concentration increases were significantly related to a higher risk of MI (OR, 2.578; 95% CI, 1.611–4.127; p = 7.920 × 10−5). Using the weighted median method, identical risk estimates were obtained as well (OR, 2.559; 95% CI, 1.303–5.029; p = 6.368 × 10−3) (Figure 2). Suggestive evidence was found between PM10 and HF (OR, 2.015; 95% CI, 1.082–3.753; p = 0.027) and IS (OR, 2.279; 95% CI, 1.099–4.723; p = 0.027) (Figure 3). In contrast, no causal relationship was found between PM2.5 and other CVDs (Figure 2). Furthermore, we did not observe the causality of PM10 on the risk of MI, AF, or stroke subtypes (Figure 3).
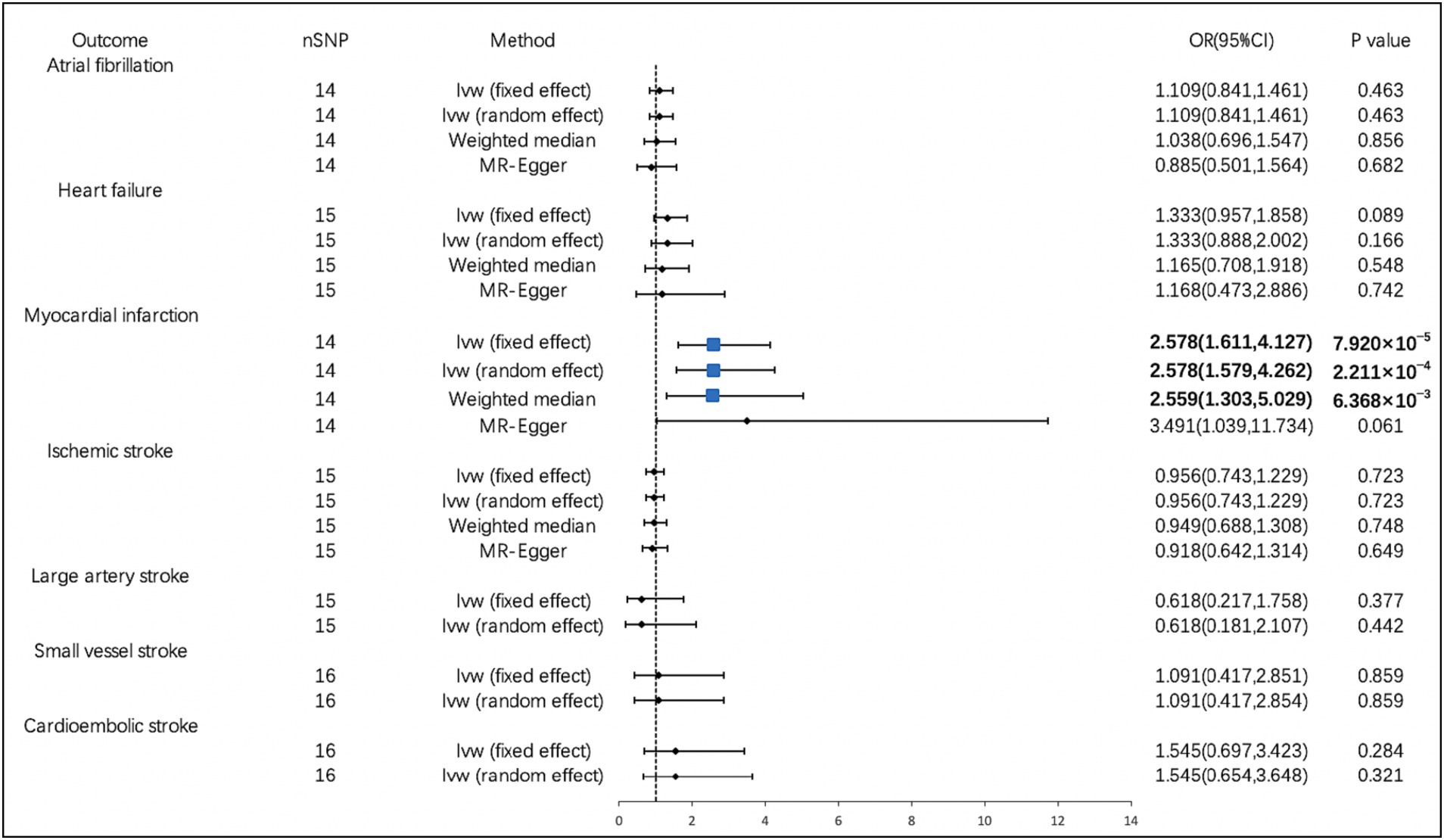
Figure 2. Associations of PM2.5 with cardiovascular diseases. CI, confidence interval; OR, odds ratio; SNP, single-nucleotide polymorphism.
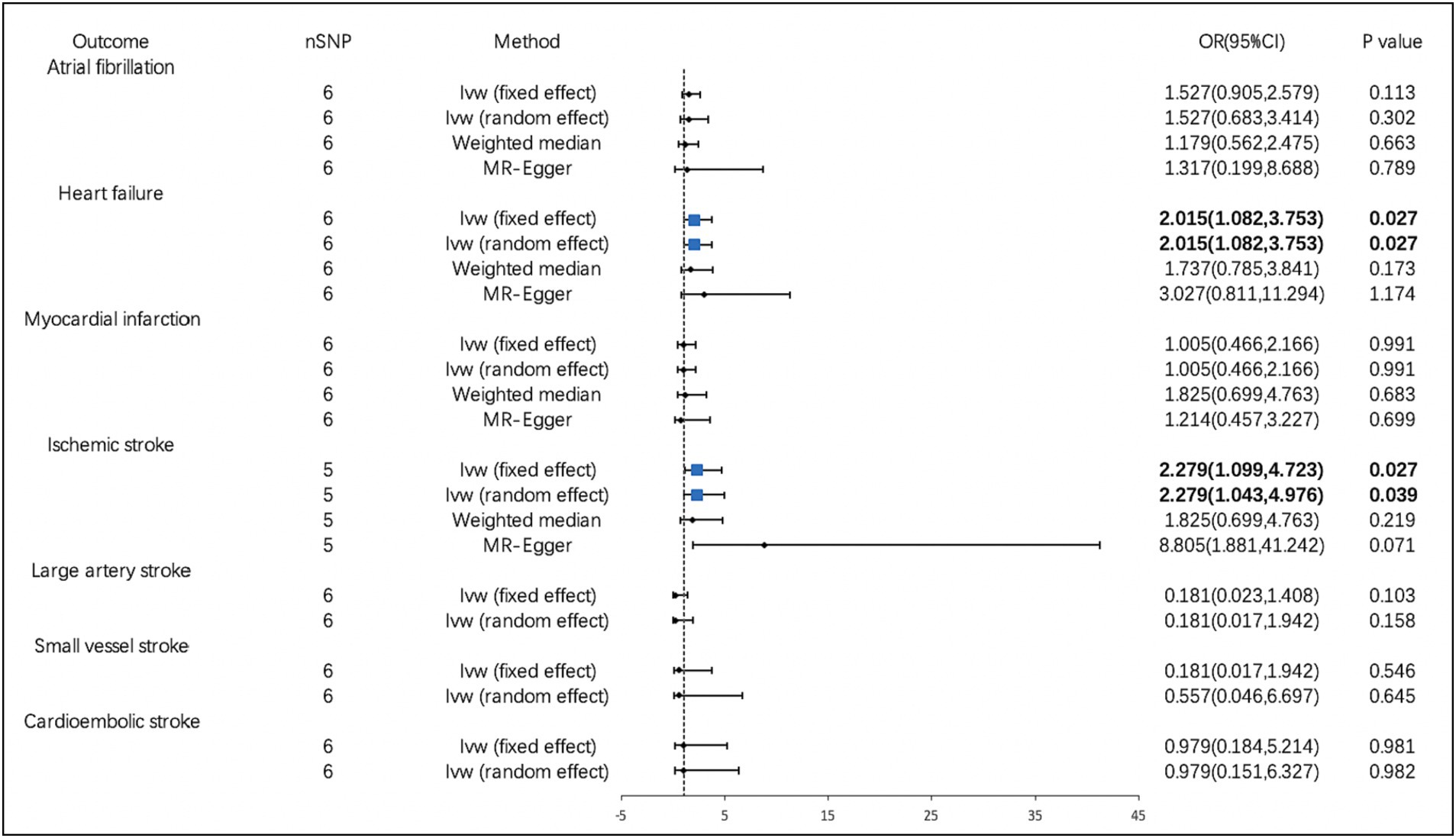
Figure 3. Associations of PM10 with cardiovascular diseases. CI, confidence interval; OR, odds ratio; SNP, single-nucleotide polymorphism.
