- 1Department of Civil and Environmental Engineering, Michigan State University, East Lansing, MI, United States
- 2Oakland County Health Division, Pontiac, MI, United States
- 3Wayne State University School of Medicine, Detroit, MI, United States
- 4Great Lakes Water Authority, Detroit, MI, United States
Introduction: Throughout the coronavirus disease 2019 (COVID-19) pandemic, wastewater surveillance has been utilized to monitor the disease in the United States through routine national, statewide, and regional monitoring projects. A significant canon of evidence was produced showing that wastewater surveillance is a credible and effective tool for disease monitoring. Hence, the application of wastewater surveillance can extend beyond monitoring SARS-CoV-2 to encompass a diverse range of emerging diseases. This article proposed a ranking system for prioritizing reportable communicable diseases (CDs) in the Tri-County Detroit Area (TCDA), Michigan, for future wastewater surveillance applications at the Great Lakes Water Authority's Water Reclamation Plant (GLWA's WRP).
Methods: The comprehensive CD wastewater surveillance ranking system (CDWSRank) was developed based on 6 binary and 6 quantitative parameters. The final ranking scores of CDs were computed by summing the multiplication products of weighting factors for each parameter, and then were sorted based on decreasing priority. Disease incidence data from 2014 to 2021 were collected for the TCDA. Disease incidence trends in the TCDA were endowed with higher weights, prioritizing the TCDA over the state of Michigan.
Results: Disparities in incidences of CDs were identified between the TCDA and state of Michigan, indicating epidemiological differences. Among 96 ranked CDs, some top ranked CDs did not present relatively high incidences but were prioritized, suggesting that such CDs require significant attention by wastewater surveillance practitioners, despite their relatively low incidences in the geographic area of interest. Appropriate wastewater sample concentration methods are summarized for the application of wastewater surveillance as per viral, bacterial, parasitic, and fungal pathogens.
Discussion: The CDWSRank system is one of the first of its kind to provide an empirical approach to prioritize CDs for wastewater surveillance, specifically in geographies served by centralized wastewater collection in the area of interest. The CDWSRank system provides a methodological tool and critical information that can help public health officials and policymakers allocate resources. It can be used to prioritize disease surveillance efforts and ensure that public health interventions are targeted at the most potentially urgent threats. The CDWSRank system can be easily adopted to geographical locations beyond the TCDA.
1. Introduction
Since the beginning of the coronavirus disease 2019 (COVID-19) pandemic, wastewater surveillance has been consistently applied to monitor severe acute respiratory syndrome coronavirus 2 (SARS-CoV-2) viral RNA worldwide (1–10). Wastewater surveillance epidemiology is a translation of the theory that human wastewater can serve as a representative community-composite sample to monitor fluctuations of disease incidence. A pathogen that can be detected in bodily fluids, including excreta, urine, sputum, and saliva, has the potential to be detected and thus, monitored (2, 11–14). Wastewater surveillance and epidemiology has a diverse range of benefits, including (1) circumventing the need for mass clinical testing, (2) conserving health, economic, and societal resources, (3) providing unbiased and unspecific monitoring of disease incidence regardless of symptomatic or asymptomatic conditions, and (4) providing early warnings of impending disease surges (4, 5, 7, 10, 12, 15). Wastewater surveillance has been extraordinarily successful at monitoring multiple pathogens, including SARS-CoV-2 (2, 4–7, 11, 16), hepatitis A and hepatitis E (17), herpesviruses (18), poliovirus (19, 20), and others. Despite its great potential, most wastewater disease monitoring to date has been limited to SARS-CoV-2. Notably, recent exceptions encompass poliovirus (21) and monkeypox virus (22–24). Thus, it is paramount that the adoption and integration of this scientifically-validated methodology is accelerated, particularly among emerging disease, neglected disease, or diseases of high outbreak potential.
Communicable diseases (CDs), for instance, tuberculosis (TB) and sexually transmitted infections (STIs), are among the leading causes of death and disability worldwide, according to the WHO (who.int). CDs are caused by microorganisms including bacteria, viruses, fungi, or various parasites that can be transmitted widely and quickly within human populations (25). Some infectious diseases are transmitted through “bites” from insect vectors, while others can be caused by ingesting contaminated food or water (who.int). The WHO, U.S. NIH, U.S. AID, U.S. CDC, and the international scientific community has long recognized the need to develop a comprehensive education, prediction, and prevention system for CDs (13, 26, 27).
A few studies have developed methodologies for ranking CD threats to the public (28–30). However, these systems have limitations and cannot be directly used by local health department to make decisions regarding appropriate targets for wastewater surveillance. Briefly, they relied heavily on subjective assessments of weights given by experts to multiple parameters. They were lacking critical quantitative information such as incidence of diseases based on clinical data, and basic reproduction numbers of CDs. Besides, most parameters were assigned a value according to the Delphi Method, which consists of gathering expert opinions to weight a disease on a parameter then multiplied by a scale of numbers such as 1–5 (29) or 0–7 (31) in terms of level of importance.
The objective of this study is to develop a comprehensive communicable disease ranking system (“CDWSRank” system) that prioritizes CDs for wastewater surveillance (Figure 1). To this end, we investigated 96 CDs in the Tri-County Detroit Area (TCDA), Michigan, Unites States, reported through the Michigan Disease Surveillance System (MDSS). All CDs were ranked through the CDWSRank system, which involved 2 categories of parameter: binary and quantitative. Binary parameters examine the presence or absence of CDs in the following inventories: (1) CDC National Notifiable Infectious Disease and Conditions List (NNIDCL), (2) Michigan Department of Health and Human Services (MDHHS) Weekly Disease Report, (3) EPA Contaminant Candidate List (CCL), (4) CDC bioterrorism agents list, (5) pathogen's detectability in wastewater or excreta, and (6) association of disease with single or multiple pathogens. Quantitative parameters include: (1) clinical case trend in Michigan, (2) clinical case trend in the TCDA, (3) ratio of clinical case incidence between Michigan and the TCDA (geographic ratio), (4) annual clinical cases in Michigan, (5) annual clinical cases in the TCDA, and (6) the R0 (basic reproduction number) of the disease.
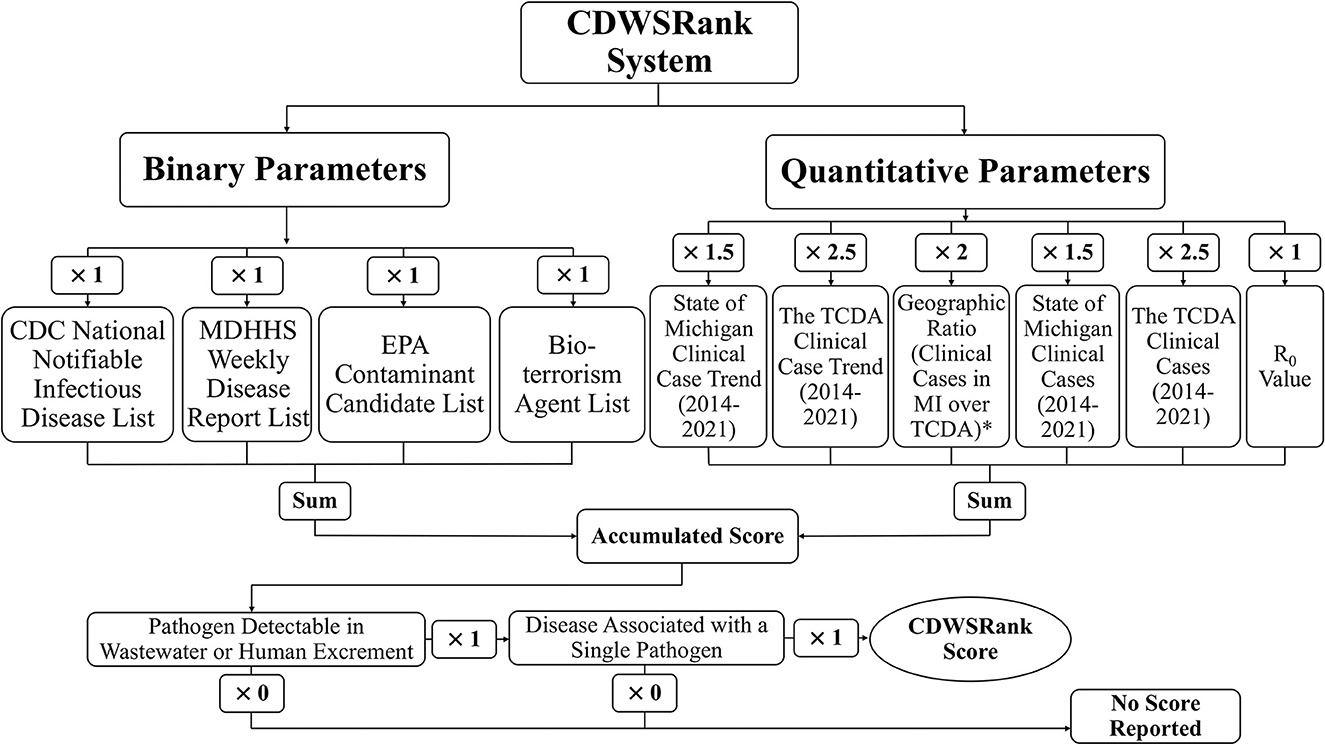
Figure 1. Overall schematic of CDWSRank system. *marked parameter indicates that the annual caseload for Michigan from 2014-2021 was divided by the annual caseload for the TCDA, where the average of those was then taken for downstream analyses. The A+1 value was assigned to those CDs with an average ratio of <1.
The CDWSRank system is one of the first of its kind to provide an empirical method for selecting CDs for wastewater surveillance, in geographies serviced by centralized wastewater collection and treatment. To demonstrate the importance of site-specific ranking, CD trends were analyzed for both the TCDA and Michigan as a whole for the period between 2014 and 2021. This manuscript will moreover summarize wastewater sampling methods based on pathogen type. Ultimately, this article should contribute to the reduced impact of CDs by procuring valuable information for public health practitioners, researchers, and medical professionals.
2. Materials and methods
2.1. Communicable disease data acquisition
Weekly reports from the MDSS between 2014 and 2021 were accessed from the MDHHS website (michigan.gov/mdhhs). Data in the weekly reports were provisional, based on current data at the time that the report was published. Communicable disease incidence (per 100,000) for the state of Michigan are shown in Figure 2. Similar data was collected for the TCDA, including City of Detroit, and Wayne, Macomb, and Oakland Counties. Examples of disease trends between 2014 and 2017 are shown in Figures 3–6. MDSS weekly disease reports define the epidemiological “week” in concurrence with the CDC's Morbidity and Mortality Weekly Report (MMWR) (cdc.gov/mmwr), which runs from Sunday (day 1) to Saturday (day 7). All CDs were cross-referenced against multiple regulatory lists including the U.S. CDC's NNIDCL (cdc.gov/nndss), the U.S. EPA's CCL (epa.gov/ccl), and the U.S. CDC's bioterrorism agents list (cdc.gov/bioterrorism). Additionally, the detectability of the pathogens associated with each CD in human excreta and wastewater, which is crucial evidence for the applicability of wastewater surveillance for monitoring CDs, was investigated through an extensive literature review (Tables 1–4). R0′s were also collected through a literature review and are summarized in Table 5.
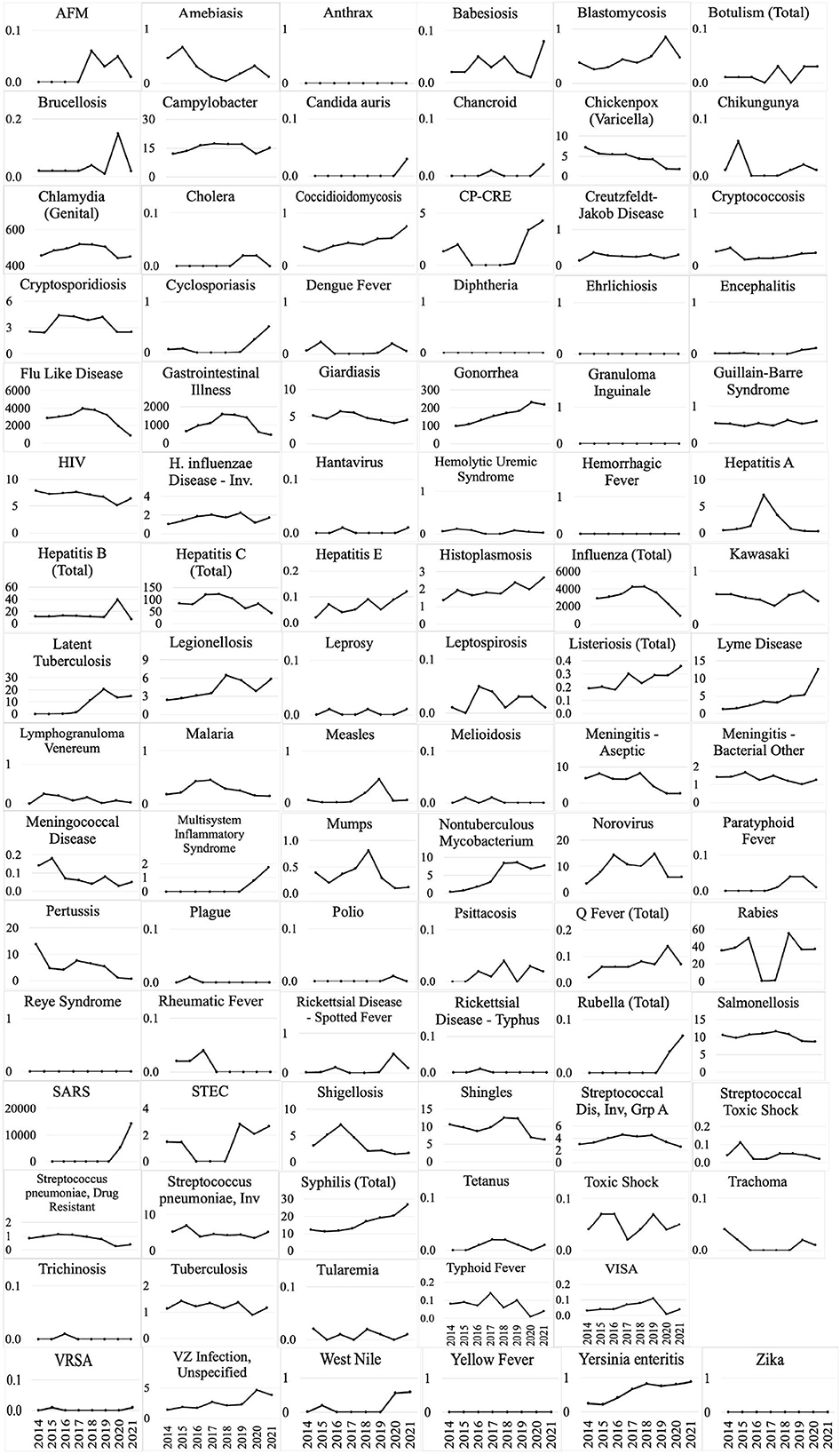
Figure 2. Disease incidence (per 100,000) for 95 CDs between 2014 and 2021 in the state of Michigan (Disease incidence for Monkeypox was unavailable during this period).
