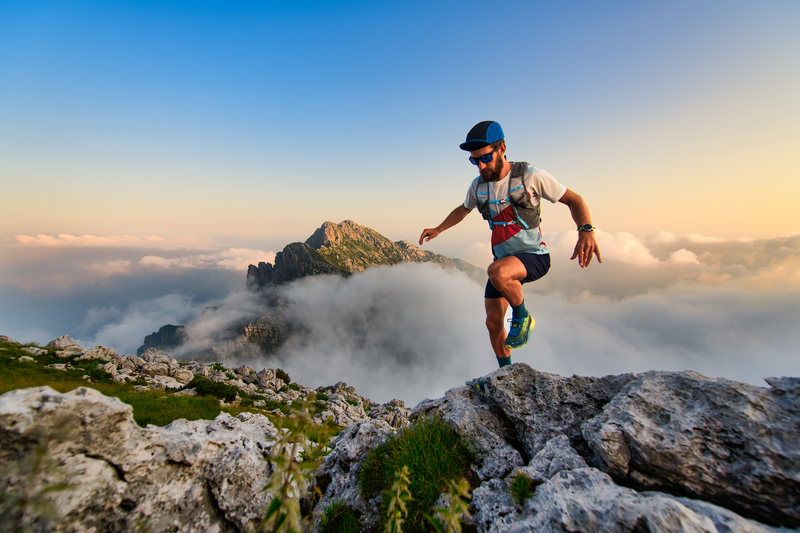
95% of researchers rate our articles as excellent or good
Learn more about the work of our research integrity team to safeguard the quality of each article we publish.
Find out more
ORIGINAL RESEARCH article
Front. Public Health , 02 June 2023
Sec. Public Health Policy
Volume 11 - 2023 | https://doi.org/10.3389/fpubh.2023.1193428
Introduction: Multimorbidity, defined as the coexistence of two or more chronic diseases in the same individual, represents a significant health challenge. However, there is limited evidence on its prevalence and associated factors in developing countries, such as Brazil, especially stratified by sex. Thus, this study aims to estimate the prevalence and analyze the factors associated with multimorbidity in Brazilian adults according to sex.
Methods: Cross-sectional population-based household survey carried out with Brazilian adults aged 18 years or older. The sampling strategy consisted of a three-stage conglomerate plan. The three stages were performed through simple random sampling. Data were collected through individual interviews. Multimorbidity was classified based on a list of 14 self-reported chronic diseases/conditions. Poisson regression analysis was performed to estimate the magnitude of the association between sociodemographic and lifestyle factors with the prevalence of multimorbidity stratified by sex.
Results: A total of 88,531 individuals were included. In absolute terms, the prevalence of multimorbidity was 29.4%. The frequency in men and women was 22.7 and 35.4%, respectively. Overall, multimorbidity was more prevalent among women, the older people, residents of the South and Southeast regions, urban area residents, former smokers, current smokers, physically inactive, overweight, and obese adults. Individuals with complete high school/incomplete higher education had a lower prevalence of multimorbidity than those with higher educational level. The associations between education and multimorbidity differed between sexes. In men, multimorbidity was inversely associated with the strata of complete middle school/incomplete high school and complete high school/incomplete higher education, while in women, the association between these variables was not observed. Physical inactivity was positively associated with a higher prevalence of multimorbidity only in men. An inverse association was verified between the recommended fruit and vegetable consumption and multimorbidity for the total sample and both sexes.
Conclusion: One in four adults had multimorbidity. Prevalence increased with increasing age, among women, and was associated with some lifestyles. Multimorbidity was significantly associated with educational level and physical inactivity only in men. The results suggest the need to adopt integrated strategies to reduce the magnitude of multimorbidity, specific by gender, including actions for health promotion, disease prevention, health surveillance and comprehensive health care in Brazil.
Chronic noncommunicable diseases (NCDs) are one of the major challenges faced by governments and global health systems. These diseases account for approximately 71% of global mortality, especially cardiovascular diseases, cancers, chronic respiratory diseases, and diabetes (1). NCDs are responsible for 41 million deaths annually, most in low- and middle-income countries (1).
Multimorbidity is the coexistence of two or more chronic diseases/conditions in an individual (2); however, some studies have used other definitions, such as ≥3, ≥4, and ≥ 5 (3). The most common chronic diseases used to define multimorbidity are cardiovascular diseases, hypertension, diabetes, and dyslipidemias (3). Previous systematic reviews identified the prevalence of multimorbidity from 33.1 to 43.0% (3–5). A meta-analysis found a pooled multimorbidity prevalence of 42.4% in adults (3). Multimorbid individuals have a higher probability of hospitalization, disability, and death than those with a single chronic disease/condition (6, 7). Multimorbidity is also related to declining physical function (8), worse quality of life (9), and higher socioeconomic costs for countries, individuals, and families (10).
In Brazil, multimorbidity is a serious challenge for public health systems. A recent systematic review reported a substantial variation in the estimated prevalence of multimorbidity from 16.8 to 93.4% (5). National-level assessment using data from the Brazil National Household Sample Survey and the National Health Survey (NHS) showed an increase in multimorbidity prevalence from 17.1 to 21.0% between 1998 and 2013 (8).
Multiple factors are associated with multimorbidity. Systematic reviews have shown a higher prevalence in older populations, women (3), and people with lower socioeconomic status and education (11). Several NCD risk factors, including cigarette smoking, alcohol use, decreased physical activity, and unhealthy diet, are associated with various diseases used to calculate multimorbidity (12). Prior studies have reported a positive association between multimorbidity and smoking (current or former smoker) and physical inactivity (13–15). However, healthy eating indicators are controversial in the literature. Data show the association of protection (16), risk (17, 18), and no association (19, 20). Thus, it remains unclear which risk factors most influence multimorbidity.
Despite the existing literature, the epidemiology and factors associated with multimorbidity are still poorly understood, especially in developing countries like Brazil (3). Furthermore, few studies have explored the prevalence according to sociodemographic and lifestyle variables. Many also do not include potential interactions in regression models, such as between race/skin color, education, and geographic region. Investigations of associated factors stratified by sex are also limited. Studies indicate that factors related to multimorbidity may differ between sexes (11, 21).
Most previous studies have found a higher prevalence of multimorbidity among women (4, 19, 21, 22), but not all studies have reported this (23–25). In almost all countries of the world, women have a higher life expectancy than men (26), they are more often affected by a series of non-fatal chronic diseases that negatively affect their quality of life and daily physical capacity (27). Thus, studies on multimorbidity require greater knowledge about its prevalence, the diseases involved and their relationship with possible gender differences. In Brazil, the prevalence of multimorbidity by gender has not been well documented in large populations.
Identifying multimorbidity risk factors stratified by sex is essential to effectively establish specific strategies for disease prevention, health promotion, and integrated care, particularly in a scenario of increased demand for health services due to population aging (28). The analysis of multimorbidity patterns can contribute to the planning of interventions to achieve Sustainable Development Goal 3 (Health and Wellbeing) and monitoring the Global Action Plan for the Prevention and Control of NCDs (29) and Strategic Plan for Tackle Chronic Diseases and Noncommunicable Diseases of the Brazilian Ministry of Health (30). Thus, this study aims to estimate the prevalence and analyze the factors associated with multimorbidity in Brazilian adults according to sex.
Cross-sectional population-based study using data from the 2019 National Health Survey (NHS). The NHS is a household-based nationwide survey conducted by the Ministry of Health in partnership with the Oswaldo Cruz Foundation and the Brazilian Institute of Geography and Statistics (IBGE). One of the core objectives of the NHS is to outline the health situation and lifestyle behaviors of the Brazilian population, including the assessment of NCD patterns. The NHS is representative of urban and rural areas, large regions of Brazil, federative units, state capitals, and metropolitan regions (31).
