- 1Department of Community Nutrition, School of Nutritional Sciences and Dietetics, Tehran University of Medical Sciences (TUMS), Tehran, Iran
- 2Department of Clinical Nutrition, School of Nutritional Science Dietetics, Tehran University of Medical Sciences, Tehran, Iran
- 3Department of Community Nutrition, Faculty of Nutrition and Food Sciences, Tabriz University of Medical Sciences, Tabriz, Iran
- 4Centre for Intelligent Healthcare, Coventry University, Coventry, United Kingdom
Background: Obesity and overweight status increase the risk of cardiovascular disease. Diet quality can also predict the risk of cardiovascular diseases in obese and overweight patients. Therefore, in this study, we sought to examine the relationship between diet quality index (DQI) and cardiometabolic risk factors in obese and overweight women.
Method: A cross-sectional study was conducted on 197 Iranian women with a Body Mass Index (BMI) > 25, 18–48 years, and recruited from 20 Tehran Health Centers. Nutrition intake and DQI were assessed using a 147-item semi-quantitative food frequency questionnaire (FFQ). Additionally, anthropometric measurements, body composition, biochemical evaluations, and cardiometabolic risk factors were evaluated.
Results: There was an association between DQI and waist-to-hip ratio (WHR), atherogenic index of plasma (AIP), and CHOLINDEX in obese women, after adjusting for potential confounders. Whereas, there were no significant associations of the tertiles of DQI compared with the first tertile in other cardiometabolic risk factors, before and after adjustment.
Conclusion: This study provides evidence that dietary intake and DQI are associated with cardiometabolic risk factors and that dietary modification may be a predictor for reducing WHR, AIP, and CHOLINDEX. However, more research is needed to develop a DQI that reflects changes in cardiometabolic risk factors by considering women's eating habits and patterns.
Introduction
Cardiovascular disease (CVD) has been reported as being responsible for 46% of all deaths and 20–23% of the burden of disease in Iran in 2019 (1). The term cardiometabolic risk refers to clinical abnormalities, including obesity, diabetes, and high blood pressure, that should be diagnosed and treated early (2). Furthermore, young women have increasingly prevalent risk factors for CVD (3). However, it is evident that women are less likely to receive preventive treatment, relative to men, and is influenced by behaviors, environment, lifestyle, and nutrition (3).
There are several important cardiometabolic risk factors, including lipid accumulation product (LAP), which is associated with glucose intolerance, CVD, and MetS (4), while the hypertriglyceridemic waist (HW) phenotype (5) is a clinical indicator of visceral obesity diagnosis that may be related to coronary risk factors among women (6). AIP and atherogenic coefficient (AC) are early biomarkers of CVD in developing countries (7), and Castelli Risk Index 1 (CRI-I) and CRI-II are additional, independent, and more precise, risk factors in comparison with traditional lipid parameters (8). Furthermore, an additional simple index, namely CHOLINDEX, considering three main cholesterol parameters, low-density lipoprotein (LDL), high-density lipoprotein (HDL), and triglycerides (TG) levels, has been developed to evaluate cardiometabolic risk (9).
Another relevant predictor of health status is ABSI, an alternative anthropometric measurement that considers height, weight, and waist circumference (WC) and thus more representative of abdominal adipose tissue, and a significant risk factor in predicting premature mortality (10). In line with this, cohort studies have indicated that diabetes and mortality could be predicted by ABSI (11, 12). However, Maessen et al. showed that ABSI was not significantly associated with CVD risks, as compared to BMI and WC in adults (13), and among Iranian adults, it was concluded that ABSI was a weak predictor for CVD risks and MetS (14). In a cohort study conducted on 8,248 participants (4,471 women), the authors aimed to examine whether ABSI could improve the predictive performances for CVD of Framingham's general CVD algorithm. The results showed that ABSI was associated with an increased risk of incident CVD among both men and women but could not improve the predictability of the Framingham algorithm (15). In addition, the triglyceride glucose index (TyG) is one of the methods by which insulin resistance can be estimated (16). Furthermore, it has been indicated that TyG may be associated with high blood pressure, vascular stiffness, and coronary artery calcification (17–19).
Modifiable factors, including physical inactivity and nutrition, may contribute to accelerating cardiometabolic risk (20, 21). Earlier research has largely been concerned with the role of single nutrients and foods in chronic diseases; however, contemporary research has tended to assess the total diet (22, 23). To have a better understanding of overall eating patterns and behaviors in a population, the diet quality index (DQI) has been developed and evaluated (24). In postmenopausal women, it has been demonstrated that a low-quality diet, including the low intake of vegetables and fruits and excessive consumption of sodium, has detrimental impacts on cardiometabolic risk factors, such as abdominal obesity (25). A cross-sectional study based on the Australian Diabetes, Obesity, and Lifestyle study indicated that higher diet quality was significantly associated with cardiometabolic risk factors, including lower blood pressure, lower fasting plasma glucose, and greater insulin sensitivity among men and women (26). Moreover, it was reported that a higher DQI score at baseline was inversely associated with WC, TG, TG to HDL ratio, and the total cholesterol to HDL ratio after 9 years in Spanish adults (27). To our knowledge, the association of DQI with cardiometabolic risk factors and ABSI has scarcely been investigated. Therefore, in this study, we sought to examine the relationship between diet quality index (DQI) and cardiometabolic risk factors in obese and overweight women.
Subjects and methods
This cross-sectional study was conducted using 197 women recruited from 20 Tehran Health Centers, using simple random sampling. The inclusion criteria were as follows: aged from 18 to 48 years, BMI ≥25 kg/m2, without a history of hypertension, no intake of alcohol and opiate drugs, not being pregnant, and not having an acute or chronic infection; and the exclusion criteria were having a history of CVDs, thyroid, cancer, diabetes, liver disease, kidney disease, and smoking. In addition, participants who had been following any arbitrary special dietary regimen, those with chronic disease(s) affecting their diet, or if their daily energy intake was < 800 or >4,200 kcal (28) were excluded. All participants were asked to sign the written consent before the study commencement, and the study was approved by the ethics committee of Tehran University of Medical Sciences (R.TUMS.VCR.REC.1395.1234).
Anthropometric measurements
The body compositions, including weight, fat and lean mass, and WHR, of the female participants were assessed by the bioelectric impedance analyzer (In Body 770 scanner, Korea) (29). In addition, height was measured to the nearest 0.5 cm to evaluate BMI as weight (kg) divided by height (m2). WC measurement was performed at the point of the umbilicus after exhalation. According to the World Health Organization (WHO) criteria for the classification of weight, a BMI of ≥ 25 kg/m2 was considered overweight, and a BMI of ≥ 30 kg/m2 was considered obesity (30).
Biochemistry measurements
Blood samples were drawn after 12 h of overnight fasting to assess LDL, HDL-C, TG, fasting blood sugar (FBS), and total cholesterol by Pars Azmoon laboratory kits (test Pars Inc, Tehran, Iran). The serum was separated and stored at a temperature of −70°C until the analyses were carried out after centrifugation. Levels of TG, total cholesterol, HDL-C, LDL-C, and fasting blood sugar (FBS) were measured using glycerol-3-phosphate oxidase phenol 4-amino antipyrine peroxidase (GPO-PAP), enzymatic endpoint, direct enzymatic clearance, and glucose oxidase phenol 4-amino, respectively.
Cardiometabolic risk factors calculation
ABSI = (10);
LAP index =(WC (cm)-58) × TG (mmol) (31);
AIP: (32);
AC: (TC– HDL)/HDL (8);
CRI-I , respectively (33);
CRI-II , respectively (33);
TyG = Ln [fasting triglycerides (mg/dL) × fasting glucose (mg/dL)/2] (16);
CHOLINDEX =LDL-C-HDL-C (TG < 400 mg/dL), LDL-C-HDL-C + 1/5 of TG (TG ≥ 400 mg/dL) (9);
TyG-BMI (triglyceride glucose index- body mass index) = Ln [fasting plasma glucose (mg/dL) × fasting triglycerides (mg/dL)/2] × BMI (34);
TyG-WC (triglyceride glucose index- waist circumference) = Ln [fasting plasma glucose (mg/dL) × fasting triglycerides (mg/dL)/2] × WC (35).
