- 1Department of Pulmonary and Critical Care Medicine, Center of Respiratory Medicine, China-Japan Friendship Hospital, Beijing, China
- 2National Center for Respiratory Medicine, Beijing, China
- 3Institute of Respiratory Medicine, Chinese Academy of Medical Sciences, Beijing, China
- 4National Clinical Research Center for Respiratory Diseases, Beijing, China
- 5Peking Union Medical College, Chinese Academy of Medical Sciences, Beijing, China
- 6Peking University China-Japan Friendship School of Clinical Medicine, Beijing, China
- 7State Key Laboratory of Genetic Engineering, School of Life Sciences, Human Phenome Institute, Metabonomics and Systems Biology Laboratory at Shanghai International Centre for Molecular Phenomics, Fudan University, Shanghai, China
Introduction: The metabolomic changes caused by airborne fine particulate matter (PM2.5) exposure in patients with chronic obstructive pulmonary disease (COPD) remain unclear. The aim of this study was to determine whether it is possible to predict PM2.5-induced acute exacerbation of COPD (AECOPD) using metabolic markers.
Methods: Thirty-eight patients with COPD diagnosed by the 2018 Global Initiative for Obstructive Lung Disease were selected and divided into high exposure and low exposure groups. Questionnaire data, clinical data, and peripheral blood data were collected from the patients. Targeted metabolomics using liquid chromatography-tandem mass spectrometry was performed on the plasma samples to investigate the metabolic differences between the two groups and its correlation with the risk of acute exacerbation.
Results: Metabolomic analysis identified 311 metabolites in the plasma of patients with COPD, among which 21 metabolites showed significant changes between the two groups, involving seven pathways, including glycerophospholipid, alanine, aspartate, and glutamate metabolism. Among the 21 metabolites, arginine and glycochenodeoxycholic acid were positively associated with AECOPD during the three months of follow-up, with an area under the curve of 72.50% and 67.14%, respectively.
Discussion: PM2.5 exposure can lead to changes in multiple metabolic pathways that contribute to the development of AECOPD, and arginine is a bridge between PM2.5 exposure and AECOPD.
1. Introduction
Chronic obstructive pulmonary disease (COPD) is a common chronic disease, mainly characterized by persistent respiratory symptoms and airflow restriction, resulting in airway and/or alveolar abnormalities. Its pathogenesis is not clear and is generally considered to be related to exposure to toxic air pollutants, especially particulate matters (1). The 2017 Global Burden of Disease Study showed that approximately 300 million individuals worldwide suffer from COPD, and the number of deaths due to COPD is as high as 3.2 million every year (2, 3), ranking fifth in the world's burden of disease. It is expected that COPD will become the third leading cause of death in the world by 2022 (4). The pathogenesis of COPD is very complex, and its potential cellular and molecular mechanisms remain unclear. COPD is a heterogeneous disease, which brings many challenges in its diagnosis, prognosis, and management. Therefore, it is imperative to identify reliable, easily measurable, and clinically relevant biomarkers of COPD to improve the prognosis of patients.
Among the pathogenic factors of COPD, particulate matter (PM) is closely related to the occurrence and development of COPD, especially PM with particle diameter < 2.5 μm (PM2.5), the main pathogenic component of air pollutants. Due to the small particle diameter, PM2.5 can be deposited in the alveoli, which leads to disorders of the airway immune system. PM2.5 enhances lung inflammation and enters blood circulation through the blood-gas barrier, causing serious health hazards to the body (5). Exposure to PM2.5 in the air is significantly associated with an increase in COPD prevalence and an increase in the acute exacerbation risk, hospitalization rate, and mortality of patients with COPD (6, 7). Concurrently, exposure to PM2.5 for a short time could lead to a systemic inflammatory response in patients with COPD (8). Therefore, it is important to explore the mechanism of PM2.5 exposures leading to COPD.
Metabolomics is the quantitative description of metabolites in organisms and their responses to changes in physiology, disease, and environment. It can provide mechanistic clues regarding biological processes and functions, which help deepen the understanding of the occurrence and development of diseases. It has been shown that disorders of phospholipid metabolism are associated with the development of emphysema and acute exacerbation of COPD (AECOPD) (9). Meanwhile, air pollutants are known to have an effect on metabolic disorders (10). Sun et al. showed that air pollution exposure can affect lipid metabolism disorders and ultimately lead to the development of diabetes mellitus (11). Air pollutants can affect arginine and proline metabolism, which can affect the cardiopulmonary system (12). Air pollutants can also promote atherosclerotic lesions by affecting cholesterol dysregulation (13). However, limited studies have examined the effects of air pollution on the development of COPD.
Therefore, the present study aims to recognize which metabolites and metabolic pathways are influenced by outdoor air pollutants and the predictive value of the metabolites on AECOPD. We divided the patients with COPD into high and low pollutant exposure groups and explored the effect of PM2.5 on metabolism by performing targeted metabolomic assays on plasma to identify relevant markers that can predict AECOPD due to air pollution.
