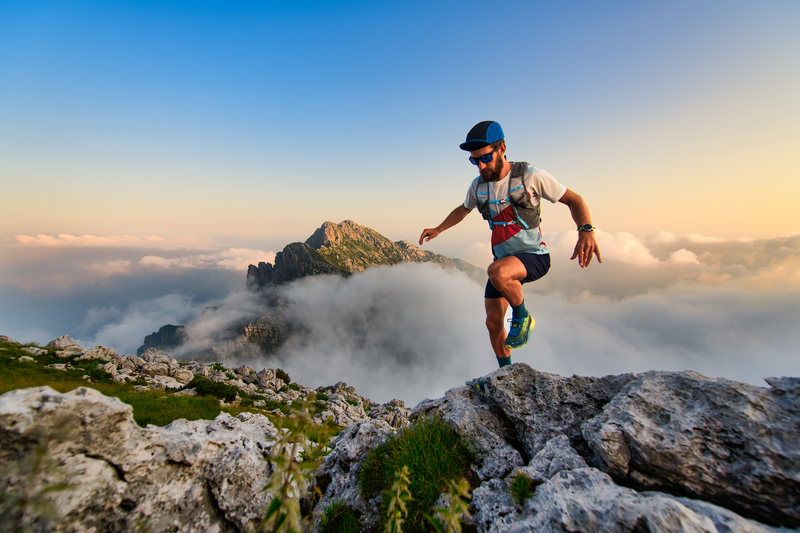
94% of researchers rate our articles as excellent or good
Learn more about the work of our research integrity team to safeguard the quality of each article we publish.
Find out more
PERSPECTIVE article
Front. Public Health , 15 June 2023
Sec. Infectious Diseases: Epidemiology and Prevention
Volume 11 - 2023 | https://doi.org/10.3389/fpubh.2023.1179261
This article is part of the Research Topic Impact of public health and social measures for COVID-19 control on infectious disease epidemiology View all 14 articles
The COVID-19 pandemic has had a significant impact on global mortality. While the causal relationship between SARS-CoV-2 and the anomalous increase in deaths is established, more precise and complex models are needed to determine the exact weight of epidemiological factors involved. Indeed, COVID-19 behavior is influenced by a wide range of variables, including demographic characteristics, population habits and behavior, healthcare performance, and environmental and seasonal risk factors. The bidirectional causality between impacted and impacting aspects, as well as confounding variables, complicates efforts to draw clear, generalizable conclusions regarding the effectiveness and cost-benefit ratio of non-pharmaceutical health countermeasures. Thus, it is imperative that the scientific community and health authorities worldwide develop comprehensive models not only for the current pandemic but also for future health crises. These models should be implemented locally to account for micro-differences in epidemiological characteristics that may have relevant effects. It is important to note that the lack of a universal model does not imply that local decisions have been unjustified, and the request to decrease scientific uncertainty does not mean denying the evidence of the effectiveness of the countermeasures adopted. Therefore, this paper must not be exploited to denigrate either the scientific community or the health authorities.
The dramatic impact of COVID-19 on global mortality is a scientific fact (1–3). Indeed, the size and significance of the effect are so large and in agreement with the vast literature on the subject that bias analysis is not needed to ascertain the mere existence of this causal relationship (4). However, more precise and complex models are required to attribute the exact weight to all the epidemiological factors involved. Indeed, although it is true that the ability of a pathogen to compromise public health—in all its aspects—is part of its inherent hazard (e.g., overloading of health facilities due to high contagiousness and virulence), it is also true that such a threat is determined by the variables that it affects (e.g., the performance of health facilities). Moreover, COVID-19 behavior appears to be influenced by a wide range of risk factors and determinants, the assessment of which is undermined by known confounders and bidirectional relationships (Table 1). Specifically, in such a mathematically and scientifically complex system, constructing a global statistical cost function and ascertain primary causes of phenomena can be demanding. Since these elements are essential to draw up a prioritization scheme of interventions (i.e., which variables to tackle or influence first to obtain the best benefit) as well as a methodology of intervention (i.e., how to tackle or influence a specific variable to obtain the best benefit), the whole public health decision-making process is potentially compromised and/or severely slowed down. To the best of the authors' knowledge, no current model satisfactorily incorporates all these variables. This also makes scientific conclusions, and therefore public health actions, varyingly exposed to authors' interpretations and biases (5).
