- 1Center for Primary Care and Public Health (Unisanté), University of Lausanne, Lausanne, Switzerland
- 2Neuchâtel and Jura Cancer Registry, Neuchâtel, Switzerland
- 3Geneva Cancer Registry, University of Geneva, Geneva, Switzerland
- 4Valais Cancer Registry, Valais Health Observatory, Sion, Switzerland
- 5Department of Occupational Epidemiology, National Research and Safety Institute (INRS), Vandoeuvre lès Nancy, France
Introduction: Occupation can contribute to differences in risk and stage at diagnosis of breast cancer. This study aimed at determining whether occupation, along with skill level and the socio-professional category, affect the breast cancer survival (BCS) up to 10 years after diagnosis.
Materials and methods: We used cancer registry records to identify women diagnosed with primary invasive breast cancer in western Switzerland over the period 1990–2014 and matched them with the Swiss National Cohort. The effect of work-related variables on BCS was assessed using non-parametric and parametric net survival methods.
Results: Study sample included 8,678 women. In the non-parametric analysis, we observed a statistically significant effect of all work-related variables on BCS. Women in elementary occupations, with low skill level, and in paid employment not classified elsewhere, had the lowest BCS, while professionals, those with the highest skill level and belonging to top management and independent profession category had the highest BCS. The parametric analysis confirmed this pattern. Considering elementary occupations as reference, all occupations but Craft and related trades had a hazard ratio (HR) below 1. Among professionals, technicians and associate professionals, and clerks, the protective effect of occupation was statistically significant and remained unchanged after adjustment for age, calendar period, registry, nationality, and histological type. After adjusting for tumor stage, the HRs increased only slightly, though turned non-significant. The same effect was observed in top management and independent professions and supervisors, low level management and skilled laborers, compared to unskilled employees.
Conclusion: These results suggest that work-related factors may affect BCS. Yet, this study was conducted using a limited set of covariates and a relatively small study sample. Therefore, further larger studies are needed for more detailed analyses of at risk occupations and working conditions and assessing the potential interaction between work-related variables and tumor stage.
1. Introduction
1.1. Epidemiology of breast cancer
Breast cancer (BC) is the most frequent cancer in women, representing 25% of malignancies (1). In Switzerland, BC is considered a public health priority; with 31% of new cancer cases, it accounts for the highest number of potential life-years lost before age 70 (2). Since 2003–2007, BC incidence started to decline in most high income countries, including Switzerland (3). Nevertheless, in younger women, an increasing trend was recently reported in Switzerland and some other European countries (4–7).
With screening generalization and the progress made in treatment, the mortality has been constantly declining and survival improving. Regarding the BC prognosis, Switzerland ranks the best among the European countries (8). The age-standardized relative cumulative survival at 10 years after BC diagnosis is currently estimated at 77.5% (9). However, depending on tumor stage at diagnosis, it ranges from 94.5% for Stage I to 9.3% for Stage IV (8).
While tumor stage and age at diagnosis are two major prognostics factors, other factors including tumor characteristics, treatment, comorbidities, and socioeconomic status have been suggested to impact the BC survival (8, 10). Yet, neither socioeconomic differences in stage at diagnosis nor other sociodemographic factors such as age, nationality and marital status could explain the survival inequalities observed in Switzerland (11). In contrast, age beyond of the recommended screening age, unmarried marital status, low socioeconomic status, and residence in a canton without organized BC screening program were associated with an increased risk of being diagnosed with a later-stage BC (11). Moreover, after controlling for calendar time, canton, age, marital status and nationality, BC stage at diagnosis was found gradually associated with the socio-professional category and skill level required for the occupation. This gradient was also observed for occupation and occupational activity sector, though to a lesser extent (12).
1.2. Occupation and breast cancer
Occupation is a key variable in occupational health since work is an important health determinant (13, 14). Occupation enables estimating or approximating the working conditions and exposures to carcinogens in the workplace and analyzing their effect on incidence and mortality from specific causes of death, including cancer (15). Occupation influences both incidence and mortality of BC (12). As a result, several occupations have been identified at risk of BC, namely occupations in dry cleaning, hairdressing, metalworking, aircraft maintenance, textile, leather and fur processing, electronics manufacturing, and military, as well as dentists, physicians, journalists, administrators, flight attendants and artistic workers (12, 16–19).
The fraction of BC attributable to occupational exposures to ionizing radiation, shift work and cytostatic drugs was estimated at 9.8% as a central core estimate and 18.5% as a midpoint estimate (20). Yet, women can be exposed to many other risk factors in the workplace (21), namely the circadian rhythm disruptors through night shift work (22–24), electromagnetic fields (25), and carcinogenic metals and chemicals (19, 26). Compared with occupational exposures, the first established risk factors of BC (i.e., family BC history and reproductive, hormonal, and morphological characteristics) account for less than 30% of incident cases (27, 28). A high fasting glucose, high BMI, and diet high in red meat were identified as having the highest estimated fractions of BC deaths and DALYs attributable to metabolic risk factors (6.1%, 4.7%, and 3.2% of DALYs, respectively) (29). Among behavioral risk factors, alcohol consumption ranks the first (5.2% of DALYs), followed by second hand smoke exposure and smoking (2.7% and 2.5%, respectively), and by low physical activities (1.0% of DALYs) (29). Occupation thus plays a critical role in the onset of BC, contributing directly or indirectly to the development and progression of BC (19, 30).
Occupation and work-related factors can impact the woman’s life-course at virtually all steps of her BC journey (Figure 1). For instance, depending on occupation, flexible working hours, supportive supervisors and availability of occupational health programs and services could facilitate employee’s participation in BC screening and an early BC diagnosis, or conversely, hamper it. In our previous studies, we found an association between occupation and tumor stage at diagnosis for lung and BC (12, 15).
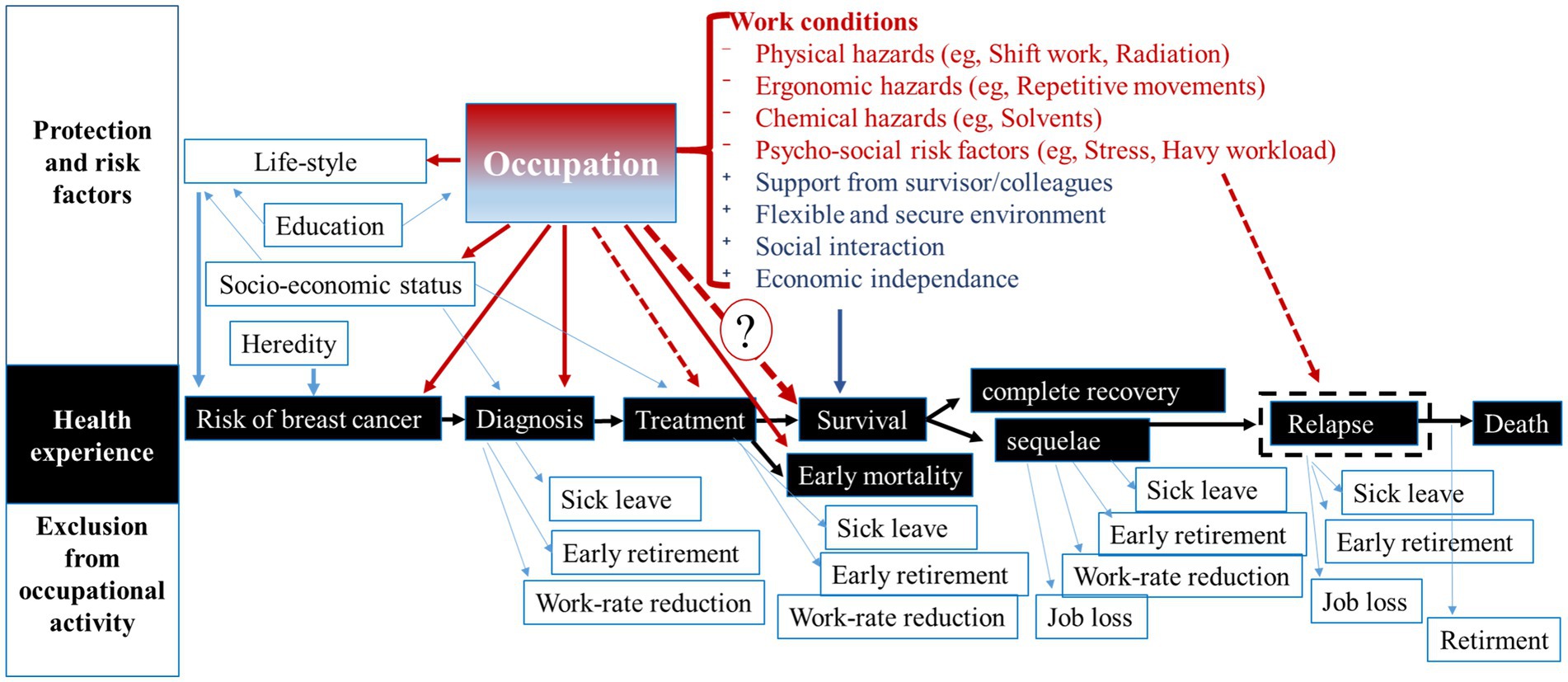
Figure 1. Relationships between occupation and breast cancer experience for a working woman. Dot-lines correspond to the hypothesized relationships, which have not been studied yet. Question mark indicates the relationship investigated in this study. Text in red corresponds to examples of breast cancer risk factors, text in blue corresponds to examples of protective factors.