The HW phenotype was defined by the presence of increased WC (>88 percentile by age and sex of the sample itself) and increased serum triglycerides (>100 mg/DL) (36).
Dietary intake measurement and DQI calculation
The dietary intake of the women was collected by an instructing nutritionist via a face-to-face interview with a 147-item semi-quantitative food frequency questionnaire (FFQ), where its validity and reliability were previously avowed in the Iranian population (37). The average consumption frequency was considered over the past year on a daily, weekly, and monthly basis. Household measures were taken into account for portion sizes and then converted to grams (38). The food composition table (FCT) of the United States Department of Agriculture (USDA) was used to evaluate energy and nutrients. The Iranian FCT was considered for local foods that were not present in the USDA FCT. There are seven food groups considered in the DQI, which are as follows: fast food (four items); vegetables and fruit (seven items); legumes, chicken, soy protein or fish (four items); sweets (six items); butter, hydrogenated oil, animal fats, or ghee (four items); egg, whole dairy products, or meat (four items); and olive and non-hydrogenated oil (two items). The calculation of this index is based on adequacy, variety, moderation, and balance (39). Variety was assessed by two components: “between-food groups” (0–15 points) and “within-protein source group” (0–5 points). Adequacy evaluates fruits, vegetables, grains, protein, fiber, calcium, iron, and vitamin C intakes (40 points). Moderation assesses total fat, saturated fat, cholesterol, sodium, and empty-calorie foods (30 points). The balance of the micronutrient distribution in the diet and the fatty acid ratio are also examined (10 points). Details about the scoring method of DQI were published previously (40). The whole DQI score is 100, such that a higher score indicates a better quality of the diet. Ultimately, the DQI score of participants was categorized based on tertiles.
Physical activity (PA) measurement
A valid and reliable international PA questionnaire (IPAQ), designed by the WHO and validated in Iranian women adults, was used to assess PA levels (41). The participants were asked to answer questions such as the time they spent on walking, moderate, and vigorous PA during the last week. Then, the time of each PA was converted to minutes per week and calculated as the metabolic equivalent of the task (MET/minutes/week).
Other covariates assessments
Demographic characteristics, including age, marital status, supplement consumption (multivitamins and minerals), socioeconomic status, education, and occupation status were collected.
Blood pressure measurements
Systolic and diastolic blood pressure (SBP and DBP) were evaluated after 15-min rest, using a mercury sphygmomanometer.
Statistical analysis
In this study, SPSS software version 26 (Chicago, USA) was used for all data analysis, and a p-value of < 0.05 was considered statistically significant. Participants were categorized according to tertiles of DQI. Kolmograph–Smirnov test and histogram inspection were used to determine the normal distribution of the data. All variables with normal distribution were analyzed by parametric tests, where one-way analysis of variance (ANOVA) for continuous variables and chi-square analysis for categorical variables were used to compare subject characteristics and dietary intake across tertiles of DQI score, reported as mean (SD). Analysis of covariance (ANCOVA) was used to examine demographic characteristics, anthropometric measurements, clinical assessments, and dietary intake across tertiles of DQI score via adjusting for age, BMI, physical activity, and energy intake in model 1 and education, economic status, and supplement consumption in model 2. Notably, BMI, as a colinear variable, was not adjusted for outcomes including BMI, BFM, WHR, WC, TyG-BMI, ti, LAP, and ABSI in model 1. To examine the association between DQI score and cardiometabolic risk factors, a binary logistic regression model was applied and reported as β and 95% confidence interval (CI).
Results
Study population characteristics
In total, 197 women with a mean age and BMI of 35.5 years and 30.5 kg/m2, respectively, participated in this study. The baseline characteristics of participants were presented in Table 1.
Baseline characteristics of participants across the tertiles of DQI scores
The characteristics of participants across the tertiles of DQI scores in overweight and obese women are shown in Table 2. Participants with a higher score of DQI were more physically active (P: 0.002), before and after adjusting for age, energy intake, BMI, and physical activity. Interestingly, there was a statistically significant age difference among women across the tertiles of DQI scores after controlling for confounders (P: 0.011), but not in the crude model (P: 0.118). However, there were no significant differences between tertiles in other characteristics.
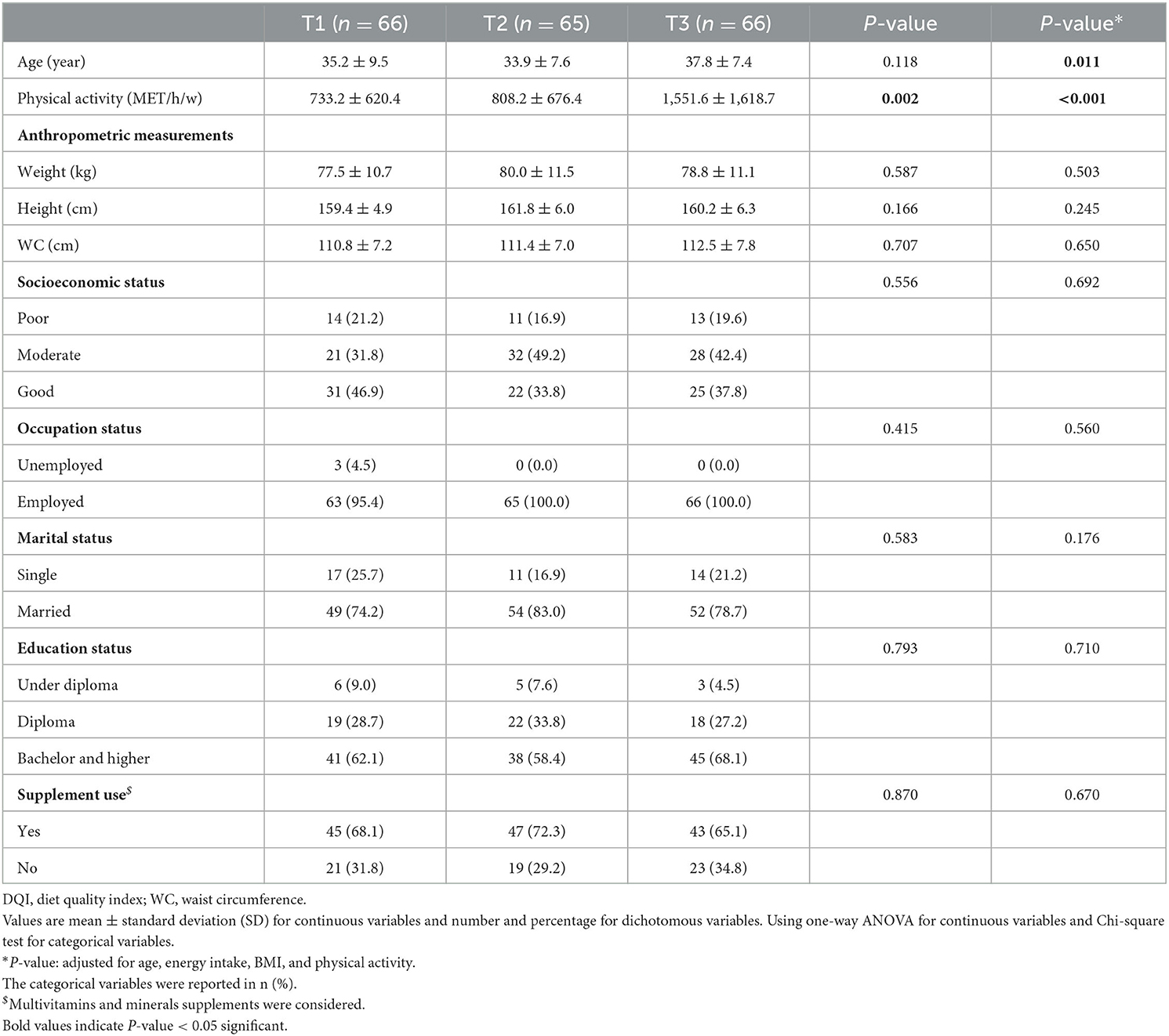
Table 2. General characteristics of participants across tertiles of DQI among overweight and obese women (n = 197).