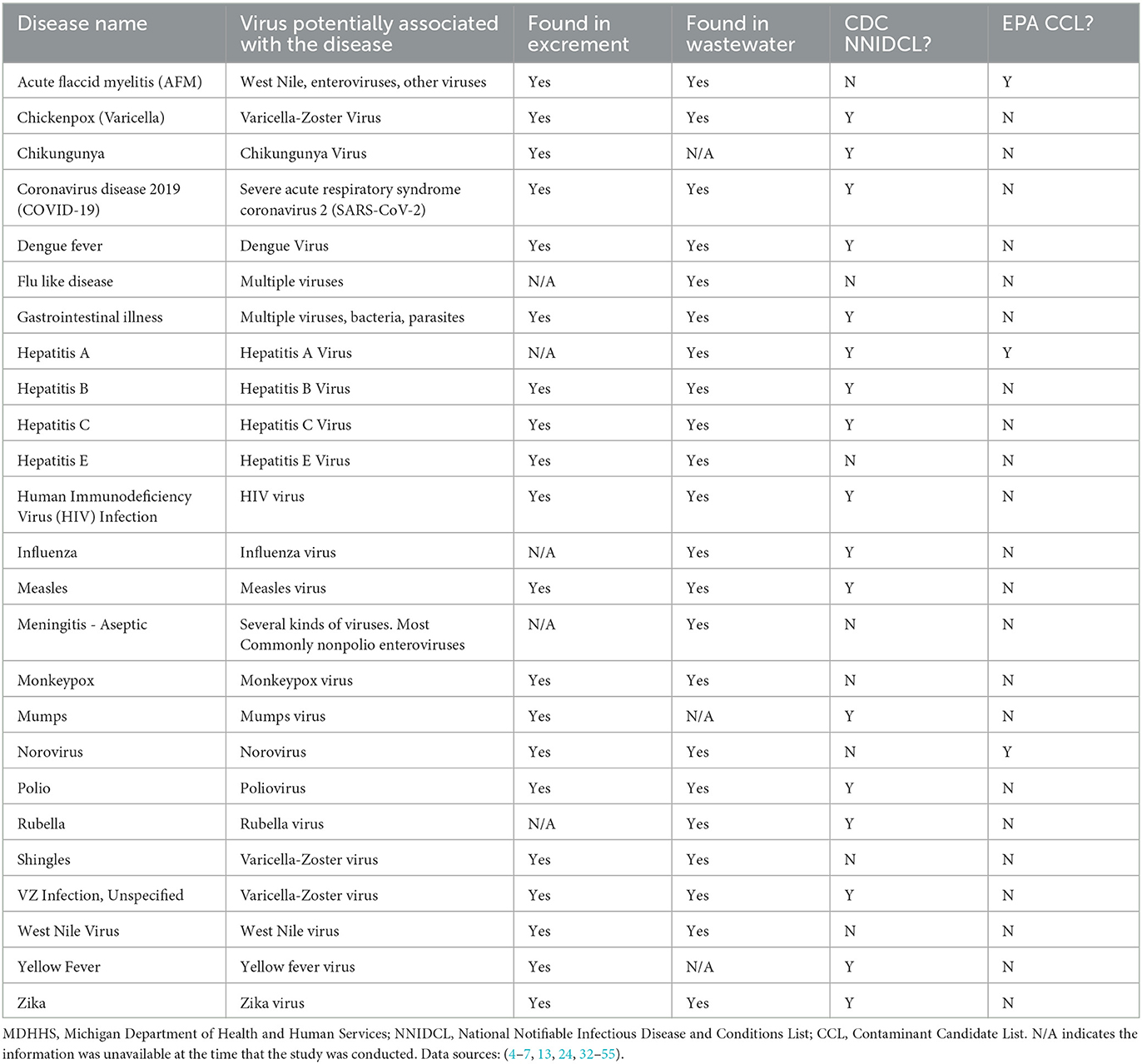
Table 1. MDHHS-reported conditions associated with viruses that can potentially be monitored with wastewater surveillance.
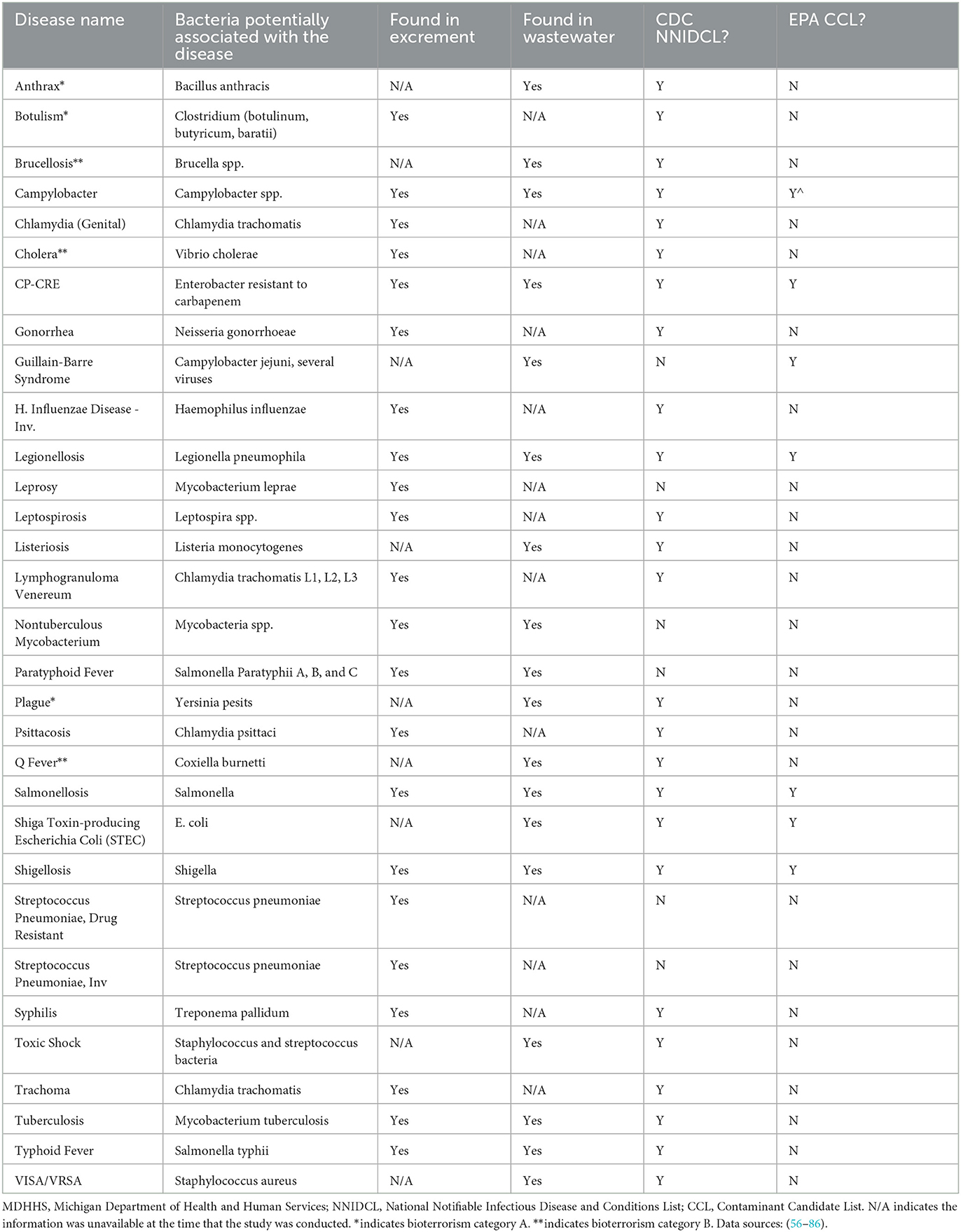
Table 2. MDHHS-reported conditions associated with bacteria that can potentially be monitored with wastewater surveillance.
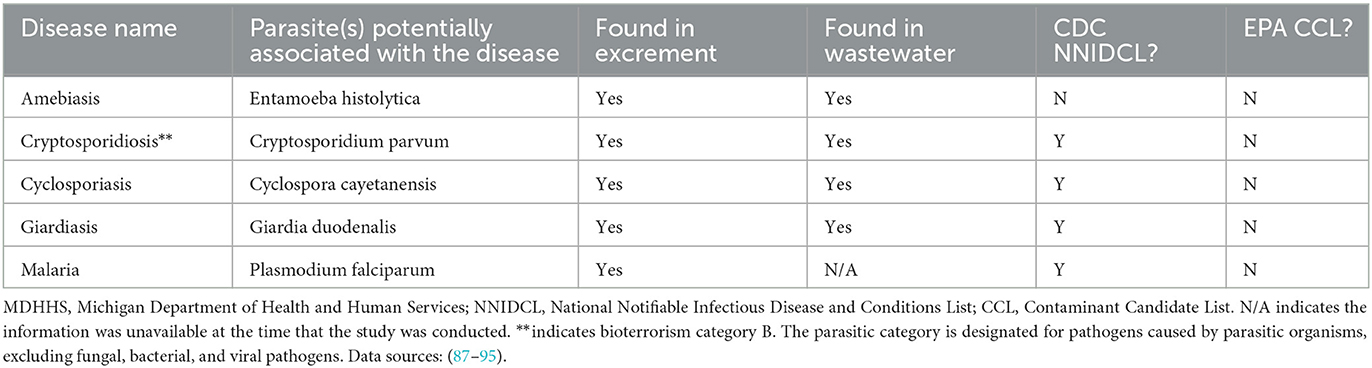
Table 3. MDHHS-reported conditions associated with parasites that can potentially be monitored with wastewater surveillance.

Table 4. MDHHS-reported conditions associated with fungi that can potentially be monitored with wastewater surveillance.
2.2. The CDWSRank system
The following sections demonstrate the design of the CDWSRank system and its associated parameters. The presence and absence of all CDs in regulatory lists including NNIDCL, WDR, and CCL, as well as being described as a bioterrorism agent, the association of the disease with a single or multiple pathogens, and detectability of pathogens in human wastewater were modeled as binary parameters. Quantitative parameters include: (1) clinical case trend in Michigan, (2) clinical case trend in the TCDA, (3) ratio of clinical case incidence between Michigan and the TCDA (geographic ratio), (4) annual clinical cases in Michigan, (5) annual clinical cases in the TCDA, and (6) the R0 (basic reproduction number) of the disease. The overall schematic of the parameters and weighting factors of each parameter is presented in Figure 1.
2.2.1. Binary parameters
The presence or absence of CDs for each binary parameter was treated as a ×1 weighting factor (multiplier) and ×0 weighting factor (multiplier), respectively, which were then summed for the final ranking score. The CDC's NNIDCL provides comprehensive reporting of CDs that occur in the USA. Diseases that are reported in the NNIDCL are considered notifiable, but whether or not they are reported at the state level, varies (cdc.gov). Furthermore, internationally notifiable diseases reported in WHO's International Health Regulations (IHR), such as cholera, are also reportable in NNIDCL (cdc.gov). The IHR covers not only CDs but also other public health concerns including chemical and radiological threats (cdc.gov). All CDs were assessed for whether they are listed on the CDC's NNIDCL, and the corresponding presence or absence was marked with “Y” (presence in NNIDCL) or “N” (absence in NNIDCL). A multiplier of 1 was assigned to any CD's presence on NNIDCL. Similarly, the presence of a CD in the MDHHS Weekly Disease Report (WDR) was given a weighting factor or “multiplier” of 1.
The EPA's CCL includes drinking water contaminants that are recognized or expected to occur in public water systems and are not currently subject to EPA drinking water regulations (epa.gov). The EPA uses the CCL to identify priority contaminants for regulatory decision-making and information gathering (epa.gov). The EPA announced Draft CCL 5 on July 19, 2021, followed by the publication of Final CCL 5 on November 14, 2022 (epa.gov). All CDs were assessed for whether they appear on EPA CCL 5, and the corresponding presence or absence was marked with a “Y” (presence in CCL) or “N” (absence in CCL).
The CDC classifies bioterrorism agents into 3 categories, namely, A, B, and C, depending, primarily, on how easily the diseases can be transmitted and the severity of illness (cdc.gov). Agents in category A are considered of the highest risk, as they can be easily transmitted within human populations and can result in high death rates and significant public health impacts. Examples include anthrax and plague. Agents in category B have the second highest priority risk, as they are moderately easy to spread and can result in moderate morbidity rates. Examples include Q fever and typhus fever. Agents in category C are considered the third highest priority risk and they can easily spread among humans and cause health impacts (cdc.gov). Examples include hantavirus and Nipah virus. The presence of CDs as CDC-defined bioterrorism agents was marked with “*” for category A and “**” for category B. A weighting factor or multiplier of 1 was assigned to a CD listed as a CDC bioterrorism agent, regardless of category.
The detectability of pathogens causative of CDs in human wastewater is crucial to the successful implementation of wastewater surveillance. Following extensive literature reviews, the detectability of the causative pathogen in excreta or wastewater was marked with a “Y” (detectable), “N” (non-detectable), or “N/A” (data unavailable) in Tables 1–4. For the final ranking score, a multiplier of 1 or 0 was given to CDs with a causative pathogen that is detectable or non-detectable, respectively, in excreta and/or wastewater.
The binary parameter of disease associated with single or multiple pathogens considers the exact source of a causative pathogen of a CD. In this system, CDs with multiple causative pathogens would make them nearly impossible to be determined or detected. Therefore, CDs with multiple causative pathogens were assigned a multiplier of 0 at the final ranking score, to moderate the over-ranking of these CDs. A final ranking multiplier of 1 was assigned to CDs with a single causative pathogen.
2.2.2. Quantitative parameters
Quantitative parameters include: (1) clinical case trend in Michigan, (2) clinical case trend in the TCDA, (3) ratio of clinical case incidence between Michigan and the TCDA (geographic ratio), (4) annual clinical cases in Michigan, (5) annual clinical cases in the TCDA, and (6) the R0 (basic reproduction number) of the disease. Clinical case trends in Michigan as a whole and in the TCDA specifically, were determined by calculating the correlation R-value between disease incidence (per 100,000) each year (2014 to 2021) and the given year, for all CDs. The weighting factor or multiplier of 1.5 and 2.5 were assigned to clinical case trends in Michigan and the TCDA, respectively, providing greater emphasis on the TCDA.