Similarly, BC treatment can be more or less compatible with usual working conditions and influence the treatment efficacy. As some antineoplastic drugs come with side-effects such as ototoxicity, neurotoxicity and nephrotoxicity (31–33), the exposure to noise, solvents, and some metals in the workplace can worsen the treatment tolerance or make it incompatible with work. Exposure to artificial light during night changes the expression of circadian genes acting as tumor suppressors (34, 35). Altered expression of these genes is related with atypical cell proliferation, DNA repair impairment and apoptosis. It also increases human cancer cells’ drug resistance (22, 36). A suboptimal or ineffective treatment is related to a poorer survival and occupation can modify this relationship. Although research has still neglected this topic, an excessive mortality from BC in some occupations supports this hypothesis.
Regarding the relationship between occupation and survival, the evidence comes from studies on return to work (RTW) after BC diagnosis and treatment (37–41). This research shows that for many women, RTW is a “symbol of recovery,” raising their self-esteem and helping overcome the treatment side effects (42–44). Compared with other cancers, BC survivors have the greatest chance of RTW (41). Consequently, work ability, social reintegration and (re-)employment are a highly relevant concern for BC survivors. Most of them (85%) are motivated either to return to work or be re-employed after rehabilitation (45, 46). However, many BC survivors experience a significantly increased risk for unemployment and early retirement (47–49), as shown in Figure 1.
The prevalence of RTW after a treatment–related sick-leave depends on national legislation (45, 50), yet in liberal countries like Switzerland or South Korea, it can be company and occupation dependent. Availability of the rehabilitation and support systems both in hospitals and workplace enabling to “work around the treatment schedule” (40) or gradually resuming work in accordance with their health condition are facilitators of RTW. Indeed, ongoing physical and/or cognitive limitations, such as persisting fatigue, treatment-induced menopausal symptoms, difficulties with lifting, coping issues, anxiety, and depression -common in BC survivors- can limit their workability and require a work rate adjustment (37, 51–54). While reduction of working hours is associated with financial difficulties, these difficulties are more important among those who had to quit their job after RTW (55–57).
The probability of RTW is strongly related with younger age at diagnosis, less advanced stage of BC, higher education, non-manual work, or being self-employed. However, in some countries, self-employed workers, especially those on short-term contracts or employed by very small companies (with less than 5 employees) are less protected by employment lows and have limited right to paid sick-leave. Workplace policies, procedures, culture, but also workload emerged as major factors impacting RTW experience in many studies (37, 39, 43, 58). Thus, cancer survivors able to return to work usually have unstable employment trajectories than other workers (45, 59). As a result, occupation-related and financial factors that could vary while the disease can damage their quality of life and, consequently, their survival (53, 60).
1.3. Research question and hypotheses
Occupation has been frequently and relevantly used as a proxy of occupational exposures, either directly or in linkage with job-exposure matrices (61). In line with a life-course exposure model “Exposome” (62), the “Worksome” has been proposed for the “explicit consideration of both physical and psychosocial exposures and effects derived from work and working conditions” (63). The authors of the worksome framework concluded that the relationship between health and work should be examined with classifications specific to occupation or industry instead of socio-economic class classifications (63). Therefore, in this study, we aimed to assess the relationship between occupation and BC survival among women with known occupation living in Western-Switzerland. As BC incidence and stage at diagnosis was found related to the skill level required for occupation and the socio-professional category we hypothesized that these variables can be also associated with BC survival.
2. Materials and methods
In this study we applied the methodology developed for our previous study dedicated to the lung cancer survival (15). To help the reader understand it, we summarized below the most important points.
2.1. Study sample, predictors, and outcome definition
Data from the cancer registries of Western Switzerland (cantons of Geneva, Neuchâtel, Vaud, and Wallis) for the period 1990–2014 were used as source data. These data were matched with the Swiss National Cohort (SNC) to retrieve information on occupation and mortality. The SNC is based on data from the 1990 and 2000 federal censuses, which were linked to mortality, birth, and emigration records (64). The SNC has an estimated population coverage of 98.6% (65).
The study sample included female breast cancer cases aged between 18 and 65 years at the time of either of the census with a known occupation. Participants were followed from the date of breast cancer diagnosis until the earliest of the following events: date of emigration, 85th birthday, death, or study termination (December 31, 2014).
Three different work-related variables were analyzed as independent predictors. The first was the participant’s occupation, which was collected twice (in 1990 and 2000) and coded according to the International Standard Classification of Occupations, 1988 version (ISCO-88) established by the International Labour Organization (ILO). In this study, we used the first digit of the ISCO-88 code, which identifies nine major occupational groups such as clerks or technicians. The skill level required for the occupation was the second predictor variable. This variable was also established by the ILO (66). Finally, the socio-professional category was the third work-related variable considered. It was defined by the Swiss Federal Statistical Office (SFSO) as a composite of occupation, the highest level of education completed, occupational status, and legal form of business (67). As start and end dates of employment were not known, we assigned the 1990 census occupational information for participants diagnosed with BC between 1990 and 2000, and the 2000 information thereafter.
The study outcome was incident primary malignant BC, coded C50 based on the International Classification of Diseases for Oncology (ICD-O), 3rd edition. BC cases were selected according to the International Agency for Research on Cancer (IARC) rules for multiple primary cancers (68). Cases were grouped according to the histological types into the following categories: ductal carcinoma (8,500, 8,522 and 8,523), lobular carcinoma (8,520 and 8,524) and other, excluding sarcomas (8,800) and lymphomas (9,590) localized in the breast.
Cancer registries coded tumor stage at diagnosis according to the classification of malignant tumors (TNM) (69). Tumors localized to the organ of origin constituted stages I and II, locally extensive spread, particularly to regional lymph nodes, stage III, and tumors with distant metastasis, stage IV. When missing, stage at diagnosis was imputed, using multivariate imputation by chained equations (70). Nelson−Aalen cumulative hazard, cancer registry, language region, age at diagnosis, marital, status skill level required for the occupation, and socio-professional category were used as stage predictors. All models were run with 25 imputations, in order to reduce the impact of the random sampling inherent in multiple imputation procedures (71). Stages III and IV were grouped in one category, since it allowed a better match when comparing the proportions of each stage between the observed and imputed data.
2.2. Statistical analyses
Net survival can be used to estimate the survival that would be observed if the only possible underlying cause of death was the disease under study (72). Prior findings showed that the relative survival approach was more robust than the cause-specific one and more suitable for net survival analysis (73). In the relative survival setting, the cause of death of participants who die during follow-up is ignored; net survival is estimated using life tables and can be defined as the ratio of the observed survival to the one expected from the life tables (74). In other words, it approximates the net survival probability and can be seen as the survival probability from the disease under study after all other risk have been removed.
In this study, we applied two methods of net survival analysis. First, the Pohar-Perme non-parametric method (75) was used along with the log-rank type test (76) to compare the net survival curves between groups. Secondly, for every predictor variable, we applied a parametric method that models the excess hazard in a framework of multivariable proportional hazard regression model (77).
For the non-parametric approach, we used the STNS package (78) developed in STATA. It requires the all-cause mortality rate table, which is used to compute the expected hazard and survival of each subject at each event time in the dataset. The latter was calculated using the mortality rates of the female population of the cantons of Geneva, Neuchâtel, Vaud, and Wallis stratified by 5-year age group (18–85 years) and 5-year calendar period (1990–2014). These categories were chosen to smooth the rates and avoid large differences in mortality by age or calendar year. Because we were mainly interested in the survival by occupation, we also stratified our rates by ISCO-88 1-digit code (79). Net breast cancer survival was computed at 5 years for occupation (ISCO-88 1-digit code), skill level and socio-professional category. We applied a log-rank test to compare the net survival curves between groups.
For the parametric approach, we used the flexrsurv R package (80). We fitted excess hazard model with a cubic spline with 3 knots (1, 5, and 10 years of follow-up) as baseline hazard. Background mortality rates were the same as in the non-parametric survival analysis. Again, we calculated the excess hazards by occupation (ISCO-88 1-digit), skill level and socio-professional category. For each of these variables, we fitted three models. The Model 1 was adjusted for age, calendar period at diagnosis and cancer registry. The Model 2 was furthermore adjusted for nationality and for histological type of tumor, to control for the potential confounding of nationality and smoking. Finally, the Model 3 was additionally adjusted for the tumor stage at diagnosis. We also tested the non-proportional effect of stage using B-Splines (81). In order to compare the fit of our models, we used the Akaike Information Criterion (AIC) (82).