A very contagious and aggressive virus like SARS-CoV-2 can impair the health system causing facilities overload (6), shortage of healthcare personnel (7, 8), shortage of medical-related supplies (6, 9), physical and mental exhaustion of healthcare workers (10), and other human errors in managing the emergency (10, 11) (impacted aspects). At the same time, poor healthcare can obviously increase COVID-19 (and other diseases) severity, fatality, and mortality. For this reason, causality between impacted and impacting aspects is bidirectional and subject to confounding.
The scientific literature on COVID-19 reports various risk factors related to the individual's health status, including age, gender, and a long list of specific pre-existing conditions (12–15), population habits, movements and adherence to anti-pandemic regulations (15, 16), insufficient or delayed healthcare, information overabundance and success of misleading and/or incorrect news (even among healthcare workers) (17), air pollution (18), environmental and meteorological factors including temperature, relative humidity, sunlight, and wind (19–22), and seasonal risks such as the arrival of cold weather (19, 20).
Historical differences in risk factors and health service adequacy may create apparent differences in virus fatality and severity as intrinsic epidemiological characteristics. Furthermore, lockdowns and social distancing—considered by the majority of the scientific community as an essential tool for the containment of the infection (23–25)—have caused heterogeneous detrimental effects, varying in effect size and prevalence, both at the socio-psychological level (26–28) (which can have repercussions on physical health), in healthcare services, and even contagion dynamics (29). Alongside this, asymptomatic cases, insufficient testing capacity and quality can further bias the estimation of deaths possibly associated with COVID-19 (30–33).
In light of this evidence, I ask that the scientific community and health authorities worldwide begin to develop comprehensive models not only for the current pandemic emergency but also for future health crises. Considering a typical epidemiological study design, this means conducting a thorough literature search on all known or suspected variables that may potentially interact with the pathogen of interest. This also means developing multivariable models with parameters based on local empirical characteristics (from the availability of drugs to suspected evolutionary mutations) to determine the epidemiological role and weight of each variable by fitting the observed data. One possible approach to achieving this goal is to use mixed models with reciprocal effects, training established algorithms (e.g., hierarchical regression and extended SEIR) enhanced with artificial intelligence (e.g., machine learning and neural networks) on both historical and current data (34–38). Naturally, bias analysis and expertise play a crucial role in accurate implementation. This also calls for further research on mathematical-epidemiological modeling of human aspects in various contexts, including general (e.g., people behavior), professional (e.g., hospital assistance dynamics), psychological (e.g., psychological reactions), and infodemiological (e.g., the effects of infodemics on concrete actions). Although the inclusion of all relevant variables may be an unattainable objective, successful modeling of some additional single or even groups of factors would allow for a better estimation of the impact of the remaining (un-modeled). The sensitivity analysis should be utilized to assess the reliability and predictive power of the models, as well as to examine the intercorrelations among inputs and outputs (39). Besides, the mission should not solely be to