The 2019 NHS was carried out in the 27 federative units of Brazil distributed in five macro-regions (32). In 2019, the country had 5,570 municipalities and a total population of 210,147,125 inhabitants with a Human Development Index of 0.765, Gross Domestic Product (GDP) per capita of BRL 35,161.70, and household income per capita of BRL 1,439 (33).
The target population of the NHS consisted of individuals aged 15 years and older residing in permanent private households, which are built for the sole purpose of housing. Households located in special census tracts or sparsely populated areas were excluded from the sample such as indigenous villages, barracks, military bases, lodgings, campsites, prison units, long-stay institutions for the older people, comprehensive care facilities for children and adolescents, convents, hospitals, settlement project farming and quilombola communities (31). In the present study, participants aged 18 years and older were included.
The sampling strategy consisted of a three-stage conglomerate plan: (i) selection of Primary Sampling Units (PSU) constituted by census tracts, defined as the territorial unit formed by a continuous area located in a single urban or rural setting, with a defined dimension and number of households from the Geographic Operational Base of the 2010 Demographic Census of Brazil; (ii) selection of Secondary Sampling Units including households in each PSU, and; (iii) selection of Tertiary Sampling Units including individuals aged 15 or over who answered the research questionnaire. All individuals drawn from permanent private households were included. The three stages were performed through simple random sampling (31).
The sample size defined for the 2019 NHS considered the desired accuracy for estimating the main risk and protective factors for NCDs, disease prevalence, and access to health services, among other variables. The average values and variances of the indicators were included, in addition to a 20% non-response rate. The sample was estimated at 108,525 households, and data were collected from 94,114 households, with a 6.4% non-response rate (31). In this study, the sample consisted of 88,531 adults.
Data collection occurred during household visits from August 2019 to March 2020. The questionnaire used was validated by specialists and applied by trained professionals using mobile data collection devices for the interviews (31).
The dependent variable was multimorbidity, defined as the coexistence of ≥2 chronic diseases/conditions in an individual (2) using a list that includes one chronic condition and 13 self-reported chronic diseases: chronic back problem, hypertension, hypercholesterolemia, diabetes, arthritis or rheumatism, asthma or asthmatic bronchitis, work-related musculoskeletal disorders (WMSDs), cancer, cardiovascular diseases, stroke, chronic kidney failure, and chronic lung disease (such as pulmonary emphysema, chronic bronchitis or Chronic Obstructive Pulmonary Disease—COPD), depression and mental disorder. In order to assess people’s self-reports of chronic illnesses and conditions, participants answered a series of questions (Supplementary Table S1).
The independent variables included were: sociodemographics [sex, age group (34), self-declared race/skin color (35), education (36), living with a spouse/partner, geographic region (37), and area of residence (37)]; lifestyle [tobacco use (38), alcohol abuse (39), leisure-time physical activity (40), recommended consumption of fruits and vegetables (41), ultra-processed food consumption (41), regular consumption of soft drinks or artificial juices (41), and nutritional status (42)]. Questions, answer options, operational definitions and, analysis categories are in Supplementary Table S2.
The data collected using mobile was stored in a database. The participant’s identification data has been deleted and replaced by a unique code. Data quality, such as the number of missing data, was checked. All variables were treated and categorized if necessary. In addition, sample weights were calculated.
Statistical analysis was conducted using the STATA software (StataCorp LLC, version 15.0, College Station, TX, EUA). First, a descriptive analysis of the variables stratified by sex was performed, using relative frequencies (%) and their respective 95% confidence intervals (95% CI). Then, the prevalence of each chronic disease/condition by sex and age group was determined. Statistical differences between groups were verified using the chi-square test adjusted by the study design.
Subsequently, bivariate Poisson regression was used to predict the association between each independent variable and multimorbidity. The final models were reported as unadjusted Prevalence Ratios (PR) and respective 95% CI. Sociodemographic and lifestyle variables with value of p < 0.20 in the bivariate analysis were included in the multiple regression model. We also incorporated interaction terms between the following variables: age group versus geographic region; education versus self-reported race/skin color; geographic region versus self-reported race/skin color; education versus geographic region; and ultra-processed food consumption versus recommended fruit and vegetable consumption. These factors were included based on statistical significance among all interactions and/or the theory of potential interactions described in the literature. The results of this analysis were reported as adjusted PR (APR) and respective 95% CI. Analyzes were performed for the total sample and stratified by sex. p-values < 0.05 were considered statistically significant.
The NHS was approved by the Research Ethics Committee of the National Health Council under protocol number 3,529,376/2019. Written consent was obtained from all participants.
A total of 88,531 participants were included. The mean age was 44.9 years (95% CI: 44.7–45.2, minimum 18 years and maximum 102 years), and most were women (53.2, 95% CI: 52.6–53.8).
According to Table 1, the majority were aged between 40 and 59 years old (35.3%), brown (43.8%), had completed high school or incomplete higher education (34.9%), reported living with a spouse (61.4%), in the Southeast region (43.4%), urban area (86.2%), and did not live in capitals or metropolitan regions (58.4%). Regarding lifestyle-related risk factors, 26.6% were former smokers, 17.1% reported binge drinking, most were physically inactive (69.9%), 13.0% regularly consumed fruits and vegetables, 14.3% consumed five or more ultra-processed food groups, and 32.9% regularly drink soda or artificial juice. Significant differences between men and women were observed for most variables, including those related to lifestyle.
The average number of diseases in the study population was 1.13 (standard deviation = 0.01; 95% CI: 1.11–1.15; minimum 0 and maximum 12) and was lower in men (0.88, standard deviation = 0.01; 95% CI: 0.86–0.90; minimum 0 and maximum 10) compared to women (1.34, standard deviation = 1.34; 95% CI: 1.31–1.37; minimum 0 and maximum 12) (p < 0.001).
Multimorbidity prevalence was 29.4% (95% CI: 28.9–30.0), being higher among women (35.4%; 95% CI: 34.6–36.2) compared to men (22.7%; 95% CI: 21.9–23.4) (p < 0.001). Prevalence rates of multimorbidity also increased substantially with age for the total sample and both sexes (p < 0.001). Compared to 8.3% (95% CI: 7.3–9.4) of people aged 18–24 years, the prevalence of multimorbidity in the 40–59 age group (34.1%; 95% CI: 33.2–35.1) and ≥ 60 years (56.5%; 95% CI: 55.4–57.6) increased significantly. Regardless of sex, the most reported morbidities were hypertension (23.9%), chronic back problems (21.6%), and hypercholesterolemia (14.6%; Table 2).
Table 2. Prevalence of morbidities and multimorbidity in the study population, according to sex and age group.
The supplementary tables display the results of the bivariate Poisson regression analysis in the total sample (Supplementary Table S3), men (Supplementary Table S4), and women (Supplementary Table S5).
After adjusting the multiple regression model, a positive gradient in the multimorbidity prevalence with age was observed in the study population, with the greatest magnitude for the older people (APR: 6.25; 95% CI: 4.75–8.23). The prevalence of multimorbidity was higher among women (APR: 1.47, 95% CI: 1.42–1.53), residents of the South (APR: 1.99, 95% CI: 1.34–2.96) and Southeast (APR: 1.58, 95% CI: 1.07–2.33) regions of Brazil, urban residents (APR: 1.10, 95% CI: 1.06–1.15), former smokers (APR: 1.24, 95% CI: 1.20–1.29), current smokers (APR: 1.09, 95% CI: 1.04–1.16), physically inactive (APR: 1.07, 95% CI: 1.03–1.11), overweight (APR: 1.24, 95% CI: 1.20–1.29), and obese (APR: 1.52, 95% CI: 1.46–1.59) individuals. In turn, a lower prevalence was found for participants with higher educational level (complete high school/incomplete higher education; p = 0.002) and for people who reported insufficient fruit and vegetable consumption (p = 0.003) (Table 3). The results of the interaction terms exhibited significant interactions between age group/geographic region, education/self-declared race/skin color, and geographic region/self-declared race/skin color (Supplementary Table S4).