3. Results
3.1. Cohort description
Of the 20,113 female BC cases diagnosed between 1990 and 2014, we excluded 10,963 cases (55.51%) because of a lack of information on their occupations. Unemployed and job-seeking women (472 cases), who represented 2.34% of the total, were also excluded. The study sample consisted of 8,678 participants (Table 1). Most participants (81%) were Swiss. Sixty-one percent were married, while 19% were single. The mean age at diagnosis was 54.5 ± 9.3 years and the mean duration of follow-up was 9.2 ± 6.3 years. The most represented occupational group was clerks (26%), followed by technicians and associate professionals (24%). About half of the study sample had occupations requiring the second lowest level of skills. In addition, participants were more likely to be in the supervisors/low-level management and skilled labor socio-professional category. Participants in top management and independent occupations accounted for only 3% of study sample.
The tumor stage at diagnosis was known for 79% of cases (Table 2). Participants were more likely to be diagnosed at stage I or II (38 and 31% of cases respectively). Ductal carcinoma was the most common histological type of BC (Table 2).
3.2. Ten-year net survival per occupation
In the nonparametric setting, we observed a strong statistically significant difference in BC survival across occupational groups (p < 0.001). Overall, Professionals had the highest survival and Elementary occupations and Craft and related trades workers had the lowest survival, and, with a 10% lower net survival at 10 years compared to Professionals (Figure 2A).
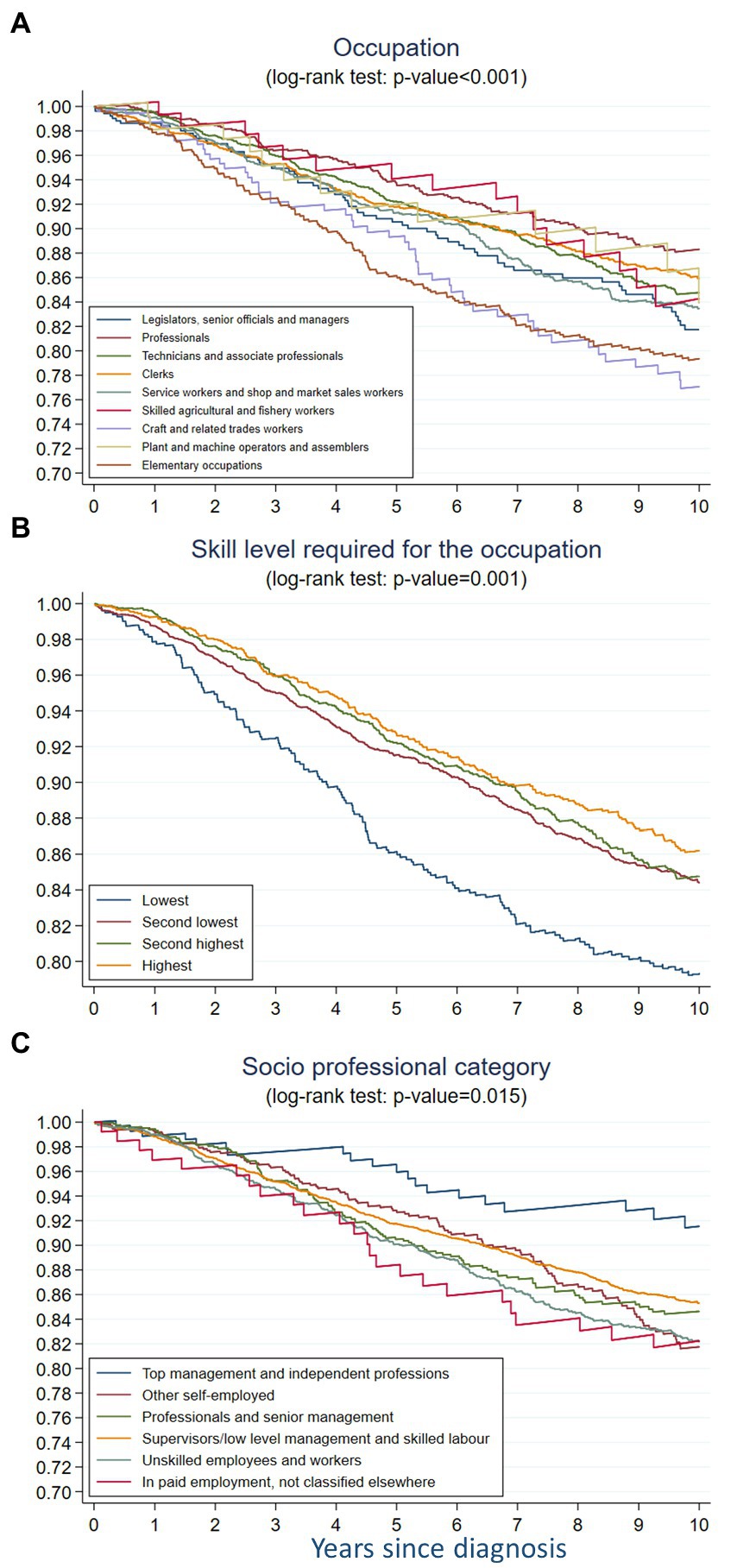
Figure 2. Non-parametric 10-year net survival of breast cancer across (A) occupation, (B) skill level required for the occupation, and (C) socio-professional category among women aged 18–85 in French-speaking Switzerland (1990–2014).
In the parametric analysis, taking elementary occupations as the reference, Craft and related workers had the highest HR in the three models, with 11% higher risk of mortality in the 10 years after BC diagnosis in models 1 and 2 and 23%-risk increase in the model 3 (Table 3). The HR for Plant and machine operators and assemblers was also above 1 but in the Model 3 only. All the other occupational groups had the HR below 1 in the three models, compared to the elementary occupations although the HR were higher in model 3 than in models 1 and 2.
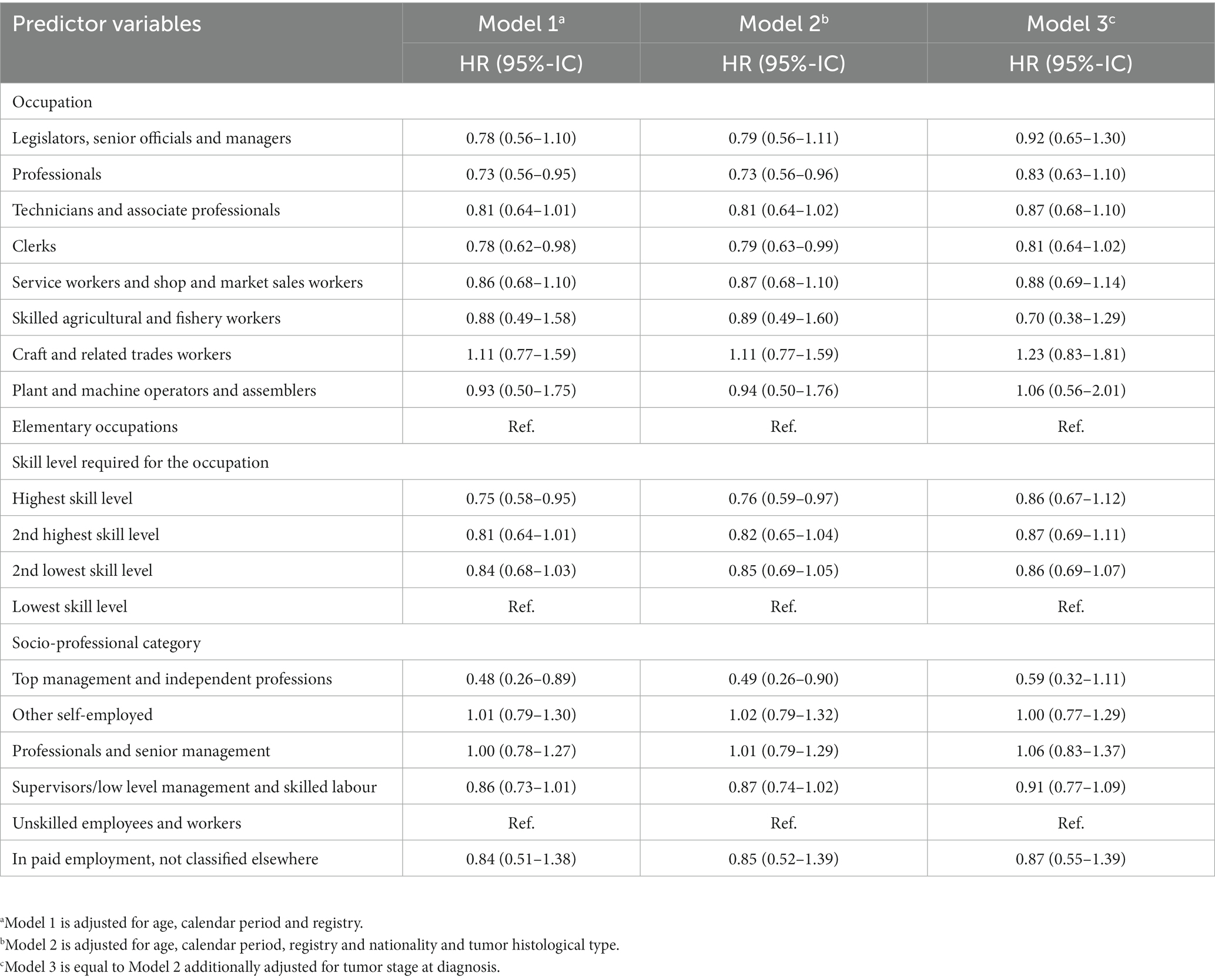
Table 3. Hazard ratios (HR) and Confidence Interval (95%-IC) for breast cancer relative survival by work-related variables among women aged 18–85 in French-speaking Switzerland (1990–2014).