Dietary intake of participants across the tertiles of DQI scores
The dietary intake of participants across the tertiles of DQI scores in overweight and obese women is presented in Table 3. Before adjusting for confounding factors, the consumptions of energy (P: 0.041), protein (P: 0.028), and carbohydrate (P < 0.001) increased from the first to the third tertiles of DQI. Women who adhered highly to DQI had a lower intake of total fat (P: 0.006), saturated fatty acids (P: 0.006), and mono-unsaturated fatty acids (P: 0.003); while, in contrast, results indicated a higher intake of iron (P < 0.001), calcium (P: 0.006), magnesium (P < 0.001), zinc (P: 0.006), selenium (P: 0.001), β carotene (P: 0.048), vitamin D (P: 0.049), vitamin B1 (P: 0.001), vitamin B6 (P: 0.009), folate (P < 0.001), and total fiber (P < 0.001). Regarding food groups, it should be mentioned that refined (P: 0.015) and whole (P: 0.002) grains, fruits (P < 0.001), vegetables (P: 0.001), and legumes (P: 0.001) were also consumed in higher quantities by women with a higher score of DQI. After controlling for energy intake, some nutrients remained statistically significant as follows: carbohydrate (P < 0.001), total fat (P < 0.001), saturated fatty acids (P < 0.001), mono-unsaturated fatty acids (P < 0.001), iron (P < 0.001), calcium (P: 0.039), magnesium (P < 0.001), zinc (P: 0.025), selenium (P: 0.029), thiamin (P: 0.001), folate (P < 0.001), and total fiber (P < 0.001). Also, whole grains (P: 0.009), fruits (P: 0.009), vegetables (P: 0.011), and legumes (P: 0.002) were the same as before adjustments. However, other nutrients showed no significant results across tertiles of DQI, before or after adjustment.
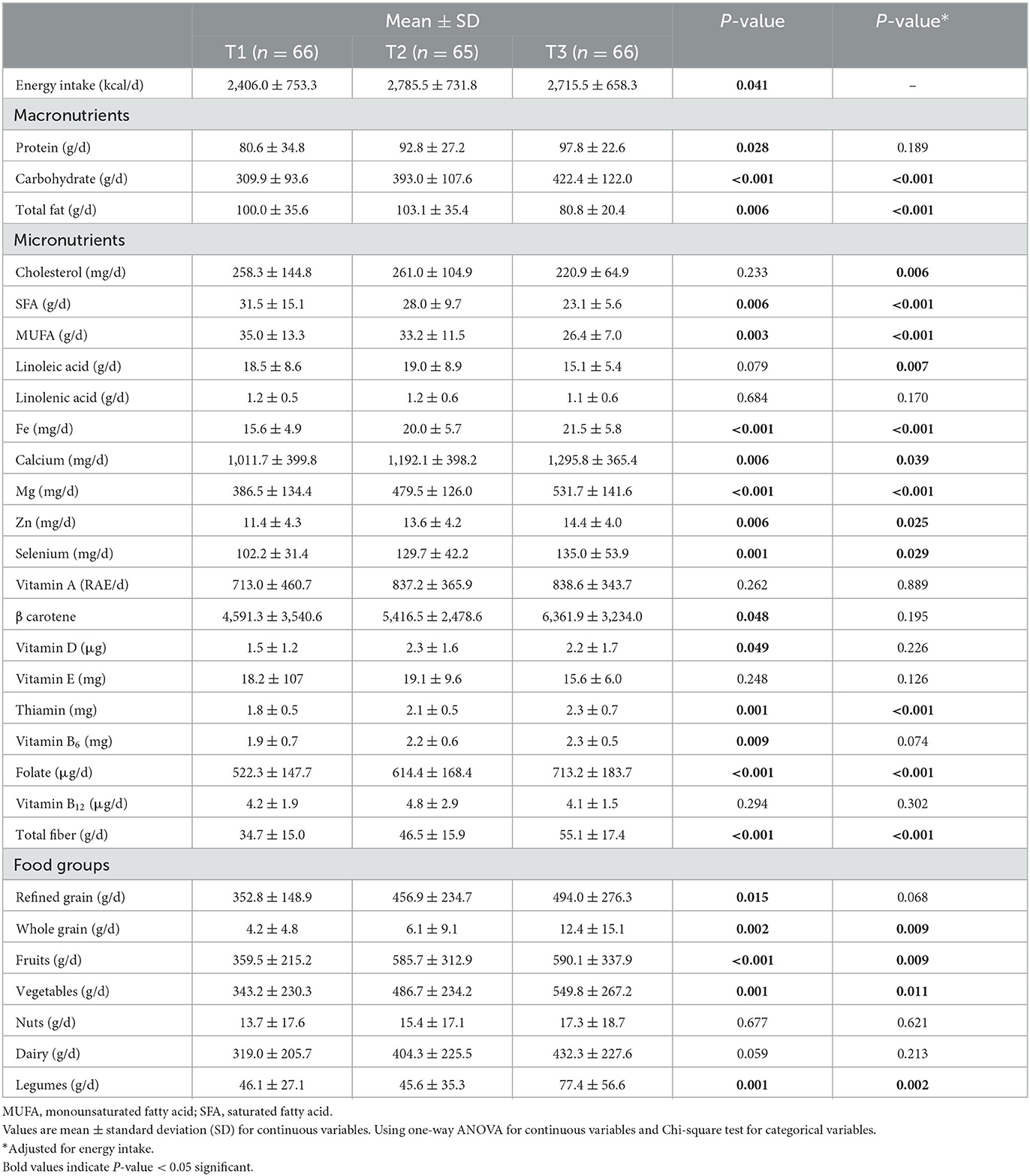
Table 3. Dietary intake of participants across tertiles of DQI among overweight and obese women (n = 197).
Association of DQI with cardiometabolic risk factors and ABSI
The associations of DQI with cardiometabolic risk factors in overweight and obese women are shown in Table 4. There were significant associations between DQI and WHR (β: −0.014, 95% CI: −0.039, 0.011, P-trend: 0.047), AIP (β: −0.086, 95% CI: −0.207, −0.006, P-trend: 0.027), and CHOLINDEX (β: −4.998, 95% CI: −15.597, 0.000, P-trend: 0.031) with the third tertile of DQI scores, as compared to people in the first tertile, in obese women after adjusting for age, BMI, PA, energy intake, education, socioeconomic status, and supplement use. However, in other cardiometabolic risk factors, no significant relationship was seen after adjusting for potential confounders. In the crude model, women who were in the second tertile of DQI had a lower score of TyG-BMI (β: −5.245, 95% CI: −22.555, −1.065, P: 0.043) and TyG-WC (β: −31.464, 95% CI: −91.980, −9.052, P: 0.038) than women in the first tertile, which was statistically significant. Although, this significant relationship disappeared after adjusting for potential confounders. Despite the level of other cardiometabolic risk factors, including BMI, BFM, WC, FBS, cholesterol, TG, HDL, LDL, SBP, DBP, ABSI, CRI-I and II, TyG, LAP, and AC, before and after adjustments, was lower in the third tertile of DQI compared to the first tertile, this relationship was not significant (P-value > 0.05).