Table 3. Multiple regression analysis of factors associated with multimorbidity in the total sample.
Similar associations were found between men (Table 4) and women (Table 5). However, we observed that some associations differed between sexes, where people aged 25–39 years (p < 0.001), residents of the South (p < 0.001) and Midwest (p = 0.039) regions of Brazil were associated with a higher prevalence of multimorbidity only in women. Physical inactivity was positively associated with a higher prevalence of multimorbidity only in men (p = 0.001). The educational levels complete middle school/incomplete high school (p = 0.031) and complete high school/incomplete higher education (p < 0.001) were associated with a lower prevalence of multimorbidity only in men. The results of the interaction terms in men showed significant interactions between education/self-reported race/skin color and geographic region/self-reported race/skin color (Supplementary Table S5). In women, significant interactions were observed between age group/geographic region, education/self-reported race/skin color, geographic region/self-reported race/skin color, and ultra-processed food consumption/recommended fruit and vegetable consumption (Supplementary Table S6).
Using a nationally representative sample, we found that multimorbidity was prevalent in the Brazilian adult population. Alarmingly, one in four Brazilian adults had multimorbidity. Overall, multimorbidity was more prevalent among women, the older people, residents of the South and Southeast regions, urban area residents, former smokers, current smokers, physically inactive, overweight, and obese individuals. In turn, individuals with complete high school/incomplete higher education showed a lower prevalence of multimorbidity than those with higher education. The associations between education and multimorbidity differed between sexes. In men, multimorbidity was inversely associated with the strata of complete middle school/incomplete high school and complete high school/incomplete higher education, while in women, the association was not significant. The South and Midwest regions were associated with a higher prevalence in women, while leisure-time physical inactivity remained associated only in men. The recommended fruit and vegetable consumption was inversely related to multimorbidity for the total sample and both sexes.
The prevalence found in this study was similar to that estimated in low- and middle-income countries (29.7%), according to a meta-analysis involving 1,180,111 individuals from 57 countries (4). The prevalence ratio of multimorbidity is higher in high-income countries compared to low- and middle-income countries, possibly due to differences in diagnostic and data management systems between countries (4).
The results were also higher than those found in the Brazilian adult population in 2013 (23.6%), indicating an increase in multimorbidity burden in Brazil (43). This increment was reported in different countries (44, 45). The rise in multimorbidity is related to demographic, nutritional, and epidemiological transition, besides other determinants such as urbanization (46).
Regardless of sex, we observed increasing multimorbidity with age. This association is well described in the literature (3, 4). Different mechanisms may explain this relationship. First, due to the demographic transition that is responsible for the increase in the number of older people (47). Second, changes inherent to aging predispose to the development of chronic diseases/conditions (48). Several physiological changes, for example, are associated with senescence, such as increased body fat, especially in women, which often leads to a higher prevalence of overweight and obesity; decrease in fat-free mass and reduced capacity of the glucose uptake system, contributing to insulin resistance and diabetes; and reduced cardiac output, which can lead to increased cardiovascular disease risk (49). Other mechanisms have also been associated with a higher incidence of chronic diseases/conditions (49). Third, owing to the accumulation of risk factors. Longevity is related to longer exposure to these covariates, such as smoking status, unhealthy diet, alcohol consumption, and physical inactivity (50). Therefore, as the older people population grows, an increasing prevalence of multimorbidity is expected, with a potential overload of health care systems (12). Despite this, our results indicated that multimorbidity is not restricted to elderly. Indeed, younger people showed a high prevalence, consistent with previous observations (19, 23, 43).
Women had a higher prevalence of multimorbidity than men, corroborating other studies (4, 16, 51). One of the most consistent explanations for this result is a possible residual confounding due to higher consultation rates in women, leading to higher rates of chronic disease/condition diagnosis, regardless of age (52). In fact, in this investigation, women had a higher prevalence of multimorbidity than men in all age strata. Another explanation would be related to survival bias, as men have a lower life expectancy (53). Thus, the burden of chronic diseases/conditions in women tends to increase.
The lowest prevalence of multimorbidity was observed among participants with complete high school/incomplete higher education compared to people with higher education in the study population, which is in agreement with previous findings (54–56). A meta-analysis reported that low education level was associated with a 64% increased odds of multimorbidity (11). Considering only low- and middle-income countries, such as Brazil, earlier studies have shown inconsistencies, with a positive association (19, 57, 58), negative association (32, 59), or no significant difference between education and multimorbidity (60). Behavioral aspects are probably related to this result. The prevalence of risk factors differs by educational level; smoking, unhealthy eating habits, and obesity are more frequent among less educated individuals. In turn, leisure-time physical activity and the recommended fruit and vegetable consumption are higher among people with higher education (34). For example, a study carried out with Chinese residents showed that participants with a high level of education had positive knowledge, attitudes and behaviors related to diet that contributed to the prevention of hypertension (61).
Our findings suggest different associations between education and multimorbidity by sex. Intermediate educational levels (complete middle school/incomplete high school and complete high school/incomplete higher education) were a protective factor for multimorbidity among men, while this association was not verified among women. Previous investigations demonstrated relevant similarities (55, 62). However, a systematic review reported that most works found associations for sex-matched samples, thereby limiting the scope of evidence stratified by sex (11). The relationship between education and multimorbidity by sex can be explained, besides other factors, by differences in the magnitude between low education and the most prevalent chronic diseases in the study (55). For example, additional analysis showed that the strength of the association between low education (no education/incomplete middle education) and diseases, such as diabetes (PRwomen: 4.22 versus PRmen: 1.69), hypertension (PRwomen: 2.65 versus PRmen: 1.41), and high cholesterol (PRwomen: 1.73 versus PRmen: 0.77), is higher in women compared to men (data not shown in the results).
We found a higher prevalence of multimorbidity among urban residents compared to rural residents, regardless of sex, which is in accordance with previous reports (51, 63, 64). People in urban areas have different behavior patterns and lifestyles than those in rural areas. Urban residents, for example, have a higher consumption of energy-dense foods, rich in sugar, trans fats, and sodium (65), as they are more exposed to ultra-processed foods and marketing strategies of food companies (66). These ultra-processed foods require less preparation time, hence meeting the needs of urban populations who work outside the home and may have limited time or resources to cook (67). Furthermore, scientific literature reports that urban residents spend less time on moderate and vigorous-intensity physical activity, and consume more alcohol, increasing the likelihood of developing multiple chronic diseases/conditions (68).
The five regions of Brazil showed inequalities in the prevalence of multimorbidity. The South and Southeast regions had higher prevalence, similar to that previously reported (63, 69, 70). No significant associations were observed in men. Conversely, women living in the South and Midwest regions had a higher multimorbidity prevalence than the others, corroborating a study conducted with a similar population (71). These outcomes probably reflect socioeconomic disparities in access to health services between sexes and regions. For example, states in the South and Southeast regions, which include the cities of São Paulo and Rio de Janeiro, have a higher percentage of urban population (72), higher level of socioeconomic development (73), are more industrialized, with better infrastructure (74) and have greater access to health services (75) compared to the North and Northeast regions. These aspects can facilitate access to the diagnosis of chronic diseases/conditions (76). In Brazil, the North (1.0/1,000 inhabitants) and Northeast (1.2/1,000 inhabitants) regions have the lowest ratio of doctors per 1,000 inhabitants in comparison to the Southeast (2.6/1,000 inhabitants) and South (2.0/1,000 inhabitants) (76). Another explanation for this result is the difference in demographic and epidemiological transition processes between the Brazilian regions. Data from the Global Burden of Disease Study 2016 showed that the transition from communicable, maternal, neonatal, and nutritional diseases to NCDs is more advanced in the South and Southeast regions, in addition to the higher life expectancy in these regions compared to the others (77).