3.3. Ten-year net survival per skill level
The nonparametric analysis showed a statistically significant difference in BC survival across skill levels required for occupation (p < 0.001) (Figure 2B). The survival decreased gradually from that in women with the highest skill level to that in the lowest skill level.
The parametric analysis confirmed this gradient in both Model 1 and Model 2 (Table 3). Having a high skill level had a significant protective effect in both models, but decreased after adjustment for the tumor stage at diagnosis (Model 3). Noteworthy, for women with an intermediate high and an intermediate low skill level, this adjustment induced a very small change.
3.4. Ten-year net survival per socio-professional category
In the non-parametric analysis, the BC survival differed across socio-professional categories (p = 0.015) (Figure 2C). Women belonging to the top management and independent professions category had the best survival, while women in paid employment not classified elsewhere had the worse one. The second worse BC survival was observed among unskilled employees and workers. The gradient was less obvious then for the skill level variable and the differences between categories were more difficult to disentangle. During the first 2 years of follow-up, the survival among the women in top management and independent professions, those in other self-employed occupations and those working as professionals and senior managers was quite close, then diverged between these three categories and strongly decreased among the latter category. After 3 years of follow-up, the survival of professionals and senior managers became lower than the survival of supervisors/low level managers and skilled laborers, though this observation is based on a small number of cases.
The parametric analysis showed that compared to unskilled employees, women working in top management and independent professions had a twice-lower net rate within 10 years after BC diagnosis without adjusting for the tumor stage (Table 3) and a 40%-lower but statistically non-significant risk after accounting for tumor stage.
4. Discussion
4.1. Summary of the main results
In this study, we assessed the relationship between the 10-year net survival for breast cancer and occupation, considering three complementary work-related variables: occupation, skill level required for the occupation, and the socio-professional category. Moreover, we applied an original analytical approach including both parametric and non-parametric analyses. To our knowledge, this study is the first to apply such a methodology to investigate the potential work-related determinants of BC survival.
In the non-parametric analysis, we found that the net survival varied across occupations, skill levels, and socio-professional category. Women in elementary occupations, with low skill level, and in paid employment not classified elsewhere, had the lowest BC survival, while professionals, those with the highest skill level and belonging to top management and independent profession category had the highest BC survival. The parametric analysis confirmed this pattern. Considering elementary occupations as reference, all occupations but Craft and related trades had a hazard ratio below 1. Among professionals, technicians and associate professionals, and clerks, the protective effect of occupation (adjusted for age, calendar period and registry) was statistically significant and remained unchanged after further adjusting for nationality and histological type. After adjusting for tumor stage, the hazard ratios remained below 1, though turned statistically non-significant. The same effect was observed in top management and independent professions and in supervisors/low level management and skilled laborers, compared to unskilled workers.
4.2. Results interpretation
The results observed in the analysis of the relationship between occupation and skill level are consistent and in line with our research hypothesis. Although the skill-level variable is constructed based on ISCO, it offers a complementary information, helpful for the result interpretation. The skill level was shown as a risk factor in BC incidence, with the highest incidence associated with the highest skill level (12). Such a trend has been also reported for the education (83, 84). However, a recent study using casual mediation analysis concluded that a low educational level is a causal risk factor in the BC development “as it is associated with poor lipid profile, obesity, smoking, and types of physical activity” (85). The protective effect of education with respect to BC survival is also questionable, given the discrepancy between studies (83, 84, 86) and a weak effect-size in the meta-analysis (87).
We observed an increasing survival per increasing skill level required for the occupation, suggesting its protective effect with respect to BC survival. This effect might operate via the access to a better (i.e., non-manual, intellectually stimulating and providing a better income) occupation. In such occupations, women have no or low exposure to occupational BC risk factors and benefit from early BC diagnosis and therefore from a less invasive and more effective treatment and return to work. The former was confirmed in our pervious study (12).
The parametric analysis showed clearly that non-manual occupations have a better survival (HR < 0.85), with technicians and associate professionals at a cross-section between manual and non-manual occupations. Professionals and clerks had the lowest HRs in all models, compared to women working in elementary occupations, used as reference. It worth mentioning that in the previous analysis, elementary occupations had a significantly reduced risk of BC, but a higher proportion of advanced (III or IV) and missing tumor stage at diagnosis (12). In Switzerland, elementary occupations have the highest proportion (>60%) of part-time workers, and women represent three fourths of them (88). Part-time work could be either protective or a risk factor for survival depending on whether it is voluntary or involuntary, for example when it is not possible to return to full-time work (59). Nevertheless, the evidence is still very scarce. Some women with low skill levels belong to this occupational group, and the result could be explained, at least partially, by financial constrains (60, 89).
The results of analysis according to the socio-professional category are less easily interpretable. On the one hand, the protective effect of the top management and independent professions in both parametric and non-parametric analysis appears a consistent finding overall. On the other hand, low level management corresponded to a better survival and hazard ratio than the reference category (unskilled workers). Furthermore, professional and senior management and other self-employment exhibited a hazard ratios similar to the reference category and higher than the low level management. However, net survival during the follow-up showed different patterns between these groups which may reveal non proportional effect of occupation. The classification of socio-professional categories used in this study accounts for all levels of the population’s socio-professional structure (90). Therefore, the risk of misclassification can reasonably be ruled out. A tentative explanation of this finding could thus lie in a set of conditions associated with the above-mentioned socio-professional categories, especially when considered the temporal change in survival in the non-parametric analysis. This could be the BC treatment-related side effects, and particularly, their impact on mental health and cognitive functions (52, 91–96). In fact, the return to work in these socio-professional groups can be easier than elsewhere, but long-term or chronic functional cognitive deficiencies can impact their work performance and participation (52). Cognitive difficulties affect approximatively 30% of BC survivors (97, 98) and can be more troublesome than physical side effects (52, 99).
4.3. Methodological aspects
Despite an intensive effort to complete and confirm this data using cancer registry records, the tumor stage at diagnosis was missing for 21% of women in our study sample. To properly manage missing values for this important variable, we used multiple imputations. The complete case analyses provided estimated hazard ratios with wider confidence intervals (Supplementary Table S1). This suggests that the imputation permitted to increase the precision in study results.
Regarding the occupation variable, the 2-digit ISCO variable showed the best predictive accuracy for all work-related health measures (63). However, the AIC was smaller in the model with the occupation variable coded using one-digit ISCO-88 than the model with occupation coded using two-digit ISCO-88 codes, suggesting that the former fitted the data better than the latter. Therefore, we used the occupation variable coded using one-digit ISCO-88 in our analyses. We recognize that occupational groups at this large level of aggregation (1 or 2 digits) might be insufficiently specific to incorporate factors directly related to occupational exposures and working conditions. Information on working conditions before and after diagnosis along with information on RTW after cancer treatment were not available but are important to collect and analyze with respect to cancer survival in the future. RTW reduces economic hardship, maintains mental well-being, facilitates physical recovery, and may improve BC survival (47, 100). Consequently, we believe that analyses at a finer level of ISCO-88 (3 or 4 digits), combined with information on the duration of sick leave, RTW, and working conditions and exposures could improve the understanding of net BC survival.
4.4. Strengths and limitations
The use of the international classification of occupation and skill level required for the occupation is a major strength of this study. Standardized classifications enable a common and replicable definition and measurement of these work-related variables. A high completeness of case ascertainment in western Swiss cancer registries during the study period along with the incidence information of a very good quality (101) is another strength of this study. By focusing on female BC we addressed the recommendation of the European Union information agency for occupational safety and health to investigate female workers’ health more specifically (102).