3.2. Causal effect of PM and cardiovascular biomarkers
The IVW analysis revealed that the genetically predicted PM2.5 concentration increases were significantly associated with increases in TG (OR, 1.426; 95% CI, 1.133–1.795; p = 2.469 × 10−3). We also found that the increases in PM2.5 concentration were associated with the decreases in HDL-C (OR, 0.779; 95% CI, 0.615–0.986; p = 0.038). The PM10 concentration increases were also closely related to the decreases in HDL-C (OR, 0.563; 95% CI, 0.366–0.865; p = 8.756 × 10−3). We observed no causal effect of PM on BMI, LDL-C, fasting glucose, fasting insulin, DBP, or SBP. The results of the remaining methods can be found in Supplementary materials (Figure 4).
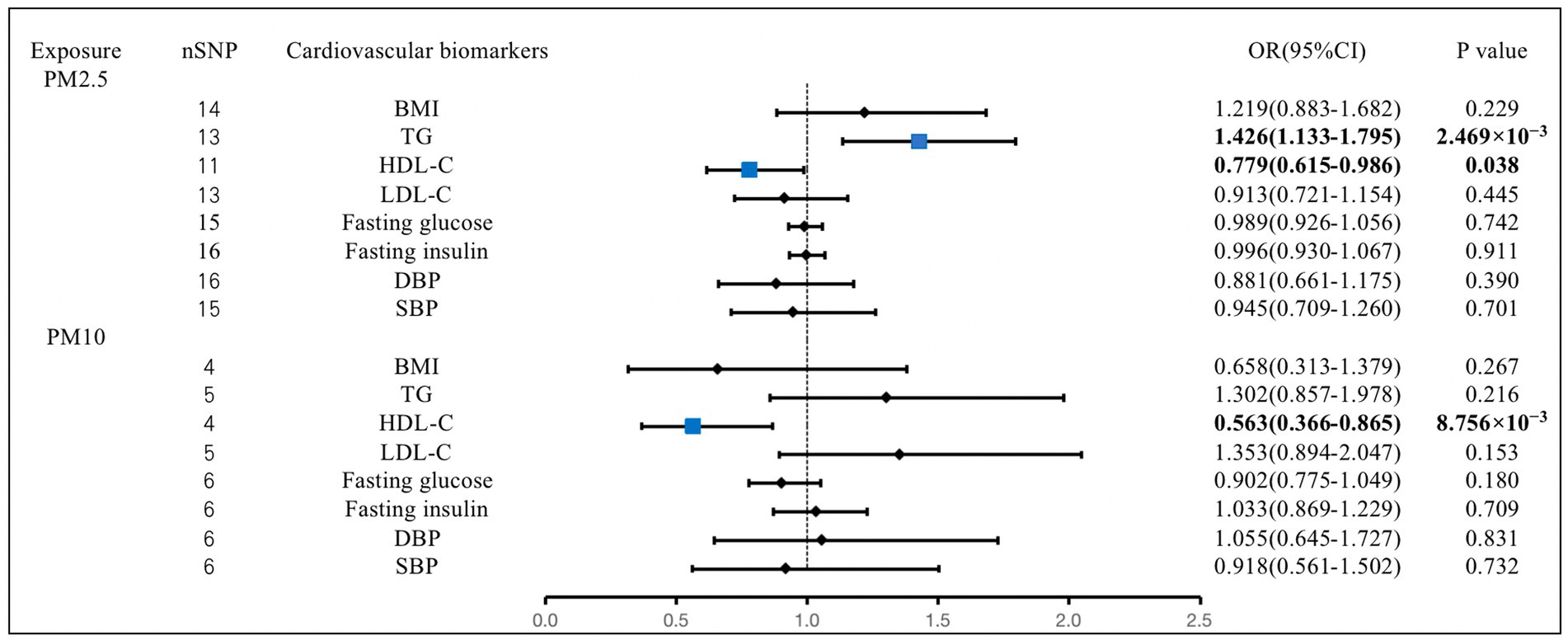
Figure 4. Associations of PM with cardiovascular biomarkers. CI, confidence interval; OR, odds ratio; SNP, single-nucleotide polymorphism. BMI, body mass index; TG, triglyceride; HDL-C, high-density lipoprotein cholesterol; LDL-C, low-density lipoprotein cholesterol; DBP, diastolic blood pressure; SBP, systolic blood pressure.
3.3. Sensitivity analysis
In sensitivity analysis, before we conducted the MR estimate, we used the method of MR-PRESSO to correct for the horizontal pleiotropy via outlier removal. After excluding these pleiotropic variants, no horizontal pleiotropy was found by conducted the MR-PRESSO method. For all outcomes, according to the MR-Egger regression, there did not appear to be horizontal pleiotropy based on the intercept term (Pintercept >0.05). We used the leave-one-out study to test the robustness of the results. All error lines are to the left of 0, indicating that the results are reliable and demonstrating that no SNPs with a large horizontal pleiotropic effect. We found modest heterogeneity in several analyses. However, heterogeneity did not affect the reliability of our conclusion. Plots of the leave-one-out analyses were also exhibited in Supplementary Figures. Details of the sensitivity analyses can be found in Tables 2–5.