The ratio of clinical case incidence between Michigan and the TCDA is assessed through calculating case incidence (per 100,000) for each CD, for the state of Michigan, then the TCDA. Next, the ratio of these values is calculated as the quotient of Michigan cases and TCDA cases, done for each year in the study period. Finally, the average of annual ratios was calculated, and each CD was assigned a value of 1 if the average was less than 1 (indicating that the CD was more prevalent in the TCDA than the state of Michigan as a whole). A CD was assigned a value of 0 if the ratio was equal to, or greater than 1. A weighting factor or “multiplier” of 2 was given to this metric.
Clinical cases in Michigan and in the TCDA were determined by computing the decadic log of the average clinical caseload for the years studied. Taking the common logarithm was necessary as clinical caseloads varied greatly in magnitude; this operation, therefore, allowed for the comparison of CDs even with disparate magnitudes of caseloads, while still preserving accurate variation measures. The weighting factor or “multiplier” of 1.5 and 2.5 were assigned to clinical cases in Michigan and the TCDA, respectively, providing greater emphasis on the TCDA.
The R0 of CDs were determined through literature investigation (Table 5). This parameter was included to increase the ranking score of CDs that can be transmitted efficiently, through person-to-person contact (167). This parameter prioritizes CDs that have the potential to spread rapidly. This parameter was given a weighting factor of 1.
2.2.3. Overall CDWSRank system ranking score
An overall ranking score (RCD) of the CDWSRank system for CDs is calculated using the following Eq. (1), where RCD is the overall ranking score of the ith CD, Wi is the weighting factor for binary parameters, Ni is the weighting factor for quantitative parameters, Bi represents binary parameters, Qi represents quantitative parameters, Di represents the detectability of causative pathogens in human excreta or wastewater, and Mi represents the association of a CD with a single or multiple pathogens.
An equation for calculating an overall rank score of the ith CD with all binary and quantitative parameters displayed, can be expressed as follows:
RCD = [1 × (NNIDCL) + 1 × (WDR) + 1 × (CCL) + 1 × (Bioterrorism) + 2 × (Geographic ratio) + 1.5 × (Clinical case trend in Michigan) + 2.5 × (Clinical case trend in the TCDA) + 1.5 × (Clinical case in Michigan) + 2.5 × (Clinical case in TCDA) + 1 × (R0)] × [1 × (Detectability in human excreta or wastewater)] × [1 × (Association of disease with single or multiple pathogens)] (2)
For example, an overall rank score of SARS-CoV-2 can be computed as: [1 × (1) + 1 × (1) + 1 × (0) + 1 × (0) + 2 × (1) + 1.5 × (0.57) + 2.5 × (0.6) + 1.5 × (5.39) + 2.5 × (4.98) + 1 × (2.11)] × (1) × (1) = 29.
2.3. Wastewater surveillance concentration methods based on pathogen type
In addition to the development of the CDWSRank system, a comprehensive literature review was conducted to summarize appropriate wastewater sample concentration surveillance methods based pathogen type, namely: bacterial, fungal, parasitic, and viral (Table 6).
3. Results
3.1. Classification of CDs
Tables 1–3 present viruses, bacteria, parasites and fungi that are detectable in human excrement or wastewater, indicating their potential to be monitored by wastewater surveillance. Notably, some of the listed pathogens were successfully detected in worldwide wastewater samples, with disease incidence monitored using wastewater surveillance. These include dengue virus (32), hepatitis B (33), monkeypox virus (22–24), norovirus (168, 169), Poliovirus (19, 20), SARS-CoV-2 (2, 4–7, 10, 16), yellow fever virus, and zika virus (32).
Twenty-five CDs are associated with viral pathogens, including chickenpox, COVID-19, monkeypox, norovirus, West Nile fever and so on (Table 1). The viruses that are associated with the diseases are also summarized in Table 1. For instance, varicella-zoster virus is the causative agent of chickenpox. Notably, only 3 of the 25 viruses, including acute flaccid myelitis-related enterovirus, hepatitis A, and norovirus, appear on the EPA's CCL. Some viral diseases can be found on the CDC's NNIDCL, including COVID-19, HIV/AIDS, and Zika. No viral CDs in the list are classified as CDC bioterrorism agents. Table 2 shows 31 CDs associated with bacterial pathogens, including anthrax, cholera, gonorrhea, plague, syphilis, and so forth. The bacteria that are potentially associated with the diseases were also summarized in Table 2. For instance, clostridium (botulinum, butyricum, baratii) is the potential causative agent associated with botulism. Seven of the 31 bacteria are listed on the EPA's CCL, including chlamydia, CP-CRE, Guillain-Barre syndrome, legionellosis, salmonellosis, STEC, and shigellosis. And 25 of the 31 of the bacterial-related CDs are listed on the CDC's NNIDCL. Six of 31 bacterial-related CDs are not listed on the CDC's NNIDCL, including Guillain-Barre syndrome, leprosy, non-tuberculous mycobacterium, paratyphoid fever, and streptococcus pneumoniae. Among all bacterial CDs, anthrax, botulism, and plague are listed in bioterrorism category A, while brucellosis, cholera, and Q fever are listed in bioterrorism category B. Table 3 includes 5 parasitic CDs that can be detected in either human excreta or wastewater. The potentially causative agents of these diseases were also summarized in Table 3. For instance, cryptosporidium parvum is the parasite associated with cryptosporidiosis. None of pathogens related to parasitic CDs are listed on the EPA's CCL, and 4 of them are listed on the CDC's NNIDCL, including cryptosporidiosis, cyclosporiasis, giardiasis, and malaria, expect for amebiasis. Cryptosporidiosis is listed in the CDC's bioterrorism category B. Lastly, Table 4 shows 3 fungal-related CDs, including blastomycosis, cryptococcosis, and candidiasis. The fungi associated with the diseases are summarized in Table 4. For instance, blastomyces dermatitidis and gilchristii are the potential causes of blastomycosis. None of them are listed on the EPA's CCL and only candidiasis (candida auris) was listed on the CDC's NNIDCL (Table 4).
3.2. Observations of CDs' incidence and trend
3.2.1. Comparison of CD incidence in the TCDA vs. the state of Michigan
All CD incidences (per 100,000) from 2014 to 2021 in Michigan are demonstrated in Figure 2. Influenza, “influenza-like” or “flu-like” diseases, chlamydia, gonorrhea, and gastrointestinal illness (GI) have among the highest average incidences in Michigan.
Notably, multiple CDs presented lower incidences (per 100,000) in the TCDA than in broader Michigan (Figure 3). GI presented much higher cases per 100,000 in Michigan than in TCDA. Between 2017 and 2019, more than 1,400 incidences per 100,000 were observed in Michigan. In contrast, during the same period, approximate 400 incidences per 100,000 were observed in TCDA (Figure 3). Likewise, incidences per 100,000 of cryptosporidiosis, giardiasis, and norovirus were observed as much as twice higher in Michigan than in TCDA.
On the contrary, multiple CDs presented higher incidences (per 100,000) in the TCDA than in broader Michigan (Figure 4). CDs, such as gonorrhea, which can cause severe and permanent health issues (cdc.gov), has increased continuously and dramatically from 5,245 cases in 2014 to 12,034 cases in 2020 (and slightly decreased to 10,483 cases in 2021) in the TCDA. Gonorrhea incidence in TCDA is approximately five times higher than the rest of Michigan (Michigan.gov). Likewise, sextually transmitted diseases such as HIV, syphilis, and chlamydia were observed with consistent higher incidences per 100,000 in TCDA than in statewide Michigan (Figure 4). Also, West Nile fever incidences per 100,000 have increased dramatically in TCDA from 2019 to 2020 (Figure 4).
Figure 5 demonstrates selected CDs with approximately the same disease incidence (per 100,000), between the TCDA and Michigan, including AFM, brucellosis, Guillain-Barre syndrome, hepatitis E and C, as well as shigellosis.
3.2.2. Potential impact of COVID-19 pandemic on CDs
Multiple CDs were potentially affected by the COVID-19 pandemic (Figure 6). For instance, cases of hepatitis B surged from 675 (Michigan) and 1,081 (TCDA) in 2019, to 3,064 (Michigan) and 4,007 (TCDA) in 2020, during the inchoate stages of the COVID-19 pandemic. Afterwards, incidences in both Michigan and the TCDA decreased significantly, during COVID-19 stabilization, suggesting that a pandemic could cause an impact on disease incidence. The pandemic also affected the incidence of several vector-borne diseases, for example Lyme disease. Lyme disease surged in both Michigan as a whole and the TCDA between 2020 and 2021 (Figure 6). The incidence of influenza per 100,000 individuals in both TCDA and Michigan has been consistently decreasing since 2018. However, the decrease has been particularly significant from 2020 to 2021, concurring with the global spread of COVID-19. This may suggest that the health control measures implemented in response to the COVID-19 pandemic, such as shelter-in-place orders and social distancing, have had a positive impact on reducing the incidence of influenza.
3.3. Overall ranking
Figure 7 presents the final ranking (top 30 out of 96 CDs) generated from the CDWSRank system, prioritizing wastewater surveillance target applications in the TCDA. Several CDs caused by viruses that are detectable in human excreta or wastewater were among the top 30 listed. These include COVID-19 (ranked 1st), hepatitis B (ranked 2nd), measles (ranked 3rd), influenza (ranked 6th), hepatitis C (ranked 8th), polio (ranked 18th), HIV/AIDS (ranked 19st), hepatitis E (ranked 21st), and norovirus (ranked 27th). Among the top 30 ranked CDs, some did not present relatively high incidences but were prioritized upon using the CDWSRank system. Examples include measles, polio, HIV/AIDS, hepatitis E, and norovirus, suggesting that such CDs require significant attention by wastewater surveillance practitioners, despite their relatively low incidence rates in the geographic study area in recent years.
Though not unexpected, the highest ranked CDs are those that do not spread solely by direct contact with animals, but rather those that are transmitted from person to person or from food or fomites. Only one vector-borne disease appears within the top 30, which is West Nile fever (ranked 29th). Over 50% (16/30) of CDs in the top 30 are either foodborne or STIs.
It is worth noting that 4 of the top 30 ranked CDs are known to health agencies to be vaccine preventable, highlighting the need for surveillance to warn against conditions that are not easily preventable, or those that could be particularly devastating to those not able to be immunized, such as infants or the immunodeficient. One CD ranked by this system was assigned a negative RCD, melioidosis. This indicates that, though detectable using wastewater surveillance methods, this disease has been trending downward in the geographic areas and timeframe of this study, precluding it as a priority for monitoring.
Additionally, certain CDs (mentioned in Sections 3.2.1 and 3.2.2) received a ranking score of 0 since a multiplier of 0 for binary parameters was assigned. Lyme disease, for example, received a score of 0 since the detectability of Lyme disease in excreta or wastewater was set to 0. It was set to 0 because at the time of this study there were no published reports available indicating the ability to detect the bacteria (Borrelia burgdorferi and Borrelia mayonii) that causes Lyme disease in excreta or wastewater. As research efforts of the scientific community progress this may change.
4. Discussion
4.1. Differences of CDs in TCDA and state of Michigan
Differences in incidence among CDs in the TCDA vs. the state of Michigan demonstrate epidemiological trends that differ, possibly due to population density, wildlife/ecology, climate, socioeconomic and racial inequities, cultural or behavioral differences, age distribution, and access to healthcare and/or medical insurance (170–174). The ranking system results focus on TCDA which is an urban area with high-density population. However, as of 2021 (175), approximately 1.8 million residents, which accounts for nearly 20 percent of Michigan's population, live in rural areas. Consequently, Michiganders as a whole face a relatively elevated risk of contracting CDs such as cryptosporidiosis, giardiasis, and norovirus (Figure 3).
Residents in rural areas may have limited accessibility to medical care for diseases that require extensive or sophisticated care regimens (176). A study demonstrated possible causes for disparities between urban and rural areas by comparing outdoor time, where longer outdoor time were spent by rural residents than their urban counterparts (174), potentially creating an elevated risk of being infected by zoonotic pathogens. In rural areas, zoonotic diseases are of particular concern for farm workers, especially those working with livestock (177). In addition to zoonotic disease, residents of rural areas of Michigan are of great concern for vector-borne diseases, such as babesiosis (Figure 3), and others (178). It is important to note that human behavior, such as water related human activities, can also impact the transmission of vector-borne diseases, in addition to the effects of a warming climate in Michigan, especially the TCDA area (179). For example, higher average incidence of West Nile fever in the TCDA than in statewide Michigan can be attributed to both factors (180, 181).
Multiple CDs presented higher incidences per 100,000 in TCDA than in statewide Michigan, such as HIV and syphilis. This could possibly be related to a limited access to healthcare among the socioeconomically disadvantaged and racial minorities in TCDA (182). There are multiple causes of higher disease incidence of HIV and other STIs in TCDA, such as gonorrhea and syphilis (Figure 4). Briefly, a recent investigation indicated that elevated HIV prevalence in the TCDA was associated with minorities, gay and bisexual populations up to 29 years old, and the socioeconomically disadvantaged, such as those experiencing homelessness, poverty, and unemployment (170). It is worth noting that this trend is observed nationwide (183, 184). Researchers have also found that TCDA had a TB incidence twice than that of Michigan, affected by both racial inequity and places of interaction (185).
4.2. Impact of COVID-19 pandemic on CDs in TCDA and state of Michigan
Incidences per 100,000 of diseases such as hepatitis B, influenza and others, in both Michigan and the TCDA changed significantly, during COVID-19 inception, suggesting that a pandemic could cause an impact on disease incidence (Figure 7). This was corroborated in recent studies (186–188), and has been shown in countless epidemics worldwide (189, 190). Interestingly, several CDs whose incidences fell during the pandemic were those that traditionally rose in the other reported years, such as influenza. It is likely that reduced human contact and heightened hygiene in response to COVID-19 may have caused the dramatic decrease (191). On the contrary, Lyme disease surged in both Michigan as a whole and the TCDA between 2020 and 2021 (Figure 6). This may be attributable to an increasing number of outdoor recreational activities as result of diminished indoor options, due to COVID-19 social distancing restrictions (192). Another potential explanation for the pandemic's effect on CD incidence is that some CDs are caused by opportunistic pathogens that reactivate in a host when an individual's immune response is weakened, often by another pathogenic condition (193). The renewed prevalence of these CDs can be a direct effect of COVID-19 disease, creating the conditions of pathogen reactivation or new infections (194).
Studies have investigated the impact of the COVID-19 pandemic on sexually transmitted infections (STIs), such as syphilis (Figure 4) (195, 196). The disease incidence (per 100,000) of syphilis increased significantly between 2020 and 2021 in both the TCDA and broader Michigan, amid the pandemic (Figure 4). Potential causes may include the diversion of funding and health resources from STI programs, shutdown of STI clinics, less available treating physicians, a reticence to appear in-office to meet clinicians, and longer laboratory turnaround times (195). It is worth noting that during the COVID-19 pandemic, many health reporting systems faced challenges due to the increased workload and limited resources in the public health workforce (197). This may have led to delays in reporting some diseases or with lower-quality data. However, it is important to note that COVID-19 has also resulted in improvements in health reporting systems in some areas, as public health agencies and governments have recognized the importance of timely and accurate reporting of disease data (198). The impact on health reporting systems by COVID-19 pandemic varied depending on the region, the disease, and the public health response to the pandemic.