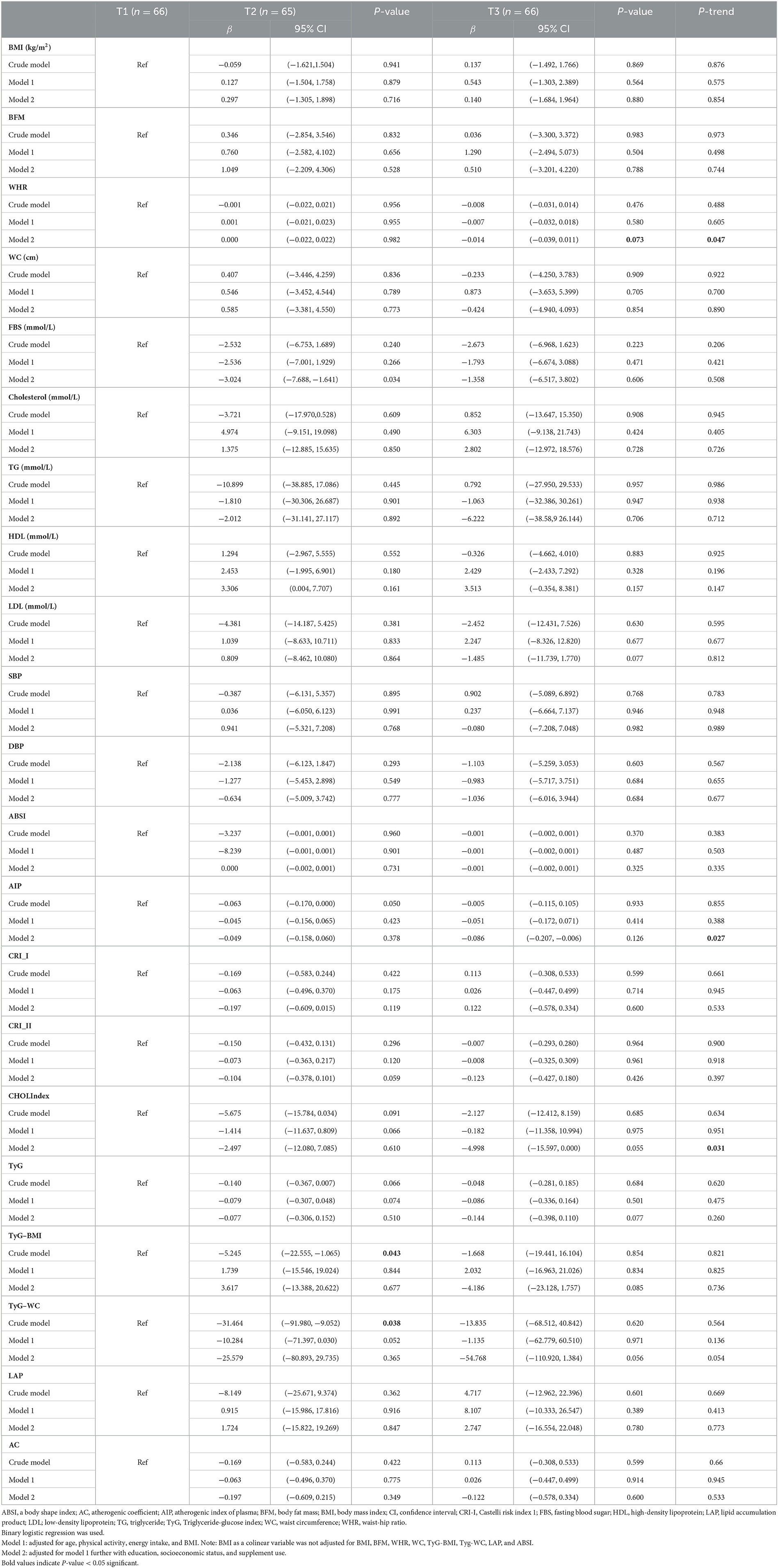
Table 4. Association of DQI with cardiometabolic risk factors among overweight and obese women (n = 197).
Discussion
This cross-sectional study was conducted to evaluate the association between DQI and cardiometabolic risk factors and ABSI in Iranian women. Accordingly, our findings showed that a high-quality diet in women, assessed by adherence to the DQI, was inversely associated with cardiometabolic risk factors, including WHR, AIP, CHOLINDEX, TyG-BMI, and TyG-WC. There were no significant associations between DQI and other cardiometabolic risk factors and anthropometric indices, including ABSI in women.
Previous studies have shown that a high-quality diet can contribute to the maintenance of cardiovascular health in young and middle-aged adults (42–44). For instance, following a modified Mediterranean diet in the Coronary Artery Risk Development in Young Adults (CARDIA) study led to a reduction of metabolic disorders in middle-aged participants (43). Moreover, higher Alternate Healthy Eating Index scores in the China Health and Nutrition Survey (CHNS) study were related to lower odds of disorders, such as diabetes, and low LDL levels in male participants (45). In a cross-sectional study, it was demonstrated that a higher DASH score was associated with lower insulin resistance and lower serum levels of LDL, HDL, and VLDL, while subjects in the highest DASH quartile had lower odds of metabolic syndrome (46). In the cross-sectional Australian Health Survey, higher scores on the Dietary Guideline Index (DGI) were associated with lower glucose, body mass index, and waist circumference (47). On the other hand, a systematic review and meta-analysis demonstrated that there was no considerable relation between dietary diversity score and most CMRs (48). However, little is known regarding the association between DQI and cardiometabolic risk factors and anthropometric indices.
In a cohort study, a 13-year follow-up among 4,390 adult participants indicated that lower scores of DQI were associated with higher scores of impaired HDL, FBG, LDL, and WC (49). In a cross-sectional study, which was carried out in 2021, it was observed that DQI score was inversely associated with serum level of TC, but positively associated with HDL (50). Alkerwi et al., in the Observation of Cardiovascular Risk Factors in Luxembourg (ORISCAV-LUX) study, found an inverse association between DQI score and total cholesterol, LDL, and HDL levels (51). In a cohort study among 451 participants, after 6.7 years of follow-up, a positive association was found between DQI score and HDL, although only in male participants (52). In a study by Kim et al., DQI had a significant association with fasting plasma glucose in diabetic patients (53). Moreover, results of a 10-year follow-up cohort study, across 10 European countries with over 450,000 participants, indicated that DQI scores were inversely associated with cardiovascular disease mortality and its risk factors, including blood lipids and glucose (54).
Based on our results, the DQI score was not related to the serum glycemic and lipid profile markers; however, the DQI score was inversely associated with CHOLINDEX, TyG-BMI, and TyG-WC, which are related indices to insulin resistance and lipid parameters. In a cross-sectional study conducted on patients with type 2 diabetes, there was no significant relationship between DQI scores and glycemic status and lipid profile parameters in diabetic patients (55). In addition, Daneshzad et al. did not observe any significant association between DQI and CVD risk factors in a cross-sectional study on diabetic women (56). However, in the Korea National Health and Nutritional Examination Survey, higher scores of DQI in middle-aged and older women were associated with a lower risk of body composition abnormalities (57), while Gregory et al.'s DQI was positively associated with BMI and WC (58). Nevertheless, in a cross-sectional study from the results of the Tehran Lipid and Glucose Study, no significant association was observed between DQI and BMI and WC (59). In this study, our results suggested that DQI was inversely associated with WHR, but there was no association between DQI and BMI, BFM, and WC. According to the extant literature, possible reasons for the inconsistency of the published results could be related to the differences in the sample size, different designs of the studies, and differences in the health status of participants.