2. Methods
2.1. Study design and participants
This was an observational clinical study conducted from January 2018 to March 2019. The participants were patients with COPD in the Beijing-Tianjin-Hebei region, China. The inclusion criteria were as follows: (1) age 45–75 years; (2) met the diagnostic criteria for the global initiative for COPD (GOLD-2018) and were in a stable stage of disease within 1 month at the time of enrollment; (3) resided in the local area continuously for more than 2 years, with no long-term plan to leave the area during the survey period; and (4) had no smoking history or had stopped smoking for more than half a year to rule out the effect of smoking on AECOPD. The exclusion criteria were as follows: (1) had complications, such as malignant tumor, severe cardiovascular and cerebrovascular disease, liver and kidney insufficiency, and active pulmonary tuberculosis; (2) received efficacy evaluation for epilepsy or mental illness; (3) underwent chest, abdominal or ophthalmic surgery in the past 3 months; and (4) pregnant and lactating women. All patients were surveyed using a questionnaire that included the basic information on the COPD assessment test (CAT), modified Medical Research Council (mMRC) dyspnea scale, St. George's respiratory questionnaire, pulmonary function examination, physical examination (height, weight, and blood pressure), and peripheral blood collection. After 3 months, telephone follow-up was conducted to monitor any acute exacerbations during this period, including requiring treatment with corticosteroids and/or antibiotics or hospitalization (9). The research protocol was approved by the ethics committee of the China-Japan Friendship Hospital, and all participants signed informed consent (IRB Approval Number: 2017–19). All methods were carried out in accordance with the Declaration of Helsinki.
2.2. Outdoor PM2.5 exposure assessment
The outdoor PM2.5 exposure concentrations in this study were obtained from the China National Environmental Monitoring Center (http://www.cnemc.cn/), and the daily estimates of pollutants at each monitoring station were calculated as the 24-hour average concentrations at the corresponding monitoring station. After matching the PM2.5 data with the subjects' addresses from the questionnaires, the average concentration of PM2.5 for the month before the survey date in the participant's city was obtained. The participants were divided into a high exposure group (>75 μg/m3) and a low exposure group (< 35 μg/m3) based on the PM2.5 concentrations according to the National Ambient Air Quality Standards (GB 3095-2012).
2.3. Plasma-targeted metabolomic assays
Blood samples were collected from all patients in a fasting state and transferred to the Department of Laboratory Medicine of China-Japan Friendship Hospital and stored at −80°C for metabolomic analysis.
Targeted quantitative metabolomics analysis was performed using the Biocrates P500 platform (Biocrates Life Sciences AG, Innsbruck, Austria). Using a previously described method, 10 μL of each plasma sample was pretreated in 96-well plates (14) and then analyzed using liquid chromatography-tandem mass spectrometry (LC-MS) and flow injection analysis (FIA). LC-MS used two liquid chromatography separation methods, and mass spectrometry used the multiple reaction monitoring mode, collecting positive and negative ion modes, respectively. FIA used the same liquid chromatography separation method and collected data by two mass spectrometry methods in the positive ion mode. The raw mass spectrometry data were analyzed using the MetLIMS software (Biocrates Life Sciences AG), and the concentration of the metabolites in the samples was calculated using the 7-point calibration standard curve method and single-point method for LC-MS and FIA data, respectively. All samples were randomly assigned, and quality control (QC) samples with known concentrations were inserted into the sample cohort to assess the reproducibility of the data. The filter thresholds for metabolites were QC samples with coefficients of variation ≥ 25% and missing values ≥ 30%. The metabolites were annotated via the Human Metabolite Database (HMDB).
2.4. Statistical analysis
The differences in the demographic characteristics between the groups were analyzed using t-tests for continuous variables and chi-square tests for categorical variables. For metabolomic analysis, the Mann–Whitney non-parametric test and orthogonal partial least squares discriminant analysis (OPLS-DA) were used to analyze between-group differences in metabolites after metabolite concentrations were log-transformed. P-value < 0.01 and variable importance in the projection score (VIP) > 1.5 were used as the threshold for differential metabolite screening. Pathway analysis was performed for significantly changed metabolites with HMDB numbers using the Kyoto Encyclopedia of Genes and Genomes (KEGG; Kanehisa Laboratories, Kyoto, Japan) database, and enrichment analysis was performed using the Small Molecule Pathway Database. Logistic regression was used to assess the association of variables with the risk of acute exacerbation during the 3 months of follow-up, with sex, age, smoking history, and BMI as covariates. The predictive power of important metabolites was assessed using receiver operating characteristic (ROC) curves and the area under the curve (AUC). Data and statistical analyses were performed using SPSS 26.0 (IBM, Armonk, NY, USA). OPLS-DA was performed using SIMCA 14.0 (Umetrics, Umeå, Sweden). Pathway analyses were performed on the platform MetaboAnalyst 5.0 (https://www.metaboanalyst.ca). Logistics regression was calculated by SPSS 26.0 (IBM, Armonk, NY), and ROC curves were drawn by GraphPad Prism 9.0. A P-value < 0.05 was considered statistically significant.