ensure the short-term survival of as many people as possible, but rather to seek a solution that ensures a sustainable lifestyle (i.e., both socially and psychologically viable). For example, by establishing varying degrees of lockdown severity and quality of life, the aim should be to scientifically establish the minimum severity level at which the mere epidemiological risk and quality of life are deemed acceptable. Such a point is vital for the success of long-term policies since people's adherence is strongly affected by pandemic fatigue and similar phenomena (40–42). Thus, decisions should be made based on the related cost functions. Indeed, at present, it is challenging to draw clear, unequivocal, and generalizable conclusions not so much on the effectiveness as on the cost-benefit ratio of non-pharmaceutical health countermeasures. Likewise, comparisons between countries' policies are also often arbitrary. In this scope, such models should be implemented locally to account for micro-differences in epidemiological characteristics that may have relevant effects (e.g., evolutionary mutations and/or particularly polluted areas that increase the pathogen virulence in a certain region). By doing so, it would be possible to ensure and protect public health in a timely manner based on the best available scientific evidence, minimizing the epidemiological impact and uncertainty in decision-making thanks to more targeted and specific investigations and interventions. This could also lead to greater trust in institutions (which could plausibly translate into greater adherence to required health regulations) and a decrease in fallacious and misleading debates on counterfactual scenarios (e.g., what would have happened if...). Undoubtedly, such a strategy would necessitate increased investment in local resources, such as surveillance systems and appropriately trained personnel. However, comparing provincial, regional, and national models could yield valuable insights into their differences and similarities, allowing for a better understanding of which factors require local analysis versus those that can be effectively modeled at larger scales. Whether this paper is too ambitious or not, the above considerations highlight that it is paramount to establish a theoretical goal to strive for and to call for moderation among those scientists who express too much certainty on inherently dubious topics, risking fostering distrust toward institutions and science (43, 44). Finally, I conclude by saying that this perspective must not be exploited to denigrate either the scientific community or the health authorities since (i) the lack of a universal model does not in any way imply that local decisions have been unjustified, and (ii) the request to decrease scientific uncertainty does not mean denying the evidence on the effectiveness of the countermeasures adopted but only expecting greater precision in ascertaining the entity of costs and benefits for future implementations. In this regard, the author of this paper expresses their solidarity with the victims of the COVID-19 pandemic phenomenon and with those who made great responsibility decisions disposing only of limited and uncertain data during a period of extreme social tension.
The original contributions presented in the study are included in the article/supplementary material, further inquiries can be directed to the corresponding author.
The author confirms being the sole contributor of this work and has approved it for publication.
The author declares that the research was conducted in the absence of any commercial or financial relationships that could be construed as a potential conflict of interest.