The present results of the study showed that women with the highest score of DQI had a higher intake of whole grains, fruits, vegetables, legumes, and total fiber, in addition to a lower intake of total fat and saturated fatty acids. It has been reported that a high-quality diet is related to a decreased risk of mortality caused by cardiovascular diseases (60). Dietary combinations can have considerable efficacy on insulin sensitivity (61). The types of fat consumption have been investigated and discussed as a factor in cardiovascular (62), as part of nutritional planning, and for the increase of insulin sensitivity (63). The quality of dietary fats can affect the composition of cell membranes and their functions and also cause to alter in insulin signaling pathways in tissues (64). For example, saturated fatty acids can induce insulin resistance by reducing the release of adiponectin and disrupting insulin signaling pathways associated with glucose uptake in adipose tissue. In addition, fatty acids lead to insulin resistance by causing chronic and mild inflammation through inflammatory cytokines, lipids and their metabolites, and reactive oxygen species (65–67). The saturated fatty acid could cause activates serine kinase proteins, leading to inflammatory pathways, and through reduction tyrosine phosphorylation of insulin receptor substrate 1, adverse affect insulin transportation (57). In addition, other negative results of its consumption include formation of antagonists against insulin activity, such as sphingolipids (68), and the expression of nuclear factor kappa B (NF-kB) and cyclooxygenase-2 (COX-2) (69). Intake of complex carbohydrates, such as fiber, and sources rich in polyphenols and antioxidants, including vegetables, fruits, and legumes, can contribute to an ameliorated cardiovascular status, due to their anti-inflammatory properties (70–72). Dietary fiber intake can, through its fermentation by intestinal bacteria and the production of short-chain fatty acids, lead to a reduction in the synthesis of cholesterol in the body (73, 74). The short-chain fatty acids lead to a decrease in the levels of C-reactive protein and interleukin-6 (75, 76). The benefits of polyphenols are related to their anti-inflammatory and antioxidant properties (77) because they can reduce the release of NO and PGE2 by suppressing the expression of iNOS and PGE2, and also reduce the release of IL-1b and TNF-a, an effect that has been related to suppressing activation of the NF-kB pathway (78, 79). Consumption of these products leads to ameliorate regulation of fat and carbohydrate metabolism, improvement of hyperglycemia, a decrease of dyslipidemia, increased sensitivity to insulin, amendment of adipose tissue metabolism, and decrease in oxidative stress (80). One of the most well-known effects of polyphenols on carbohydrate metabolism is the suppression of the function of the key enzymes (including alpha-glucosidase and alpha-amylase) responsible for its digestion in the gastrointestinal tract (81, 82). Some types of polyphenols can interfere with the absorption of glucose from the small intestine by inhibiting sodium-dependent glucose transporters, such as SGLT1 (83, 84). Polyphenolic ingredients regulated at blood sugar level after meals and improved glucose intolerance by facilitating insulin response and reducing the secretion of hormones such as glucose-dependent insulinotropic polypeptide (GIP) and glucagon-like polypeptide-1 (GLP-1) (85, 86). Some polyphenols can regulate the lipolysis mechanism through the induction of adipose tissue lipase, hormone sensitive lipase, enhances gene expression of mitochondrial uncoupling protein 2 (UCP-2) and carnitin palmitoyl transferase-1 (CPT-1) (87, 88). Most polyphenolic compounds, because of their particular structure, have been known as antioxidant phytochemicals (89). They improve the oxidant-antioxidant equilibrium through the endogenous antioxidant system. These bioactive factors diminish lipid peroxidation and increase the total antioxidant capacity of plasma. In addition, they induce enzymes including superoxide dismutase, catalase, and glutathione peroxidase (90).
This study represents a novel addition to the literature, where we elucidate the relationship between DQI and cardiometabolic risk factors in obese and overweight women. Indeed, among the strengths of our study, unlike most of the studies conducted on the relationship between DQI and cardiometabolic risk factors, which only used BMI, WC, and usual glycemic and lipid serum parameters, our study also investigated the relationship of other atherogenic indices, lipid ratios, and anthropometric indices. Nevertheless, despite the strength and novelty of this study, some limitations should be noted. First, the sample included only women; although this was a purposeful decision, based on the dearth of data in the literature. In addition, since this study was performed only in one province, the results cannot be attributed to the total population. Furthermore, using FFQ makes measurement errors inevitable; these largely emanant from recall and other subjective biases. Finally, because of the cross-sectional design of the study, causal inferences cannot be made.
Conclusion
In this study, we showed that the higher DQI score was associated with lower WHR, AIP, CHOLINDEX, TyG-BMI, and TyG-WC in overweight and obese women. However, more studies are needed to confirm these findings and elucidate their mechanistic etiology in the future.
Data availability statement
The raw data supporting the conclusions of this article will be made available by the authors, without undue reservation.
Ethics statement
Ethics approval and consent to participate was presented in article. Each participant was informed completely regarding the study protocol and provided a written and informed consent form before taking part in the study. The study protocol was approved by the ethics committee of Tehran University of Medical Sciences (TUMS) with the following identification (R.TUMS.VCR.REC.1395.1234.).
Author contributions
AMo, AMi, and KM designed the search. AMi and MG conducted the sampling. FS performed statistical analysis. AMo, AA, and CC wrote the manuscript. CC and YA revised the article. KM and AMi have responsibility for the final content. All authors have read and approved the final manuscript.
Funding
This study was supported by Tehran University of Medical Sciences.
Conflict of interest
The authors declare that the research was conducted in the absence of any commercial or financial relationships that could be construed as a potential conflict of interest.
Publisher's note
All claims expressed in this article are solely those of the authors and do not necessarily represent those of their affiliated organizations, or those of the publisher, the editors and the reviewers. Any product that may be evaluated in this article, or claim that may be made by its manufacturer, is not guaranteed or endorsed by the publisher.
Abbreviations
BMI, body mass index; DBP, diastolic blood pressure; FBS, fasting blood sugar; HDL, high-density lipoprotein; LDL, low-density lipoprotein; SBP, systolic blood pressure; WHR, waist-hip ratio; CI, confidence interval; OR, odds ratio; WC, waist circumference; DASH, Dietary Approaches to Stop Hypertension; WHO, World Health Organization; FFQ, food frequency questionnaire; FCT, food composition table; USDA, United States Department of Agriculture; IPAQ, physical activity questionnaire; MetS, metabolic syndrome; CVD, cardiovascular disease; BFM, body fat mass; TG, triglyceride; ABSI, a body shape index; CRI- I, CRI- II, Castelli Risk Index; TyG, triglyceride glucose index; LAP, Lipid accumulation product; AC, atherogenic coefficient; AIP, Atherogenic index of plasma; TyG-BMI, triglyceride glucose index- body mass index; TyG-WC, triglyceride glucose index- waist circumference; HW, hypertriglyceridemic waist.
References
1. Sarrafzadegan N, Mohammmadifard N. Cardiovascular disease in Iran in the last 40 years: prevalence, mortality, morbidity, challenges and strategies for cardiovascular prevention. Arch Iran Med. (2019) 22:204–10.
2. Cannon CP. Cardiovascular disease and modifiable cardiometabolic risk factors. Clin Cornerstone. (2007) 8:11–28. doi: 10.1016/S1098-3597(07)80025-1
3. Garcia M, Mulvagh SL, Bairey Merz CN, Buring JE, Manson JE. Cardiovascular disease in women: clinical perspectives. Circ Res. (2016) 118:1273–93. doi: 10.1161/CIRCRESAHA.116.307547
4. Kahn HS. The “lipid accumulation product” performs better than the body mass index for recognizing cardiovascular risk: a population-based comparison. BMC Cardiovasc Disord. (2005) 5:1–10. doi: 10.1186/1471-2261-5-26
5. Brisson D, Perron P, Guay SP, Gaudet D, Bouchard L. The “hypertriglyceridemic waist” phenotype and glucose intolerance in pregnancy. CMAJ. (2010) 182:E722–5. doi: 10.1503/cmaj.100378
6. LaMonte MJ, Ainsworth BE, DuBose KD, Grandjean PW, Davis PG, Yanowitz FG, et al. The hypertriglyceridemic waist phenotype among women. Atherosclerosis. (2003) 171:123–30. doi: 10.1016/j.atherosclerosis.2003.07.008
7. Fernández-Macías JC, Ochoa-Martínez AC, Varela-Silva JA, Pérez-Maldonado IN. Atherogenic index of plasma: novel predictive biomarker for cardiovascular illnesses. Arch Med Res. (2019) 50:285–94. doi: 10.1016/j.arcmed.2019.08.009
8. Bhardwaj S, Bhattacharjee J, Bhatnagar M, Tyagi S, Delhi N. Atherogenic index of plasma, castelli risk index and atherogenic coefficient-new parameters in assessing cardiovascular risk. Int J Pharm Biol Sci. (2013) 3:359–64.