3. Results
3.1. Characteristics of the participants
Thirty-eight participants with complete data were included in the analysis, with 19 in the high exposure group (mean age 62.8 ± 7.8 years, 84.2% male) and 19 in the low exposure group (mean age 62.7 ± 4.7 years, 63.2% male). The demographic characteristics of the study population are shown in Table 1. The average concentration of outdoor PM2.5 exposure was 19.0 ± 1.02 μg/m3 and 106.0 ± 15.3 μg/m3 in the low and high exposure groups, respectively. The presence or absence of AECOPD during the 3 months of follow-up and CAT questionnaire scores were statistically different between the two groups (P = 0.02, 0.01, and 0.008, respectively).
3.2. Effects of PM2.5 exposure on metabolites
Plasma metabolomics data from the 38 participants were collected. The LC-MS method and FIA method were used to obtain 106 and 524 metabolites, respectively. Thus, 311 metabolites were included in the analysis after data QC. OPLS-DA analysis showed a separation trend between the low- and high-exposure groups. Twenty-one metabolites were statistically different between the two groups based on a P-value < 0.01 and VIP >1.5 (Figure 1A and Table 2, see also Supplementary material 1). These metabolites included cholesterol ester, triglycerides, sphingolipids, phospholipids, lyso-phospholipids, amino acids, choline, and bile acids. The volcano plot and heat map showed that six metabolites showed elevated expression, and the expression levels of 15 metabolites decreased with exposure to high PM2.5 levels compared with exposure to low PM2.5 levels (Figure 1, see also Supplementary material 2). Although the inclusion criteria are patients who had no smoking history or had stopped smoking for more than half a year to rule out the influence of smoking, we also divided the patients into two groups according to smoking status (ever smoker vs. never smoker), and compared the different metabolites after exposure to high and low PM2.5 level (Supplementary material 3). The results showed that, in both groups, most metabolites have certain statistical significance. This means that air pollution exposure had a certain impact on ever smoker and never smoker. To further investigate the effects of molecular pathways underlying PM2.5 exposure and AECOPD, we performed pathway analysis based on the KEGG database (Figure 2). It was observed that seven metabolic pathways were correlated with PM2.5 exposure, including glycerophospholipid, alanine, aspartate, glutamate, arginine, proline metabolism, and arginine biosynthesis. These results suggest that metabolic disturbances occur after PM2.5 exposure, including disturbances in amino acid, bile acid, and lipid metabolisms (Figure 3).
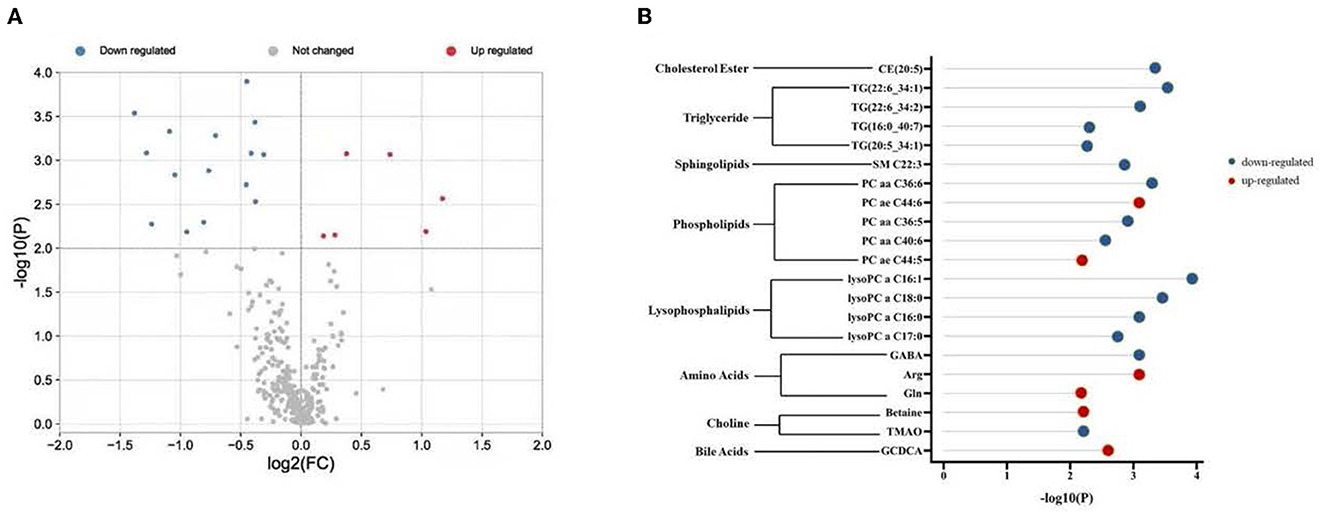
Figure 1. Different metabolites in two groups. (A) Volcano plot shows -log10 P against the log2-fold change of 311 metabolites in the high exposure group vs. the low exposure group. Blue denotes down-regulated metabolites, red denotes up-regulated metabolites, and gray denotes no change. (B) Twenty-one differentially regulated metabolites were associated with PM2.5 exposure. Red indicates up-regulation and blue indicates down-regulation. PM2.5, PM with particle diameter <2.5 μm; GABA, γ-aminobutyric acid; Arg, arginine; Gln, Glutamine; TMAO, trimethylamine N-oxide; GCDCA, glycochenodeoxycholic acid.