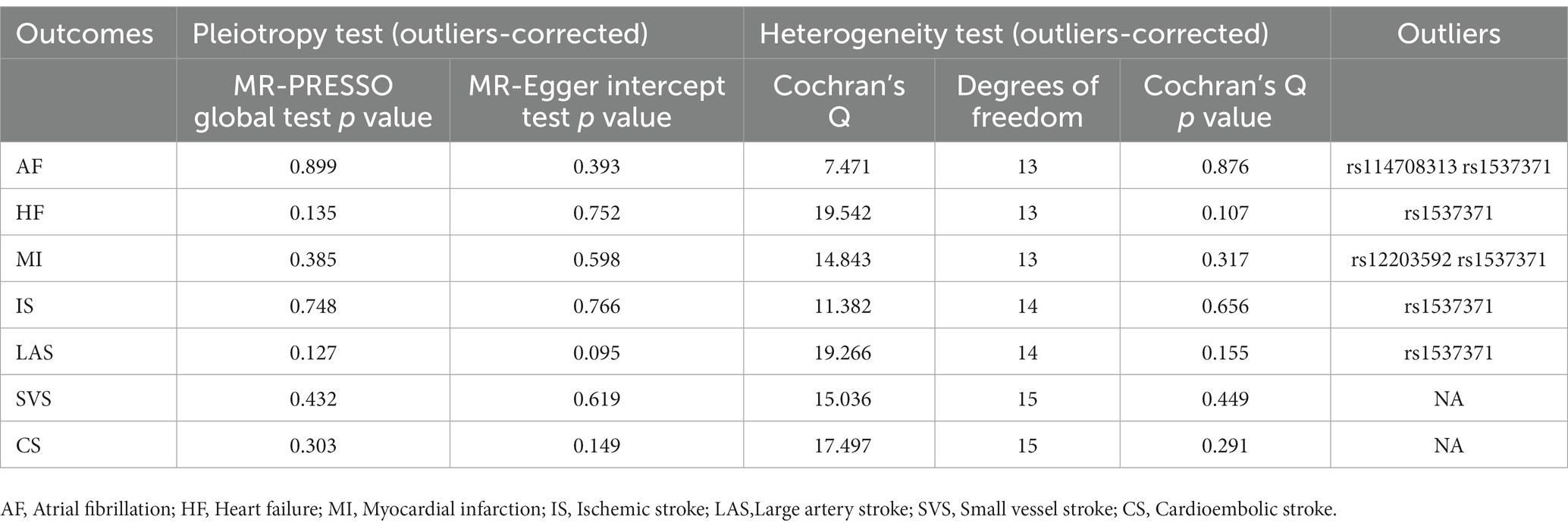
Table 2. Sensitive analyses for the Mendelian randomization analysis between PM 2.5 and cardiovascular diseases.
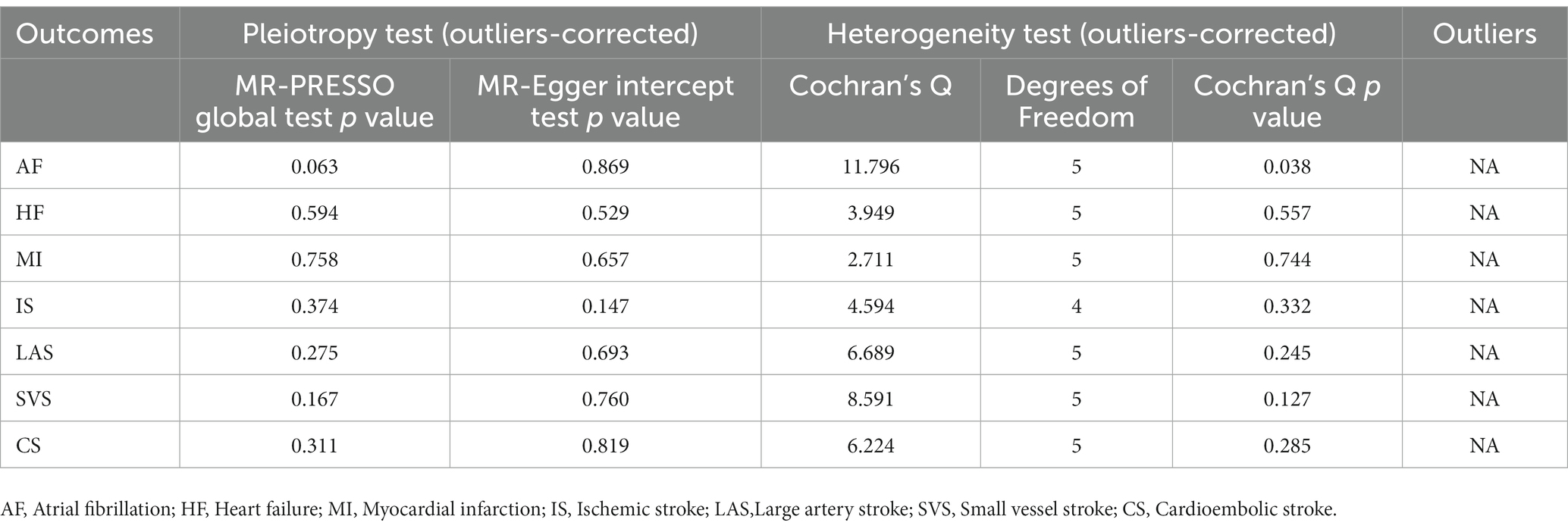
Table 3. Sensitive analyses for the Mendelian randomization analysis between PM 10 and cardiovascular diseases.
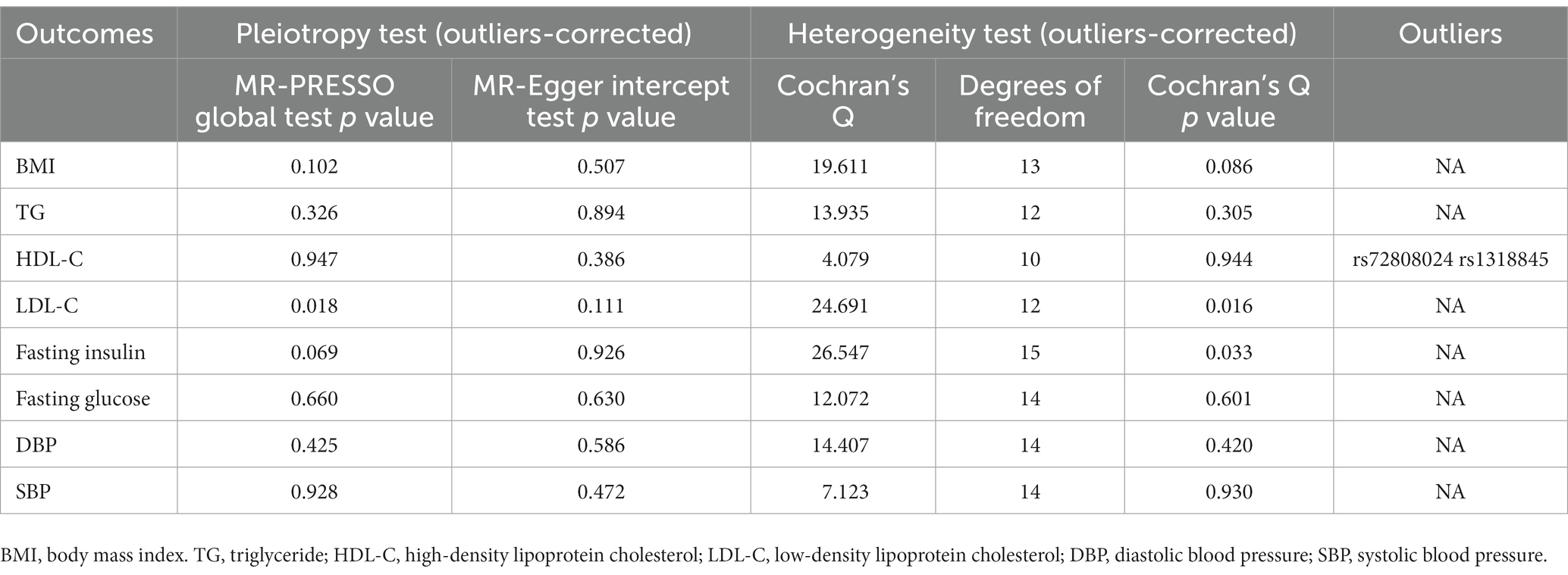
Table 4. Sensitive analyses for the Mendelian randomization analysis between PM 2.5 and cardiovascular biomarkers.