Our findings revealed that smoking (former and current smokers) was positively associated with a higher prevalence of multimorbidity, regardless of sex, confirming existing literature (13–15). The greatest magnitude of association was observed in former smokers, which is in line with other investigations (78, 79). The cross-sectional nature of this study does not allow to establish causality between smoking cessation and multimorbidity. However, longitudinal studies have shown that a former history of smoking and current smoking increase the risk of multimorbidity (80, 81). Smoking is associated with the occurrence of multiple diseases. Recent research indicated that smoking was associated with 470 diseases, such as lung cancer, COPD, acute myocardial infarction, ischemic stroke, and ischemic heart disease (82). Cigarette smoke contains more than 7,000 chemical substances, of which approximately 70 are carcinogens. For instance, inhaling these chemicals leads to changes in airway inflammation, resulting in abnormal processes that can cause serious smoking-related illnesses, such as cancer and COPD (83, 84).
We observed that leisure-time physical inactivity was associated with multimorbidity in the total sample, as found in other studies (13–15). In the analysis stratified by sex, this association was verified only in men, corroborating previous investigations (85, 86). Another research estimated that physical inactivity is a primary cause of 35 chronic diseases/conditions that affect different body systems (87). Physical activity confers benefits for several health outcomes, such as improved physical fitness (cardiorespiratory and muscular fitness), cardiometabolic health (blood pressure, dyslipidemia, and insulin resistance), bone health, mental health, and reduced adiposity (88).
We found an inverse association between recommended fruit and vegetable consumption and multimorbidity, regardless of sex. Evidence suggests that high consumption of fruits and vegetables is a protective factor for several NCDs, such as obesity (89), ischemic stroke (90), and diabetes (91), contrary to our findings. This can be explained by the following factors: (i) information on the recommended fruit and vegetable consumption is self-reported in the NHS. Although this indicator provided satisfactory validity (92–94), response bias may have occurred; also, the data refer to the day before the interview, not reflecting the relationship between lifetime consumption and multimorbidity; (ii) people with multimorbidity tend to adopt healthier eating habits (17); and (iii) additional analysis (not shown in the results) demonstrated that the recommended fruit and vegetable consumption was twice as high in the older people compared to young people who, in turn, had a prevalence of multimorbidity almost seven times lower. This inverse association between healthy eating indicators and age may reflect a greater concern with health among older people, as described in the literature (20, 65, 95–98). Additionally, given the disagreements about the positive relationship between multimorbidity and recommended fruit and vegetable consumption (15, 16, 18, 19, 99), further in-depth investigations are required, especially using Food-Frequency Questionnaires. However, studies using data from the NHS 2013 (17, 18) showed similar results to ours.
The associations between overweight and multimorbidity are well known (18, 81, 100, 101), as found in this work. Obesity can lead to dyslipidemia, systemic inflammation, increased blood pressure, insulin resistance, and other metabolic changes, which may be common pathways for the development of cardiovascular disease and diabetes (102).
The results of this study indicate that the low educational level of the population and physical inactivity are determining factors for multimorbidity. Emphasizing the importance of actions that reduce health inequalities and promote the practice of physical activity. In addition, we showed that the older adults and women are vulnerable groups for the occurrence of multimorbidity. Therefore, tailored interventions for these groups are needed to prevent a scenario with an even higher prevalence of multimorbidity and its burden on the health system in the future.
This study has some limitations. First, the cross-sectional nature does not allow to establish causality between the independent variables and multimorbidity, as there may be reverse causality. Thus, longitudinal studies are needed. Second, data on chronic diseases/conditions were self-reported, considered less accurate than objective measures, and susceptible to memory and response bias. Therefore, some morbidities may be underestimated. Third, the definition of multimorbidity was based on the number of chronic diseases/conditions without considering severity. Ultimately, the analysis was based on a list of only 14 morbidities and the interviewee’s report of a medical diagnosis, which has a strong relationship with access to health services and may reduce the frequency of multimorbidity among the socioeconomically disadvantaged population. Moreover, mental disorders were grouped, and anxiety, the most prevalent mental illness in Brazil (103), was not analyzed. However, our investigation has several strengths, such as the complex sampling design, which makes it representative of the Brazilian adult population. Also, we examined the association of multimorbidity with sociodemographic and lifestyle factors stratified by sex. The results are valuable for future evidence-based recommendations, such as gender-specific prevention strategies to address multimorbidity. Importantly, our findings can be used to provide a deeper understanding of the association between sociodemographic and lifestyle determinants and multimorbidity in developing countries, considering the knowledge gap in the literature.
In conclusion, the higher prevalence of multimorbidity was associated with sociodemographic characteristics, including older age, female sex, living in the South and Southeast regions of Brazil, living in urban areas, in addition to unhealthy lifestyles, such as smoking and physical inactivity. Overweight and obesity also increased the prevalence of multimorbidity. Intriguingly, the recommended fruit and vegetable consumption demonstrated an inverse association. Compared with participants with higher educational levels, those with intermediate education (complete high school/incomplete higher education) had a lower prevalence of multimorbidity. In men and women, the association between education and multimorbidity showed differences, as well as for physical activity.
Publicly available datasets were analyzed in this study. This data can be found: Brazilian Institute of Geography and Statistics with the Ministry of Health/Brazil (https://biblioteca.ibge.gov.br/index.php/biblioteca-catalogo?view=detalhes&id=2101764).
The studies involving human participants were reviewed and approved by the Research Ethics Committee of the National Health Council under protocol number 3,529,376/2019. The patients/participants provided their written informed consent to participate in this study.
RG contributed to the conception and design of the study. CCP and RG organized the database and performed the statistical analysis. CCP wrote the first draft of the manuscript. CFP and SB wrote sections of the manuscript. All authors contributed to manuscript revision, read, and approved the submitted version.
This study was funded by the Ministry of Health of Brazil.
The authors thank the Ministry of Health of Brazil for funding the study. We also thank the Coordination for the Improvement of Higher Education Personnel (Coordination for the Improvement of Higher Education Personnel - CAPES) for helping with the publication fee in the article, process AUXPE number 88881.845026/2023-01.
The authors declare that the research was conducted in the absence of any commercial or financial relationships that could be construed as a potential conflict of interest.
All claims expressed in this article are solely those of the authors and do not necessarily represent those of their affiliated organizations, or those of the publisher, the editors and the reviewers. Any product that may be evaluated in this article, or claim that may be made by its manufacturer, is not guaranteed or endorsed by the publisher.