All claims expressed in this article are solely those of the authors and do not necessarily represent those of their affiliated organizations, or those of the publisher, the editors and the reviewers. Any product that may be evaluated in this article, or claim that may be made by its manufacturer, is not guaranteed or endorsed by the publisher.
1. Rovetta A, Bhagavathula AS. The impact of COVID-19 on mortality in Italy: retrospective analysis of epidemiological trends. JMIR Public Health Surv. (2022) 8:e36022. doi: 10.2196/36022
2. Rovetta A. Annual excess crude mortality in Europe during the COVID-19 pandemic: a longitudinal joinpoint regression analysis of historical trends from 2000 to 2021. COVID. (2022) 2:1778–86. doi: 10.3390/covid2120128
3. COVID-19 Excess Mortality Collaborators (2022). Estimating excess mortality due to the COVID-19 pandemic: a systematic analysis of COVID-19-related mortality, 2020–21. Lancet. 399:1513–36. doi: 10.1016/S0140-6736(21)02796-3
4. Lash TL, Fox MP, MacLehose RF, Maldonado G, McCandless LC, Greenland S. Good practices for quantitative bias analysis. Int J Epidemiol. (2014) 43:1969–85. doi: 10.1093/ije/dyu149
5. Greenland S. Transparency and disclosure, neutrality and balance: shared values or just shared words? J Epidemiol Commun Health. (2012) 66:967–70. doi: 10.1136/jech-2011-200459
6. Ferrara P, Albano L. COVID-19 and healthcare systems: what should we do next? Public Health. (2020) 185:1–2. doi: 10.1016/j.puhe.2020.05.014
7. Blanchard A. C., Lamarre V., Lamarche J., Audy N., Quach C. (2022). Mitigating healthcare staffing shortages: Should healthcare workers with severe acute respiratory coronavirus virus 2 (SARS-CoV-2) household exposures work? Infect Cont Hosp Epidemiol, 1–3. doi: 10.1017/ice.2022.195
8. Leber AL Peterson E Dien Bard J Personnel Standards and Workforce Subcommittee American Society for Microbiology. The hidden crisis in the times of COVID-19: critical shortages of medical laboratory professionals in clinical microbiology. J Clin Microbiol. (2022) 60:e0024122. doi: 10.1128/jcm.00241-22
9. Aljadeed R, AlRuthia Y, Balkhi B, Sales I, Alwhaibi M, Almohammed O, et al. The impact of COVID-19 on essential medicines and personal protective equipment availability and prices in Saudi Arabia. Healthcare. (2021) 9:290. doi: 10.3390/healthcare9030290
10. Leo CG, Sabina S, Tumolo MR, Bodini A, Ponzini G, Sabato E, et al. Burnout among healthcare workers in the COVID 19 era: a review of the existing literature. Front Public Health. (2021) 9:750529. doi: 10.3389/fpubh.2021.750529
11. Gholami M, Fawad I, Shadan S, Rowaiee R, Ghanem H, Hassan Khamis A, et al. COVID-19 and healthcare workers: a systematic review and meta-analysis. Int J Infect Dis. (2021) 104:335–46. doi: 10.1016/j.ijid.2021.01.013
12. Centers for Disease Control and Prevention. People with Certain Medical Conditions. COVID-19. (2023). Available online at: https://www.cdc.gov/coronavirus/2019-ncov/need-extra-precautions/people-with-medical-conditions.html (accessed May 02, 2023).
13. Rahman A, Sathi NJ. Risk factors of the severity of COVID-19: a meta-analysis. Int J Clin Pract. (2021) 75:e13916. doi: 10.1111/ijcp.13916
14. Booth A, Reed AB, Ponzo S, Yassaee A, Aral M, Plans et al. Population risk factors for severe disease and mortality in COVID-19: a global systematic review and meta-analysis. PLoS ONE. (2021) 16:e0247461. doi: 10.1371/journal.pone.0247461
15. Dessie ZG, Zewotir T. Mortality-related risk factors of COVID-19: a systematic review and meta-analysis of 42 studies and 423,117 patients. BMC Infect Dis. (2021) 21:855. doi: 10.1186/s12879-021-06536-3
16. Hosseinabadi R, Biranvand S, Anbari K. Adherence to preventive behaviours and risk of COVID-19 infection: a case-control study. Medicinski glasnik: official publication of the Medical Association of Zenica-Doboj Canton. Bosnia Herzegovina. (2022) 19:22. doi: 10.17392/1490-22