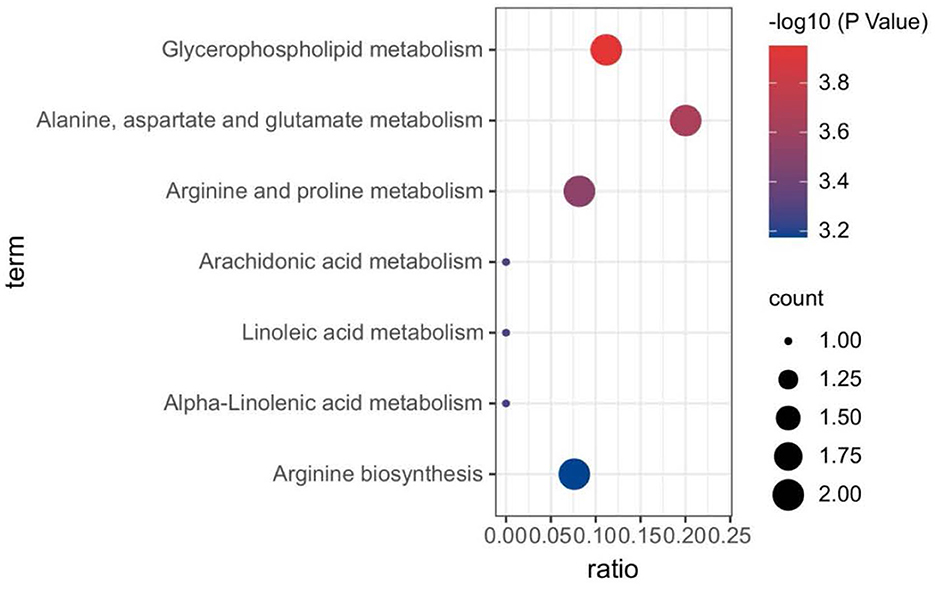
Figure 2. Biological pathways associated with PM2.5 exposure. The color gradient indicates the significance of the pathway ranked by P-value (blue: higher P-values and red: lower P-values), and circle size indicates the pathway impact score (the larger circle the higher impact score). PM2.5, PM with particle diameter <2.5 μm.
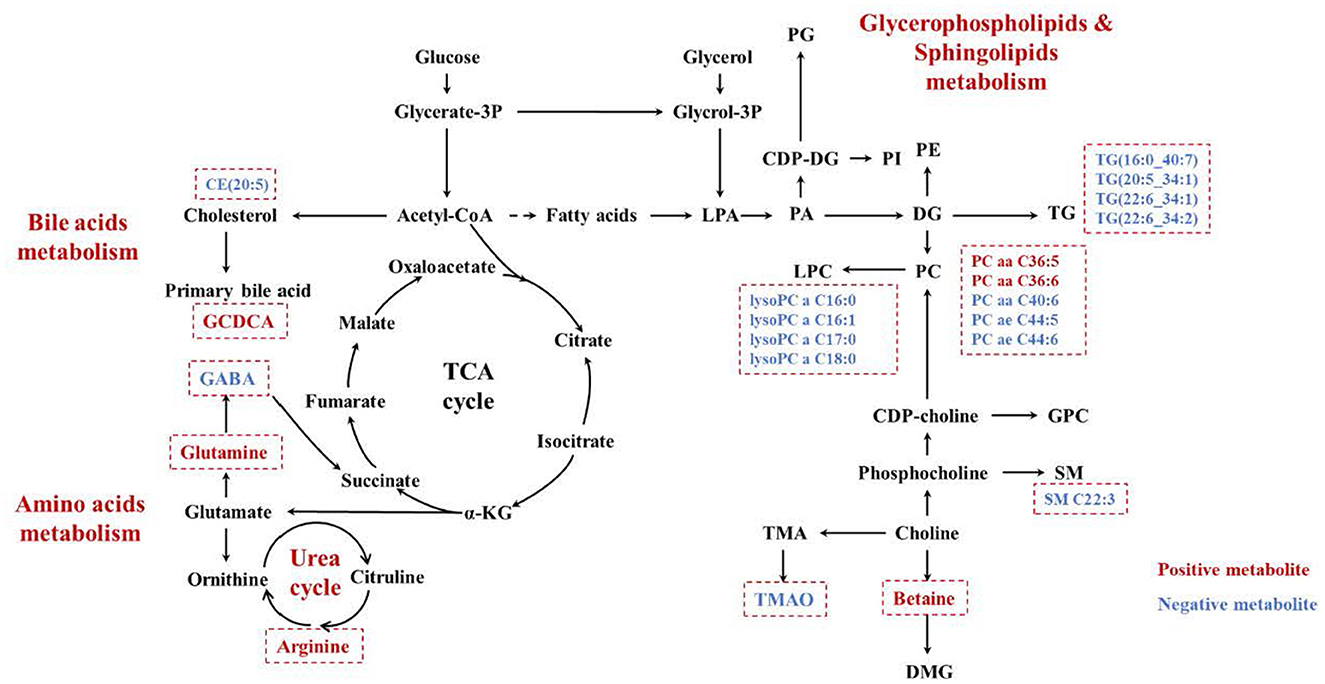
Figure 3. The increased metabolites were marked in red, while the decreased ones were marked in blue. PG, polygalacturonase; CDP-DG, cytidine 5'-diphosphate 1,2-diacyl-sn-glycerol; LPA, lysophosphatidic acid; PA, phosphatidic acid; PI, phosphoinositide; PE, phosphatidylethanolamine; DG, diacylglycerol; PC, phosphatidylcholine; LPC, lysophosphatidylcholine; TG, triglyceride; GPC, l-α- Glycerophosphatidylcholine; SM, sphingomyelin; DMG, dimethylglycine; TMA, trimethylamine; TMAO, trimethylamine N-oxide; PM2.5, PM with particle diameter <2.5 μm.
3.3. Predicting the risk of AECOPD based on metabolic changes
The association of 21 metabolites with the risk of AECOPD in the next 3 months is shown in Table 3. Arginine and glycochenodeoxycholic acid (GCDCA) were positively associated with AECOPD when corrected for sex, age, BMI, and smoking history (OR = 1.028, 95%CI 1.001–1.056, P = 0.045 and OR = 3.974, 95%CI 1.017–15.537, P = 0.047, respectively). Subsequently, the AUC obtained from the ROC analysis was used to assess the predictive performance of these two metabolites for AECOPD (Figure 4). Arginine had better predictive performance (AUC = 72.50%, 95% CI 0.53–0.92) compared with that of GCDCA (AUC = 67.14%, 95% CI 0.45–0.90).
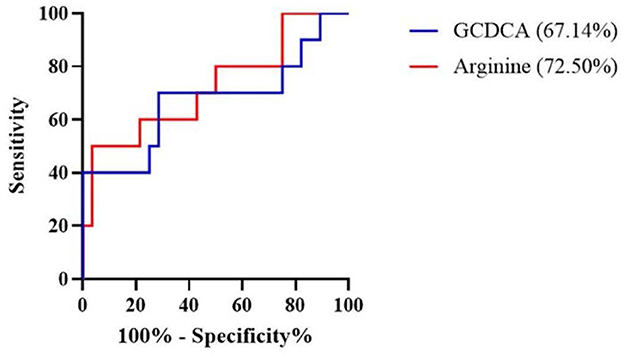
Figure 4. Receiver operating characteristic (ROC) curve revealed candidate biomarkers for predicting AECOPD. AECOPD, acute exacerbation of chronic obstructive pulmonary disease; GCDCA, glycochenodeoxycholic acid.