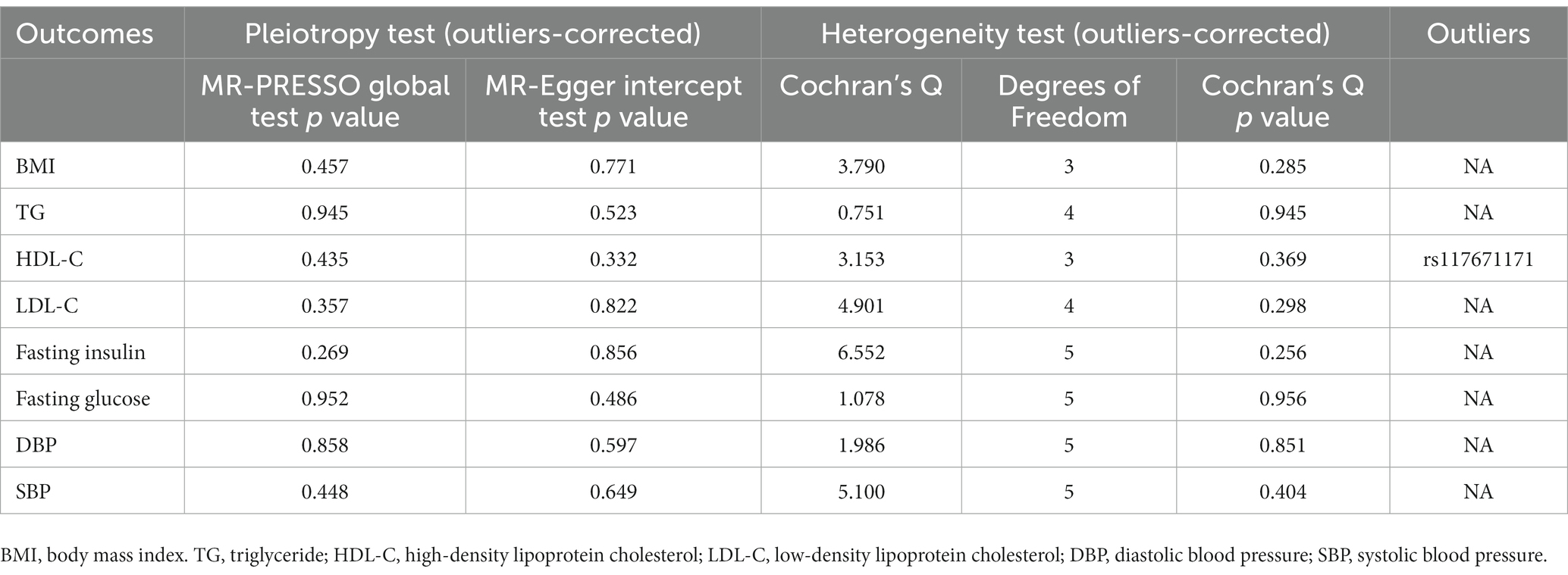
Table 5. Sensitive analyses for the Mendelian randomization analysis between PM 10 and cardiovascular biomarkers.
4. Discussion
In the present MR study, we examined the causality of PM on CVDs and cardiovascular biomarkers. Our findings showed PM2.5 was significantly related to a higher risk of MI and revealed a suggestive causal association between PM10 and the risk of HF and IS, which might be explained by deleterious effects on blood lipid levels.
With the increasing abundance of GWAS data, many scholars conducted MR analysis to infer causality between PM2.5 and diseases (42, 43). Zhang et al. identified 7 SNPs related to PM2.5 and conducted two sample MR methods to conclude that PM2.5 concentrations can increase the risk of hypothyroidism (43). Yang et al. used 85 SNPs as genetic variants for PM2.5 and demonstrated a causal relationship between PM2.5 and gestational diabetes mellitus (42). Our research is the first MR Study focusing on the causal effect of PM on CVDs and cardiovascular biomarkers. Previous analyses have shown that exposure to PM is associated with an increased risk of MI, IS, and HF (44). To identify whether PM2.5 contributes to a rise in the incidence of MI, Li et al. carried out a meta-analysis that comprised twenty-seven cohort studies involving 6,764,987 participants and 94,540 patients with MI. The analysis concluded that long-term exposure to PM2.5 plays a role in MI (45). A meta-analysis by Li et al., which included 27 cohort studies involving more than 6.5 million people, showed a positive association between exposure to PM2.5 and the risk of MI (45). Farhadi and his colleagues noted the severity of the relationship between PM2.5 and MI in a meta-analysis of 26 studies, confirming the notion that PM2.5 levels are a key factor in the development of MI hospitalization (15). Studies have confirmed that air pollutants can trigger oxidative stress and inflammation in the body through direct entry into the blood or indirectly through particles, metals, and other components in the substance, leading to vasoconstriction, endothelial dysfunction, platelet hyperresponsiveness, and even autonomic nervous system disorders, thus increasing the risk of CVDs (46–48).
A meta-analysis of 35 studies showed that exposure to PM pollution was associated with an increased risk of hospitalization or death from HF. For every 10 μg/m3 increase in exposure to PM10, the risk of hospitalization or death from HF increased by 1.6% (49). A study of more than 100,000 hospitalizations for HF from 26 major cities in China analyzed the association between various air pollutants and hospitalizations for HF. The concentration of PM10 increased by one quartile (76.9 μg/m3), the risk of hospitalization for HF increased by 1.3% (50). As Brook and his colleagues reported, PM has been linked with higher systemic blood pressure and vasoconstriction (51). Exposure to PM increases right ventricular and pulmonary diastolic filling pressures (52). Air pollution will dramatically raise the requirements based on the failing heart, possibly precipitating acute decompensation.
Our results concerning the potential causal relationship between PM10 and the risk of IS cohere with a systematic review that included 94 studies from 28 countries that reported that the short-run exposure concentration of PM10 increased by 10 μg/m3, the risk of hospitalization and death from stroke increased by 0.3%. On the other hand, Hossein et al. expressed that the level of PM was immediately connected with the number of stroke inpatients in the emergency room. Long-run changes in PM10 also increased the risk of IS (53). Research has speculated that increased blood pressure triggered by acute increases in pollutant concentrations can contribute to the development of IS (54, 55).
In terms of cardiovascular biomarkers, our study showed that PM2.5 concentration increases were significantly associated with increases in TG and decreases in HDL-C. The PM10 concentration increases were also closely related to the decreases in HDL-C. Information concerning the relationship between PM and changes in lipids and lipoproteins was disputable, especially regarding HDL-C (56–59). Multiple studies have demonstrated an inverse relationship between high concentrations of PM exposure and the level of HDL-C (60–62). Zhang et al. revealed that PM10 was negatively associated with HDL-C (63). A study conducted by Wang et al. showed that each increase in PM2.5 was associated with higher levels of TG and lower levels of HDL-C (64). A health study involving 33 communities concluded that per 10-μg/m3 increment in PM2.5 was significantly associated with 1.1% (95% CI: 0.4, 1.8%) increases in TG and 1.1% (95% CI: 0.8, 1.4%) decreases in HDL-C (65). Consistently, a longitudinal study performed in a multiethnic US cohort free of cardiovascular disease showed that higher exposure to PM2.5 was associated with lower HDL particle numbers or lower HDL-C levels (57). The precise mechanisms between PM exposures and lipid metabolism have not been fully determined. Several studies have shown that exposure to air pollution can lead to adverse lipid metabolism and lipid oxidation through systemic inflammation and oxidative stress (66–68). Other studies suggested that ambient air pollution might cause DNA methylation, leading to changes in particular genes associated with lipid metabolism (69, 70). Larger and more controlled studies are needed to fully address this issue.