4.3. Wastewater surveillance for viral CDs
CDWSRank placed 16 viral CDs in the top 30 for wastewater surveillance (Figure 7). Hepatitis B, for example, ranked 2nd (Figure 7). Recently, researchers conducted wastewater surveillance to monitor hepatitis B in 19 cities across China, after clinical cases had increased dramatically (33). The wastewater surveillance results were consistent with the prevalence reported in surveys, indicating that estimating Hepatitis B prevalence through wastewater surveillance is feasible in large cities in Southern China. Hepatitis C ranked 8th in CDWSRank for the TCDA region. Its RNA was detected and quantified in human fecal specimens in multiple studies, suggesting a significant potential for using wastewater monitoring as a tool for detecting hepatitis C virus (199). Chickenpox (ranked 11th) has been persistent in the statewide Michigan between 2014 and 2021, as shown in Figure 2. A few studies have attempted to test human bodily fluids, particularly urine, for monitoring varicella-zoster virus (which causes chickenpox and shingles), and other similar pathogens, such as in the Poxviridae family (34, 200). Notably, belonging to the same orthopoxvirus genus as varicella-zoster (201), the monkeypox virus has been spreading worldwide (outside of its traditional range) since May 2022. The virus has been detected in wastewater in Rome, Italy (23), and California, USA (24), showcasing the immense potential of wastewater surveillance as a tool for monitoring viruses in the Poxviridae family (200).
Viral pathogens, such as measles virus (measles is ranked 3rd) and varicella-zoster (shingles is ranked 23rd) were detected in urine specimens, indicating their potential to be monitored through wastewater surveillance as well (35, 202). Influenza, which ranked 6th on CDWSRank, was investigated in previous studies regarding the potential of wastewater surveillance (203).
Notably, polio ranks 18th in our CDWSRank system primarily due to its high R0 value, indicating that it has the potential to spread widely and quickly. Although polio cases have not been identified in Michigan between 2014–2021, the disease can have severe health consequences and can be dangerous if it emerges. It is worth noting that the data published by the MDHHS is subject to yearly review. New information and inclusion of recent data could potentially affect the ranking of polio or any other CDs in our CDWSRank system. Polio's inclusion in our system is based on its potential to pose a significant public health threat, highlighting the importance of ongoing disease surveillance efforts to prevent the resurgence of CDs like polio. Overall, our CDWSRank system is designed to indicate which diseases should be prioritized in the context of wastewater surveillance for TCDA based on local clinical data and other parameters such as R0.
4.4. Wastewater surveillance for bacterial, fungal, and parasitic CDs
CDWSRank placed 12 bacterial CDs ranked in the top 30 (Figure 7). These include tuberculosis (ranked 9th), CP-CRE (ranked 17th), legionellosis (ranked 15th), salmonellosis (ranked 26th), and shigellosis (ranked 28th), all detecteble both in human excreta and wastewater. Also, campylobacter (ranked 13th) was identified as a highly-sensitive pathogen for wastewater surveillance (204). Bacterial pathogens, such as Chlamydia trachomatis can be detected in wastewater (205). Despite being detectable in human excreta and wastewater, paratyphoid fever, Q fever, and typhoid fever were not ranked among the top 30 CDs.
Only two parasitic CDs, giardiasis (ranked 20th) and amebiasis (ranked 25th) ranked among the top 30. No fungal CDs were ranked among the top 30 CDs. Despite this, fungal CDs, including blastomycosis and cryptococcosis have great potential to be monitored using wastewater, as they can be detected in either human excreta or wastewater (Table 4).
4.5. Strengths and limitations of CDWSRank system
The goal of this study is to develop a quantitative prioritization system for wastewater surveillance of CDs in the TCDA. Several studies have developed methodologies to rank the threat of CDs with different scopes and methodologies (28–31). However, these studies have many limitations in their ranking systems which were refined and improved by the CDWSRank system.
Firstly, these ranking systems did not include parameters such as actual disease cases and basic reproduction numbers (R0) for CDs (28–31). For instance, Balabanova et al., applied criteria such as incidence rate to prioritize 127 CDs in Germany (28). However, the study did not include the actual annual incidence number of the CDs. Instead, the importance of incidence for each disease was evaluated by weights given by experts. In our CDWSRank system, the actual disease incidence data between 2014 and 2021 for 96 CDs were extensively investigated and included in the system. Besides, in this study we investigated the R0 for 96 CDs and incorporated them in the system when available.
Secondly, existing ranking systems relied heavily on experts' opinions on weighting the parameters when ranking the diseases (28–31). For instance, Cardoen et al., proposed a ranking system for 51 zoonotic agents which replied on scores given by 35 scientific experts in the field of animal and public health, food, clinical microbiology, and epidemiology (30). Likewise, Humblet et al., applied multicriteria decision-making methodologies based on expert opinions and data to rank 100 infectious diseases, in a system that included 57 criteria and 5 categories encompassing epidemiology, economy, public health, society, and prevention/control (31). The systems are affected by individual opinions of experts evaluating qualitative parameters. Experts' opinions could be subject to bias, which can affect the final ranking results. The subjective nature of weighting parameters by individuals for some criteria, such as public health impact, animal health impact, and food impact, can lead to uncertainty and variation in final ranking scores depending on individual interpretations of these parameters (30). In contrast, to circumvent the bias of subjective opinions of experts, we designed the CDWSRank system based on a data-driven approach that considers critical factors including quantitative parameters of disease incidence and trend, geographical ratio, and R0 for all CDs. In this way, the proposed ranking system differs from existing systems that are primarily based on the subjective, albeit expert, opinions. Besides, the weights given by experts for the specific locations can be hardly applied to other areas. However, by replacing the quantitative parameters in CDWSRank system, it can be applied beyond TCDA to other locations with accessible data. For instance, the clinical case trend in the State of Michigan and TCDA can be replaced by clinical disease databases based on different geographical information, henceforth enhancing the CDWSRank system's potential for wider applications.
Thirdly, the ranking systems in previous studies were designed for specific events or areas, which can be hardly applied beyond their scope. For instance, Balabanova et al. (28) included notifiable diseases in Germany and reportable diseases within the European Union. Likewise, Economopoulou et al. (29) focused only on the risk of CDs associated with the hosting of the London 2012 Olympic Games. To circumvent those biases, the 96 CDs included in CDWSRank system were selected based on U.S. CDC reportable disease lists and other governmental lists including the EPA CCL and CDC Bio-terrorism List, and local disease report lists including MDHHS WDR, which distinguishes it from all previous ranking systems for ranking CDs (28–31). This proposed ranking system is highly adaptable to other regions, especially those with similar reporting models which most states in the United Sates have, as a result of the CDC National Notifiable Disease Surveillance System requirements. Furthermore, to the best of our knowledge, there have been no published studies ranking CDs of public health importance that can be monitored using wastewater surveillance.
The goal of this study was to develop a prioritization system for wastewater surveillance of CDs in the TCDA. Limitations of this study are expounded below. Firstly, a multiplier of 0 was applied to a given CD if their causative pathogen has not been detected in wastewater or human excreta according to published studies thus far. This excludes potentially harmful CDs which can result in severe public health consequences, such as anthrax, hantavirus, and plague. Secondly, the weighting factors or multipliers for both binary and quantitative parameters were determined by researchers of this study and specifically designed with an emphasis on the TCDA. Nonetheless, weighting factors are adjustable and can vary across studies and regions with dissimilar research emphases. Thirdly, data unavailability limited the parameter types that could be involved in the proposed ranking system. For instance, mortality rate, case fatality, or incidence rate of some CDs could not be located in any published studies or publicly-available datasets for the TCDA. Additionally, due to a lack of R0 information on some CDs, the ranking system may have disregarded diseases that are potentially harmful to human health but that do not yet have an established, specific R0. R0 values are situation-dependent and can significantly affect the rank (167). Besides, the CDWSRank system is limited since it does not explore the connection between severity and economic impact of the diseases ranked in this study. The severity of the disease in many instances would vary significantly with access to health care and the economic impact would vary with the severity. Despite the researchers' initial attempts to include parameters of mortality rate and severity, very few studies were found that adequately quantified these values in the TCDA region. It is, however, possible to include these parameters when adapting the CDWSRank system for a different locale if those data are available in the new area studied. Another significant limitation on the CDWSRank system is its reliance on case data being publicly and readily available. The implications of this limitation become particularly salient in locations where clinical data and information for reportable diseases are unavailable. However, as the CDWSRank system did produce a ranking score for Monkeypox, a disease without the case numbers published at the time of study, it is evident that the system can still create a ranking based on the other parameters. Hence, the CDWSRank system retains its utility in settings where access to data is restricted.
Social determinants of health such as socioeconomic status, environment, race and ethnicity, gender, culture, and access to health care would be other parameters for future development of the CDWSRank system. However, measuring and quantifying these factors for all 96 CDs in TCDA pose significant challenges, given the limited availability and accessibility of relevant data. Nonetheless, the insights generated by the CDWSRank system can be particularly valuable for guiding wastewater surveillance of emerging CDs which is beneficial for socioeconomically disadvantaged communities with limited healthcare access or traditional surveillance systems. Nevertheless, it is critical to note that as the aforementioned constraints become known, updating the CDWSRank system becomes necessary.
It is worth noting that some of the diseases of concern are seasonal (such as influenza) or rare (such as polio) and therefore only occasional surveillance may be recommended. In addition, some CDs, such as chlamydia, gonorrhea, and HIV, prioritized by CDWSRank system in TCDA are associated not only with urban areas, but also with socioeconomical and racial inequality, which can skew statistical designs. Social determinants of health, such as poverty, poor housing conditions, lack of access to healthcare, can disproportionately affect certain racial or ethnic groups and increase their risk of contracting and transmitting communicable diseases (170–173). For example, individuals living in crowded and unsanitary conditions are more likely to contract infectious diseases like TB or hepatitis A (206, 207). Therefore, surveillance of specific regions of concern may be recommended.
4.6. Future directions
In the State of Michigan, as in multiple other regions across the nation, the COVID-19 pandemic prompted the creation of wastewater surveillance networks. As the primary health focus shifts away from COVID-19 these currently available networks and their infrastructure and resources can be adapted to monitor other emerging diseases. This study offers a tool for transitioning to wastewater surveillance programs beyond COVID-19. By identifying and ranking the CDs that pose the most significant risk to public health in TCDA, the CDWSRank system provides a methodological tool and critical information that can help public health officials and policymakers allocate resources more effectively. This information can be used to prioritize disease surveillance efforts and ensure that public health interventions are targeted at the most potentially urgent threats.
Furthermore, with regards to the extension of the CDWSRank system's applicability beyond the TCDA region, it is worth noting that the quantitative parameters heavily rely on local clinical data, while the binary parameters are primarily developed from regulatory lists obtained from local health departments as well as from U.S. governmental agencies. It is worth mentioning that all states in the U.S. are mandated to report to the C.D.C. and have their respective local health departments responsible for reporting notifiable diseases. Therefore, extending the application of the CDWSRank system to other regions within the U.S. would be relatively straightforward.
5. Conclusion
In this study, we developed a comprehensive and effective ranking system (CDWSRank) of wastewater surveillance prioritization for 96 CDs in the Tri-County Detroit Area (TCDA), Michigan, USA. The CDWSRank system comprises 6 binary and 6 quantitative parameters, with CDs classified into four groups: viral, bacterial, fungal, and parasitic. Critical regulatory lists, including the CDC's NNIDCL, MDHHS's WDR, EPA's CCL, and CDC's bioterrorism agents list were incorporated into the CDWSRank system. Disease incidences and trends of reportable CDs in the TCDA and broader state of Michigan were also incorporated into the system. Disparities in incidences of CDs were identified between the TCDA and state of Michigan, indicating epidemiological differences. Appropriate sampling and sample concentration methods for wastewater surveillance application were summarized as per our four categories, viral, bacterial, fungal, and parasitic.
The CDWSRank system is one of the first of its kind with the potential to prioritize resources and efforts toward monitoring and preventing the spread of CDs through wastewater surveillance. It helps researchers and public health practitioners to identify CDs that at a higher risk of disease transmission and prioritize monitoring efforts to mitigate their spread. The CDWSRank system provides an evidence- and data-based approach to decision making, ensuring the resources are allocated for wastewater surveillance beyond the COVID-19 pandemic. Ultimately, the development and implementation of the CDWSRank system for CDs can help reduce the impact of CDs on public health and promote broader applications of wastewater surveillance for public health benefits. CDWSRank can and should be adopted for ranking CDs in other geographical locations, with updated etiological and epidemiological information.
Data availability statement
The original contributions presented in the study are included in the article/supplementary material, further inquiries can be directed to the corresponding author.
Author contributions
ZG and LZ: methodology, investigation, data acquisition, data curation, formal analysis, visualization, writing—original draft, writing—review and editing, and are co-led manuscript development. RF and RD: writing—review and editing. JN: funding acquisition and writing—review and editing. IX: conceptualization, funding acquisition, methodology, investigation, project administration, resources, supervision, and writing—review and editing. All authors contributed to the article and approved the submitted version.
Funding
This study was funded by the Michigan Department of Health and Human Services (MDHHS) and the Great Lakes Water Authority (GLWA).
Acknowledgments
We acknowledge the support from operators, the laboratory team, and managers of the Wastewater Resource Recovery Facility at the Great Lakes Water Authority. We furthermore thank CDM Smith for supporting this work.
Conflict of interest
The authors declare that the research was conducted in the absence of any commercial or financial relationships that could be construed as a potential conflict of interest.
Publisher's note
All claims expressed in this article are solely those of the authors and do not necessarily represent those of their affiliated organizations, or those of the publisher, the editors and the reviewers. Any product that may be evaluated in this article, or claim that may be made by its manufacturer, is not guaranteed or endorsed by the publisher.
Abbreviations
CCL, Contaminant Candidate List; CDWSRank system, communicable disease wastewater surveillance ranking system; CDs, communicable diseases; GI, Gastrointestinal Illness; GLWA, Great Lakes Water Authority; HIV/AIDS, Human Immunodeficiency Virus and Acquired Immunodeficiency Syndrome; TCDA, Tri-County Detroit Area; MDHHS, Michigan Department of Health and Human Services; MDSS, Michigan Disease Surveillance System; WDR, MDHHS/MDSS Weekly Disease Report; NNIDCL, National Notifiable Infectious Disease and Conditions List; STDs, sexually transmitted diseases; U.S. EPA, United States Environmental Protection Agency; U.S. AID, United States Agency of International Development; U.S. CDC, United States Centers for Disease Control and Prevention; U.S. NIH, United States National Institutes of Health; WHO, World Health Organization.