The Supplementary material for this article can be found online at: https://www.frontiersin.org/articles/10.3389/fpubh.2023.1193428/full#supplementary-material
1. Malta, DC, França, E, Abreu, DMX, Perillo, RD, Salmen, MC, Teixeira, RA, et al. Mortalidade por doenças não transmissíveis no brasil, 1990 a 2015, segundo estimativas do estudo de carga global de doenças. Sao Paulo Med J. (2017) 135:213–21. doi: 10.1590/1516-3180.2016.0330050117
2. Johnston, MC, Crilly, M, Black, C, Prescott, GJ, and Mercer, SW. Defining and measuring multimorbidity: a systematic review of systematic reviews. Eur J Pub Health. (2019) 29:182–9. doi: 10.1093/eurpub/cky098
3. Ho, ISS, Azcoaga-Lorenzo, A, Akbari, A, Davies, J, Hodgins, P, Khunti, K, et al. Variation in the estimated prevalence of multimorbidity: systematic review and meta-analysis of 193 international studies. BMJ Open. (2022) 12:1–11. doi: 10.1136/bmjopen-2021-057017
4. Nguyen, H, Manolova, G, Daskalopoulou, C, Vitoratou, S, Prince, M, and Prina, AM. Prevalence of multimorbidity in community settings: a systematic review and meta-analysis of observational studies. J Comorbidity. (2019) 9:2235042X1987093. doi: 10.1177/2235042X19870934
5. Huaquiá-Diáz, AM, Chalán-Dávila, TS, Carrillo-Larco, RM, and Bernabe-Ortiz, A. Multimorbidity in Latin America and the Caribbean: a systematic review and meta-analysis. BMJ Open. (2021) 11:e050409–8. doi: 10.1136/bmjopen-2021-050409
6. Menotti, A, Mulder, I, Nissinen, A, Giampaoli, S, Feskens, EJM, and Kromhout, D. Prevalence of morbidity and multimorbidity in elderly male populations and their impact on 10-year all-cause mortalitym: the FINE study (Finland, Italy, Netherlands, elderly). J Clin Epidemiol. (2001) 54:680–6. doi: 10.1016/S0895-4356(00)00368-1
7. Vogeli, C, Shields, AE, Lee, TA, Gibson, TB, Marder, WD, Weiss, KB, et al. Multiple chronic conditions: prevalence, health consequences, and implications for quality, care management, and costs. J Gen Intern Med. (2007) 22:391–5. doi: 10.1007/s11606-007-0322-1
8. Bayliss, EA, Bayliss, MS, Ware, JE, and Steiner, JF. Predicting declines in physical function in persons with multiple chronic medical conditions: what we can learn from the medical problem list. Health Qual Life Outcomes. (2004) 2:1–8. doi: 10.1186/1477-7525-2-47
9. Fortin, M, Lapointe, L, Hudon, C, Vanasse, A, Ntetu, AL, and Maltais, D. Multimorbidity and quality of life in primary care: a systematic review. Health Qual Life Outcomes. (2004) 2:1–12. doi: 10.1186/1477-7525-2-51
10. Lehnert, T, Heider, D, Leicht, H, Heinrich, S, Corrieri, S, Luppa, M, et al. Review: health care utilization and costs of elderly persons with multiple chronic conditions. Med Care Res Rev. (2011) 68:387–420. doi: 10.1177/1077558711399580
11. Pathirana, TI, and Jackson, CA. Socioeconomic status and multimorbidity: a systematic review and meta-analysis. Aust N Z J Public Health. (2018) 42:186–94. doi: 10.1111/1753-6405.12762
12. Ng, R, Sutradhar, R, Yao, Z, Wodchis, WP, and Rosella, LC. Smoking, drinking, diet and physical activity - modifiable lifestyle risk factors and their associations with age to first chronic disease. Int J Epidemiol. (2020) 49:113–30. doi: 10.1093/ije/dyz078
13. Dhalwani, NN, O’Donovan, G, Zaccardi, F, Hamer, M, Yates, T, Davies, M, et al. Long terms trends of multimorbidity and association with physical activity in older English population. Int J Behav Nutr Phys Act. (2016) 13:1–9. doi: 10.1186/s12966-016-0330-9
14. Katikireddi, SV, Skivington, K, Leyland, AH, Hunt, K, and Mercer, SW. The contribution of risk factors to socioeconomic inequalities in multimorbidity across the lifecourse: a longitudinal analysis of the Twenty-07 cohort. BMC Med. (2017) 15:152. doi: 10.1186/s12916-017-0913-6
15. Mounce, LTA, Campbell, JL, Henley, WE, Tejerina Arreal, MC, Porter, I, and Valderas, JM. Predicting incident multimorbidity. Ann Fam Med [Internet]. (2018) 16:322–9. doi: 10.1370/afm.2271
16. Shi, J, Guo, Y, Li, Z, Liang, Z, Pan, L, Yu, Y, et al. Sociodemographic and behavioral influences on multimorbidity among adult residents of northeastern China. BMC Public Health. (2022) 22:342–12. doi: 10.1186/s12889-022-12722-y
17. dos Santos, JEM, Crispim, SP, Murphy, J, and de Camargo, CM. Health, lifestyle and sociodemographic characteristics are associated with Brazilian dietary patterns: Brazilian national health survey. PLoS One. (2021) 16:e0247078. doi: 10.1371/journal.pone.0247078
18. Carvalho, JN, De Camargo, CM, Leandro, D, and De Souza, B. Lifestyle factors and high body mass index are associated with different multimorbidity clusters in the Brazilian population. PLoS One. (2018) 13:1–15. doi: 10.1371/journal.pone.0207649
19. Khan, N, Rahman, M, Mitra, D, and Afsana, K. Prevalence of multimorbidity among Bangladeshi adult population: a nationwide cross-sectional study. BMJ Open. (2019) 9:e030886. doi: 10.1136/bmjopen-2019-030886
20. Adams, J, and White, M. Characterisation of UK diets according to degree of food processing and associations with socio-demographics and obesity: cross-sectional analysis of UK National Diet and nutrition survey (2008-12). Int J Behav Nutr Phys Act. (2015) 12:160. doi: 10.1186/s12966-015-0317-y
21. Alimohammadian, M, Majidi, A, Yaseri, M, Ahmadi, B, Islami, F, Derakhshan, M, et al. Multimorbidity as an important issue among women: results of a gender difference investigation in a large population-based cross-sectional study in West Asia. BMJ Open. (2017) 7:e013548–8. doi: 10.1136/bmjopen-2016-013548
22. Sakib, MN, Shooshtari, S, St John, P, and Menec, V. The prevalence of multimorbidity and associations with lifestyle factors among middle-aged Canadians: an analysis of Canadian longitudinal study on aging data. BMC Public Health. (2019) 19:243. doi: 10.1186/s12889-019-6567-x
23. Chen, H, Cheng, M, Zhuang, Y, and Broad, JB. Multimorbidity among middle-aged and older persons in urban China: prevalence, characteristics and health service utilization. Geriatr Gerontol Int. (2018) 18:1447–52. doi: 10.1111/ggi.13510
24. Fortin, M, Bravo, G, Hudon, C, Vanasse, A, and Lapointe, L. Prevalence of multimorbidity among adults seen in family practice. Ann Fam Med. (2005) 3:223–8. doi: 10.1370/afm.272
25. Rizza, A, Kaplan, V, Senn, O, Rosemann, T, Bhend, H, Tandjung, R, et al. Age- and gender-related prevalence of multimorbidity in primary care: the Swiss FIRE project. BMC Fam Pract. (2012) 13:113. doi: 10.1186/1471-2296-13-113
26. Barford, A, Dorling, D, Smith, GD, and Shaw, M. Life expectancy: women now on top everywhere. Br Med J. (2006) 332:808. doi: 10.1136/bmj.332.7545.808
27. Marengoni, A, Angleman, S, Melis, R, Mangialasche, F, Karp, A, Garmen, A, et al. Aging with multimorbidity: a systematic review of the literature. Ageing Res Rev. (2011) 10:430–9. doi: 10.1016/j.arr.2011.03.003
28. Amuthavalli Thiyagarajan, J, Mikton, C, Harwood, RH, Gichu, M, Gaigbe-Togbe, V, Jhamba, T, et al. The un decade of healthy ageing: strengthening measurement for monitoring health and wellbeing of older people. Age Ageing. (2022) 51:1–5. doi: 10.1093/ageing/afac147
29. World Health Organization . Global action plan for the prevention and control of noncommunicable diseases 2013–2020. Geneva: WHO (2013).