4. Discussion
PM2.5 exposure can reportedly lead to acute exacerbation in patients with COPD, but the exact mechanism of action is unclear. In this study, we divided the patients into high and low exposure groups according to the exposure level of outdoor PM2.5, and detected the plasma metabolites. We found that 21 metabolites showed significant changes between the two groups. Meanwhile, we found that PM2.5 exposure could affect lipid-, amino acid-, and bile acid-related metabolism (Figure 3) by performing targeted metabolomic assays on the plasma of patients with COPD with different PM2.5 exposure levels. Correlation analysis of the metabolites with the occurrence of acute exacerbations in 3 months revealed that arginine and GCDCA were positively correlated with the occurrence of AECOPD, while arginine better predicted AECOPD. These findings suggest that arginine is a potential marker of PM2.5-induced AECOPD.
Several studies have confirmed the correlation between PM2.5 and the development of COPD. Yang et al. found that long-term PM2.5 exposure can reduce the airway and small airway function and impair lung function (15). Liu et al. conducted a 16-year cohort study and found that for every 5 μg/m3 increase in PM2.5 concentration, the adjusted hazard ratio for associations with COPD incidence was 1.17 (16). The hospitalization and mortality rates for COPD increase during periods of high air pollution. Each 10 μg/m3 increase in PM2.5 is associated with a 1.61% increase in the risk of hospitalization for patients with COPD in the United States (17) and 0.82% in Beijing (18). In a meta-analysis, Chen et al. summarized the effect of air pollution exposure on COPD mortality and showed that a 10 μg/m3 increase in PM2.5 was associated with a 1.11% increase in the risk of COPD-related death (19).