References
1. Ahmed W, Tscharke B, Bertsch PM, Bibby K, Bivins A, Choi P, et al. SARS-CoV-2 RNA monitoring in wastewater as a potential early warning system for COVID-19 transmission in the community: A temporal case study. Sci Total Environ. (2021) 761:144216. doi: 10.1016/j.scitotenv.2020.144216
2. Ahmed W, Bivins A, Simpson SL, Bertsch PM, Ehret J, Hosegood I, et al. Wastewater surveillance demonstrates high predictive value for COVID-19 infection on board repatriation flights to Australia. Environ Int. (2022) 158:106938. doi: 10.1016/j.envint.2021.106938
3. Ahmed W, Bertsch PM, Angel N, Bibby K, Bivins A, Dierens L, et al. Detection of SARS-CoV-2 RNA in commercial passenger aircraft and cruise ship wastewater: a surveillance tool for assessing the presence of COVID-19 infected travellers. J Travel Med. (2020) 27:taaa116. doi: 10.1093/jtm/taaa116
4. Li Y, Miyani B, Zhao L, Spooner M, Gentry Z, Zou Y, et al. Surveillance of SARS-CoV-2 in nine neighborhood sewersheds in Detroit Tri-County area, United States: Assessing per capita SARS-CoV-2 estimations and COVID-19 incidence. Sci Total Environ. (2022) 851:158350. doi: 10.1016/j.scitotenv.2022.158350
5. Zhao L, Zou Y, Li Y, Miyani B, Spooner M, Gentry Z, et al. Five-week warning of COVID-19 peaks prior to the Omicron surge in Detroit, Michigan using wastewater surveillance. Sci Total Environ. (2022) 844:157040. doi: 10.1016/j.scitotenv.2022.157040
6. Miyani B, Fonoll X, Norton J, Mehrotra A, Xagoraraki I. SARS-CoV-2 in Detroit Wastewater. J Environ Eng. (2020) 146:06020004. doi: 10.1061/(ASCE)EE.1943-7870.0001830
7. Miyani B, Zhao L, Spooner M, Buch S, Gentry Z, Mehrotra A, et al. Early Warnings of COVID-19 Second Wave in Detroit. J Environ Eng. (2021) 147:06021004. doi: 10.1061/(ASCE)EE.1943-7870.0001907
8. Sherchan SP, Shahin S, Ward LM, Tandukar S, Aw TG, Schmitz B, et al. First detection of SARS-CoV-2 RNA in wastewater in North America: A study in Louisiana, USA. Sci Total Environ. (2020) 743:140621. doi: 10.1016/j.scitotenv.2020.140621
9. Medema G, Heijnen L, Elsinga G, Italiaander R, Brouwer A. Presence of SARS-Coronavirus-2 RNA in Sewage and Correlation with Reported COVID-19 Prevalence in the Early Stage of the Epidemic in the Netherlands. Environ Sci Technol Lett. (2020) 7:511–6. doi: 10.1021/acs.estlett.0c00357
10. Zhao L, Zou Y, David RE, Withington S, McFarlane S, Faust RA, et al. Simple methods for early warnings of COVID-19 surges: Lessons learned from 21 months of wastewater and clinical data collection in Detroit, Michigan, United States. Sci Total Environ. (2022) 864:161152. doi: 10.1016/j.scitotenv.2022.161152
11. Ahmed W, Angel N, Edson J, Bibby K, Bivins A, O'Brien JW, et al. First confirmed detection of SARS-CoV-2 in untreated wastewater in Australia: A proof of concept for the wastewater surveillance of COVID-19 in the community. Sci Total Environ. (2020) 728:138764. doi: 10.1016/j.scitotenv.2020.138764
12. Bibby K, Bivins A, Wu Z, North D. Making waves: Plausible lead time for wastewater based epidemiology as an early warning system for COVID-19. Water Res. (2021) 202:117438. doi: 10.1016/j.watres.2021.117438
13. Xagoraraki I, O'Brien E. Wastewater-based epidemiology for early detection of viral outbreaks. In:O'Bannon DJ, , editor. Women in Water Quality: Investigations by Prominent Female Engineers. Cham: Springer International Publishing; (2020). p. 75–97. doi: 10.1007/978-3-030-17819-2_5
14. Xagoraraki I. Can we predict viral outbreaks using wastewater surveillance? J Environ Eng. (2020) 146:1820003. doi: 10.1061/(ASCE)EE.1943-7870.0001831
15. Kitajima M, Ahmed W, Bibby K, Carducci A, Gerba CP, Hamilton KA, et al. SARS-CoV-2 in wastewater: State of the knowledge and research needs. Sci Total Environ. (2020) 739:139076. doi: 10.1016/j.scitotenv.2020.139076
16. Ahmed W, Bivins A, Bertsch PM, Bibby K, Gyawali P, Sherchan SP, et al. Intraday variability of indicator and pathogenic viruses in 1-h and 24-h composite wastewater samples: Implications for wastewater-based epidemiology. Environ Res. (2021) 193:110531. doi: 10.1016/j.envres.2020.110531
17. McCall C, Wu H, O'Brien E, Xagoraraki I. Assessment of enteric viruses during a hepatitis outbreak in Detroit MI using wastewater surveillance and metagenomic analysis. J Appl Microbiol. (2021) 131:1539–54. doi: 10.1111/jam.15027
18. Miyani B, McCall C, Xagoraraki I. High abundance of human herpesvirus 8 in wastewater from a large urban area. J Appl Microbiol. (2021) 130:1402–11. doi: 10.1111/jam.14895
19. Hovi T, Shulman L, Van Der Avoort H, Deshpande J, Roivainen M, De Gourville E. Role of environmental poliovirus surveillance in global polio eradication and beyond. Epidemiol Infect. (2012) 140:1–13. doi: 10.1017/S095026881000316X
20. Roberts L. Israel's silent polio epidemic breaks all the rules. Science. (2013) 342:679–80. doi: 10.1126/science.342.6159.679
21. Link-Gelles R, Lutterloh E, Schnabel Ruppert P, Backenson PB, George K, Rosenberg ES, et al. Public health response to a case of paralytic poliomyelitis in an unvaccinated person and detection of poliovirus in wastewater — New York. MMWR Morb Mortal Wkly Rep. (2022) 71:1065–8. doi: 10.15585/mmwr.mm7133e2
22. de Jonge EF, Peterse CM, Koelewijn JM, van der Drift AMR, van der Beek RFHJ, Nagelkerke E, et al. The detection of monkeypox virus DNA in wastewater samples in the Netherlands. Sci Total Environ. (2022) 852:158265. doi: 10.1016/j.scitotenv.2022.158265
23. la Rosa G, Mancini P, Veneri C, Bonanno Ferraro G, Lucentini L, Iaconelli M, et al. Detection of Monkeypox virus DNA in the wastewater of an airport in Rome, Italy: expanding environmental surveillance to emerging threats. medRxiv. (2022). doi: 10.1101/2022.08.18.22278932
24. Wolfe MK, Duong D, Hughes B, Chan-Herur V, White BJ, Boehm AB. Detection of monkeypox viral DNA in a routine wastewater monitoring program. medRxiv. (2022). doi: 10.1101/2022.07.25.22278043
25. van Seventer JM, Hochberg NS. Principles of Infectious Diseases: Transmission, Diagnosis, Prevention, and Control. In: International Encyclopedia of Public Health. Elsevier (2017). p. 22–39. doi: 10.1016/B978-0-12-803678-5.00516-6
26. Morse SS, Mazet JA, Woolhouse M, Parrish CR, Carroll D, Karesh WB, et al. Prediction and prevention of the next pandemic zoonosis. Lancet. (2012) 380:1956–65. doi: 10.1016/S0140-6736(12)61684-5
27. Chharia A, Jeevan G, Jha RA, Liu M, Berman JM, Glorioso C. Accuracy of US CDC COVID-19 Forecasting Models. medRxiv. (2022). doi: 10.1101/2022.04.20.22274097
28. Balabanova Y, Gilsdorf A, Buda S, Burger R, Eckmanns T, Gärtner B, et al. Communicable Diseases Prioritized for Surveillance and Epidemiological Research: Results of a Standardized Prioritization Procedure in Germany. PLoS ONE. (2011) 6:e25691. doi: 10.1371/journal.pone.0025691
29. Economopoulou A, Kinross P, Domanovic D, Coulombier D. Infectious diseases prioritisation for event-based surveillance at the European Union level for the 2012 Olympic and Paralympic Games. Eurosurveillance. (2014) 19:20770. doi: 10.2807/1560-7917.ES2014.19.15.20770
30. Cardoen S, van Huffel X, Berkvens D, Quoilin S, Ducoffre G, Saegerman C, et al. Evidence-Based Semiquantitative Methodology for Prioritization of Foodborne Zoonoses. Foodborne Pathog Dis. (2009) 6:1083–96. doi: 10.1089/fpd.2009.0291
31. Humblet MF, Vandeputte S, Albert A, Gosset C, Kirschvink N, Haubruge E, et al. Multidisciplinary and Evidence-based Method for Prioritizing Diseases of Food-producing Animals and Zoonoses. Emerg Infect Dis. (2012) 18:e1. doi: 10.3201/eid1804.111151
32. Chandra F, Lee WL, Armas F, Leifels M, Gu X, Chen H, et al. Persistence of Dengue (Serotypes 2 and 3), Zika, Yellow Fever, and Murine Hepatitis Virus RNA in Untreated Wastewater. Environ Sci Technol Lett. (2021) 8:785–91. doi: 10.1021/acs.estlett.1c00517
33. Hou C, Hua Z, Xu P, Xu H, Wang Y, Liao J, et al. Estimating the prevalence of hepatitis B by wastewater-based epidemiology in 19 cities in China. Sci Total Environ. (2020) 740:139696. doi: 10.1016/j.scitotenv.2020.139696
34. McCall C, Wu H, Miyani B, Xagoraraki I. Identification of multiple potential viral diseases in a large urban center using wastewater surveillance. Water Res. (2020) 184:116160. doi: 10.1016/j.watres.2020.116160
35. Rota PA, Khan AS, Durigon E, Yuran T, Villamarzo YS, Bellini WJ. Detection of measles virus RNA in urine specimens from vaccine recipients. J Clin Microbiol. (1995) 33:2485–8. doi: 10.1128/jcm.33.9.2485-2488.1995
36. Faleye TOC, Adewumi MO, Japhet MO, David OM, Oluyege AO, Adeniji JA, et al. Non-polio enteroviruses in faeces of children diagnosed with acute flaccid paralysis in Nigeria. Virol J. (2017) 14:175. doi: 10.1186/s12985-017-0846-x
37. Leung J, Harpaz R, Baughman AL, Heath K, Loparev V, Vázquez M, et al. Evaluation of Laboratory Methods for Diagnosis of Varicella. Clin Infect Dis. (2010) 51:23–32. doi: 10.1086/653113
38. Musso D, Teissier A, Rouault E, Teururai S, de Pina JJ, Nhan TX. Detection of chikungunya virus in saliva and urine. Virol J. (2016) 13:102. doi: 10.1186/s12985-016-0556-9
39. Lamb LE, Bartolone SN, Tree MO, Conway MJ, Rossignol J, Smith CP, et al. Rapid Detection of Zika Virus in Urine Samples and Infected Mosquitos by Reverse Transcription-Loop-Mediated Isothermal Amplification. Sci Rep. (2018) 8:3803. doi: 10.1038/s41598-018-22102-5
40. Jain S, Su YH, Su YP, McCloud S, Xue R, Lee TJ, et al. Characterization of the hepatitis B virus DNA detected in urine of chronic hepatitis B patients. BMC Gastroenterol. (2018) 18:40. doi: 10.1186/s12876-018-0767-1
41. Numata N, Ohori H, Hayakawa Y, Saitoh Y, Tsunoda A, Kanno A. Demonstration of hepatitis C virus genome in saliva and urine of patients with type C hepatitis: Usefulness of the single round polymerase chain reaction method for detection of the HCV genome. J Med Virol. (1993) 41:120–8. doi: 10.1002/jmv.1890410207
42. Aggarwal R, McCaustland KA. Hepatitis E virus RNA detection in serum and feces specimens with the use of microspin columns. J Virol Methods. (1998) 74:209–13. doi: 10.1016/S0166-0934(98)00049-4
43. Beyer S, Szewzyk R, Gnirss R, Johne R, Selinka HC. Detection and Characterization of Hepatitis E Virus Genotype 3 in Wastewater and Urban Surface Waters in Germany. Food Environ Virol. (2020) 12:137–47. doi: 10.1007/s12560-020-09424-2
44. Kevill JL, Lambert-Slosarska K, Pellett C, Woodhall N, Richardson-O'Neill I, Pântea I, et al. Assessment of two types of passive sampler for the efficient recovery of SARS-CoV-2 and other viruses from wastewater. Sci Total Environ. (2022) 838:156580. doi: 10.1016/j.scitotenv.2022.156580
45. Krause CH, Eastick K, Ogilvie MM. Real-time PCR for mumps diagnosis on clinical specimens—Comparison with results of conventional methods of virus detection and nested PCR. J Clin Virol. (2006) 37:184–9. doi: 10.1016/j.jcv.2006.07.009
46. Baek SH, Kim MW, Park CY, Choi CS, Kailasa SK, Park JP, et al. Development of a rapid and sensitive electrochemical biosensor for detection of human norovirus via novel specific binding peptides. Biosens Bioelectron. (2019) 123:223–9. doi: 10.1016/j.bios.2018.08.064
47. Kitajima M, Haramoto E, Phanuwan C, Katayama H, Ohgaki S. Detection of genogroup IV norovirus in wastewater and river water in Japan. Lett Appl Microbiol. (2009) 49:655–8. doi: 10.1111/j.1472-765X.2009.02718.x
48. Kim JM, Kim HM, Lee EJ, Jo HJ, Yoon Y, Lee NJ, et al. Detection and Isolation of SARS-CoV-2 in Serum, Urine, and Stool Specimens of COVID-19 Patients from the Republic of Korea. Osong Public Health Res Perspect. (2020) 11:112–7. doi: 10.24171/j.phrp.2020.11.3.02
49. Lago PM, Gary HE, Pérez LS, Cáceres V, Olivera JB, Puentes RP, et al. Poliovirus detection in wastewater and stools following an immunization campaign in Havana, Cuba. Int J Epidemiol. (2003) 32:772–7. doi: 10.1093/ije/dyg185
50. Gdoura M, Fares W, Bougatef S, Inoubli A, Touzi H, Hogga N, et al. The value of West Nile virus RNA detection by real-time RT-PCR in urine samples from patients with neuroinvasive forms. Arch Microbiol. (2022) 204:238. doi: 10.1007/s00203-022-02829-6