4.1. Limitations and strengths
The advantages of our research are as follows. Our research is the first MR study focusing on the effect of PM on CVDs and cardiovascular biomarkers. MR estimates can avoid the interference of confounding factors and reveal the causal relationship between PM and CVDs more confidently. MR estimates can avoid the interference of confounding factors and reverse causality compared with other observational studies. However, this study also has some limitations. Firstly, the GWAS of exposure failed to distinguish the time of PM pollution, so our results cannot explain the time of exposure effect, which limited us from conducting a further analysis. Several studies have also proposed a difference between “long-term” and “short-term” outcomes. Short-term exposure to air pollution is thought to cause acute CVDs events by destabilizing susceptible plaques, although long-term exposure may increase the risk of atherosclerosis by accelerating dyslipidemia, hypertension, and other metabolic disturbances (71). The future GWAS study of PM needs to distinguish the duration of exposure. Secondly, when we selected IVs, we used a more lenient threshold (p < 5 × 10−7). Although this may boost statistical power, the more instrumental variables included in the study, the greater the possibility of producing more pleiotropy. In order to eliminate horizontal pleiotropy, we conducted MR sensitive analyses such as the MR-Egger intercept, MR-PRESSO, and leave-one-out method. However, it is very difficult to completely exclude directional pleiotropy because SNPs affect exposure and outcome through unknown pathways, which has decreased the reliability of the findings. At last, the GWAS selected in this study are from European populations, and whether the findings of this study are applicable to other populations remains to be determined. In particular, there are regional differences in PM pollution, especially in some developing countries, but Europe, the main body of this study, is mostly developed countries. Therefore, our results could not be easily generalized to populations in high-pollution areas. Finally, there was modest sample overlap between the GWAS of PM and the GWAS of AF, HF, MI, SBP, and DBP, which might result in bias. Nevertheless, the F-statistic was large enough to moderate the effect of potential bias by sample overlap (72). Moreover, if the genetic link with PM was solely evaluated in non-cases, this sample overlap would not result in bias.
4.2. Perspectives and future research
Several epidemiological and experimental studies have regarded all components of PM as associated risk factors for the occurrence and deterioration of cardiovascular disease, which is independent of other conventional risk factors, including smoking, obesity, and diabetes. Our findings demonstrated the different effects of PM10 and PM2.5 on CVDs and cardiovascular biomarkers. Despite many studies suggesting that smaller size fractions are more harmful, especially PM2.5 (73, 74), the risks to human health posed by coarse particles like PM10 cannot be ignored. It was reported that PM10 contained more lipopolysaccharide (LPS) than PM2.5, which could trigger inflammation by directly activating Toll-like receptors and induce metabolic syndromes (75–77). Furthermore, a study conducted by Chang et al. suggested that heart rate variability (HRV) changes are reliant on PM10 as opposed to smaller particles (78). It is urgent for us to conduct more research to identify the nature of PM in all its size fractions and investigate the molecular mechanisms underlying the effects of PM with different sizes on the CVDs. Randomized controlled trials on this topic should be conducted in the future, although this is highly challenging. Besides, a handful of studies have shown that higher levels of PM impair the function of HDL (60, 79, 80), which might affect the development of CVDs beyond possible changes in plasma HDL-C levels. Larger and more controlled studies are needed to investigate the mechanisms by which HDL functionality and levels are affected by PM exposure and explore the potential efficiency of preventative interventions like statins and antioxidant therapy. To protect the earth together, we hope that all countries will strengthen the effective control of pollution sources, adopt strict law enforcement and management measures, and pay attention to the efficient combination of policy and scientific and technological innovation.
5. Conclusion
At the genetic level, our study provides evidence supporting the causality of PM on CVDs and cardiovascular biomarkers. Regarding CVDs, PM2.5 concentration increases were significantly associated with a higher risk of MI. PM10 concentration increases were significantly associated with a higher risk of HF and IS. In terms of cardiovascular biomarkers, PM2.5 concentration increases were significantly correlated with increases in TG and decreases in HDL-C. The PM10 concentration increases were also closely correlated with the decreases in HDL-C. The underlying pathophysiological mechanisms need to be further studied. Therefore, more research should be carried out to explore the mechanism and prevention of PM exposure in CVDs to better contribute to people’s healthy lives.
Data availability statement
The original contributions presented in the study are included in the article/Supplementary material, further inquiries can be directed to the corresponding authors.
Author contributions
QW came up with the concept and designed the study and prepared the manuscript. ZW, MC, WM, and ZX processed the data. MX polished the draft. All authors contributed to the article and approved the submitted version.
Conflict of interest
The authors declare that the research was conducted in the absence of any commercial or financial relationships that could be construed as a potential conflict of interest.
Publisher’s note
All claims expressed in this article are solely those of the authors and do not necessarily represent those of their affiliated organizations, or those of the publisher, the editors and the reviewers. Any product that may be evaluated in this article, or claim that may be made by its manufacturer, is not guaranteed or endorsed by the publisher.