Chronic exposure to air pollution can lead to oxidative stress and inflammatory responses in the body. Air pollutants may act directly as oxidants or generate free radicals, causing oxidative stress (20). The glycerolphospholipid metabolism found in this study may be associated with this oxidative stress response. Air pollutants activate phospholipase A2, which hydrolyzes phospholipids in the cell membrane, thereby disrupting the alveolar structure (21, 22). This result is consistent with the results of the study by Nassan et al. (23). In addition to glycerolphospholipids metabolism, we found that PM2.5 exposure was associated with bile acid metabolism. An intermediate in the biosynthesis pathway of bile acid, 27-Hydroxycholesterol (27-OHC), was significantly enhanced in the lung tissues of patients with COPD. Meanwhile, amino acid metabolism, including alanine, aspartate, glutamate, arginine, and proline metabolism and arginine biosynthesis were also affected by PM2.5 exposure. Similarly, a study in 2018 showed elevated expression of amino acid metabolites in the blood of participants from high pollution exposure areas close to roadways, including arginine, histidine, γ-linolenic acid, and hypoxanthine (24). Meanwhile, a 2021 analysis of the effects of air pollution exposure on amino acid metabolites in the plasma of participants using a lagged effects model showed that exposure to PM2.5 was significantly and positively associated with elevated asparagine and glutamine over a 24-h period (25). These results suggest that amino acid metabolism plays an important role in PM2.5 pathogenesis.
On performing logistic regression of metabolites screened for association with PM2.5 exposure and AECOPD in the next 3 months, we found that arginine and GCDCA were positively associated with AECOPD in controlling sex, age, BMI, and smoking history, while ROC curves indicated that arginine better predicted AECOPD. This is similar to the findings of a previous study, which found that arginine expression was elevated in the plasma of patients with COPD compared with that in the healthy population and was further elevated in AECOPD (26). Meanwhile, Grasemann et al. showed that the arginine content in sputum was negatively correlated with FEV1 (27). Arginine is a semi-essential amino acid that is an important substrate for enzymes such as nitric oxide synthase (NOS) and arginase (28). Arginase converts arginine to ornithine and urea, while NOS converts arginine to citrulline with the production of NO. Exhaled NO is associated with airway inflammation, and ornithine is a precursor of proline and polyamines (e.g., putrescine, spermidine, and spermidine), which promote collagen production and cell proliferation (29, 30). In addition, asymmetric dimethylarginine (ADMA) is an endogenous competitive inhibitor of NOS, produced by arginine (31). ADMA is evolving as a marker of cardiovascular risk and has been studied in allergic asthma models (32), obesity-induced asthma studies (33, 34), obstructive sleep apnea syndrome studies, and COPD studies (35). Therefore, an increase in arginine concentration will lead to an increase in the expression of NOS and ADMA, which has a potential effect on airway inflammation and airway remodeling.
The present study has some limitations. First, the PM2.5 exposure data obtained in this study were from atmospheric monitoring stations, and no indoor air pollution levels were detected, which is not a good representation of the true PM2.5 exposure levels of patients. In addition, this study only explored the effects of PM2.5 on COPD patients and did not investigate the effects of other pollutants on metabolites, which has some limitations. Second, we did not consider indicators related to the patients' diet, exercise, sleep, and region that may affect metabolism. Third, the information about AECOPD was obtained from the patient's memories, and the questionnaire and blood samples were not tested when the patients were in acute exacerbation. In the future study, patients will be evaluated for acute exacerbation, so as to determine the impact of PM on AECOPD. Moreover, this study had a small sample size, restricting the generalizability of our findings. Further large sample studies are needed to detect metabolites in sputum or bronchoalveolar lavage fluid and conduct related mechanism studies to further explore the effect of air pollution on metabolites and the mechanism of AECOPD.
5. Conclusions
In summary, this study showed that PM2.5 exposure can lead to changes in multiple metabolic pathways that contribute to the development of AECOPD using metabolomic assays in plasma from patients with COPD subjected to high and low exposure levels. Our results suggest that arginine is a bridge between PM2.5 exposure and AECOPD; future studies can further explore the mechanism of elevated arginine expression caused by PM2.5 exposure and the correlation between arginine and AECOPD. Simultaneously, reducing PM2.5 concentration and going out in polluted weather are essential measures to prevent AECOPD.
Data availability statement
The raw data supporting the conclusions of this article will be made available by the authors, without undue reservation.
Ethics statement
The studies involving human participants were reviewed and approved by the Ethics Committee of the China-Japan Friendship Hospital. The patients/participants provided their written informed consent to participate in this study.
Author contributions
The study was proposed and the manuscript was revised by TiY. Material preparation and data collection were performed by TaY, HN, XL, and RD. Data analysis was performed by TaY, HW, FD, and QH. The first draft of the manuscript was written by TaY and HW. All authors commented on subsequent versions of the manuscript, read, approved the final manuscript, and contributed to the study conception and design.