51. Domingo C, Yactayo S, Agbenu E, Demanou M, Schulz AR, Daskalow K, et al. Detection of Yellow Fever 17D Genome in Urine. J Clin Microbiol. (2011) 49:760–2. doi: 10.1128/JCM.01775-10
52. Peiró-Mestres A, Fuertes I, Camprubí-Ferrer D, Marcos MÁ, Vilella A, Navarro M, et al. Frequent detection of monkeypox virus DNA in saliva, semen, and other clinical samples from 12 patients, Barcelona, Spain. Eurosurveillance. (2022) 27:2200503. doi: 10.2807/1560-7917.ES.2022.27.28.2200503
53. Battistone A, Buttinelli G, Fiore S, Amato C, Bonomo P, Patti AM, et al. Sporadic isolation of sabin-like polioviruses and high-level detection of non-polio enteroviruses during sewage surveillance in seven italian cities, after several years of inactivated poliovirus vaccination. Appl Environ Microbiol. (2014) 80:4491–501. doi: 10.1128/AEM.00108-14
54. Palmer CJ, Bonilla GF, Tsai YL, Lee MH, Javier BJ, Siwak EB. Analysis of sewage effluent for human immunodeficiency virus (HIV) using infectivity assay and reverse transcriptase polymerase chain reaction. Can J Microbiol. (1995) 41:809–15. doi: 10.1139/m95-111
55. Li JJ, Huang YQ, Poiesz BJ, Zaumetzger-Abbot L, Friedman-Kien AE. Detection of human immunodeficiency virus type 1 (HIV-1) in urine cell pellets from HIV-1-seropositive individuals. J Clin Microbiol. (1992) 30:1051–5. doi: 10.1128/jcm.30.5.1051-1055.1992
56. Wattiau P, Klee SR, Fretin D, van Hessche M, Ménart M, Franz T, et al. Occurrence and genetic diversity of Bacillus anthracis strains isolated in an active wool-cleaning factory. Appl Environ Microbiol. (2008) 74:4005–11. doi: 10.1128/AEM.00417-08
57. Kalb SR, Moura H, Boyer AE, McWilliams LG, Pirkle JL, Barr JR. The use of Endopep–MS for the detection of botulinum toxins A, B, E, and F in serum and stool samples. Anal Biochem. (2006) 351:84–92. doi: 10.1016/j.ab.2006.01.027
58. LaGier MJ, Joseph LA, Passaretti T, Musser KA, Cirino NM, A. real-time multiplexed PCR assay for rapid detection and differentiation of Campylobacter jejuni and Campylobacter coli. Mol Cell Probes. (2004) 18:275–82. doi: 10.1016/j.mcp.2004.04.002
59. Bonetta S, Pignata C, Lorenzi E, de Ceglia M, Meucci L, Bonetta S, et al. Detection of pathogenic Campylobacter, E coli O157:H7 and Salmonella spp in wastewater by PCR assay. Environ Sci Pollut Res. (2016) 23:15302–9. doi: 10.1007/s11356-016-6682-5
60. Gaydos CA, Theodore M, Dalesio N, Wood BJ, Quinn TC. Comparison of Three Nucleic Acid Amplification Tests for Detection of Chlamydia trachomatis in Urine Specimens. J Clin Microbiol. (2004) 42:3041–5. doi: 10.1128/JCM.42.7.3041-3045.2004
61. Rahman M, Sack DA, Mahmood S, Hossain A. Rapid diagnosis of cholera by coagglutination test using 4-h fecal enrichment cultures. J Clin Microbiol. (1987) 25:2204–6. doi: 10.1128/jcm.25.11.2204-2206.1987
62. Galler H, Feierl G, Petternel C, Reinthaler FF, Haas D, Grisold AJ, et al. KPC-2 and OXA-48 carbapenemase-harbouring Enterobacteriaceae detected in an Austrian wastewater treatment plant. Clin Microbiol Infect. (2014) 20:O132–4. doi: 10.1111/1469-0691.12336
63. Akduman D, Ehret JM, Messina K, Ragsdale S, Judson FN. Evaluation of a Strand Displacement Amplification Assay (BD ProbeTec-SDA) for Detection of Neisseria gonorrhoeae in Urine Specimens. J Clin Microbiol. (2002) 40:281–3. doi: 10.1128/JCM.40.1.281-283.2002
64. Riera L. Detection of Haemophilus influenzae type b antigenuria by Bactigen and Phadebact kits. J Clin Microbiol. (1985) 21:638–40. doi: 10.1128/jcm.21.4.638-640.1985
65. Murdoch DR, Walford EJ, Jennings LC, Light GJ, Schousboe MI, Chereshsky AY, et al. Use of the polymerase chain reaction to detect legionella DNA in urine and serum samples from patients with pneumonia. Clin Infect Dis. (1996) 23:475–80. doi: 10.1093/clinids/23.3.475
66. Catalan V, Garcia F, Moreno C, Vila MJ, Apraiz D. Detection of Legionella pneumophila in wastewater by nested polymerase chain reaction. Res Microbiol. (1997) 148:71–8. doi: 10.1016/S0923-2508(97)81902-X
67. Caleffi KR, Hirata RDC, Hirata MH, Caleffi ER, Siqueira VLD, Cardoso RF. Use of the polymerase chain reaction to detect Mycobacterium leprae in urine. Brazilian J Med Biol Res. (2012) 45:153–7. doi: 10.1590/S0100-879X2012007500011
68. Mérien F, Amouriaux P, Perolat P, Baranton G, Girons I. Polymerase chain reaction for detection of Leptospira spp in clinical samples. J Clin Microbiol. (1992) 30:2219–24. doi: 10.1128/jcm.30.9.2219-2224.1992
69. Moreno Y, Ballesteros L, García-Hernández J, Santiago P, González A, Ferrús MA. Specific detection of viable Listeria monocytogenes in Spanish wastewater treatment plants by Fluorescent In Situ Hybridization and PCR. Water Res. (2011) 45:4634–40. doi: 10.1016/j.watres.2011.06.015
70. Lee DY, Lauder H, Cruwys H, Falletta P, Beaudette LA. Development and application of an oligonucleotide microarray and real-time quantitative PCR for detection of wastewater bacterial pathogens. Sci Total Environ. (2008) 398:203–11. doi: 10.1016/j.scitotenv.2008.03.004
71. Guo F, Zhang T, Li B, Wang Z, Ju F, Liang Y. Mycobacterial species and their contribution to cholesterol degradation in wastewater treatment plants. Sci Rep. (2019) 9:836. doi: 10.1038/s41598-018-37332-w
72. Amalina ZN, Khalid MF, Rahman SF, Ahmad MN, Ahmad Najib M, Ismail A, et al. Nucleic Acid-Based Lateral Flow Biosensor for Salmonella Typhi and Salmonella Paratyphi: A Detection in Stool Samples of Suspected Carriers. Diagnostics. (2021) 11:700. doi: 10.3390/diagnostics11040700
73. Liu P, Ibaraki M, Kapoor R, Amin N, Das A, Miah R, et al. Development of Moore Swab and Ultrafiltration Concentration and Detection Methods for Salmonella Typhi and Salmonella Paratyphi A in Wastewater and Application in Kolkata, India and Dhaka, Bangladesh. Front Microbiol. (2021) 15:12. doi: 10.3389/fmicb.2021.684094
74. Branley JM, Roy B, Dwyer DE, Sorrell TC. Real-time PCR detection and quantitation of Chlamydophila psittaci in human and avian specimens from a veterinary clinic cluster. Eur J Clin Microbiol Infect Dis. (2008) 27:269–73. doi: 10.1007/s10096-007-0431-0
75. Schets FM, de Heer L, de Roda Husman AM. Coxiella burnetii in sewage water at sewage water treatment plants in a Q fever epidemic area. Int J Hyg Environ Health. (2013) 216:698–702. doi: 10.1016/j.ijheh.2012.12.010
76. Araj GF, Chugh TD. Detection of Salmonella spp. in clinical specimens by capture enzyme-linked immunosorbent assay. J Clin Microbiol. (1987) 25:2150–3. doi: 10.1128/jcm.25.11.2150-2153.1987
77. Cohen D, Orr N, Robin G, Slepon R, Ashkenazi S, Ashkenazi I, et al. Detection of antibodies to Shigella lipopolysaccharide in urine after natural Shigella infection or vaccination. Clin Diagn Lab Immunol. (1996) 3:451–5. doi: 10.1128/cdli.3.4.451-455.1996
78. Pant A, Mittal AK. New Protocol for the Enumeration of Salmonella and Shigella from Wastewater. J Environ Eng. (2008) 134:222–6. doi: 10.1061/(ASCE)0733-9372(2008)134:3(222)
79. Domanguez J, Gal N, Blanco S, Pedroso P, Prat C, Matas L, et al. Detection of Streptococcus pneumoniae Antigen by a Rapid Immunochromatographic Assay in Urine Samples. Chest. (2001) 119:243–9. doi: 10.1378/chest.119.1.243
80. Wang C, Zheng X, Guan Z, Zou D, Gu X, Lu H, et al. Quantified Detection of Treponema pallidum DNA by PCR Assays in Urine and Plasma of Syphilis Patients. Microbiol Spectr. (2022) 10:e01772–21. doi: 10.1128/spectrum.01772-21
81. Gómez P, Lozano C, Benito D, Estepa V, Tenorio C, Zarazaga M, et al. Characterization of staphylococci in urban wastewater treatment plants in Spain, with detection of methicillin resistant Staphylococcus aureus ST398. Environ Pollut. (2016) 212:71–6. doi: 10.1016/j.envpol.2016.01.038
82. Bulterys MA, Wagner B, Redard-Jacot M, Suresh A, Pollock NR, Moreau E, et al. Point-Of-Care Urine LAM Tests for Tuberculosis Diagnosis: A Status Update. J Clin Med. (2019) 9:111. doi: 10.3390/jcm9010111
83. Mtetwa HN, Amoah ID, Kumari S, Bux F, Reddy P. Molecular surveillance of tuberculosis-causing mycobacteria in wastewater. Heliyon. (2022) 8:e08910. doi: 10.1016/j.heliyon.2022.e08910
84. Bahl MI, Rosenberg K. High abundance and diversity of Bacillus anthracis plasmid pXO1-like replicons in municipal wastewater. FEMS Microbiol Ecol. (2010) 74:241–7. doi: 10.1111/j.1574-6941.2010.00922.x
85. Al-Gheethi AAS, Abdul-Monem MO, AL-Zubeiry AHS, Efaq AN, Shamar AM, Al-Amery RMA. Effectiveness of selected wastewater treatment plants in Yemen for reduction of faecal indicators and pathogenic bacteria in secondary effluents and sludge. Water Pract Technol. (2014) 9:293–306. doi: 10.2166/wpt.2014.031
86. Berendes D, Kirby A, Brown J, Wester AL. Human faeces-associated extended-spectrum β-lactamase-producing Escherichia coli discharge into sanitation systems in 2015 and 2030: a global and regional analysis. Lancet Planet Health. (2020) 4:e246–55. doi: 10.1016/S2542-5196(20)30099-1
87. Stark D, van Hal S, Fotedar R, Butcher A, Marriott D, Ellis J, et al. Comparison of Stool Antigen Detection Kits to PCR for Diagnosis of Amebiasis. J Clin Microbiol. (2008) 46:1678–81. doi: 10.1128/JCM.02261-07
88. Srikanth R, Naik D. Health Effects of Wastewater Reuse for Agriculture in the Suburbs of Asmara City, Eritrea. Int J Occup Environ Health. (2004) 10:284–8. doi: 10.1179/oeh.2004.10.3.284
89. Guy RA, Payment P, Krull UJ, Horgen PA. Real-Time PCR for Quantification of Giardia and Cryptosporidium in Environmental Water Samples and Sewage. Appl Environ Microbiol. (2003) 69:5178–85. doi: 10.1128/AEM.69.9.5178-5185.2003
90. Dixon BR, Bussey JM, Parrington LJ, Parenteau M. Detection of Cyclospora cayetanensis Oocysts in Human Fecal Specimens by Flow Cytometry. J Clin Microbiol. (2005) 43:2375–9. doi: 10.1128/JCM.43.5.2375-2379.2005
91. Sturbaum GD, Ortega YR, Gilman RH, Sterling CR, Cabrera L, Klein DA. Detection of Cyclospora cayetanensis in Wastewater. Appl Environ Microbiol. (1998) 64:2284–6. doi: 10.1128/AEM.64.6.2284-2286.1998
92. Johnston SP, Ballard MM, Beach MJ, Causer L, Wilkins PP. Evaluation of Three Commercial Assays for Detection of Giardia and Cryptosporidium Organisms in Fecal Specimens. J Clin Microbiol. (2003) 41:623–6. doi: 10.1128/JCM.41.2.623-626.2003
93. Mayer CL, Palmer CJ. Evaluation of PCR, nested PCR, and fluorescent antibodies for detection of Giardia and Cryptosporidium species in wastewater. Appl Environ Microbiol. (1996) 62:2081–5. doi: 10.1128/aem.62.6.2081-2085.1996
94. Jirku M, Pomajbíková K, PetrŽelková KJ, Huzová Z, Modrý D, Lukeš J. Detection of Plasmodium spp. in Human Feces Emerg Infect Dis. (2012) 18:634. doi: 10.3201/eid1804.110984
95. Khurana S, Chaudhary P. Laboratory diagnosis of cryptosporidiosis. Trop Parasitol. (2018) 8:2. doi: 10.4103/tp.TP_34_17
96. Jia S, Zhang X. Biological HRPs in wastewater. In: High-Risk Pollutants in Wastewater. Elsevier; (2020). p. 41–78. doi: 10.1016/B978-0-12-816448-8.00003-4
97. Kauffman CA, Pappas PG, Sobel JD, Dismukes WE. Essentials of Clinical Mycology. New York, NY: Springer. (2011). doi: 10.1007/978-1-4419-6640-7
98. Mataraci-Kara E, Ataman M, Yilmaz G, Ozbek-Celik B. Evaluation of antifungal and disinfectant-resistant Candida species isolated from hospital wastewater. Arch Microbiol. (2020) 202:2543–50. doi: 10.1007/s00203-020-01975-z
99. Walchak RC, Buckwalter SP, Zinsmaster NM, Henn KM, Johnson KM, Koelsch JM, et al. Candida auris direct detection from surveillance swabs, blood, and urine using a laboratory-developed PCR method. J Fungi. (2020) 6:224. doi: 10.3390/jof6040224
100. Hategekimana F, Saha S, Chaturvedi A. Dynamics of amoebiasis transmission: stability and sensitivity analysis. Mathematics. (2017) 5:58. doi: 10.3390/math5040058
101. van den Driessche P. Reproduction numbers of infectious disease models. Infect Dis Model. (2017) 2:288–303. doi: 10.1016/j.idm.2017.06.002
102. Dunn J. The mathematical epidemiology of human babesiosis in the North-Eastern United States. Doctoral dissertation, RMIT University. (2014).