9. Akpinar O, Bozkurt A, Acarturk E, Seydaoglu G. A new index (CHOLINDEX) in detecting coronary artery disease risk. Anatol J Cardiol. (2013) 13:98. doi: 10.5152/akd.2013.098
10. Krakauer NY, Krakauer JC. A new body shape index predicts mortality hazard independently of body mass index. PLoS ONE. (2012) 7:e39504. doi: 10.1371/journal.pone.0039504
11. He S, Chen X. Could the new body shape index predict the new onset of diabetes mellitus in the Chinese population? PLoS ONE. (2013) 8:e50573. doi: 10.1371/journal.pone.0050573
12. Krakauer NY, Krakauer JC. Dynamic association of mortality hazard with body shape. PLoS ONE. (2014) 9:e88793. doi: 10.1371/journal.pone.0088793
13. Maessen MF, Eijsvogels TM, Verheggen RJ, Hopman MT, Verbeek AL, Vegt FD. Entering a new era of body indices: the feasibility of a body shape index and body roundness index to identify cardiovascular health status. PLoS ONE. (2014) 9:e107212. doi: 10.1371/journal.pone.0107212
14. Haghighatdoost F, Sarrafzadegan N, Mohammadifard N, Asgary S, Boshtam M, Azadbakht L. Assessing body shape index as a risk predictor for cardiovascular diseases and metabolic syndrome among Iranian adults. Nutrition. (2014) 30:636–44. doi: 10.1016/j.nut.2013.10.021
15. Bozorgmanesh M, Sardarinia M, Hajsheikholeslami F, Azizi F, Hadaegh F. CVD-predictive performances of “a body shape index” versus simple anthropometric measures: Tehran lipid and glucose study. Eur J Nutr. (2016) 55:147–57. doi: 10.1007/s00394-015-0833-1
16. Simental-Mendía LE, Rodríguez-Morán M, Guerrero-Romero F. The product of fasting glucose and triglycerides as surrogate for identifying insulin resistance in apparently healthy subjects. Metab Syndr Relat Disord. (2008) 6:299–304. doi: 10.1089/met.2008.0034
17. Lee SB, Ahn CW, Lee BK, Kang S, Nam JS, You JH, et al. Association between triglyceride glucose index and arterial stiffness in Korean adults. Cardiovasc Diabetol. (2018) 17:1–6. doi: 10.1186/s12933-018-0692-1
18. Kim MK, Ahn CW, Kang S, Nam JS, Kim KR, Park JS. Relationship between the triglyceride glucose index and coronary artery calcification in Korean adults. Cardiovasc Diabetol. (2017) 16:1–7. doi: 10.1186/s12933-017-0589-4
19. Lambrinoudaki I, Kazani MV, Armeni E, Georgiopoulos G, Tampakis K, Rizos D, et al. The TyG index as a marker of subclinical atherosclerosis and arterial stiffness in lean and overweight postmenopausal women. Heart Lung Circul. (2018) 27:716–24. doi: 10.1016/j.hlc.2017.05.142
20. Laslett LJ, Alagona P, Clark BA, Drozda JP, Saldivar F, Wilson SR, et al. The worldwide environment of cardiovascular disease: prevalence, diagnosis, therapy, and policy issues: a report from the American College of Cardiology. J Am Coll Cardiol. (2012) 60:S1–S49. doi: 10.1016/j.jacc.2012.11.002
21. Jardim TV, Mozaffarian D, Abrahams-Gessel S, Sy S, Lee Y, Liu J, et al. Cardiometabolic disease costs associated with suboptimal diet in the United States: a cost analysis based on a microsimulation model. PLoS Med. (2019) 16:e1002981. doi: 10.1371/journal.pmed.1002981
22. Newby P, Tucker KL. Empirically derived eating patterns using factor or cluster analysis: a review. Nutr Rev. (2004) 62:177–203. doi: 10.1111/j.1753-4887.2004.tb00040.x
23. Waijers PM, Feskens EJ, Ocké MC. A critical review of predefined diet quality scores. Br J Nutr. (2007) 97:219–31. doi: 10.1017/S0007114507250421
24. Gil Á, de Victoria EM, Olza J. Indicators for the evaluation of diet quality. Nutricion Hospitalaria. (2015) 31:128–44.
25. de Almeida Ventura D, de Matos Fonseca V, Ramos EG, Marinheiro LPF, de Souza RAG, de Miranda Chaves CRM, et al. Association between quality of the diet and cardiometabolic risk factors in postmenopausal women. Nutr J. (2014) 13:1–10. doi: 10.1186/1475-2891-13-121
26. McNaughton SA, Dunstan DW, Ball K, Shaw J, Crawford D. Dietary quality is associated with diabetes and cardio-metabolic risk factors. J Nutr. (2009) 139:734–42. doi: 10.3945/jn.108.096784
27. Funtikova A, Baena-Díez J, Koebnick C, Gomez S, Covas M, Goday A, et al. Validity of a short diet-quality index to predict changes in anthropometric and cardiovascular risk factors: a simulation study. Eur J Clin Nutr. (2012) 66:1369–71. doi: 10.1038/ejcn.2012.131
28. Willett W. Issues in analysis and presentation of dietary data. Nutrition Epidemiol. (1998) 13:321–46. doi: 10.1093/acprof:oso/9780195122978.003.13
29. Mirzababaei A, Shiraseb F, Abaj F, Khosroshahi RA, Tavakoli A, Koohdani F, et al. The effect of dietary total antioxidant capacity (DTAC) and Caveolin-1 gene variant interaction on cardiovascular risk factors among overweight and obese women: a cross-sectional investigation. Clin Nutr. (2021) 40:4893–903. doi: 10.1016/j.clnu.2021.07.013
30. WHO. Nutrition. (2023). Available online at: https://www.euro.who.int/en/health-topics/disease-prevention/nutrition/a-healthy-lifestyle/body-mass-index-bmi (accessed 2023).
31. Vieira BA, Sauer P, Marcadenti A, Piovesan CH. Association between LAP Index (Lipid Accumulation Product) and metabolic profile in hospitalized patients. Nutr Hosp. (2015) 31:2771–4.
32. Shen S, Lu Y, Qi H, Li F, Shen Z, Wu L, et al. Association between ideal cardiovascular health and the atherogenic index of plasma. Medicine. (2016) 95:3866. doi: 10.1097/MD.0000000000003866
33. Bhardwaj S, Bhattacharjee J, Bhatnagar M, Tyagi S. Atherogenic index of plasma, castelli risk index and atherogenic coefficient-new parameters in assessing cardiovascular risk. Int J Pharm Biol Sci. (2013) 3:359–64.
34. Wang X, Liu J, Cheng Z, Zhong Y, Chen X, Song W. Triglyceride glucose-body mass index and the risk of diabetes: a general population-based cohort study. Lipids Health Dis. (2021) 20:1–10. doi: 10.1186/s12944-021-01532-7
35. Hameed EK. TyG index a promising biomarker for glycemic control in type 2 Diabetes Mellitus. Diabetes Metab Syndr. (2019) 13:560–3. doi: 10.1016/j.dsx.2018.11.030
36. Costa PRF, Assis AMO, Cunha CM, Pereira EM, Jesus GDS, Silva L, et al. Hypertriglyceridemic waist phenotype and changes in the fasting glycemia and blood pressure in children and adolescents over one-year follow-up period. Arq Bras Cardiol. (2017) 109:47–53. doi: 10.5935/abc.20170067
37. Mirmiran P, Esfahani FH, Mehrabi Y, Hedayati M, Azizi F. Reliability and relative validity of an FFQ for nutrients in the Tehran lipid and glucose study. Public Health Nutr. (2010) 13:654–62. doi: 10.1017/S1368980009991698
38. Ghaffarpour M, Houshiar-Rad A, Kianfar H. The manual for household measures, cooking yields factors and edible portion of foods. Tehran. (1999) 7:42–58.