Funding
This work was supported by National Key Research and Development Program of China (2022YFC3702704), CAMS Institute of Respiratory Medicine Grant for Young Scholars (2023-ZF-14), China-Japan Friendship Hospital Crosswise Tasks (2019-2-QN-74), and National Natural Science Foundation of China (81970043 and 82270038). The sponsors had no role in the design of the study, the collection and analysis of the data, or the preparation of the manuscript.
Acknowledgments
The authors thank the patients who enrolled in this study, the physicians, nurses, and secretaries who have taken part in this study for their important contributions.
Conflict of interest
The authors declare that the research was conducted in the absence of any commercial or financial relationships that could be construed as a potential conflict of interest.
Publisher's note
All claims expressed in this article are solely those of the authors and do not necessarily represent those of their affiliated organizations, or those of the publisher, the editors and the reviewers. Any product that may be evaluated in this article, or claim that may be made by its manufacturer, is not guaranteed or endorsed by the publisher.
Supplementary material
The Supplementary Material for this article can be found online at: https://www.frontiersin.org/articles/10.3389/fpubh.2023.1069906/full#supplementary-material
References
1. Vogelmeier CF, Criner GJ, Martinez FJ, Anzueto A, Barnes PJ, Bourbeau J, et al. Global strategy for the diagnosis, management, and prevention of chronic obstructive lung disease 2017 report. Gold executive summary. Am J Respir Crit Care Med. (2017) 195:557–82. doi: 10.1164/rccm.201701-0218PP
2. GBD 2017 Disease and Injury Incidence and Prevalence Collaborators. Global, regional, and national incidence, prevalence, and years lived with disability for 354 diseases and injuries for 195 countries and territories, 1990–2017: a systematic analysis for the global burden of disease study 2017. Lancet. (2018) 392:1789–858. doi: 10.1016/S0140-6736(18)32279-7
3. GBD 2017 Causes of Death Collaborators. Global, regional, and national age-sex-specific mortality for 282 causes of death in 195 countries and territories, 1980–2017: A systematic analysis for the global burden of disease study 2017. Lancet. (2018) 392:1736–88. doi: 10.1016/S0140-6736(18)32203-7
4. GBD 2017 DALYs and HALE collaborators. Global, regional, and national disability-adjusted life-years. (DALYs) for 359 diseases and injuries and healthy life expectancy. (HALE) for 195 countries and territories, 1990–2017: a systematic analysis for the global burden of disease study 2017. Lancet. (2018) 392:1859–922. doi: 10.1016/S0140-6736(18)32335-3
5. Gao N, Xu W, Ji J, Yang Y, Wang ST, Wang J, et al. Lung function and systemic inflammation associated with short-term air pollution exposure in chronic obstructive pulmonary disease patients in Beijing, China. Environ Health. (2020) 19:12. doi: 10.1186/s12940-020-0568-1
6. Sun Q, Liu C, Chen R, Wang C, Li J, Sun J, et al. Association of fine particulate matter on acute exacerbation of chronic obstructive pulmonary disease in Yancheng, China. Sci Total Environ. (2019) 650:1665–70. doi: 10.1016/j.scitotenv.2018.09.278
7. Li MH, Fan LC, Mao B, Yang JW, Choi AMK, Cao WJ, et al. Short-term exposure to ambient fine particulate matter increases hospitalizations and mortality in COPD: a systematic review and meta-analysis. Chest. (2016) 149:447–58. doi: 10.1378/chest.15-0513
8. Huang S, Garshick E, Vieira CLZ, Grady ST, Schwartz JD, Coull BA, et al. Short-term exposures to particulate matter gamma radiation activities and biomarkers of systemic inflammation and endothelial activation in COPD patients. Environ Res. (2020) 180:108841. doi: 10.1016/j.envres.2019.108841
9. Bowler RP, Jacobson S, Cruickshank C, Hughes GJ, Siska C, Ory DS, et al. Plasma sphingolipids associated with chronic obstructive pulmonary disease phenotypes. Am J Respir Crit Care Med. (2015) 191:275–84. doi: 10.1164/rccm.201410-1771OC
10. Wu G, Brown J, Zamora ML, Miller A, Satterfield MC, Meininger CJ, et al. Adverse organogenesis and predisposed long-term metabolic syndrome from prenatal exposure to fine particulate matter. Proc Natl Acad Sci U S A. (2019) 116:11590–5. doi: 10.1073/pnas.1902925116