103. Haider N, Vairo F, Ippolito G, Zumla A, Kock RA. Basic reproduction number of chikungunya virus transmitted by aedes mosquitoes. Emerg Infect Dis. (2020) 26:2429. doi: 10.3201/eid2610.190957
104. Potterat JJ, Zimmerman-Rogers H, Muth SQ, Rothenberg RB, Green DL, Taylor JE, et al. Chlamydia transmission: concurrency, reproduction number, and the epidemic trajectory. Am J Epidemiol. (1999) 150:1331–9. doi: 10.1093/oxfordjournals.aje.a009965
105. Phelps M, Perner ML, Pitzer VE, Andreasen V, Jensen PKM, Simonsen L. Cholera epidemics of the past offer new insights into an old enemy. J Infect Dis. (2018) 217:641–9. doi: 10.1093/infdis/jix602
106. Tien JH, Earn DJD. Multiple transmission pathways and disease dynamics in a waterborne pathogen model. Bull Math Biol. (2010) 72:1506–33. doi: 10.1007/s11538-010-9507-6
108. Matsuyama R, Akhmetzhanov AR, Endo A, Lee H, Yamaguchi T, Tsuzuki S, et al. Uncertainty and sensitivity analysis of the basic reproduction number of diphtheria: a case study of a Rohingya refugee camp in Bangladesh. PeerJ. (2018) 6:e4583. doi: 10.7717/peerj.4583
109. Mpeshe S, Nyerere N. A human-animal model of giardiasis infection in contaminated environment. Int J Adv Appl Mathem Mech. (2021) 8:37–47.
110. Jolly AM, Wylie JL. Gonorrhoea and chlamydia core groups and sexual networks in Manitoba. Sex Transm Infect. (2002) 78:i145–51. doi: 10.1136/sti.78.suppl_1.i145
111. Khan A, Naveed M, Dur-e-Ahmad M, Imran M. Estimating the basic reproductive ratio for the Ebola outbreak in Liberia and Sierra Leone. Infect Dis Poverty. (2015) 4:1–8. doi: 10.1186/s40249-015-0043-3
112. Hung HF, Wang YC, Yen AMF, Chen HH. Stochastic model for hepatitis B virus infection through maternal (vertical) and environmental (horizontal) transmission with applications to basic reproductive number estimation and economic appraisal of preventive strategies. Stochastic Environ Res Risk Assess. (2014) 28:611–25. doi: 10.1007/s00477-013-0776-0
113. Pybus OG, Charleston MA, Gupta S, Rambaut A, Holmes EC, Harvey PH, et al. The epidemic behavior of the hepatitis C virus. Science. (1979) 292:2323–5. doi: 10.1126/science.1058321
114. Dalton HR, Hunter JG, Bendall RP. Hepatitis e. Curr Opin Infect Dis. (2013) 26:471–8. doi: 10.1097/01.qco.0000433308.83029.97
115. Lietman T, Porco T, Blower S. Leprosy and tuberculosis: the epidemiological consequences of cross-immunity. Am J Public Health. (1997) 87:1923–7. doi: 10.2105/AJPH.87.12.1923
116. Engida HA, Theuri DM, Gathungu D, Gachohi J, Alemneh HT. A mathematical model analysis for the transmission dynamics of leptospirosis disease in human and rodent populations. Comput Math Methods Med. (2022) 2022:1806585. doi: 10.1155/2022/1806585
117. Guerra FM, Bolotin S, Lim G, Heffernan J, Deeks SL, Li Y, et al. The basic reproduction number (R0) of measles: a systematic review. Lancet Infect Dis. (2017) 17:e420–8. doi: 10.1016/S1473-3099(17)30307-9
118. Opoku NKDO, Borkor RN, Adu AF, Nyarko HN, Doughan A, Appiah EM, et al. Modelling the Transmission Dynamics of Meningitis among High and Low-Risk People in Ghana with Cost-Effectiveness Analysis. In: Abstract and Applied Analysis Hindawi. (2022). doi: 10.1155/2022/9084283
119. Trotter CL, Gay NJ, Edmunds WJ. Dynamic models of meningococcal carriage, disease, and the impact of serogroup C conjugate vaccination. Am J Epidemiol. (2005) 162:89–100. doi: 10.1093/aje/kwi160
120. Grant R, Nguyen LBL, Breban R. Modelling human-to-human transmission of monkeypox. Bull World Health Organ. (2020) 98:638. doi: 10.2471/BLT.19.242347
121. Caley P, Hone J. Assessing the host disease status of wildlife and the implications for disease control: Mycobacterium bovis infection in feral ferrets. J Appl Ecol. (2005) 42:708–19. doi: 10.1111/j.1365-2664.2005.01053.x
122. Gaythorpe KAM, Trotter CL, Lopman B, Steele M, Conlan AJK. Norovirus transmission dynamics: a modelling review. Epidemiol Infect. (2018) 146:147–58. doi: 10.1017/S0950268817002692
123. Pitzer VE, Bowles CC, Baker S, Kang G, Balaji V, Farrar JJ, et al. Predicting the impact of vaccination on the transmission dynamics of typhoid in South Asia: a mathematical modeling study. PLoS Negl Trop Dis. (2014) 8:e2642. doi: 10.1371/journal.pntd.0002642
124. Kretzschmar M, Teunis PFM, Pebody RG. Incidence and reproduction numbers of pertussis: estimates from serological and social contact data in five European countries. PLoS Med. (2010) 7:e1000291. doi: 10.1371/journal.pmed.1000291
125. Sichone J, Simuunza MC, Hang'ombe BM, Kikonko M. Estimating the basic reproduction number for the 2015 bubonic plague outbreak in Nyimba district of Eastern Zambia. PLoS Negl Trop Dis. (2020). 14:e0008811. doi: 10.1371/journal.pntd.0008811
126. Eichner M, Dietz K. Eradication of poliomyelitis: when can one be sure that polio virus transmission has been terminated? Am J Epidemiol. (1996) 143:816–22. doi: 10.1093/oxfordjournals.aje.a008820
127. Polo G, Labruna MB, Ferreira F. Basic reproduction number for the Brazilian Spotted Fever. J Theor Biol. (2018) 458:119–24. doi: 10.1016/j.jtbi.2018.09.011
128. Sfikas N, Greenhalgh D, Lewis F. The basic reproduction number and the vaccination coverage required to eliminate rubella from England and Wales. Math Popul Stud. (2007) 14:3–29. doi: 10.1080/08898480601090634
129. Khan MA, Atangana A. Mathematical modeling and analysis of COVID-19: A study of new variant Omicron. Physica A. (2022) 599:127452. doi: 10.1016/j.physa.2022.127452
130. Chen S, Sanderson MW, Lee C, Cernicchiaro N, Renter DG, Lanzas C. Basic reproduction number and transmission dynamics of common serogroups of enterohemorrhagic Escherichia coli. Appl Environ Microbiol. (2016) 82:5612–20. doi: 10.1128/AEM.00815-16
131. Joh RI, Hoekstra RM, Barzilay EJ, Bowen A, Mintz ED, Weiss H, et al. Dynamics of shigellosis epidemics: estimating individual-level transmission and reporting rates from national epidemiologic data sets. Am J Epidemiol. (2013) 178:1319–26. doi: 10.1093/aje/kwt122
132. Obolski U, Lourenço J, Thompson C, Thompson R, Gori A, Gupta S. Vaccination can drive an increase in frequencies of antibiotic resistance among nonvaccine serotypes of Streptococcus pneumoniae. Proc Nat Acad Sci. (2018) 115:3102–7. doi: 10.1073/pnas.1718712115
133. Garnett GP, Aral SO, Hoyle D, Cates W, Anderson RM. The natural history of syphilis: implications for the transmission dynamics and control of infection. Sex Transm Dis. (1997) 24:185–200. doi: 10.1097/00007435-199704000-00002
134. Martin DL, Wiegand R, Goodhew B, Lammie P, Black CM, West S, et al. Serological measures of trachoma transmission intensity. Sci Rep. (2015) 5:1–5. doi: 10.1038/srep18532
135. Dobay A, Pilo P, Lindholm AK, Origgi F, Bagheri HC, König B. Dynamics of a tularemia outbreak in a closely monitored free-roaming population of wild house mice. PLoS ONE. (2015) 10:e0141103. doi: 10.1371/journal.pone.0141103
136. Marangi L, Mirinaviciute G, Flem E, Scalia Tomba G, Guzzetta G, Freiesleben de Blasio B, et al. The natural history of varicella zoster virus infection in Norway: Further insights on exogenous boosting and progressive immunity to herpes zoster. PLoS ONE. (2017) 12:e0176845. doi: 10.1371/journal.pone.0176845
137. Towers S, Brauer F, Castillo-Chavez C, Falconar AKI, Mubayi A, Romero-Vivas CME. Estimate of the reproduction number of the 2015 Zika virus outbreak in Barranquilla, Colombia, and estimation of the relative role of sexual transmission. Epidemics. (2016) 17:50–5. doi: 10.1016/j.epidem.2016.10.003
138. Lemarchand K, Berthiaume F, Maynard C, Harel J, Payment P, Bayardelle P, et al. Optimization of microbial DNA extraction and purification from raw wastewater samples for downstream pathogen detection by microarrays. J Microbiol Methods. (2005) 63:115–26. doi: 10.1016/j.mimet.2005.02.021
139. Volkmann H, Schwartz T, Kirchen S, Stofer C, Obst U. Evaluation of inhibition and cross-reaction effects on real-time PCR applied to the total DNA of wastewater samples for the quantification of bacterial antibiotic resistance genes and taxon-specific targets. Mol Cell Probes. (2007) 21:125–33. doi: 10.1016/j.mcp.2006.08.009
140. Shannon KE, Lee DY, Trevors JT, Beaudette LA. Application of real-time quantitative PCR for the detection of selected bacterial pathogens during municipal wastewater treatment. Sci Total Environ. (2007) 382:121–9. doi: 10.1016/j.scitotenv.2007.02.039
141. Caldwell JM, Levine JF. Domestic wastewater influent profiling using mitochondrial real-time PCR for source tracking animal contamination. J Microbiol Methods. (2009) 77:17–22. doi: 10.1016/j.mimet.2008.11.007
142. Moges F, Endris M, Belyhun Y, Worku W. Isolation and characterization of multiple drug resistance bacterial pathogens from waste water in hospital and non-hospital environments, Northwest Ethiopia. BMC Res Notes. (2014) 7:215. doi: 10.1186/1756-0500-7-215
143. Kumaraswamy R, Amha YM, Anwar MZ, Henschel A, Rodríguez J, Ahmad F. Molecular analysis for screening human bacterial pathogens in municipal wastewater treatment and reuse. Environ Sci Technol. (2014) 48:11610–9. doi: 10.1021/es502546t
144. Weidhaas JL, Macbeth TW, Olsen RL, Sadowsky MJ, Norat D, Harwood VJ. Identification of a Brevibacterium marker gene specific to poultry litter and development of a quantitative PCR assay. J Appl Microbiol. (2010) 109:334–47. doi: 10.1111/j.1365-2672.2010.04666.x
145. Ezeonuegbu BA, Abdullahi MD, Whong CMZ, Sohunago JW, Kassem HS, Yaro CA, et al. Characterization and phylogeny of fungi isolated from industrial wastewater using multiple genes. Sci Rep. (2022) 12:2094. doi: 10.1038/s41598-022-05820-9
146. Zhang H, Feng J, Chen S, Li B, Sekar R, Zhao Z, et al. Disentangling the drivers of diversity and distribution of fungal community composition in wastewater treatment plants across spatial scales. Front Microbiol. (2018) 18:9. doi: 10.3389/fmicb.2018.01291
147. Dalecka B, Oskarsson C, Juhna T, Kuttava Rajarao G. Isolation of Fungal Strains from Municipal Wastewater for the Removal of Pharmaceutical Substances. Water (Basel). (2020) 12:524. doi: 10.3390/w12020524
148. Guest RK, Smith DW. Isolation and screening of fungi to determine potential for ammonia nitrogen treatment in wastewater. J Environ Eng Sci. (2007) 6:209–17. doi: 10.1139/s06-050
149. Ali EAM, Sayed MA, Abdel-Rahman TMA, Hussein R. Fungal remediation of Cd(ii) from wastewater using immobilization techniques. RSC Adv. (2021) 11:4853–63. doi: 10.1039/D0RA08578B
150. Zhou L, Singh A, Jiang J, Xiao L. Molecular surveillance of Cryptosporidium spp. in raw wastewater in milwaukee: implications for understanding outbreak occurrence and transmission dynamics. J Clin Microbiol. (2003) 41:5254–7. doi: 10.1128/JCM.41.11.5254-5257.2003
151. Li N, Xiao L, Wang L, Zhao S, Zhao X, Duan L, et al. Molecular Surveillance of Cryptosporidium spp, Giardia duodenalis, and Enterocytozoon bieneusi by Genotyping and Subtyping Parasites in Wastewater. PLoS Negl Trop Dis. (2012) 6:e1809. doi: 10.1371/journal.pntd.0001809
152. Berglund B, Dienus O, Sokolova E, Berglind E, Matussek A, Pettersson T, et al. Occurrence and removal efficiency of parasitic protozoa in Swedish wastewater treatment plants. Sci Total Environ. (2017) 598:821–7. doi: 10.1016/j.scitotenv.2017.04.015
153. Martins FDC, Ladeia WA, Toledo R, dos Garcia JL, Navarro IT, Freire RL. Surveillance of Giardia and Cryptosporidium in sewage from an urban area in Brazil. Rev Brasil Parasitol Veter. (2019) 28:291–7. doi: 10.1590/s1984-29612019037
154. Ulloa-Stanojlović FM, Aguiar B, Jara LM, Sato MIZ, Guerrero JA, Hachich E, et al. Occurrence of Giardia intestinalis and Cryptosporidium sp. in wastewater samples from São Paulo State, Brazil, and Lima, Peru. Environ Sci Pollut Res. (2016) 23:22197–205. doi: 10.1007/s11356-016-7537-9
155. Wang Y, Liu P, Zhang H, Ibaraki M, VanTassell J, Geith K, et al. Early warning of a COVID-19 surge on a university campus based on wastewater surveillance for SARS-CoV-2 at residence halls. Sci Total Environ. (2022) 821:153291. doi: 10.1016/j.scitotenv.2022.153291
156. ben Ayed L, Yang W, Widmer G, Cama V, Ortega Y, Xiao L. Survey and genetic characterization of wastewater in Tunisia for Cryptosporidium spp., Giardia duodenalis, Enterocytozoon bieneusi, Cyclospora cayetanensis and Eimeria spp. J Water Health. (2012) 10:431–44. doi: 10.2166/wh.2012.204
157. Stensvold CR, Lebbad M, Hansen A, Beser J, Belkessa S, O'Brien Andersen L, et al. Differentiation of Blastocystis and parasitic archamoebids encountered in untreated wastewater samples by amplicon-based next-generation sequencing. Parasite Epidemiol Control. (2020) 9:e00131. doi: 10.1016/j.parepi.2019.e00131