From the methodological point of view, this study has also brought several advantages. The application of the non-parametric method enabled us calculating the BC net survival for each of the three occupational variables without making any specific assumption. Furthermore, the application of the parametric method enabled us quantifying the differences between groups in terms of hazard ratios and test the proportional hazards assumption for the tumor stage at diagnosis.
The use of a relative survival framework in our study was appropriate to investigate inequalities in BC survival. This permitted accounting for disparities in mortality between study groups with respect to multiple causes of death (15). This was done using life table that we stratified by occupation. As a result, the socio-economic conditions were more homogenous within each strata. This is important to emphasize because socio-economic conditions are expected to influence general mortality (79). This enabled us limiting biases in the estimate of the effect of this variable but also, to a lesser extent, in the estimates of the effects of the other covariates included in the model (103). Because of the association between mortality and occupational variables, we believe that future studies on occupational factors should also focus on relative survival methods using life table stratified by occupation.
Regarding limitations, occupation was missing for 55% of women in the SNC, and the study sample was half smaller after exclusion of these cases. A comparison of participants with and without occupational information showed that they had similar net survival characteristics (Supplementary Table S2). However, we could not fully rule out a selection bias since no comparison of the distribution of work-related variables for patients with and without information on occupation was possible.
Assigning occupations as a time-dependent variable based on two time points could result in some misclassification, especially given that cancer survivors have a less stable employment trajectory than other workers (59). Nevertheless, the information on the occupation at the time of the federal censuses was correct and we believe that it was assigned accurately, as the majority of patients kept the same occupation between the two censuses (104, 105). Having occupational information at the time of diagnosis would be better, but it was not available.
The quality of occupational data in all western Swiss cancer registries and in the SNC were assessed in a prior study and varied across registries (106). Thus, to avoid differential misclassification of occupations, we decided to use SNC data rather than registry data. Moreover, we were constrained to aggregating the occupation under one-digit codes, reducing the variability of occupational situations, due to the limited number of observations per occupational group. This can explain a likely lack of statistical power in parametric analysis and non-statically significant hazard ratios.
Finally, information on smoking, duration of sick leave, and working conditions after RTW, particularly the night shift work, was not available to study their effect on BC survival. Information on BC treatment was also unavailable. Yet, since the implementation of the Swiss federal law on cancer registration in 2020, all Swiss cancer registries should collect this information systematically (107). The use of these data in future large, nationwide, or international studies will allow a more accurate estimation of factors affecting net BC survival. Such studies are important to informing health and social protection systems, which should guarantee appropriate work conditions for cancer survivors and educate them on their rights and obligations during sick leave (108). It is essential that clinicians and institutions consider work-related issues in BC patients and perform adequate organizational and normative interventions, particularly in the most vulnerable occupational groups (38).
5. Conclusion
This study reports the net survival for breast cancer across three complementary occupation-related variables: occupation, skill level required for the occupation, and the socio-professional category of employment. We found that the net BC survival depended on these variables. The lowest skill level was associated with the worst survival prospects, while working in non-manual occupations in general, and in top management and independent professions particularly was related to a better survival. In the parametric models, the adjustment for the histological type of BC and tumor stage at diagnosis allowed us to control for the effect of these variables and indirectly control for smoking. After this adjustment, the hazard ratio estimates are closer to unity with few impacts on their order suggesting a limited impact of the inequality in diagnosis on the net survival.
As this study was conducted using a limited set of covariates and a relatively small sample, further studies are required, taking into account smoking habits and treatments administrated to the BC patients. Information on RTW and working conditions before and after BC diagnosis will also be highly valuable to analyzing their effect on BC net survival in large nationwide or international studies. Such studies are essential to informing health and social protection systems, which should guarantee appropriate work conditions for BC survivors, beneficial for their quality of life and survival.
Data availability statement
The datasets presented in this article are not readily available because The data were generated under the mandate of the Swiss federal statistical office cannot be used for any other study. Requests to access the datasets should be directed to aXJpbmEuZ3VzZXZhLWNhbnVAdW5pc2FudGUuY2g=.
Ethics statement
The studies involving human participants were reviewed and approved by Cantonal Ethics Committees of Bern and Zurich and by the Commission cantonale d’éthique de la recherche sur l’être humain (CER-VD) (Project-ID 2018–02077). Written informed consent for participation was not required for this study in accordance with the national legislation and the institutional requirements.
Author contributions
NB, MG, and IC: conceptualization. NB: data curation. IC: funding acquisition. PA, J-LB, EF, SG, IK, MM, and ER: resources. NB, MG, and IC: validation. NB and IC: writing—original draft. NB, MG, PA, J-LB, EF, SG, IK, MM, ER, and IC: writing—review and editing. All authors contributed to the article and approved the submitted version.
Funding
This research was funded by the Swiss Cancer Research Foundation and the Swiss Cancer League, grant number No KFS-4699-02-2019. Open access funding provided by the University of Lausanne.
Acknowledgments
We thank the Swiss federal statistical office for providing mortality and census data, and for the support that made the Swiss National Cohort and this study possible. The members of the Swiss National Cohort Study Group are Matthias Egger (Chairman of the Executive Board), Adrian Spoerri and Marcel Zwahlen (all Bern), Milo Puhan (Chairman of the Scientific Board), Matthias Bopp (both Zurich), Martin Röösli (Basel), Michel Oris (Geneva) and Murielle Bochud (Lausanne). We also thank all the Western cancer registries for providing their data and for their support.
Conflict of interest
The authors declare that the research was conducted in the absence of any commercial or financial relationships that could be construed as a potential conflict of interest.
Publisher’s note
All claims expressed in this article are solely those of the authors and do not necessarily represent those of their affiliated organizations, or those of the publisher, the editors and the reviewers. Any product that may be evaluated in this article, or claim that may be made by its manufacturer, is not guaranteed or endorsed by the publisher.
Supplementary material
The Supplementary material for this article can be found online at: https://www.frontiersin.org/articles/10.3389/fpubh.2023.1129708/full#supplementary-material
References
1. Sung, H, Ferlay, J, Siegel, RL, Laversanne, M, Soerjomataram, I, Jemal, A, et al. Global cancer statistics 2020: GLOBOCAN estimates of incidence and mortality worldwide for 36 cancers in 185 countries. CA Cancer J Clin. (2021) 71:209–49. doi: 10.3322/caac.21660
2. OFS, NICER, & CCR. Le Cancer en Suisse, Rapport2021—Etat des Lieux et Évolutions. Neuchatel, Switzerland: Office fédéral de la statistique. (2021).
3. Bouchardy, C, Pury, P, Lorez, M, Clough-Gorr, K, and Bordoni, A, Group., N. W. Trends in breast cancer survival in Switzerland. Swiss Cancer Bull. (2011) 31:326–8.
4. Bodmer, A, Feller, A, Bordoni, A, Bouchardy, C, Dehler, S, Ess, S, et al. Breast cancer in younger women in Switzerland 1996–2009: a longitudinal population-based study. Breast. (2015) 24:112–7. doi: 10.1016/j.breast.2014.11.004
5. Leclère, B, Molinié, F, Trétarre, B, Stracci, F, Daubisse-Marliac, L, and Colonna, M. Trends in incidence of breast cancer among women under 40 in seven European countries: a GRELL cooperative study. Cancer Epidemiol. (2013) 37:544–9. doi: 10.1016/j.canep.2013.05.001
6. Merlo, DF, Ceppi, M, Filiberti, R, Bocchini, V, Znaor, A, Gamulin, M, et al. Breast cancer incidence trends in European women aged 20-39 years at diagnosis. Breast Cancer Res Treat. (2012) 134:363–70. doi: 10.1007/s10549-012-2031-7
7. Wanner, M, Matthes, KL, Karavasiloglou, N, Limam, M, Korol, D, and Rohrmann, S. 37-year incidence and mortality time trends of common cancer types by sex, age, and stage in the canton of Zurich. Swiss Med Wkly. (2020) 150:w20388. doi: 10.4414/smw.2020.20388
8. Bouchardy, C, Lorez, M, and Arndt, V, Group., N. W. Effects of age and stage on breast cancer survival in Switzerland. Swiss Cancer Bull. (2015) 35:152–7.