30. Brasil . Plano de Ações Estratégicas para o Enfrentamento das Doenças Crônicas e Agravos não Transmissíveis no Brasil 2021–2030. Brasília: Ministério da Saúde (2021) Available at: http://bvsms.saude.gov.br/bvs/publicacoes/plano_enfrentamento_doencas_cronicas_agravos_2021_2030.pdf.
31. Stopa, SR, Szwarcwald, CL, de Oliveira, MM, Gouvea, ECDP, Vieira, MLFP, de Freitas, MPS, et al. Pesquisa Nacional de Saúde 2019: histórico, métodos e perspectivas. Epidemiol Serv Saude. (2020) 29:e2020315. doi: 10.1590/s1679-49742020000500004
32. Zhang, Y, Zhou, L, Liu, S, Qiao, Y, Wu, Y, Ke, C, et al. Prevalence, correlates and outcomes of multimorbidity among the middle-aged and elderly: findings from the China health and retirement longitudinal study. Arch Gerontol Geriatr. (2020) 90:104135. doi: 10.1016/j.archger.2020.104135
33. Instituto Brasileiro de Geografia e Estatistica – IBGE . Pesquisa Nacional por Amostra de Domicílios Contínua - PNAD Contínua - 2019. (2022). Available at: https://painel.ibge.gov.br/pnadc/
34. Rogers, B, Nabholz, C, and Woodward, S. Risk dialogue series: health risk factors Brazil. Zurich: Swiss Re Centre for Global Dialogue. (2014) 58.
35. Instituto Brasileiro de Geografia e Estatística . Características étnico-raciais da população: um estudo das categorias de classificação de cor ou raça 2008. Rio de Janeiro: IBGE (2011).
36. Gomes, CS, Gonçalves, RPF, da Silva, AG, de Sá, ACMGN, Alves, FTA, Ribeiro, ALP, et al. Factors associated with cardiovascular disease in the Brazilian adult population: National Health Survey, 2019. Rev Bras Epidemiol. (2021) 24:1–16. doi: 10.1590/1980-549720210013.supl.2
37. Guimarães, RA, de Neto, OLM, de Souza, MR, Cortez-Escalante, JJ, de Santos, TAP, CFW, R, et al. Epidemiology of self-reported diabetes mellitus in the state of Maranhão, northeastern Brazil: results of the national health survey, 2013. Int J Environ Res Public Health. (2019) 16:1–9. doi: 10.3390/ijerph16010047
38. Malta, DC, Gomes, CS, de Andrade, FMD, Prates, EJS, Alves, FTA, de Oliveira, PPV, et al. Tobacco use, cessation, secondhand smoke and exposure to media about tobacco in Brazil: results of the National Health Survey 2013 and 2019. Rev Bras Epidemiol. (2021) 24:1–16. doi: 10.1590/1980-549720210006.supl.2
39. Sá da Silva, LE, Helman, B, da Luz e Silva, DC, de Aquino, ÉC, de Freitas, PC, de Santos, RO, et al. Prevalence of heavy episodic drinking in the Brazilian adult population: National Health Survey 2013 and 2019. Epidemiol Serv Saude. (2022) 31:e2021379. doi: 10.1590/ss2237-9622202200003.especial
40. Mielke, GI, Stopa, SR, Gomes, CS, da Silva, AG, Alves, FTA, Vieira, MLFP, et al. Leisure time physical activity among Brazilian adults: National Health Survey 2013 and 2019. Rev Bras Epidemiol. (2021) 24:1–11. doi: 10.1590/1980-549720210008.supl.2
41. IBGE . Pesquisa Nacional de Saúde: Percepção do estado de saúde, estilos de vida, doenças crônicas e saúde bucal: Brasil e grandes regiões/IBGE, Coordenação de Trabalho e Rendimento. Rio de Janeiro: IBGE (2020) Available at: http://www.pns.icict.fiocruz.br/arquivos/Portaria.pdf.
42. World Health Organization . Physical status: the use and interpretation of anthropometry. Geneva: WHO (1995) Available at: https://www.analesdepediatria.org/en-tuberculosis-in-paediatric-age-group-articulo-S2341287920302544.
43. De Carvalho, JN, Roncalli, ÂG, De Camargo, CM, and De Souza, DLB. Prevalence of multimorbidity in the Brazilian adult population according to socioeconomic and demographic characteristics. PLoS One. (2017) 12:1–13. doi: 10.1371/journal.pone.0174322
44. Puri, P, Singh, SK, and Pati, S. Temporal dynamics, patterns and correlates of single and multimorbidity in India, 1994–2018. J Comorb. (2021) 11:263355652110627. doi: 10.1177/26335565211062756
45. Weimann, A, Dai, D, and Oni, T. A cross-sectional and spatial analysis of the prevalence of multimorbidity and its association with socioeconomic disadvantage in South Africa: a comparison between 2008 and 2012. Soc Sci Med. (2016) 163:144–56. doi: 10.1016/j.socscimed.2016.06.055
46. Oni, T, McGrath, N, BeLue, R, Roderick, P, Colagiuri, S, May, CR, et al. Chronic diseases and multi-morbidity - a conceptual modification to the WHO ICCC model for countries in health transition. BMC Public Health. (2014) 14:1–7. doi: 10.1186/1471-2458-14-575
47. Galor, O . The demographic transition: causes and consequences. NIH Public Access. (2012) 6:1–28. doi: 10.1007/s11698-011-0062-7
48. Jaul, E, and Barron, J. Age-related diseases and clinical and public health implications for the 85 years old and over population. Front Public Health. (2017) 5:1–7. doi: 10.3389/fpubh.2017.00335
49. Aalami, OO, Fang, TD, Song, HM, and Nacamuli, RP. Physiological features of aging persons. Arch Surg. (2003) 138:1068–76. doi: 10.1001/archsurg.138.10.1068
50. Chudasama, YV, Khunti, KK, Zaccardi, F, Rowlands, AV, Yates, T, Gillies, CL, et al. Physical activity, multimorbidity, and life expectancy: a UK biobank longitudinal study. BMC Med. (2019) 17:1–13. doi: 10.1186/s12916-019-1339-0
51. Fortin, M, Stewart, M, Poitras, ME, Almirall, J, and Maddocks, H. A systematic review of prevalence studies on multimorbidity: toward a more uniform methodology. Ann Fam Med. (2012) 10:142–51. doi: 10.1370/afm.1337
52. Violan, C, Foguet-Boreu, Q, Flores-Mateo, G, Salisbury, C, Blom, J, Freitag, M, et al. Prevalence, determinants and patterns of multimorbidity in primary care: a systematic review of observational studies. PLoS One. (2014) 9:1–9. doi: 10.1371/journal.pone.0102149
53. Dennerstein, L, Feldman, S, Murdaugh, C, Rossouw, J, and Tennstedt, S. Gender and health issues in ageing. Australas J Ageing. (1998) 17:19–21. doi: 10.1111/j.1741-6612.1998.tb00851.x
54. Jantsch, AG, Alves, RFS, and Faerstein, E. Educational inequality in Rio de Janeiro and its impact on multimorbidity: evidence from the pró-saúde study. A cross-sectional analysis. Sao Paulo Med J. (2018) 136:51–8. doi: 10.1590/1516-3180.2017.0209100917
55. Nagel, G, Peter, R, Braig, S, Hermann, S, Rohrmann, S, and Linseisen, J. The impact of education on risk factors and the occurrence of multimorbidity in the EPIC-Heidelberg cohort. BMC Public Health. (2008) 8:1–10. doi: 10.1186/1471-2458-8-384
56. Costa, AK, Bertoldi, AD, Fontanella, AT, Ramos, LR, Arrais, PSD, Luiza, VL, et al. Does socioeconomic inequality occur in the multimorbidity among Brazilian adults? Rev Saude Publica. (2020) 54:138–12. doi: 10.11606/s1518-8787.2020054002569
57. Mini, GK, and Thankappan, KR. Pattern, correlates and implications of non-communicable disease multimorbidity among older adults in selected Indian states: a cross-sectional study. Open [Internet]. (2017) 7:e013529. doi: 10.1136/bmjopen-2016-013529
58. Zou, S, Wang, Z, Bhura, M, Zhang, G, and Tang, K. Prevalence and associated socioeconomic factors of multimorbidity in 10 regions of China: an analysis of 0.5 million adults. J Public Health. (2022) 44:36–50. doi: 10.1093/pubmed/fdaa204