39. Kim S, Haines PS, Siega-Riz AM, Popkin BM. The Diet Quality Index-International (DQI-I) provides an effective tool for cross-national comparison of diet quality as illustrated by China and the United States. J Nutr. (2003) 133:3476–84. doi: 10.1093/jn/133.11.3476
40. Mohammadifard N, Kelishadi R, Safavi M, Sarrafzadegan N, Sajadi F, Sadri GH, et al. Effect of a community-based intervention on nutritional behaviour in a developing country setting: the Isfahan Healthy Heart Programme. Public Health Nutr. (2009) 12:1422–30. doi: 10.1017/S1368980008004230
41. Bassett DR Jr. International physical activity questionnaire: 12-country reliability and validity. Med Sci Sports Exer. (2003) 35:1396. doi: 10.1249/01.MSS.0000078923.96621.1D
42. Chomistek AK, Chiuve SE, Eliassen AH, Mukamal KJ, Willett WC, Rimm EB. Healthy lifestyle in the primordial prevention of cardiovascular disease among young women. J Am Coll Cardiol. (2015) 65:43–51. doi: 10.1016/j.jacc.2014.10.024
43. Steffen LM, Van Horn L, Daviglus ML, Zhou X, Reis JP, Loria CM, et al. A modified Mediterranean diet score is associated with a lower risk of incident metabolic syndrome over 25 years among young adults: the CARDIA (Coronary Artery Risk Development in Young Adults) study. Br J Nutr. (2014) 112:1654–61. doi: 10.1017/S0007114514002633
44. Liu K, Daviglus ML, Loria CM, Colangelo LA, Spring B, Moller AC, et al. Healthy lifestyle through young adulthood and the presence of low cardiovascular disease risk profile in middle age: the Coronary Artery Risk Development in (Young) Adults (CARDIA) study. Circulation. (2012) 125:996–1004. doi: 10.1161/CIRCULATIONAHA.111.060681
45. Wang Z, Siega-Riz AM, Gordon-Larsen P, Cai J, Adair LS, Zhang B, et al. Diet quality and its association with type 2 diabetes and major cardiometabolic risk factors among adults in China. Nutr Metabol Cardiovasc Dis. (2018) 28:987–1001. doi: 10.1016/j.numecd.2018.06.012
46. Phillips CM, Harrington JM, Perry IJ. Relationship between dietary quality, determined by DASH score, and cardiometabolic health biomarkers: a cross-sectional analysis in adults. Clin Nutr. (2019) 38:1620–8. doi: 10.1016/j.clnu.2018.08.028
47. Livingstone KM, McNaughton SA. Association between diet quality, dietary patterns and cardiometabolic health in Australian adults: a cross-sectional study. Nutr J. (2018) 17:1–13. doi: 10.1186/s12937-018-0326-1
48. Qorbani M, Mahdavi-Gorabi A, Khatibi N, Ejtahed H-S, Khazdouz M, Djalalinia S, et al. Dietary diversity score and cardio-metabolic risk factors: an updated systematic review and meta-analysis. Eating Weight Disord Stud Anorexia Bulimia Obesity. (2022) 27:85–100. doi: 10.1007/s40519-020-01090-4
49. Nouri F, Sadeghi M, Mohammadifard N, Roohafza H, Feizi A, Sarrafzadegan N. Longitudinal association between an overall diet quality index and latent profiles of cardiovascular risk factors: results from a population based 13-year follow up cohort study. Nutr Metab. (2021) 18:1–13. doi: 10.1186/s12986-021-00560-5
50. Zamani B, Daneshzad E, Mofrad MD, Namazi N, Larijani B, Bellissimo N, et al. Dietary quality index and cardiometabolic risk factors among adult women. Iran J Public Health. (2021) 50:1713. doi: 10.18502/ijph.v50i8.6819
51. Alkerwi AA, Vernier C, Crichton GE, Sauvageot N, Shivappa N, Hébert JR. Cross-comparison of diet quality indices for predicting chronic disease risk: findings from the Observation of Cardiovascular Risk Factors in Luxembourg (ORISCAV-LUX) study. Br J Nutr. (2015) 113:259–69. doi: 10.1017/S0007114514003456
52. Asghari G, Mirmiran P, Hosseni-Esfahani F, Nazeri P, Mehran M, Azizi F. Dietary quality among Tehranian adults in relation to lipid profile: findings from the Tehran Lipid and Glucose Study. J Health Popul Nutr. (2013) 31:37. doi: 10.3329/jhpn.v31i1.14747
53. Kim J, Cho Y, Park Y, Sohn C, Rha M, Lee M-K, et al. Association of dietary quality indices with glycemic status in Korean patients with type 2 diabetes. Clin Nutr Res. (2013) 2:100–6. doi: 10.7762/cnr.2013.2.2.100
54. Lassale C, Gunter MJ, Romaguera D, Peelen LM, Van der Schouw YT, Beulens JW, et al. Diet quality scores and prediction of all-cause, cardiovascular and cancer mortality in a pan-European cohort study. PLoS ONE. (2016) 11:e0159025. doi: 10.1371/journal.pone.0159025
55. Ziaee RS, Keshani P, Salehi M, Ghaem H. Diet quality indices and their correlation with glycemic status and lipid profile in patients with type 2 diabetes. Adv Prev Med. (2021) 2021:2934082. doi: 10.1155/2021/2934082
56. Daneshzad E, Larijani B, Azadbakht L. Diet quality indices and cardiovascular diseases risk factors among diabetic women. J Sci Food Agric. (2019) 99:5926–33. doi: 10.1002/jsfa.9867
57. Kim J, Lee Y, Kye S, Chung Y-S, Kim J-H, Chon D, et al. Diet quality and osteosarcopenic obesity in community-dwelling adults 50 years and older. Maturitas. (2017) 104:73–9. doi: 10.1016/j.maturitas.2017.08.007
58. Gregory CO, McCullough ML, Ramirez-Zea M, Stein AD. Diet scores and cardio-metabolic risk factors among Guatemalan young adults. Br J Nutr. (2008) 101:1805–11. doi: 10.1017/S000711450813584X
59. Asghari G, Mirmiran P, Rashidkhani B, Asghari-Jafarabadi M, Mehran M, Azizi F. The association between diet quality indices and obesity: Tehran Lipid and Glucose Study. Archiv Iran Med. (2012) 15.