9. NICER. (2020). Swiss survival statistics 2012–2016. Avaialble at: https://www.nicer.org/en/statistics-atlas/cancer-survival/
10. Taban, F, Elia, N, Rapiti, E, Rageth, C, Fioretta, G, Benhamou, S, et al. Impact of experience in breast cancer surgery on survival: the role of quality of care in a registry-based cohort. Swiss Med Wkly. (2019) 149:w14704. doi: 10.4414/smw.2019.14704
11. Feller, A, Schmidlin, K, Bordoni, A, Bouchardy, C, Bulliard, JL, Camey, B, et al. Socioeconomic and demographic disparities in breast cancer stage at presentation and survival: a Swiss population-based study. Int J Cancer. (2017) 141:1529–39. doi: 10.1002/ijc.30856
12. Bulliard, JL, Bovio, N, Arveux, P, Bergeron, Y, Chiolero, A, Fournier, E, et al. Occupational factors and socioeconomic differences in breast cancer risk and stage at diagnosis in Swiss working women. Cancers (Basel). (2022) 14:3713. doi: 10.3390/cancers14153713
13. Graczyk, H, François, M, Krief, P, and Guseva Canu, I. The role of the Swiss list of occupational diseases in the protection of workers' health. Swiss Med Wkly. (2021) 151:w20538. doi: 10.4414/smw.2021.20538
14. Guseva Canu, I, François, M, Graczyk, H, and Vernez, D. Healthy worker, healthy citizen: the place of occupational health within public health research in Switzerland. Int J Public Health. (2020) 65:111–20. doi: 10.1007/s00038-019-01245-w
15. Bovio, N, Grzebyk, M, Arveux, P, Bulliard, JL, Chiolero, A, Fournier, E, et al. Work-related factors and lung cancer survival: a population-based study in Switzerland (1990–2014). Int J Environ Res Public Health. (2022) 19:13856. doi: 10.3390/ijerph192113856
16. Petralia, SA, Vena, JE, Freudenheim, JL, Marshall, JR, Michalek, A, Brasure, J, et al. Breast cancer risk and lifetime occupational history: employment in professional and managerial occupations. Occup Environ Med. (1998) 55:43–8. doi: 10.1136/oem.55.1.43
17. Pukkala, E, Martinsen, JI, Lynge, E, Gunnarsdottir, HK, Sparén, P, Tryggvadottir, L, et al. Occupation and cancer – follow-up of 15 million people in five Nordic countries. Acta Oncol. (2009) 48:646–790. doi: 10.1080/02841860902913546
18. Weinmann, S, Tanaka, LF, Schauberger, G, Osmani, V, and Klug, SJ. Breast cancer among female flight attendants and the role of the occupational exposures: a systematic review and meta-analysis. J Occup Environ Med. (2022) 64:822–30. doi: 10.1097/JOM.0000000000002606
19. Xiao, W, Huang, J, Wang, J, Chen, Y, Hu, N, and Cao, S. Occupational exposure to organic solvents and breast cancer risk: a systematic review and meta-analysis. Environ Sci Pollut Res. (2022) 29:1605–18. doi: 10.1007/s11356-021-17100-6
20. Vencovsky, D, Postle, D, Kalberlah, F, Vencovska, J, Fenn, T, Daly, E, et al. The cost of occupational cancer in the EU-28. Brussels, Belgium: European Trade Union Institute (ETUI). (2017).
21. Brito-Marcelino, A, Duarte-Tavares, RJ, Marcelino, KB, and Silva-Neto, JA. Breast cancer and occupational exposures: an integrative review of the literature. Rev Bras Med Trab. (2021) 18:488–96. doi: 10.47626/1679-4435-2020-595
22. Briguglio, G, Costa, C, Teodoro, M, Giambò, F, Italia, S, and Fenga, C. Women's health and night shift work: potential targets for future strategies in breast cancer (review). Biomed Rep. (2021) 15:98. doi: 10.3892/br.2021.1474
23. Cordina-Duverger, E, Menegaux, F, Popa, A, Rabstein, S, Harth, V, Pesch, B, et al. Night shift work and breast cancer: a pooled analysis of population-based case-control studies with complete work history. Eur J Epidemiol. (2018) 33:369–79. doi: 10.1007/s10654-018-0368-x
24. Manouchehri, E, Taghipour, A, Ghavami, V, Ebadi, A, Homaei, F, and Latifnejad Roudsari, R. Night-shift work duration and breast cancer risk: an updated systematic review and meta-analysis. BMC Womens Health. (2021) 21:89. doi: 10.1186/s12905-021-01233-4
25. Shih, Y-W, O'Brien, AP, Hung, C-S, Chen, K-H, Hou, W-H, and Tsai, H-T. Exposure to radiofrequency radiation increases the risk of breast cancer: a systematic review and meta-analysis retraction in /10.3892/etm.2021.9903. Exp Ther Med. (2021) 21:23. doi: 10.3892/etm.2020.9455
26. Leso, V, Ercolano, ML, Cioffi, DL, and Iavicoli, I. Occupational chemical exposure and breast cancer risk according to hormone receptor status: a systematic review. Cancers. (2019) 11:1882. doi: 10.3390/cancers11121882
27. Cantor, KP, Stewart, PA, Brinton, LA, and Dosemeci, M. Occupational exposures and female breast cancer mortality in the United States. J Occup Environ Med. (1995) 37:336–48. doi: 10.1097/00043764-199503000-00011
28. Harris, JR, Lippman, ME, Veronesi, U, and Willett, W. Breast cancer. N Engl J Med. (1992) 327:319–28. doi: 10.1056/NEJM199207303270505
29. GBD. The global burden of cancer attributable to risk factors, 2010-19: a systematic analysis for the global burden of disease study 2019. Lancet. (2022) 400:563–91. doi: 10.1016/S0140-6736(22)01438-6
30. Fenga, C. Occupational exposure and risk of breast cancer (review). Biomed Rep. (2016) 4:282–92. doi: 10.3892/br.2016.575
31. Mukherjea, D, Dhukhwa, A, Sapra, A, Bhandari, P, Woolford, K, Franke, J, et al. Strategies to reduce the risk of platinum containing antineoplastic drug-induced ototoxicity. Expert Opin Drug Metab Toxicol. (2020) 16:965–82. doi: 10.1080/17425255.2020.1806235
32. Santos, N, Ferreira, RS, and Santos, ACD. Overview of cisplatin-induced neurotoxicity and ototoxicity, and the protective agents. Food Chem Toxicol. (2020) 136:111079. doi: 10.1016/j.fct.2019.111079
33. Steyger, PS. Potentiation of chemical ototoxicity by noise. Semin Hear. (2009) 30:038–46. doi: 10.1055/s-0028-1111105
34. Lawther, AJ, Phillips, AJK, Chung, N-C, Chang, A, Ziegler, AI, Debs, S, et al. Disrupting circadian rhythms promotes cancer-induced inflammation in mice. Brain Behav Immun Health. (2022) 21:100428. doi: 10.1016/j.bbih.2022.100428
35. Shostak, A. Circadian clock, cell division, and cancer: from molecules to organism. Int J Mol Sci. (2017) 18:873. doi: 10.3390/ijms18040873
36. Hernández-Rosas, F, López-Rosas, CA, and Saavedra-Vélez, MV. Disruption of the molecular circadian clock and cancer: an epigenetic link. Biochem Genet. (2020) 58:189–209. doi: 10.1007/s10528-019-09938-w
37. Butow, P, Laidsaar-Powell, R, Konings, S, Lim, CYS, and Koczwara, B. Return to work after a cancer diagnosis: a meta-review of reviews and a meta-synthesis of recent qualitative studies. J Cancer Surviv. (2020) 14:114–34. doi: 10.1007/s11764-019-00828-z
38. Guo, YJ, Tang, J, Li, JM, Zhu, LL, and Xu, JS. Exploration of interventions to enhance return-to-work for cancer patients: a scoping review. Clin Rehabil. (2021) 35:1674–93. doi: 10.1177/02692155211021706
39. Sohn, KJ, Park, SY, and Kim, S. A scoping review of return to work decision-making and experiences of breast cancer survivors in Korea. Support Care Cancer. (2021) 29:1741–51. doi: 10.1007/s00520-020-05817-y
40. Tan, FSI, and Shorey, S. Experiences of women with breast cancer while working or returning to work: a qualitative systematic review and meta-synthesis. Support Care Cancer. (2022) 30:2971–82. doi: 10.1007/s00520-021-06615-w
41. van Muijen, P, Weevers, NL, Snels, IA, Duijts, SF, Bruinvels, DJ, Schellart, AJ, et al. Predictors of return to work and employment in cancer survivors: a systematic review. Eur J Cancer Care (Engl). (2013) 22:144–60. doi: 10.1111/ecc.12033
42. Kane, D, Rajacich, D, and Andary, C. Experiences of cancer patients' return to work. Can Oncol Nurs J. (2020) 30:113–8. doi: 10.5737/23688076302113118
43. Paltrinieri, S, Fugazzaro, S, Bertozzi, L, Bassi, MC, Pellegrini, M, Vicentini, M, et al. Return to work in European cancer survivors: a systematic review. Support Care Cancer. (2018) 26:2983–94. doi: 10.1007/s00520-018-4270-6
44. Steiner, JF, Cavender, TA, Main, DS, and Bradley, CJ. Assessing the impact of cancer on work outcomes: what are the research needs? Cancer. (2004) 101:1703–11. doi: 10.1002/cncr.20564