Supplementary material
The Supplementary material for this article can be found online at: https://www.frontiersin.org/articles/10.3389/fpubh.2023.1201479/full#supplementary-material
References
1. Roth, GA, Johnson, C, Abajobir, A, Abd-Allah, F, Abera, SF, Abyu, G, et al. Global, regional, and National Burden of cardiovascular diseases for 10 causes, 1990 to 2015. J Am Coll Cardiol. (2017) 70:1–25. doi: 10.1016/j.jacc.2017.04.052
2. Burnett, R, Chen, H, Szyszkowicz, M, Fann, N, Hubbell, B, Pope, CA 3rd, et al. Global estimates of mortality associated with long-term exposure to outdoor fine particulate matter. Proc Natl Acad Sci U S A. (2018) 115:9592–7. doi: 10.1073/pnas.1803222115
3. Miller, MR, Shaw, CA, and Langrish, JP. From particles to patients: oxidative stress and the cardiovascular effects of air pollution. Futur Cardiol. (2012) 8:577–602. doi: 10.2217/fca.12.43
4. Faraji Ghasemi, F, Dobaradaran, S, Saeedi, R, Nabipour, I, Nazmara, S, Abadi, DRV, et al. Levels and ecological and health risk assessment of PM(2.5)-bound heavy metals in the northern part of the Persian Gulf. Environ Sci Pollut Res Int. (2020) 27:5305–13. doi: 10.1007/s11356-019-07272-7
5. Goudarzi, G, Alavi, N, Geravandi, S, Idani, E, Behrooz, HRA, Babaei, AA, et al. Health risk assessment on human exposed to heavy metals in the ambient air PM(10) in Ahvaz, Southwest Iran. Int J Biometeorol. (2018) 62:1075–83. doi: 10.1007/s00484-018-1510-x
6. Abbasi-Kangevari, M, Malekpour, MR, Masinaei, M, Moghaddam, SS, Ghamari, SH, Abbasi-Kangevari, Z, et al. Effect of air pollution on disease burden, mortality, and life expectancy in North Africa and the Middle East: a systematic analysis for the global burden of disease study 2019. Lancet Planet Health. (2023) 7:e358–69. doi: 10.1016/S2542-5196(23)00053-0
7. Xing, YF, Xu, YH, Shi, MH, and Lian, YX. The impact of PM2.5 on the human respiratory system. J Thorac Dis. (2016) 8:E69–74. doi: 10.3978/j.issn.2072-1439.2016.01.19
8. Pope, CA 3rd, and Dockery, DW. Health effects of fine particulate air pollution: lines that connect. J Air Waste Manag Assoc. (2006) 56:709–42. doi: 10.1080/10473289.2006.10464485
9. Brook, RD, Franklin, B, Cascio, W, Hong, Y, Howard, G, Lipsett, M, et al. Air pollution and cardiovascular disease: a statement for healthcare professionals from the expert panel on population and prevention science of the American Heart Association. Circulation. (2004) 109:2655–71. doi: 10.1161/01.CIR.0000128587.30041.C8
10. Borsi, SH, Goudarzi, G, Sarizadeh, G, Dastoorpoor, M, Geravandi, S, Shahriyari, HA, et al. Health endpoint of exposure to criteria air pollutants in ambient air of on a populated in Ahvaz City, Iran. Front Public Health. (2022) 10:869656. doi: 10.3389/fpubh.2022.869656
11. Dastoorpoor, M, Sekhavatpour, Z, Masoumi, K, Mohammadi, MJ, Aghababaeian, H, Khanjani, N, et al. Air pollution and hospital admissions for cardiovascular diseases in Ahvaz, Iran. Sci Total Environ. (2019) 652:1318–30. doi: 10.1016/j.scitotenv.2018.10.285
12. Moradi, M, Mokhtari, A, Mohammadi, MJ, Hadei, M, and Vosoughi, M. Estimation of long-term and short-term health effects attributed to PM(2.5) standard pollutants in the air of Ardabil (using air Q + model). Environ Sci Pollut Res Int. (2022) 29:21508–16. doi: 10.1007/s11356-021-17303-x
13. Shao, Q, Liu, T, Korantzopoulos, P, Zhang, Z, Zhao, J, and Li, G. Association between air pollution and development of atrial fibrillation: a meta-analysis of observational studies. Heart Lung. (2016) 45:557–62. doi: 10.1016/j.hrtlng.2016.08.001
14. Yang, C, Chen, A, Chen, R, Qi, Y, Ye, J, Li, S, et al. Acute effect of ambient air pollution on heart failure in Guangzhou, China. Int J Cardiol. (2014) 177:436–41. doi: 10.1016/j.ijcard.2014.09.003
15. Farhadi, Z, Abulghasem Gorgi, H, Shabaninejad, H, Aghajani Delavar, M, and Torani, S. Association between PM2.5 and risk of hospitalization for myocardial infarction: a systematic review and a meta-analysis. BMC Public Health. (2020) 20:314. doi: 10.1186/s12889-020-8262-3
16. Yang, WS, Wang, X, Deng, Q, Fan, WY, and Wang, WY. An evidence-based appraisal of global association between air pollution and risk of stroke. Int J Cardiol. (2014) 175:307–13. doi: 10.1016/j.ijcard.2014.05.044
17. Pope, CA 3rd, Muhlestein, JB, May, HT, Renlund, DG, Anderson, JL, and Horne, BD. Ischemic heart disease events triggered by short-term exposure to fine particulate air pollution. Circulation. (2006) 114:2443–8. doi: 10.1161/CIRCULATIONAHA.106.636977
18. Cramer, J, Jørgensen, JT, Hoffmann, B, Loft, S, Bräuner, EV, Prescott, E, et al. Long-term exposure to air pollution and incidence of myocardial infarction: a Danish nurse cohort study. Environ Health Perspect. (2020) 128:57003. doi: 10.1289/EHP5818
19. O'Neill, MS, Diez-Roux, AV, Auchincloss, AH, Shen, M, Lima, JA, Polak, JF, et al. Long-term exposure to airborne particles and arterial stiffness: the multi-ethnic study of atherosclerosis (MESA). Environ Health Perspect. (2011) 119:844–51. doi: 10.1289/ehp.0901524
20. Barnett, AG, Williams, GM, Schwartz, J, Best, TL, Neller, AH, Petroeschevsky, AL, et al. The effects of air pollution on hospitalizations for cardiovascular disease in elderly people in Australian and New Zealand cities. Environ Health Perspect. (2006) 114:1018–23. doi: 10.1289/ehp.8674
21. Mills, NL, Robinson, SD, Fokkens, PH, Leseman, DL, Miller, MR, Anderson, D, et al. Exposure to concentrated ambient particles does not affect vascular function in patients with coronary heart disease. Environ Health Perspect. (2008) 116:709–15. doi: 10.1289/ehp.11016
22. Madaniyazi, L, Li, S, Li, S, and Guo, Y. Candidate gene expression in response to low-level air pollution. Environ Int. (2020) 140:105610. doi: 10.1016/j.envint.2020.105610
23. Zhou, Z, Qin, M, Khodahemmati, S, Li, W, Niu, B, Li, J, et al. Gene expression in human umbilical vein endothelial cells exposed to fine particulate matter: RNA sequencing analysis. Int J Environ Health Res. (2022) 32:2052–64. doi: 10.1080/09603123.2021.1935785
24. Jung, I, Park, M, Jeong, MH, Park, K, Kim, WH, and Kim, GY. Transcriptional analysis of gasoline engine exhaust particulate matter 2.5-exposed human umbilical vein endothelial cells reveals the different gene expression patterns related to the cardiovascular diseases. Biochem Biophys Rep. (2022) 29:101190. doi: 10.1016/j.bbrep.2021.101190
25. Yao, Y, Liu, L, Guo, G, Zeng, Y, and Ji, JS. Interaction of Sirtuin 1 (SIRT1) candidate longevity gene and particulate matter (PM2.5) on all-cause mortality: a longitudinal cohort study in China. Environ Health. (2021) 20:25. doi: 10.1186/s12940-021-00718-x
26. Poursafa, P, Kelishadi, R, Haghjooy-Javanmard, S, Rafiei, L, and Keramatian, K. Synergistic effects of genetic polymorphism and air pollution on markers of endothelial dysfunction in children. J Res Med Sci. (2012) 17:718–23.