59. Garin, N, Koyanagi, A, Chatterji, S, Tyrovolas, S, Olaya, B, Leonardi, M, et al. Global multimorbidity patterns: a cross-sectional, population-based, multi-country study. J Gerontol Ser A Biol Sci Med Sci. (2016) 71:205–14. doi: 10.1093/gerona/glv128
60. Pati, S, Agrawal, S, Swain, S, Lee, JT, Vellakkal, S, Hussain, MA, et al. Non communicable disease multimorbidity and associated health care utilization and expenditures in India: cross-sectional study. BMC Health Serv Res. (2014) 14:451. doi: 10.1186/1472-6963-14-451
61. Mu, T, Xu, R, Zhu, Q, Chen, L, Dong, D, Xu, J, et al. Diet-related knowledge, attitudes, and behaviors among young and middle-aged individuals with high-normal blood pressure: a cross-sectional study in China. Front Public Health. (2022) 10:1–12. doi: 10.3389/fpubh.2022.898457
62. Enroth, L, Raitanen, J, Hervonen, A, and Jylhä, M. Do socioeconomic health differences persist in nonagenarians? J Gerontol Ser B Psychol Sci Soc Sci. (2013) 68:837–47. doi: 10.1093/geronb/gbt067
63. Batista, SR, Sousa, ALL, Nunes, BP, Silva, RR, and Jardim, PCBV. Identifying multimorbidity clusters among Brazilian older adults using network analysis: findings and perspectives. PLoS One. (2022) 17:e0271639. doi: 10.1371/journal.pone.0271639
64. Violán, C, Foguet-Boreu, Q, Roso-Llorach, A, Rodriguez-Blanco, T, Pons-Vigués, M, Pujol-Ribera, E, et al. Burden of multimorbidity, socioeconomic status and use of health services across stages of life in urban areas: a cross-sectional study. BMC Public Health. (2014) 14:530. doi: 10.1186/1471-2458-14-530
65. Marrón-Ponce, JA, Sánchez-Pimienta, TG, Da Costa Louzada, ML, and Batis, C. Energy contribution of NOVA food groups and sociodemographic determinants of ultra-processed food consumption in the Mexican population. Public Health Nutr. (2018) 21:87–93. doi: 10.1017/S1368980017002129
66. Westbury, S, Ghosh, I, Jones, HM, Mensah, D, Samuel, F, Irache, A, et al. The influence of the urban food environment on diet, nutrition and health outcomes in low-income and middle-income countries: a systematic review. BMJ Glob Heal. (2021) 6:e006358. doi: 10.1136/bmjgh-2021-006358
67. Mills, S, White, M, Brown, H, Wrieden, W, Kwasnicka, D, Halligan, J, et al. Health and social determinants and outcomes of home cooking: a systematic review of observational studies. Appetite. (2017) 111:116–34. doi: 10.1016/j.appet.2016.12.022
68. Harpham, T, and Tanner, M. Urbanization and health in developing countries: a review of some trends. Urban Heal Dev Ctries. (2018) 15:1–8. doi: 10.4324/9781315070698-1
69. Christofoletti, M, Del Duca, GF, Benedetti, TRB, and Malta, DC. Sociodemographic determinants of multimorbidity in Brazilian adults and older adults: a cross-sectional study. Sao Paulo Med J. (2022) 140:115–22. doi: 10.1590/1516-3180.2021.0105.r1.31052021
70. Rzewuska, M, De Azevedo-Marques, JM, Coxon, D, Zanetti, ML, Zanetti, ACG, Franco, LJ, et al. Epidemiology of multimorbidity within the Brazilian adult general population: evidence from the 2013 National Health Survey (PNS 2013). PLoS One. (2017) 12:12(2). doi: 10.1371/journal.pone.0171813
71. Shi, X, da Silva Lima, SM, de Miranda Mota, CM, Lu, Y, Stafford, RS, and Pereira, CV. Prevalence of multimorbidity of chronic noncommunicable diseases in Brazil: population-based study. JMIR Public Heal Surveill. (2021) 7:e29693–3. doi: 10.2196/29693
72. Instituto Brasileiro de Geografia e Estatística - IBGE . Censo demográfico: 2010: resultados gerais da amostra. Rio de Janeiro: IBGE (2010).
73. Viacava, F, and Bellido, JG. Health, access to services and sources of payment, according to household surveys. Cienc Saude Coletiva. (2016) 21:351–70. doi: 10.1590/1413-81232015212.19422015
74. David, GC, Shimizu, HE, and da Silva, EN. Atenção Primária à Saúde nos municípios brasileiros: eficiência e disparidades. Saúde em. Debate. (2015) 39:232–45. doi: 10.5935/0103-1104.2015S005512
75. Stopa, SR, Malta, DC, Monteiro, CN, Szwarcwald, CL, Goldbaum, M, and Cesar, CLG. Use of and access to health services in Brazil, 2013 National Health Survey. Rev Saude Publica. (2017) 51:1S–10S. doi: 10.1590/s1518-8787.2017051000074
76. Silveira, RP, and Pinheiro, R. Understanding the need for doctors in Innerstate amazon – Brazil. Rev Bras Educ Med. (2014) 38:451–9. doi: 10.1590/S0100-55022014000400006
77. Marinho, F, de Azeredo Passos, VM, Carvalho Malta, D, Barboza França, E, Abreu, DMX, Araújo, VEM, et al. Burden of disease in Brazil, 1990–2016: a systematic subnational analysis for the global burden of disease study 2016. Lancet. (2018) 392:760–75. doi: 10.1016/S0140-6736(18)31221-2
78. Nunes, BP, Batista, SRR, de Andrade, FB, Souza Junior, PRB, de Lima-Costa, MF, and Facchini, LA. Multimorbidity em indivíduos com 50 anos ou mais de idade: ELSI-Brasil. Rev Saude Publica. (2019) 52:10s. doi: 10.11606/s1518-8787.2018052000637
79. Keomma, K, Bousquat, A, and César, CLG. Prevalência de multimorbidade em idosos em São Paulo, Brasil: um estudo com o ISA-Capital. Rev Saude Publica. (2022) 56:69. doi: 10.11606/s1518-8787.2022056004252
80. Bisquera, A, Turner, EB, Ledwaba-Chapman, L, Dunbar-Rees, R, Hafezparast, N, Gulliford, M, et al. Inequalities in developing multimorbidity over time: a population-based cohort study from an urban, multi-ethnic borough in the United Kingdom. Lancet Reg Heal Eur. (2022) 12:100247. doi: 10.1016/j.lanepe.2021.100247
81. Wikström, K, Lindström, J, Harald, K, Peltonen, M, and Laatikainen, T. Clinical and lifestyle-related risk factors for incident multimorbidity: 10-year follow-up of Finnish population-based cohorts 1982-2012. Eur J Intern Med. (2015) 26:211–6. doi: 10.1016/j.ejim.2015.02.012
82. Chan, KH, Wright, N, Xiao, D, Guo, Y, Chen, Y, Du, H, et al. Tobacco smoking and risks of more than 470 diseases in China: a prospective cohort study. Lancet Public Health. (2022) 7:e1014–26. doi: 10.1016/S2468-2667(22)00227-4
83. Stämpfli, MR, and Anderson, GP. How cigarette smoke skews immune responses to promote infection, lung disease and cancer. Nat Rev Immunol. (2009) 9:377–84. doi: 10.1038/nri2530
84. Yang, IA, Relan, V, Wright, CM, Davidson, MR, Sriram, KB, Savarimuthu Francis, SM, et al. Common pathogenic mechanisms and pathways in the development of COPD and lung cancer. Expert Opin Ther Targets. (2011) 15:439–56. doi: 10.1517/14728222.2011.555400
85. de Almeida, MGN, Nascimento-Souza, MA, Lima-Costa, MF, and Peixoto, SV. Lifestyle factors and multimorbidity among older adults (ELSI-Brazil). Eur J Ageing. (2020) 17:521–9. doi: 10.1007/s10433-020-00560-z
86. Autenrieth, CS, Kirchberger, I, Heier, M, Zimmermann, AK, Peters, A, Döring, A, et al. Physical activity is inversely associated with multimorbidity in elderly men: results from the KORA-age Augsburg study. Prev Med. (2013) 57:17–9. doi: 10.1016/j.ypmed.2013.02.014
87. Booth, FW, Roberts, CK, and Laye, MJ. Lack of exercise is a major cause of chronic diseases. Compr Physiol. (2012) 2:1143–211. doi: 10.1002/cphy.c110025
88. World Health Organization . WHO guidelines on physical activity and sedentary behaviour: at a glance. Geneva: World Health Organization (2020).