45. Arndt, V, Koch-Gallenkamp, L, Bertram, H, Eberle, A, Holleczek, B, Pritzkuleit, R, et al. Return to work after cancer. A multi-regional population-based study from Germany. Acta Oncol. (2019) 58:811–8. doi: 10.1080/0284186X.2018.1557341
46. Mehnert, A, and Koch, U. Predictors of employment among cancer survivors after medical rehabilitation--a prospective study. Scand J Work Environ Health. (2013) 39:76–87. doi: 10.5271/sjweh.3291
47. Emerson, MA, Reeve, BB, Gilkey, MB, Elmore, SNC, Hayes, S, Bradley, CJ, et al. Job loss, return to work, and multidimensional well-being after breast cancer treatment in working-age black and white women. J Cancer Surviv. (2022). doi: 10.1007/s11764-022-01252-6
48. Paltrinieri, S, Ricchi, E, Mazzini, E, Cervi, E, Sandri, E, Fugazzaro, S, et al. A social-healthcare pathway to facilitate return to work of cancer survivors in Italy: the UNAMANO project. Work. (2021) 70:1243–53. doi: 10.3233/WOR-205249
49. van Maarschalkerweerd, PEA, Schaapveld, M, Paalman, CH, Aaronson, NK, and Duijts, SFA. Changes in employment status, barriers to, and facilitators of (return to) work in breast cancer survivors 5-10 years after diagnosis. Disabil Rehabil. (2020) 42:3052–8. doi: 10.1080/09638288.2019.1583779
50. Algeo, N, Bennett, K, and Connolly, D. Breast cancer survivorship and employment in Ireland: legislative systems and the return to work of women with breast cancer. Work. (2022) 71:927–39. doi: 10.3233/WOR-205044
51. Ho, PJ, Hartman, M, Gernaat, SAM, Cook, AR, Lee, SC, Hupkens, L, et al. Associations between workability and patient-reported physical, psychological and social outcomes in breast cancer survivors: a cross-sectional study. Support Care Cancer. (2018) 26:2815–24. doi: 10.1007/s00520-018-4132-2
52. Lewis, J, and Mackenzie, L. Cognitive changes after breast cancer: a scoping review to identify problems encountered by women when returning to work. Disabil Rehabil. (2022) 44:5310–28. doi: 10.1080/09638288.2021.1919216
53. Wennman-Larsen, A, Svärd, V, Alexanderson, K, and Friberg, E. Factors of decisive importance for being in work or not during two years after breast cancer surgery: content analysis of 462 women's open answers. BMC Womens Health. (2021) 21:332. doi: 10.1186/s12905-021-01468-1
54. Zomkowski, K, Cruz de Souza, B, Moreira, GM, Volkmer, C, Da Silva Honório, GJ, Moraes Santos, G, et al. Qualitative study of return to work following breast cancer treatment. Occup Med (Lond). (2019) 69:189–94. doi: 10.1093/occmed/kqz024
55. Kong, YC, Wong, LP, Ng, CW, Taib, NA, Bhoo-Pathy, NT, Yusof, MM, et al. Understanding the financial needs following diagnosis of breast cancer in a setting with universal health coverage. Oncologist. (2020) 25:497–504. doi: 10.1634/theoncologist.2019-0426
56. Meernik, C, Sandler, DP, Peipins, LA, Hodgson, ME, Blinder, VS, Wheeler, SB, et al. Breast cancer-related employment disruption and financial hardship in the sister study. JNCI Cancer Spectr. (2021) 5:pkab024. doi: 10.1093/jncics/pkab024
57. Richter-Ehrenstein, C, and Martinez-Pader, J. Impact of breast cancer diagnosis and treatment on work-related life and financial factors. Breast Care. (2021) 16:72–6. doi: 10.1159/000507313
58. Algeo, N, Bennett, K, and Connolly, D. Rehabilitation interventions to support return to work for women with breast cancer: a systematic review and meta-analysis. BMC Cancer. (2021) 21:895. doi: 10.1186/s12885-021-08613-x
59. Ayala-Garcia, A, Serra, L, Hernando-Rodriguez, JC, and Benavides, FG. Returning to work after a sickness absence due to cancer: a cohort study of salaried workers in Catalonia (Spain). Sci Rep. (2021) 11:23953. doi: 10.1038/s41598-021-03368-8
60. Pauge, S, Surmann, B, Mehlis, K, Zueger, A, Richter, L, Menold, N, et al. Patient-reported financial distress in cancer: a systematic review of risk factors in universal healthcare systems. Cancers (Basel). (2021) 13:5015. doi: 10.3390/cancers13195015
61. Brünger, M, Bernert, S, and Spyra, K. Occupation as a proxy for job exposures? Routine data analysis using the example of rehabilitation. Gesundheitswesen. (2020) 82:S41–s51. doi: 10.1055/a-0965-6777 (Berufstätigkeit als Proxy für Arbeitsbelastungen? Routinedatenanalyse am Beispiel der Rehabilitation.)
62. Wild, CP. The exposome: from concept to utility. Int J Epidemiol. (2012) 41:24–32. doi: 10.1093/ije/dyr236
63. Eyles, E, Manley, D, and Jones, K. Occupied with classification: which occupational classification scheme better predicts health outcomes? Soc Sci Med. (2019) 227:56–62. doi: 10.1016/j.socscimed.2018.09.020
64. Bopp, M, Spoerri, A, Zwahlen, M, Gutzwiller, F, Paccaud, F, Braun-Fahrländer, C, et al. Cohort profile: the Swiss National Cohort--a longitudinal study of 6.8 million people. Int J Epidemiol. (2009) 38:379–84. doi: 10.1093/ije/dyn042
65. SFSO. Methodology report—Coverage estimation for the Swiss population census 2000. Neuchâtel: Swiss Federal Statistical Office (2004).
66. ILO. International standard classification of occupations 2008 (ISCO-08): Structure, group definitions and correspondence tables. Geneva: International Labour Office (2012).
67. SFSO. (2016). Catégories socioprofessionnelles (CSP) 2010 - Opérationnalisation des CSP dans le système des variables-clés SHAPE dès 2010. Available at: https://www.bfs.admin.ch/hub/api/dam/assets/286159/master
68. IARC. International rules for multiple primary cancers (ICD-0 third edition). Eur J Cancer Prev. (2005) 14:427–9. doi: 10.1097/00008469-200508000-00017
69. Sobin, LH, Gospodarowicz, MK, and Wittekind, C. TNM classification of malignant tumours. Hoboken, NJ: John Wiley & Sons (2011).
70. van Buuren, S, and Groothuis-Oudshoorn, K. Mice: multivariate imputation by chained equations in R. J Stat Softw. (2011) 45:1–67. doi: 10.18637/jss.v045.i03
71. Spratt, M, Carpenter, J, Sterne, JA, Carlin, JB, Heron, J, Henderson, J, et al. Strategies for multiple imputation in longitudinal studies. Am J Epidemiol. (2010) 172:478–87. doi: 10.1093/aje/kwq137
72. Berkson, J., and Gage, R. Calculation of survival rates for cancer. Proc Staff Meet Mayo Clin. (1950) 25:270-86.
73. Schaffar, R, Rachet, B, Belot, A, and Woods, LM. Estimation of net survival for cancer patients: relative survival setting more robust to some assumption violations than cause-specific setting, a sensitivity analysis on empirical data. Eur J Cancer. (2017) 72:78–83. doi: 10.1016/j.ejca.2016.11.019
74. Estève, J, Benhamou, E, and Raymond, L. Statistical methods in cancer research. Volume IV. Descriptive epidemiology. IARC Sci Publ. (1994):1–302.
75. Perme, MP, Stare, J, and Estève, J. On estimation in relative survival. Biometrics. (2012) 68:113–20. doi: 10.1111/j.1541-0420.2011.01640.x
76. Grafféo, N, Castell, F, Belot, A, and Giorgi, R. A log-rank-type test to compare net survival distributions. Biometrics. (2016) 72:760–9. doi: 10.1111/biom.12477
77. Remontet, L, Bossard, N, Belot, A, and Estève, J, the French network of cancer registries FRANCIM. An overall strategy based on regression models to estimate relative survival and model the effects of prognostic factors in cancer survival studies. Stat Med. (2007) 26:2214–28. doi: 10.1002/sim.2656
78. Clerc-Urmès, I, Grzebyk, M, and Hédelin, G. Net survival estimation with stns. Stata J. (2014) 14:87–102. doi: 10.1177/1536867X1401400107
79. Woods, LM, Rachet, B, Riga, M, Stone, N, Shah, A, and Coleman, MP. Geographical variation in life expectancy at birth in England and Wales is largely explained by deprivation. J Epidemiol Community Health. (2005) 59:115–20. doi: 10.1136/jech.2003.013003
80. Clerc-Urmès, I., Grzebyk, M., and Hédelin, G. (2021). Flexrsurv: flexible relative survival analysis.
81. Giorgi, R, Abrahamowicz, M, Quantin, C, Bolard, P, Esteve, J, Gouvernet, J, et al. A relative survival regression model using B-spline functions to model non-proportional hazards. Stat Med. (2003) 22:2767–84. doi: 10.1002/sim.1484
82. Akaike, H. Information theory and the maximum likelihood principle. In: Petrov BN, Csaki F, editors. Conference proceedings of the Second international symposium on information theory. Budapest: Akadémiai Kiadó (1973). p. 267-281.