27. Smith, GD, and Ebrahim, S. Mendelian randomization: prospects, potentials, and limitations. Int J Epidemiol. (2004) 33:30–42. doi: 10.1093/ije/dyh132
28. Lawlor, DA, Harbord, RM, Sterne, JA, Timpson, N, and Davey Smith, G. Mendelian randomization: using genes as instruments for making causal inferences in epidemiology. Stat Med. (2008) 27:1133–63. doi: 10.1002/sim.3034
29. Burgess, S, Scott, RA, Timpson, NJ, Davey Smith, G, and Thompson, SG. Using published data in Mendelian randomization: a blueprint for efficient identification of causal risk factors. Eur J Epidemiol. (2015) 30:543–52. doi: 10.1007/s10654-015-0011-z
30. Nielsen, JB, Thorolfsdottir, RB, Fritsche, LG, Zhou, W, Skov, MW, Graham, SE, et al. Biobank-driven genomic discovery yields new insight into atrial fibrillation biology. Nat Genet. (2018) 50:1234–9. doi: 10.1038/s41588-018-0171-3
31. Hartiala, JA, Han, Y, Jia, Q, Hilser, JR, Huang, P, Gukasyan, J, et al. Genome-wide analysis identifies novel susceptibility loci for myocardial infarction. Eur Heart J. (2021) 42:919–33. doi: 10.1093/eurheartj/ehaa1040
32. Shah, S, Henry, A, Roselli, C, Lin, H, Sveinbjörnsson, G, Fatemifar, G, et al. Genome-wide association and Mendelian randomisation analysis provide insights into the pathogenesis of heart failure. Nat Commun. (2020) 11:163. doi: 10.1038/s41467-019-13690-5
33. Malik, R, Chauhan, G, Traylor, M, Sargurupremraj, M, Okada, Y, Mishra, A, et al. Multiancestry genome-wide association study of 520,000 subjects identifies 32 loci associated with stroke and stroke subtypes. Nat Genet. (2018) 50:524–37. doi: 10.1038/s41588-018-0058-3
34. Wood, AR, Tyrrell, J, Beaumont, R, Jones, SE, Tuke, MA, Ruth, KS, et al. Variants in the FTO and CDKAL1 loci have recessive effects on risk of obesity and type 2 diabetes, respectively. Diabetologia. (2016) 59:1214–21. doi: 10.1007/s00125-016-3908-5
35. Chen, J, Spracklen, CN, Marenne, G, Varshney, A, Corbin, LJ, Luan, J, et al. The trans-ancestral genomic architecture of glycemic traits. Nat Genet. (2021) 53:840–60. doi: 10.1038/s41588-021-00852-9
36. Ong, JS, and MacGregor, S. Implementing MR-PRESSO and GCTA-GSMR for pleiotropy assessment in Mendelian randomization studies from a practitioner's perspective. Genet Epidemiol. (2019) 43:609–16. doi: 10.1002/gepi.22207
37. Auton, A, Brooks, LD, Durbin, RM, Garrison, EP, Kang, HM, Korbel, JO, et al. A global reference for human genetic variation. Nature. (2015) 526:68–74. doi: 10.1038/nature15393
38. Burgess, S, and Thompson, SG. Avoiding bias from weak instruments in Mendelian randomization studies. Int J Epidemiol. (2011) 40:755–64. doi: 10.1093/ije/dyr036
39. Greco, MF, Minelli, C, Sheehan, NA, and Thompson, JR. Detecting pleiotropy in Mendelian randomisation studies with summary data and a continuous outcome. Stat Med. (2015) 34:2926–40. doi: 10.1002/sim.6522
40. Bowden, J, Davey Smith, G, and Burgess, S. Mendelian randomization with invalid instruments: effect estimation and bias detection through egger regression. Int J Epidemiol. (2015) 44:512–25. doi: 10.1093/ije/dyv080
41. Verbanck, M, Chen, CY, Neale, B, and Do, R. Detection of widespread horizontal pleiotropy in causal relationships inferred from Mendelian randomization between complex traits and diseases. Nat Genet. (2018) 50:693–8. doi: 10.1038/s41588-018-0099-7
42. Yang, Y, Ma, X, Pang, W, and Jiang, C. Causal associations of PM2.5 and GDM: a two-sample Mendelian randomization study. Toxics. (2023) 11:171. doi: 10.3390/toxics11020171
43. Zhang, Y, Liu, S, Wang, Y, and Wang, Y. Causal relationship between particulate matter 2.5 and hypothyroidism: a two-sample Mendelian randomization study. Front. Public Health. (2022) 10:1000103. doi: 10.3389/fpubh.2022.1000103
44. Bourdrel, T, Bind, MA, Béjot, Y, Morel, O, and Argacha, JF. Cardiovascular effects of air pollution. Arch Cardiovasc Dis. (2017) 110:634–42. doi: 10.1016/j.acvd.2017.05.003
45. Zou, L, Zong, Q, Fu, W, Zhang, Z, Xu, H, Yan, S, et al. Long-term exposure to ambient air pollution and myocardial infarction: a systematic review and Meta-analysis. Front Med (Lausanne). (2021) 8:616355. doi: 10.3389/fmed.2021.616355
46. Fiordelisi, A, Piscitelli, P, Trimarco, B, Coscioni, E, Iaccarino, G, and Sorriento, D. The mechanisms of air pollution and particulate matter in cardiovascular diseases. Heart Fail Rev. (2017) 22:337–47. doi: 10.1007/s10741-017-9606-7
47. McGuinn, LA, Schneider, A, McGarrah, RW, Ward-Caviness, C, Neas, LM, Di, Q, et al. Association of long-term PM(2.5) exposure with traditional and novel lipid measures related to cardiovascular disease risk. Environ Int. (2019) 122:193–200. doi: 10.1016/j.envint.2018.11.001
48. Choi, YJ, Kim, SH, Kang, SH, Kim, SY, Kim, OJ, Yoon, CH, et al. Short-term effects of air pollution on blood pressure. Sci Rep. (2019) 9:20298. doi: 10.1038/s41598-019-56413-y
49. Shah, AS, Langrish, JP, Nair, H, McAllister, DA, Hunter, AL, Donaldson, K, et al. Global association of air pollution and heart failure: a systematic review and meta-analysis. Lancet. (2013) 382:1039–48. doi: 10.1016/S0140-6736(13)60898-3
50. Liu, H, Tian, Y, Song, J, Cao, Y, Xiang, X, Huang, C, et al. Effect of ambient air pollution on hospitalization for heart failure in 26 of China's largest cities. Am J Cardiol. (2018) 121:628–33. doi: 10.1016/j.amjcard.2017.11.039
51. Brook, RD, Brook, JR, Urch, B, Vincent, R, Rajagopalan, S, and Silverman, F. Inhalation of fine particulate air pollution and ozone causes acute arterial vasoconstriction in healthy adults. Circulation. (2002) 105:1534–6. doi: 10.1161/01.CIR.0000013838.94747.64
52. Rich, DQ, Freudenberger, RS, Ohman-Strickland, P, Cho, Y, and Kipen, HM. Right heart pressure increases after acute increases in ambient particulate concentration. Environ Health Perspect. (2008) 116:1167–71. doi: 10.1289/ehp.11230
53. Alimohammadi, H, Fakhri, S, Derakhshanfar, H, Hosseini-Zijoud, SM, Safari, S, and Hatamabadi, HR. The effects of air pollution on ischemic stroke admission rate. Chonnam Med J. (2016) 52:53–8. doi: 10.4068/cmj.2016.52.1.53
54. Brook, RD, Newby, DE, and Rajagopalan, S. Air pollution and Cardiometabolic disease: an update and call for clinical trials. Am J Hypertens. (2017) 31:1–10. doi: 10.1093/ajh/hpx109
55. Giorgini, P, Di Giosia, P, Grassi, D, Rubenfire, M, Brook, RD, and Ferri, C. Air pollution exposure and blood pressure: an updated review of the literature. Curr Pharm Des. (2016) 22:28–51. doi: 10.2174/1381612822666151109111712