158. Ajonina C, Buzie C, Otterpohl R. The Detection of Giardia Cysts in a Large-Scale Wastewater Treatment Plant in Hamburg, Germany. J Toxicol Environ Health A. (2013) 76:509–14. doi: 10.1080/15287394.2013.785208
159. Randazzo W, Cuevas-Ferrando E, Sanjuán R, Domingo-Calap P, Sánchez G. Metropolitan wastewater analysis for COVID-19 epidemiological surveillance. Int J Hyg Environ Health. (2020) 230:113621. doi: 10.1016/j.ijheh.2020.113621
160. Kaya D, Niemeier D, Ahmed W, Kjellerup B. Evaluation of multiple analytical methods for SARS-CoV-2 surveillance in wastewater samples. Sci Total Environ. (2022) 808:152033. doi: 10.1016/j.scitotenv.2021.152033
161. O'Brien M, Rundell ZC, Nemec MD, Langan LM, Back JA, Lugo JN, et al. comparison of four commercially available RNA extraction kits for wastewater surveillance of SARS-CoV-2 in a college population. Sci Total Environ. (2021) 801:149595. doi: 10.1016/j.scitotenv.2021.149595
162. Torii S, Furumai H, Katayama H. Applicability of polyethylene glycol precipitation followed by acid guanidinium thiocyanate-phenol-chloroform extraction for the detection of SARS-CoV-2 RNA from municipal wastewater. Sci Total Environ. (2021) 756:143067. doi: 10.1016/j.scitotenv.2020.143067
163. Hjelmsø MH, Hellmér M, Fernandez-Cassi X, Timoneda N, Lukjancenko O, Seidel M, et al. Evaluation of Methods for the Concentration and Extraction of Viruses from Sewage in the Context of Metagenomic Sequencing. PLoS ONE. (2017) 12:e0170199. doi: 10.1371/journal.pone.0170199
164. Zheng X, Deng Y, Xu X, Li S, Zhang Y, Ding J, et al. Comparison of virus concentration methods and RNA extraction methods for SARS-CoV-2 wastewater surveillance. Sci Total Environ. (2022) 824:153687. doi: 10.1016/j.scitotenv.2022.153687
165. Sherchan SP, Shahin S, Patel J, Ward LM, Tandukar S, Uprety S, et al. Occurrence of SARS-CoV-2 RNA in six municipal wastewater treatment plants at the early stage of COVID-19 pandemic in The United States. Pathogens. (2021) 10:798. doi: 10.3390/pathogens10070798
166. Ahmed W, Bivins A, Metcalfe S, Smith WJM, Verbyla ME, Symonds EM, et al. Evaluation of process limit of detection and quantification variation of SARS-CoV-2 RT-qPCR and RT-dPCR assays for wastewater surveillance. Water Res. (2022) 213:118132. doi: 10.1016/j.watres.2022.118132
167. Yadav AK, Kumar S, Singh G, Kansara NK. Demystifying R naught: Understanding what does it hide? Indian J Commun Med. (2021) 46:7. doi: 10.4103/ijcm.IJCM_989_20
168. Kazama S, Masago Y, Tohma K, Souma N, Imagawa T, Suzuki A, et al. Temporal dynamics of norovirus determined through monitoring of municipal wastewater by pyrosequencing and virological surveillance of gastroenteritis cases. Water Res. (2016) 92:244–53. doi: 10.1016/j.watres.2015.10.024
169. Fioretti JM, Fumian TM, Rocha MS, dos Santos I de AL, Carvalho-Costa FA, de Assis MR, et al. Surveillance of Noroviruses in Rio De Janeiro, Brazil: Occurrence of New GIV Genotype in Clinical and Wastewater Samples. Food Environ Virol. (2018) 10:1–6. doi: 10.1007/s12560-017-9308-2
170. Bauermeister J, Eaton L, Stephenson R. A multilevel analysis of neighborhood socioeconomic disadvantage and transactional sex with casual partners among young men who have sex with men living in metro Detroit. Behav Med. (2016) 42:197–204. doi: 10.1080/08964289.2015.1110560
171. Bayeh R, Yampolsky MA, Ryder AG. The social lives of infectious diseases: Why culture matters to COVID-19. Front Psychol. (2021) 12:648086. doi: 10.3389/fpsyg.2021.648086
172. Du WY, Yin CN, Wang HT, Li ZW, Wang WJ, Xue FZ, et al. Infectious diseases among elderly persons: Results from a population-based observational study in Shandong province, China, 2013-2017. J Glob Health. (2021) 11:08010. doi: 10.7189/jogh.11.08010
173. Greene SK, Levin-Rector A, Hadler JL, Fine AD. Disparities in reportable communicable disease incidence by census tract-level poverty, New York City, 2006–2013. Am J Public Health. (2015) 105:e27–34. doi: 10.2105/AJPH.2015.302741
174. Thulin CG, Malmsten J, Ericsson G. Opportunities and challenges with growing wildlife populations and zoonotic diseases in Sweden. Eur J Wildl Res. (2015) 61:649–56. doi: 10.1007/s10344-015-0945-1
175. Wright N, Scherdt M, Aebersold ML, McCullagh MC, Medvec BR, Ellimoottil C, et al. Rural Michigan farmers' health concerns and experiences: a focus group study. J Prim Care Commun Health. (2021) 12:21501327211053520. doi: 10.1177/21501327211053519
176. Lin YH, Tseng YH, Chen YC, Lin MH, Chou LF, Chen TJ, et al. The rural-urban divide in ambulatory care of gastrointestinal diseases in Taiwan. BMC Int Health Hum Rights. (2013) 13:1–7. doi: 10.1186/1472-698X-13-15
177. LeJeune J, Kersting A. Zoonoses: an occupational hazard for livestock workers and a public health concern for rural communities. J Agric Saf Health. (2010) 16:161–79. doi: 10.13031/2013.32041
178. Parola P, Paddock CD. Travel and tick-borne diseases: Lyme disease and beyond. Travel Med Infect Dis. (2018) 26:1–2. doi: 10.1016/j.tmaid.2018.09.010
179. Vanos JK, Kalkstein LS, Sanford TJ. Detecting synoptic warming trends across the US Midwest and implications to human health and heat-related mortality. Int J Climatol. (2015) 35:85–96. doi: 10.1002/joc.3964
180. Filho WL, Scheday S, Boenecke J, Gogoi A, Maharaj A, Korovou S. Climate change, health and mosquito-borne diseases: trends and implications to the pacific region. Int J Environ Res Public Health. (2019) 16:5114. doi: 10.3390/ijerph16245114
181. Vora N. Impact of anthropogenic environmental alterations on vector-borne diseases. Medscape J Med. (2008) 10:238.
182. Bowen VB, Braxton J, Davis DW, Flagg EW, Grey J, Grier L, et al. Sexually transmitted disease surveillance 2018. Annual report. (2019). doi: 10.15620/cdc.59237
183. Denning P, DiNenno E. Communities in crisis: is there a generalized HIV epidemic in impoverished urban areas of the United States. In: XVIII International AIDS Conference. (2010).
184. Pellowski JA, Kalichman SC, Matthews KA Adler N, A. pandemic of the poor: Social disadvantage and the US HIV epidemic. Am Psychol. (2013) 68:197–209. doi: 10.1037/a0032694
185. Noppert GA, Clarke P, Hicken MT, Wilson ML. Understanding the intersection of race and place: the case of tuberculosis in Michigan. BMC Public Health. (2019) 19:1669. doi: 10.1186/s12889-019-8036-y
186. Kang SH, Cho DH, Choi J, Baik SK, Gwon JG, Kim MY. Association between chronic hepatitis B infection and COVID-19 outcomes: A Korean nationwide cohort study. PLoS ONE. (2021). 16:e0258229. doi: 10.1371/journal.pone.0258229
187. Kondili LA, Buti M, Riveiro-Barciela M, Maticic M, Negro F, Berg T, et al. Impact of the COVID-19 pandemic on hepatitis B and C elimination: An EASL survey. JHEP Reports. (2022) 4:100531. doi: 10.1016/j.jhepr.2022.100531
188. Pley CM, McNaughton AL, Matthews PC, Lourenço J. The global impact of the COVID-19 pandemic on the prevention, diagnosis and treatment of hepatitis B virus (HBV) infection. BMJ Glob Health. (2021) 6:e004275. doi: 10.1136/bmjgh-2020-004275
189. Formenti B, Gregori N, Crosato V, Marchese V, Tomasoni LR, Castelli F. The impact of COVID-19 on communicable and non-communicable diseases in Africa: a narrative review. Infez Med. (2022) 30:30. doi: 10.53854/liim-3001-4
190. Latini A, Magri F, Donà MG, Giuliani M, Cristaudo A, Zaccarelli M. Is COVID-19 affecting the epidemiology of STIs? The experience of syphilis in Rome. Sex Transm Infect. (2021) 97:78–78. doi: 10.1136/sextrans-2020-054543
191. Chiu NC, Chi H, Tai YL, Peng CC, Tseng CY, Chen CC, et al. Impact of wearing masks, hand hygiene, and social distancing on influenza, enterovirus, and all-cause pneumonia during the coronavirus pandemic: retrospective national epidemiological surveillance study. J Med Internet Res. (2020) 22:e21257. doi: 10.2196/21257
192. Pal M. Lyme disease-an emerging metazoonosis of public health concern. J Clin Immunol Microbiol. (2021) 2:1–4. doi: 10.46889/JCIM.2021.2110
193. Mahalaxmi I, Jayaramayya K, Venkatesan D, Subramaniam MD, Renu K, Vijayakumar P, et al. Mucormycosis: An opportunistic pathogen during COVID-19. Environ Res. (2021) 201:111643. doi: 10.1016/j.envres.2021.111643
194. Maldonado MD, Romero-Aibar J, Pérez-San-Gregorio MA. COVID-19 pandemic as a risk factor for the reactivation of herpes viruses. Epidemiol Infect. (2021) 149:e145. doi: 10.1017/S0950268821001333
195. Wright SS, Kreisel KM, Hitt JC, Pagaoa MA, Weinstock HS, Thorpe PG. Impact of the COVID-19 pandemic on centers for disease control and prevention–funded sexually transmitted disease programs. Sex Transm Dis. (2022) 49:e61–3. doi: 10.1097/OLQ.0000000000001566
196. Stanford KA, Almirol E, Schneider J, Hazra A. Rising syphilis rates during the COVID-19 pandemic. Sex Transm Dis. (2021) 48:e81–3. doi: 10.1097/OLQ.0000000000001431
197. Willis K, Ezer P, Lewis S, Bismark M, Smallwood N. “Covid Just Amplified the Cracks of the System”: Working as a Frontline Health Worker during the COVID-19 Pandemic. Int J Environ Res Public Health. (2021) 18:10178. doi: 10.3390/ijerph181910178
198. Schønning K, Dessau RB, Jensen TG, Thorsen NM, Wiuff C, Nielsen L, et al. Electronic reporting of diagnostic laboratory test results from all healthcare sectors is a cornerstone of national preparedness and control of COVID-19 in Denmark. Apmis. (2021) 129:438–51. doi: 10.1111/apm.13140
199. Beld M, Sentjens R, Rebers S, Weel J, Wertheim-van Dillen P, Sol C, et al. Detection and Quantitation of Hepatitis C Virus RNA in Feces of Chronically Infected Individuals. J Clin Microbiol. (2000) 38:3442–4. doi: 10.1128/JCM.38.9.3442-3444.2000
200. Vaidya SR, Tilavat SM, Kumbhar NS, Kamble MB. Chickenpox outbreak in a tribal and industrial zone from the Union Territory of Dadra and Nagar Haveli, India. Epidemiol Infect. (2018) 146:476–80. doi: 10.1017/S0950268818000201
201. Delhon G. Poxviridae. In: Veterinary Microbiology. (2022). p. 522–32. doi: 10.1002/9781119650836.ch53
202. Sims N, Kasprzyk-Hordern B. Future perspectives of wastewater-based epidemiology: Monitoring infectious disease spread and resistance to the community level. Environ Int. (2020) 139:105689. doi: 10.1016/j.envint.2020.105689
203. Lee WL, Gu X, Armas F, Leifels M, Wu F, Chandra F, et al. Monitoring human arboviral diseases through wastewater surveillance: Challenges, progress and future opportunities. Water Res. (2022) 223:118904. doi: 10.1016/j.watres.2022.118904
204. Guo Y, Sivakumar M, Jiang G. Decay of four enteric pathogens and implications to wastewater-based epidemiology: Effects of temperature and wastewater dilutions. Sci Total Environ. (2022) 819:152000. doi: 10.1016/j.scitotenv.2021.152000
205. Marques PX, Wand H, Nandy M, Tan C, Shou H, Terplan M, et al. Serum antibodies to surface proteins of Chlamydia trachomatis as candidate biomarkers of disease: results from the Baltimore Chlamydia Adolescent/Young Adult Reproductive Management (CHARM) cohort. FEMS Microbes. (2022) 17:3. doi: 10.1093/femsmc/xtac004
206. Tanir G, Kiliçarslan F, Göl N, Arslan Z. Age-spesific seroprevalence and associated risk factors for hepatitis A in children in Ankara, Turkey. J Ankara Med School. (2003) 25:81–8. doi: 10.1501/Jms_0000000048
Keywords: wastewater surveillance, communicable disease (CD), ranking system, COVID-19, emerging disease
Citation: Gentry Z, Zhao L, Faust RA, David RE, Norton J and Xagoraraki I (2023) Wastewater surveillance beyond COVID-19: a ranking system for communicable disease testing in the tri-county Detroit area, Michigan, USA. Front. Public Health 11:1178515. doi: 10.3389/fpubh.2023.1178515
Received: 02 March 2023; Accepted: 12 May 2023;
Published: 02 June 2023.
Edited by:
David Champredon, Public Health Agency of Canada (PHAC), CanadaReviewed by:
Dustin Hill, Syracuse University, United StatesSaber Soltani, Tehran University of Medical Sciences, Iran
Copyright © 2023 Gentry, Zhao, Faust, David, Norton and Xagoraraki. This is an open-access article distributed under the terms of the Creative Commons Attribution License (CC BY). The use, distribution or reproduction in other forums is permitted, provided the original author(s) and the copyright owner(s) are credited and that the original publication in this journal is cited, in accordance with accepted academic practice. No use, distribution or reproduction is permitted which does not comply with these terms.
*Correspondence: Irene Xagoraraki, xagorara@msu.edu
†These authors have contributed equally to this work and share first authorship