60. Fox CS, Golden SH, Anderson C, Bray GA, Burke LE, De Boer IH, et al. Update on prevention of cardiovascular disease in adults with type 2 diabetes mellitus in light of recent evidence: a scientific statement from the American Heart Association and the American Diabetes Association. Circulation. (2015) 132:691–718. doi: 10.1161/CIR.0000000000000230
61. Mann JI. Nutrition recommendations for the treatment and prevention of type 2 diabetes and the metabolic syndrome: an evidenced-based review. Nutr Rev. (2006) 64:422–7. doi: 10.1111/j.1753-4887.2006.tb00227.x
62. Zong G, Li Y, Wanders AJ, Alssema M, Zock PL, Willett WC, et al. Intake of individual saturated fatty acids and risk of coronary heart disease in US men and women: two prospective longitudinal cohort studies. Br Med J. (2016) 355:i5796. doi: 10.1136/bmj.i5796
63. Vickers NJ. Animal communication: when i'm calling you, will you answer too? Curr Biol. (2017) 27:R713–5. doi: 10.1016/j.cub.2017.05.064
64. Ginsberg BH, Brown TJ, Simon I, Spector AA. Effect of the membrane lipid environment on the properties of insulin receptors. Diabetes. (1981) 30:773–80. doi: 10.2337/diab.30.9.773
65. Dali-Youcef N, Mecili M, Ricci R, Andrès E. Metabolic inflammation: connecting obesity and insulin resistance. Ann Med. (2013) 45:242–53. doi: 10.3109/07853890.2012.705015
66. Zhang P, Chen L, Zhao Q, Du X, Bi M, Li Y, et al. Ferroptosis was more initial in cell death caused by iron overload and its underlying mechanism in Parkinson's disease. Free Rad Biol Med. (2020) 152:227–34. doi: 10.1016/j.freeradbiomed.2020.03.015
67. Khodabandehloo H, Gorgani-Firuzjaee S, Panahi G, Meshkani R. Molecular and cellular mechanisms linking inflammation to insulin resistance and β-cell dysfunction. Transl Res. (2016) 167:228–56. doi: 10.1016/j.trsl.2015.08.011
68. Holland WL, Brozinick JT, Wang L-P, Hawkins ED, Sargent KM, Liu Y, et al. Inhibition of ceramide synthesis ameliorates glucocorticoid-, saturated-fat-, and obesity-induced insulin resistance. Cell Metab. (2007) 5:167–79. doi: 10.1016/j.cmet.2007.01.002
69. Lee SFA. Saturated fatty acids, But not unsaturated fatty acids, induce the expression of cyclooxygenase-2 mediated through toll-like receptor 4. J Biol Chem. 276:16683–9. doi: 10.1074/jbc.M011695200
70. Zhu F, Du B, Xu B. Anti-inflammatory effects of phytochemicals from fruits, vegetables, and food legumes: a review. Crit Rev Food Sci Nutr. (2018) 58:1260–70. doi: 10.1080/10408398.2016.1251390
71. Nielsen SJJ, Trak-Fellermeier MA, Joshipura K. The association between dietary fiber intake and CRP levels, US adults, 2007–2010. FASEB J. (2017) 31:648.8-8. doi: 10.1096/fasebj.31.1_supplement.648.8
72. Serafini M, Peluso I. Functional foods for health: the interrelated antioxidant and anti-inflammatory role of fruits, vegetables, herbs, spices and cocoa in humans. Curr Pharm Des. (2016) 22:6701–15. doi: 10.2174/1381612823666161123094235
73. Liu S, Buring JE, Sesso HD, Rimm EB, Willett WC, Manson JE, et al. prospective study of dietary fiber intake and risk of cardiovascular disease among women. J Am Coll Cardiol. (2002) 39:49–56. doi: 10.1016/S0735-1097(01)01695-3
74. Xu X, Zhang J, Zhang Y, Qi H, Wang P. Associations between dietary fiber intake and mortality from all causes, cardiovascular disease and cancer: a prospective study. J Transl Med. (2022) 20:1–8. doi: 10.1186/s12967-022-03558-6
75. Krishnamurthy VMR, Wei G, Baird BC, Murtaugh M, Chonchol MB, Raphael KL, et al. High dietary fiber intake is associated with decreased inflammation and all-cause mortality in patients with chronic kidney disease. Kidney Int. (2012) 81:300–6. doi: 10.1038/ki.2011.355
76. Jiao J, Xu J-Y, Zhang W, Han S, Qin L-Q. Effect of dietary fiber on circulating C-reactive protein in overweight and obese adults: a meta-analysis of randomized controlled trials. Int J Food Sci Nutr. (2015) 66:114–9. doi: 10.3109/09637486.2014.959898
77. Rice-Evans C, Miller N. Antioxidant activities of flavonoids as bioactive components of food. Biochem Soc Trans. (1996) 24:790–5. doi: 10.1042/bst0240790
78. Ha SK, Park H-Y, Eom H, Kim Y, Choi I. Narirutin fraction from citrus peels attenuates LPS-stimulated inflammatory response through inhibition of NF-κB and MAPKs activation. Food Chem Toxicol. (2012) 50:3498–504. doi: 10.1016/j.fct.2012.07.007
79. Comalada M, Ballester I, Bailon E, Sierra S, Xaus J, Gálvez J, et al. Inhibition of pro-inflammatory markers in primary bone marrow-derived mouse macrophages by naturally occurring flavonoids: analysis of the structure–activity relationship. Biochem Pharmacol. (2006) 72:1010–21. doi: 10.1016/j.bcp.2006.07.016
80. Bahadoran Z, Mirmiran P, Azizi F. Dietary polyphenols as potential nutraceuticals in management of diabetes: a review. J Diabet Metabol Disord. (2013) 12:1–9. doi: 10.1186/2251-6581-12-43
81. Iwai K. Antidiabetic and antioxidant effects of polyphenols in brown alga Ecklonia stolonifera in genetically diabetic KK-Ay mice. Plant Foods Hum Nutr. (2008) 63:163–9. doi: 10.1007/s11130-008-0098-4
82. Tadera K, Minami Y, Takamatsu K, Matsuoka T. Inhibition of α-glucosidase and α-amylase by flavonoids. J Nutr Sci Vitaminol. (2006) 52:149–53. doi: 10.3177/jnsv.52.149
83. Kobayashi Y, Suzuki M, Satsu H, Arai S, Hara Y, Suzuki K, et al. Green tea polyphenols inhibit the sodium-dependent glucose transporter of intestinal epithelial cells by a competitive mechanism. J Agric Food Chem. (2000) 48:5618–23. doi: 10.1021/jf0006832
84. Johnston K, Sharp P, Clifford M, Morgan L. Dietary polyphenols decrease glucose uptake by human intestinal Caco-2 cells. FEBS Lett. (2005) 579:1653–7. doi: 10.1016/j.febslet.2004.12.099
85. Johnston KL, Clifford MN, Morgan LM. Coffee acutely modifies gastrointestinal hormone secretion and glucose tolerance in humans: glycemic effects of chlorogenic acid and caffeine. Am J Clin Nutr. (2003) 78:728–33. doi: 10.1093/ajcn/78.4.728
86. Dao T-MA, Waget A, Klopp P, Serino M, Vachoux C, Pechere L, et al. Resveratrol increases glucose induced GLP-1 secretion in mice: a mechanism which contributes to the glycemic control. PLoS ONE. (2011) 6:e20700. doi: 10.1371/journal.pone.0020700
87. Nakazato K, Song H, Waga T. Effects of dietary apple polyphenol on adipose tissues weights in Wistar rats. Exp Anim. (2006) 55:383–9. doi: 10.1538/expanim.55.383
88. Osada K, Suzuki T, Kawakami Y, Senda M, Kasai A, Sami M, et al. Dose-dependent hypocholesterolemic actions of dietary apple polyphenol in rats fed cholesterol. Lipids. (2006) 41:133–9. doi: 10.1007/s11745-006-5081-y
89. Dembinska-Kiec A, Mykkänen O, Kiec-Wilk B, Mykkänen H. Antioxidant phytochemicals against type 2 diabetes. Br J Nutr. (2008) 99:ES109–7. doi: 10.1017/S000711450896579X
Keywords: ABSI, cardiometabolic risk factors, DQI, dietary intake, obesity
Citation: Mohamadi A, Shiraseb F, Mirzababaei A, AkbarySedigh A, Ghorbani M, Clark CCT, Aali Y and Mirzaei K (2023) The association between adherence to diet quality index and cardiometabolic risk factors in overweight and obese women: a cross-sectional study. Front. Public Health 11:1169398. doi: 10.3389/fpubh.2023.1169398
Received: 19 February 2023; Accepted: 22 June 2023;
Published: 13 July 2023.
Edited by:
Basil Nwaneri Okeahialam, University of Jos, NigeriaReviewed by:
Hussam Askar, Al-Azhar University, EgyptMohammad Keykhaei, Johns Hopkins Medicine, United States
Mehdi Sadeghian, Ahvaz Jundishapur University of Medical Sciences, Iran
Copyright © 2023 Mohamadi, Shiraseb, Mirzababaei, AkbarySedigh, Ghorbani, Clark, Aali and Mirzaei. This is an open-access article distributed under the terms of the Creative Commons Attribution License (CC BY). The use, distribution or reproduction in other forums is permitted, provided the original author(s) and the copyright owner(s) are credited and that the original publication in this journal is cited, in accordance with accepted academic practice. No use, distribution or reproduction is permitted which does not comply with these terms.
*Correspondence: Khadijeh Mirzaei, mirzaei_kh@tums.ac.ir; Atieh Mirzababaei, ati_babaee@yahoo.com