17. Rovetta A. Health communication is an epidemiological determinant: Public health implications for COVID-19 and future crises management. Health Promot Perspect. (2022) 12:226–8. doi: 10.34172/hpp.2022.28
18. Zang ST, Luan J, Li L, Yu HX, Wu QJ, Chang Q, et al. Ambient air pollution and COVID-19 risk: evidence from 35 observational studies. Environ Res. (2022) 204(Pt B):112065. doi: 10.1016/j.envres.2021.112065
19. Liu X, Huang J, Li C, Zhao Y, Wang D, Huang Z, et al. The role of seasonality in the spread of COVID-19 pandemic. Environ Res. (2021) 195:110874. doi: 10.1016/j.envres.2021.110874
20. Fontal A, Bouma MJ, San-José A, López L, Pascual M, Rodó X. Climatic signatures in the different COVID-19 pandemic waves across both hemispheres. Nat Comput Sci. (2021) 1:655–65. doi: 10.1038/s43588-021-00136-6
21. Scabbia G, Sanfilippo A, Mazzoni A, Bachour D, Perez-Astudillo D, Bermudez V, et al. Does climate help modeling COVID-19 risk and to what extent? PLoS ONE. (2022) 17:e0273078. doi: 10.1371/journal.pone.0273078
22. Weaver AK, Head JR, Gould CF, Carlton EJ, Remais JV. Environmental Factors Influencing COVID-19 Incidence and Severity. Annu Rev Public Health. (2022) 43:271–91. doi: 10.1146/annurev-publhealth-052120-101420
23. Iezadi S, Gholipour K, Azami-Aghdash S, Ghiasi A, Rezapour A, Pourasghari H, et al. Effectiveness of non-pharmaceutical public health interventions against COVID-19: a systematic review and meta-analysis. PLoS ONE. (2021) 16:e0260371. doi: 10.1371/journal.pone.0260371
24. Guzzetta G, Riccardo F, Marziano V, Poletti P, Trentini F, Bella A, et al. Impact of a nationwide lockdown on SARS-CoV-2 transmissibility, Italy. Emerg Infect Dis. (2021) 27:267–70. doi: 10.3201/eid2701.202114
25. Alfano V, Ercolano S. The efficacy of lockdown against COVID-19: a cross-country panel analysis. Appl Health Econ Health Policy. (2020) 18:509–17. doi: 10.1007/s40258-020-00596-3
26. Prati G, Mancini AD. The psychological impact of COVID-19 pandemic lockdowns: a review and meta-analysis of longitudinal studies and natural experiments. Psychol Med. (2021) 51:201–11. doi: 10.1017/S0033291721000015
27. Panda PK, Gupta J, Chowdhury SR, Kumar R, Meena AK, Madaan P, et al. Psychological and behavioral impact of lockdown and quarantine measures for COVID-19 pandemic on children, adolescents and caregivers: a systematic review and meta-analysis. J Trop Pediat. (2021) 67:fmaa122. doi: 10.1093/tropej/fmaa122
28. Bakaloudi DR, Barazzoni R, Bischoff SC, Breda J, Wickramasinghe K, Chourdakis M. Impact of the first COVID-19 lockdown on body weight: a combined systematic review and a meta-analysis. Clin Nutri. (2022) 41:3046–54. doi: 10.1016/j.clnu.2021.04.015
29. Yanovskiy M, Socol Y. Are lockdowns effective in managing pandemics? Int J Environ Res Public Health. (2022) 19:9295. doi: 10.3390/ijerph19159295
30. Sah P, Fitzpatrick MC, Zimmer CF, Abdollahi E, Juden-Kelly L, Moghadas SM, et al. Asymptomatic SARS-CoV-2 infection: a systematic review and meta-analysis. Proc Natl Acad Sci U S A. (2021) 118:e2109229118. doi: 10.1073/pnas.2109229118
31. Ma Q, Liu J, Liu Q, Kang L, Liu R, Jing W, et al. Global percentage of asymptomatic SARS-CoV-2 infections among the tested population and individuals with confirmed COVID-19 diagnosis: a systematic review and meta-analysis. JAMA Network Open. (2021) 4:e2137257. doi: 10.1001/jamanetworkopen.2021.37257
32. Itamura K, Wu A, Illing E, Ting J, Higgins T. YouTube videos demonstrating the nasopharyngeal swab technique for SARS-CoV-2 specimen collection: content analysis. JMIR Public Health Surveill. (2021) 7:e24220. doi: 10.2196/24220
33. Gadenstaetter AJ, Mayer CD, Landegger LD. Nasopharyngeal vs. nasal swabs for detection of SARS-CoV-2: a systematic review. Rhinology. (2021) 59:410–21. doi: 10.4193/Rhin21.162