89. Nouri, M, Shateri, Z, and Faghih, S. The relationship between intake of fruits, vegetables and dairy products with overweight and obesity in a large sample in Iran: findings of STEPS 2016. Front Nutr. (2023) 9:1082976. doi: 10.3389/fnut.2022.1082976
90. Johansson, A, Acosta, S, Mutie, PM, Sonestedt, E, Engström, G, and Drake, I. Components of a healthy diet and different types of physical activity and risk of atherothrombotic ischemic stroke: a prospective cohort study. Front Cardiovasc Med. (2022) 9:993112. doi: 10.3389/fcvm.2022.993112
91. Noori, S, Mirzababaei, A, Abaj, F, Ghaffarian-Ensaf, R, and Mirzaei, K. Does the Mediterranean diet reduce the odds of diabetic nephropathy in women? A case–control study. Front Nutr. (2022) 9:984622. doi: 10.3389/fnut.2022.984622
92. Mendes, L. L., Campos, S. F., Malta, D. C., Bernal, R. T. I., de-Sá, N. N. B., and Velásquez-Meléndez, G. Validade e reprodutibilidade de marcadores do consumo de alimentos e bebidas de um inquérito telefônico realizado na cidade de Belo Horizonte (MG).Brasil Revista Brasileira de Epidemiologia. (2011) 14:80–9.
93. Monteiro, CA, Moura, EC, and Jaime, PCCR. Validity of food and beverage intake data obtained by telephone survey. Rev Saúde Pública. (2008) 42:582–9. doi: 10.1590/S0034-89102008000400002
94. das Neves, ACM, LAA, G, IBG, M, and Moura, EC. Validação de indicadores do consumo de alimentos e bebidas obtidos por inquérito telefônico em Belém, Pará, Brasil. Cad Saude Publica. (2010) 26:2379–88. doi: 10.1590/S0102-311X2010001200016
95. Moubarac, JC, Batal, M, Louzada, ML, Martinez Steele, E, and Monteiro, CA. Consumption of ultra-processed foods predicts diet quality in Canada. Appetite. (2017) 108:512–20. doi: 10.1016/j.appet.2016.11.006
96. Cediel, G, Reyes, M, Da Costa Louzada, ML, Martinez Steele, E, Monteiro, CA, Corvalán, C, et al. Ultra-processed foods and added sugars in the Chilean diet (2010). Public Health Nutr. (2018) 21:125–33. doi: 10.1017/S1368980017001161
97. Baraldi, LG, Martinez Steele, E, Canella, DS, and Monteiro, CA. Consumption of ultra-processed foods and associated sociodemographic factors in the USA between 2007 and 2012: Evidence from a nationally representative cross-sectional study. BMJ Open. (2018) 8: 1–9.
98. Khandpur, N, Cediel, G, Obando, DA, Jaime, PC, and Parra, DC. Sociodemographic factors associated with the consumption of ultra-processed foods in Colombia. Rev Saude Publica. (2020) 54:19. doi: 10.11606/s1518-8787.2020054001176
99. Pereira, BP, Bortolotto, CC, Tomasi, E, Gonzalez, MC, Gomes, AP, Gonçalves, H, et al. Consumo alimentar e multimorbidade entre idosos não institucionalizados de Pelotas, 2014: estudo transversal. Epidemiol Serv Saude. (2020) 29:e2019050. doi: 10.5123/S1679-49742020000300015
100. Bezerra de Souza, DL, Oliveras-Fabregas, A, Espelt, A, Bosque-Prous, M, de Camargo, CM, Teixidó-Compañó, E, et al. Multimorbidity and its associated factors among adults aged 50 and over: a cross-sectional study in 17 European countries. PLoS One. (2021) 16:1–16. doi: 10.1371/journal.pone.0246623
101. Li, X, Cai, L, Cui, WL, Wang, XM, Li, HF, He, JH, et al. Association of socioeconomic and lifestyle factors with chronic non-communicable diseases and multimorbidity among the elderly in rural Southwest China. J Public Health. (2020) 42:239–46. doi: 10.1093/pubmed/fdz020
102. Kivimäki, M, Kuosma, E, Ferrie, JE, Luukkonen, R, Nyberg, ST, Alfredsson, L, et al. Overweight, obesity, and risk of cardiometabolic multimorbidity: pooled analysis of individual-level data for 120 813 adults from 16 cohort studies from the USA and Europe. Lancet Public Health. (2017) 2:e277–85. doi: 10.1016/S2468-2667(17)30074-9
Keywords: multimorbidity, multiple chronic conditions, chronic disease, risk factors, public health
Citation: Pereira CC, Pedroso CF, Batista SRR and Guimarães RA (2023) Prevalence and factors associated with multimorbidity in adults in Brazil, according to sex: a population-based cross-sectional survey. Front. Public Health. 11:1193428. doi: 10.3389/fpubh.2023.1193428
Received: 24 March 2023; Accepted: 12 May 2023;
Published: 02 June 2023.
Edited by:
Nicolai Savaskan, District Office Neukölln of Berlin Neukölln, GermanyReviewed by:
Alexandra Roth, Gesundheitsamt Neukölln, GermanyCopyright © 2023 Pereira, Pedroso, Batista and Guimarães. This is an open-access article distributed under the terms of the Creative Commons Attribution License (CC BY). The use, distribution or reproduction in other forums is permitted, provided the original author(s) and the copyright owner(s) are credited and that the original publication in this journal is cited, in accordance with accepted academic practice. No use, distribution or reproduction is permitted which does not comply with these terms.
*Correspondence: Rafael Alves Guimarães, cmFmYWVsYWx2ZXNAdWZnLmJy
Disclaimer: All claims expressed in this article are solely those of the authors and do not necessarily represent those of their affiliated organizations, or those of the publisher, the editors and the reviewers. Any product that may be evaluated in this article or claim that may be made by its manufacturer is not guaranteed or endorsed by the publisher.
Research integrity at Frontiers
Learn more about the work of our research integrity team to safeguard the quality of each article we publish.