56. Wu, XM, Broadwin, R, Basu, R, Malig, B, Ebisu, K, Gold, EB, et al. Associations between fine particulate matter and changes in lipids/lipoproteins among midlife women. Sci Total Environ. (2019) 654:1179–86. doi: 10.1016/j.scitotenv.2018.11.149
57. Bell, G, Mora, S, Greenland, P, Tsai, M, Gill, E, and Kaufman, JD. Association of air Pollution Exposures with High-Density Lipoprotein Cholesterol and Particle Number: the multi-ethnic study of atherosclerosis. Arterioscler Thromb Vasc Biol. (2017) 37:976–82. doi: 10.1161/ATVBAHA.116.308193
58. Fioravanti, S, Cesaroni, G, Badaloni, C, Michelozzi, P, Forastiere, F, and Porta, D. Traffic-related air pollution and childhood obesity in an Italian birth cohort. Environ Res. (2018) 160:479–86. doi: 10.1016/j.envres.2017.10.003
59. Gui, ZH, Yang, BY, Zou, ZY, Ma, J, Jing, J, Wang, HJ, et al. Exposure to ambient air pollution and blood lipids in children and adolescents: a national population based study in China. Environ Pollut. (2020) 266:115422. doi: 10.1016/j.envpol.2020.115422
60. Li, J, Zhou, C, Xu, H, Brook, RD, Liu, S, Yi, T, et al. Ambient air pollution is associated with HDL (high-density lipoprotein) dysfunction in healthy adults. Arterioscler Thromb Vasc Biol. (2019) 39:513–22. doi: 10.1161/ATVBAHA.118.311749
61. Li, R, Navab, M, Pakbin, P, Ning, Z, Navab, K, Hough, G, et al. Ambient ultrafine particles alter lipid metabolism and HDL anti-oxidant capacity in LDLR-null mice. J Lipid Res. (2013) 54:1608–15. doi: 10.1194/jlr.M035014
62. Chuang, KJ, Yan, YH, Chiu, SY, and Cheng, TJ. Long-term air pollution exposure and risk factors for cardiovascular diseases among the elderly in Taiwan. Occup Environ Med. (2011) 68:64–8. doi: 10.1136/oem.2009.052704
63. Zhang, K, Wang, H, He, W, Chen, G, Lu, P, Xu, R, et al. The association between ambient air pollution and blood lipids: a longitudinal study in Shijiazhuang, China. Sci Total Environ. (2021) 752:141648. doi: 10.1016/j.scitotenv.2020.141648
64. Wang, L, Chen, G, Pan, Y, Xia, J, Chen, L, Zhang, X, et al. China multi-ethnic cohort collaborative, association of long-term exposure to ambient air pollutants with blood lipids in Chinese adults: the China multi-ethnic cohort study. Environ Res. (2021) 197:111174. doi: 10.1016/j.envres.2021.111174
65. Yang, BY, Bloom, MS, Markevych, I, Qian, ZM, Vaughn, MG, Cummings-Vaughn, LA, et al. Exposure to ambient air pollution and blood lipids in adults: the 33 communities Chinese health study. Environ Int. (2018) 119:485–92. doi: 10.1016/j.envint.2018.07.016
66. Lodovici, M, and Bigagli, E. Oxidative stress and air pollution exposure. J Toxicol. (2011) 2011:487074. doi: 10.1155/2011/487074
67. Shanley, RP, Hayes, RB, Cromar, KR, Ito, K, Gordon, T, and Ahn, J. Particulate air pollution and clinical cardiovascular disease risk factors. Epidemiology. (2016) 27:291–8. doi: 10.1097/EDE.0000000000000426
68. Chen, T, Jia, G, Wei, Y, and Li, J. Beijing ambient particle exposure accelerates atherosclerosis in ApoE knockout mice. Toxicol Lett. (2013) 223:146–53. doi: 10.1016/j.toxlet.2013.09.004
69. Bind, MA, Lepeule, J, Zanobetti, A, Gasparrini, A, Baccarelli, A, Coull, BA, et al. Air pollution and gene-specific methylation in the normative aging study: association, effect modification, and mediation analysis. Epigenetics. (2014) 9:448–58. doi: 10.4161/epi.27584
70. Chen, R, Meng, X, Zhao, A, Wang, C, Yang, C, Li, H, et al. DNA hypomethylation and its mediation in the effects of fine particulate air pollution on cardiovascular biomarkers: a randomized crossover trial. Environ Int. (2016) 94:614–9. doi: 10.1016/j.envint.2016.06.026
71. Bevan, GH, Al-Kindi, SG, Brook, R, and Rajagopalan, S. Ambient air pollution and atherosclerosis: recent updates. Curr Atheroscler Rep. (2021) 23:63. doi: 10.1007/s11883-021-00958-9
72. Burgess, S, Davies, NM, and Thompson, SG. Bias due to participant overlap in two-sample Mendelian randomization. Genet Epidemiol. (2016) 40:597–608. doi: 10.1002/gepi.21998
73. Churg, A, and Brauer, M. Ambient atmospheric particles in the airways of human lungs. Ultrastruct Pathol. (2000) 24:353–61.
74. Churg, A, and Brauer, M. Human lung parenchyma retains PM2.5. Am J Respir Crit Care Med. (1997) 155:2109–11. doi: 10.1164/ajrccm.155.6.9196123
75. Cani, PD, Amar, J, Iglesias, MA, Poggi, M, Knauf, C, Bastelica, D, et al. Metabolic endotoxemia initiates obesity and insulin resistance. Diabetes. (2007) 56:1761–72. doi: 10.2337/db06-1491
76. Liu, C, Ying, Z, Harkema, J, Sun, Q, and Rajagopalan, S. Epidemiological and experimental links between air pollution and type 2 diabetes. Toxicol Pathol. (2013) 41:361–73. doi: 10.1177/0192623312464531
77. Fonceca, AM, Zosky, GR, Bozanich, EM, Sutanto, EN, Kicic, A, McNamara, PS, et al. Accumulation mode particles and LPS exposure induce TLR-4 dependent and independent inflammatory responses in the lung. Respir Res. (2018) 19:15. doi: 10.1186/s12931-017-0701-z
78. Chang, LT, Tang, CS, Pan, YZ, and Chan, CC. Association of heart rate variability of the elderly with personal exposure to PM 1, PM 1-2.5, and PM 2.5-10. Bull Environ Contam Toxicol. (2007) 79:552–6. doi: 10.1007/s00128-007-9233-4
79. Mathew, AV, Yu, J, Guo, Y, Byun, J, Chen, YE, Wang, L, et al. Effect of ambient fine particulate matter air pollution and colder outdoor temperatures on high-density lipoprotein function. Am J Cardiol. (2018) 122:565–70. doi: 10.1016/j.amjcard.2018.04.061
Keywords: particulate matter, cardiovascular diseases, cardiovascular biomarkers, Mendelian randomization, causal association
Citation: Wang Q, Wang Z, Chen M, Mu W, Xu Z and Xue M (2023) Causality of particulate matter on cardiovascular diseases and cardiovascular biomarkers. Front. Public Health. 11:1201479. doi: 10.3389/fpubh.2023.1201479
Edited by:
Barbara Piekarska, Medical University of Warsaw, PolandReviewed by:
Mohammad Javad Mohammadi, Ahvaz Jundishapur University of Medical Sciences, IranJing Han, Jinan municipal center for disease control and prevention, China
Copyright © 2023 Wang, Wang, Chen, Mu, Xu and Xue. This is an open-access article distributed under the terms of the Creative Commons Attribution License (CC BY). The use, distribution or reproduction in other forums is permitted, provided the original author(s) and the copyright owner(s) are credited and that the original publication in this journal is cited, in accordance with accepted academic practice. No use, distribution or reproduction is permitted which does not comply with these terms.
*Correspondence: Mei Xue, xuemei2575@126.com; Zhenxing Xu, wfxzhx@163.com