34. Delli Compagni R, Cheng Z, Russo S, Van Boeckel TP. A hybrid Neural Network-SEIR model for forecasting intensive care occupancy in Switzerland during COVID-19 epidemics. PLoS ONE. (2022) 17:e0263789. doi: 10.1371/journal.pone.0263789
35. Roy TJ, Mahmood MA, Mohanta A, Roy D. An Analytical Approach to Predict the COVID-19 Death Rate in Bangladesh Utilizing Multiple Regression and SEIR Model. 2021 IEEE International Conference on Robotics, Automation, Artificial-Intelligence and Internet-of-Things (RAAICON), 42-45. Dhaka, Bangladesh. (2021). doi: 10.1109/RAAICON54709.2021.9929470
36. de Andres PL, de Andres-Bragado L, Hoessly L. Monitoring and forecasting COVID-19: Heuristic regression, susceptible-infected-removed model and, spatial stochastic. Front Appl Math Stat. (2021) 7:650716. doi: 10.3389/fams.2021.650716
37. Haq I, Hossain MI, Saleheen AAS, Nayan MIH, Mila MS. Prediction of COVID-19 pandemic in Bangladesh: dual application of susceptible-infective-recovered (SIR) and machine learning approach. Interdiscip Perspect Infect Dis. (2022) 2022:8570089. doi: 10.1155/2022/8570089
38. Greenland S. Hierarchical Regression for epidemiologic analyses of multiple exposures. Environ Health Perspect. (1994) 102(Suppl 8):33–39.
39. Lu X, Borgonovo E. Global sensitivity analysis in epidemiological modeling. Eur J Oper Res. (2023) 304:9–24. doi: 10.1016/j.ejor.2021.11.018
40. Haktanir A, Can N, Seki T, Kurnaz MF, Dilmaç B. Do we experience pandemic fatigue? current state, predictors, and prevention. Current Psychol. (2022) 41:7314–25. doi: 10.1007/s12144-021-02397-w
41. Bodas M, Kaim A, Velan B, Ziv A, Jaffe E, Adini B. Overcoming the effect of pandemic fatigue on vaccine hesitancy-Will belief in science triumph? J Nurs Scholar Sigma Theta Tau Int Honor Soc Nurs. (2023) 55:262–71. doi: 10.1111/jnu.12778
42. Taylor S, Rachor GS, Asmundson GJG. Who develops pandemic fatigue? Insights from latent class analysis. PLoS ONE. (2022) 17:e0276791. doi: 10.1371/journal.pone.0276791
43. Rovetta A, Castaldo L. Are we sure we fully understand what an infodemic is? A global perspective on infodemiological problems. JMIRx Med. (2022) 3:e36510. doi: 10.2196/36510
Keywords: COVID-19, epidemiology - analytic (risk factors), public health, public health policies, epidemic determinants, confounding (epidemiology)
Citation: Rovetta A (2023) There is a need for more precise models to assess the determinants of health crises like COVID-19. Front. Public Health 11:1179261. doi: 10.3389/fpubh.2023.1179261
Received: 03 March 2023; Accepted: 31 May 2023;
Published: 15 June 2023.
Edited by:
Sukhyun Ryu, Konyang University, Republic of KoreaReviewed by:
Mazin Mohammed, University of Anbar, IraqCopyright © 2023 Rovetta. This is an open-access article distributed under the terms of the Creative Commons Attribution License (CC BY). The use, distribution or reproduction in other forums is permitted, provided the original author(s) and the copyright owner(s) are credited and that the original publication in this journal is cited, in accordance with accepted academic practice. No use, distribution or reproduction is permitted which does not comply with these terms.
*Correspondence: Alessandro Rovetta, cm92ZXR0YS5tcmVzZWFyY2hAZ21haWwuY29t
†ORCID: Alessandro Rovetta orcid.org/0000-0002-4634-279X
Disclaimer: All claims expressed in this article are solely those of the authors and do not necessarily represent those of their affiliated organizations, or those of the publisher, the editors and the reviewers. Any product that may be evaluated in this article or claim that may be made by its manufacturer is not guaranteed or endorsed by the publisher.
Research integrity at Frontiers
Learn more about the work of our research integrity team to safeguard the quality of each article we publish.