83. Hussain, SK, Altieri, A, Sundquist, J, and Hemminki, K. Influence of education level on breast cancer risk and survival in Sweden between 1990 and 2004. Int J Cancer. (2008) 122:165–9. doi: 10.1002/ijc.23007
84. Palme, M, and Simeonova, E. Does women's education affect breast cancer risk and survival? Evidence from a population based social experiment in education. J Health Econ. (2015) 42:115–24. doi: 10.1016/j.jhealeco.2014.11.001
85. Li, H, Hou, L, Yu, Y, Sun, X, Liu, X, Yu, Y, et al. Lipids, anthropometric measures, smoking and physical activity mediate the causal pathway from education to breast cancer in women: a Mendelian randomization study. J Breast Cancer. (2021) 24:504–19. doi: 10.4048/jbc.2021.24.e53
86. Medina, HN, Callahan, KE, Koru-Sengul, T, Maheshwari, S, Liu, Q, Goel, N, et al. Elevated breast cancer mortality among highly educated Asian American women. PLoS One. (2022) 17:e0268617. doi: 10.1371/journal.pone.0268617
87. Taheri, M, Tavakol, M, Akbari, ME, Almasi-Hashiani, A, and Abbasi, M. Relationship of socio economic status, income, and education with the survival rate of breast cancer: a meta-analysis. Iran J Public Health. (2019) 48:1428–38.
88. SFSO. [Swiss labor force survey. Part-time work in Switzerland 2017]. Neuchatel, Switzerland: Swiss Federal Statistical Office. (2019).
89. Slavova-Azmanova, NS, Newton, JC, Saunders, C, and Johnson, CE. ‘Biggest factors in having cancer were costs and no entitlement to compensation’—the determinants of out-of-pocket costs for cancer care through the lenses of rural and outer metropolitan Western Australians. Aust J Rural Health. (2020) 28:588–602. doi: 10.1111/ajr.12686
90. Grivaux, M, Zureik, M, Marsal, L, Asselain, B, Peureux, M, Chavaillon, JM, et al. Five-year survival for lung cancer patients managed in general hospitals. Rev Mal Respir. (2011) 28:e31–8. doi: 10.1016/j.rmr.2008.07.001
91. Engel, CL, Sharima Rasanayagam, M, Gray, JM, and Rizzo, J. Work and female breast cancer: the state of the evidence, 2002–2017. New Solut. (2018) 28:55–78. doi: 10.1177/1048291118758460
92. Hartman, SJ, Weiner, LS, Natarajan, L, Sears, DD, Palmer, BW, Parker, B, et al. A randomized trial of physical activity for cognitive functioning in breast cancer survivors: rationale and study design of I can! Improving cognition after cancer. Contemp Clin Trials. (2021) 102:106289. doi: 10.1016/j.cct.2021.106289
93. Jackson, I, Rowan, P, Padhye, N, Hwang, LY, and Vernon, SW. Trends in health-related quality of life of female breast cancer survivors using the medical expenditure panel survey (MEPS), 2008-2016. Qual Life Res. (2021) 30:3547–58. doi: 10.1007/s11136-021-02895-1
94. Kim, SY, Kissane, DW, Richardson, G, Senior, J, Morgan, J, Gregory, P, et al. The role of depression and other psychological factors in work ability among breast cancer survivors in Australia. Psychooncology. (2022) 31:167–75. doi: 10.1002/pon.5802
95. O'Hea, EL, Creamer, S, Flahive, JM, Keating, BA, Crocker, CR, Williamson, SR, et al. Survivorship care planning, quality of life, and confidence to transition to survivorship: a randomized controlled trial with women ending treatment for breast cancer. J Psychosoc Oncol. (2022) 40:574–94. doi: 10.1080/07347332.2021.1936336
96. Schmidt, ME, Scherer, S, Wiskemann, J, and Steindorf, K. Return to work after breast cancer: the role of treatment-related side effects and potential impact on quality of life. Eur J Cancer Care (Engl). (2019) 28:e13051. doi: 10.1111/ecc.13051
97. Klaver, KM, Duijts, SFA, Geusgens, CAV, Aarts, MJB, Ponds, R, van der Beek, AJ, et al. Neuropsychological test performance and self-reported cognitive functioning associated with work-related outcomes in occupationally active cancer survivors with cognitive complaints. J Cancer Surviv. (2022). doi: 10.1007/s11764-022-01223-x
98. West, T, Cavallero, C, Ceccherini, R, Foladore, S, Generali, D, Versace, F, et al. Impact of psychosocial, behavioral and lifestyle factors on subjective cognitive complaints and perceived quality of life in a large cohort of Italian breast cancer patients. Front Psychol. (2022) 13:1015573. doi: 10.3389/fpsyg.2022.1015573
99. Boykoff, N, Moieni, M, and Subramanian, SK. Confronting chemobrain: an in-depth look at survivors' reports of impact on work, social networks, and health care response. J Cancer Surviv. (2009) 3:223–32. doi: 10.1007/s11764-009-0098-x
100. Caumette, E, Vaz-Luis, I, Pinto, S, Havas, J, Bovagnet, T, Ruiz de Azua, G, et al. The challenge of return to work after breast cancer: the role of family situation, CANTO Cohort. Curr Oncol. (2021) 28:3866–75. doi: 10.3390/curroncol28050330
101. Lorez, M, Bordoni, A, Bouchardy, C, Bulliard, J-L, Camey, B, Dehler, S, et al. Evaluation of completeness of case ascertainment in Swiss cancer registration. Eur J Cancer Prev. (2017) 26:S139–46. doi: 10.1097/CEJ.0000000000000380
102. European Agency for Safety and Health at Work. Priorities for occupational safety and health research in Europe: 2013-2020 Publications Office of the European Union, Luxembourg. (2013). Available at: https://osha.europa.eu/en/publications/priorities-occupational-safety-and-health-research-europe-2013-2020
103. Grafféo, N, Jooste, V, and Giorgi, R. The impact of additional life-table variables on excess mortality estimates. Stat Med. (2012) 31:4219–30. doi: 10.1002/sim.5493
104. Bovio, N, Richardson, DB, and Guseva Canu, I. Sex-specific risks and trends in lung cancer mortality across occupations and economic activities in Switzerland (1990-2014). Occup Environ Med. (2020) 77:540–8. doi: 10.1136/oemed-2019-106356
105. Bovio, N, Wild, P, Guseva Canu, I, and Swiss National, C. Lung cancer mortality in the Swiss working population: the effect of occupational and non-occupational factors. J Occup Environ Med. (2021) 63:1029–36. doi: 10.1097/JOM.0000000000002302
106. Plys, E, Bovio, N, Arveux, P, Bergeron, Y, Bulliard, JL, Elia, N, et al. Research on occupational diseases in the absence of occupational data: a mixed-method study among cancer registries of Western Switzerland. Swiss Med Wkly. (2022) 152:w30127. doi: 10.4414/SMW.2022.w30127
107. Bulliard, JL, Ducros, C, Germann, S, Arveux, P, and Bochud, M. New law for cancer registration in Switzerland: opportunities and challenges. Rev Med Suisse. (2020) 16:2099–103. doi: 10.53738/REVMED.2020.16.713.2099
Keywords: occupational epidemiology, cohort, cancer registry, breast cancer, female worker, return to work, occupational exposure
Citation: Guseva Canu I, Bovio N, Arveux P, Bulliard J-L, Fournier E, Germann S, Konzelmann I, Maspoli M, Rapiti E and Grzebyk M (2023) Breast cancer and occupation: Non-parametric and parametric net survival analyses among Swiss women (1990–2014). Front. Public Health. 11:1129708. doi: 10.3389/fpubh.2023.1129708
Edited by:
Alberto Modenese, University of Modena and Reggio Emilia, ItalyReviewed by:
Neela Guha, California Environmental Protection Agency, United StatesTommaso Filippini, University of Modena and Reggio Emilia, Italy
Copyright © 2023 Guseva Canu, Bovio, Arveux, Bulliard, Fournier, Germann, Konzelmann, Maspoli, Rapiti and Grzebyk. This is an open-access article distributed under the terms of the Creative Commons Attribution License (CC BY). The use, distribution or reproduction in other forums is permitted, provided the original author(s) and the copyright owner(s) are credited and that the original publication in this journal is cited, in accordance with accepted academic practice. No use, distribution or reproduction is permitted which does not comply with these terms.
*Correspondence: Irina Guseva Canu, aXJpbmEuZ3VzZXZhLWNhbnVAdW5pc2FudGUuY2g=