11. Sun Q, Zhang G, Chen R, Li R, Wang H, Jiang A, et al. Central IKK2 inhibition ameliorates air pollution-mediated hepatic glucose and lipid metabolism dysfunction in mice with Type II diabetes. Toxicol Sci. (2018) 164:240–9. doi: 10.1093/toxsci/kfy079
12. Breitner S, Schneider A, Devlin RB, Ward-Caviness CK, Diaz-Sanchez D, Neas LM, et al. Associations among plasma metabolite levels and short-term exposure to PM2.5 and ozone in a cardiac catheterization cohort. Environ Int. (2016) 97:76–84. doi: 10.1016/j.envint.2016.10.012
13. Hu W, Jia Y, Kang Q, Peng H, Ma H, Zhang S, et al. Screening of house dust from Chinese homes for chemicals with liver X receptors binding activities and characterization of atherosclerotic activity using an in vitro macrophage cell line and ApoE–/– mice. Environ Health Perspect. (2019) 127:117003. doi: 10.1289/EHP5039
14. Yamaguchi Y, Zampino M, Moaddel R, Chen TK, Tian Q, Ferrucci L, et al. Plasma metabolites associated with chronic kidney disease and renal function in adults from the Baltimore longitudinal study of aging. Metabolomics. (2021) 17:9. doi: 10.1007/s11306-020-01762-3
15. Yang T, Chen R, Gu X, Xu J, Yang L, Zhao J, et al. Association of fine particulate matter air pollution and its constituents with lung function: the china pulmonary health study. Environ Int. (2021) 156:106707. doi: 10.1016/j.envint.2021.106707
16. Liu S, Jørgensen JT, Ljungman P, Pershagen G, Bellander T, Leander K, et al. Long-term exposure to low-level air pollution and incidence of chronic obstructive pulmonary disease: the ELAPSE project. Environ Int. (2021) 146:106267. doi: 10.1016/j.envint.2020.106267
17. Dominici F, Peng RD, Bell ML, Pham L, McDermott A, Zeger SL, et al. Fine particulate air pollution and hospital admission for cardiovascular and respiratory diseases. JAMA. (2006) 295:1127–34. doi: 10.1001/jama.295.10.1127
18. Gao N, Li C, Ji J, Yang Y, Wang S, Tian X, et al. Short-term effects of ambient air pollution on chronic obstructive pulmonary disease admissions in Beijing, China. (2013–2017). Int J Chron Obstruct Pulmon Dis. (2019) 14:297–309. doi: 10.2147/COPD.S188900
19. Chen J, Hoek G. Long-term exposure to PM and all-cause and cause-specific mortality: a systematic review and meta-analysis. Environ Int. (2020) 143:105974. doi: 10.1016/j.envint.2020.105974
20. Niemann B, Rohrbach S, Miller MR, Newby DE, Fuster V, Kovacic JC. Oxidative stress and cardiovascular risk: Obesity, diabetes, smoking, and pollution: Part 3 of a 3-Part Series. J Am Coll Cardiol. (2017) 70:230–51. doi: 10.1016/j.jacc.2017.05.043
21. Anthonymuthu TS, Kenny EM, Lamade AM, Kagan VE, Bayir H. Oxidized phospholipid signaling in traumatic brain injury. Free Radic Biol Med. (2018) 124:493–503. doi: 10.1016/j.freeradbiomed.2018.06.031
22. Sato H, Taketomi Y, Murakami M. Metabolic regulation by secreted phospholipase A2. Inflamm Regen. (2016) 36:7. doi: 10.1186/s41232-016-0012-7
23. Nassan FL, Kelly RS, Kosheleva A, Koutrakis P, Vokonas PS, Lasky-Su JA, et al. Metabolomic signatures of the long-term exposure to air pollution and temperature. Environ Health. (2021) 20:3. doi: 10.1186/s12940-020-00683-x
24. Liang D, Moutinho JL, Golan R, Yu T, Ladva CN, Niedzwiecki M, et al. Use of high-resolution metabolomics for the identification of metabolic signals associated with traffic-related air pollution. Environ Int. (2018) 120:145–54. doi: 10.1016/j.envint.2018.07.044
25. Hu X, Yan M, He L, Qiu X, Zhang J, Zhang Y, et al. Associations between time-weighted personal air pollution exposure and amino acid metabolism in healthy adults. Environ Int. (2021) 156:106623. doi: 10.1016/j.envint.2021.106623
26. Ruzsics I, Nagy L, Keki S, Sarosi V, Illes B, Illes Z, et al. L-arginine pathway in COPD patients with acute exacerbation: a new potential biomarker. COPD. (2016) 13:139–45. doi: 10.3109/15412555.2015.1045973
27. Grasemann H, Schwiertz R, Matthiesen S, Racké K, Ratjen F. Increased arginase activity in cystic fibrosis airways. Am J Respir Crit Care Med. (2005) 172:1523–8. doi: 10.1164/rccm.200502-253OC
28. King NE, Rothenberg ME, Zimmermann N. Arginine in asthma and lung inflammation. J Nutr. (2004) 134(10) Suppl:2830S−286S; discussion 2853S. doi: 10.1093/jn/134.10.2830S
29. Morris SM, Jr. Regulation of enzymes of the urea cycle and arginine metabolism. Annu Rev Nutr. (2002) 22:87–105. doi: 10.1146/annurev.nutr.22.110801.140547
30. Iyer R, Jenkinson CP, Vockley JG, Kern RM, Grody WW, Cederbaum S. The human arginases and arginase deficiency. J Inherit Metab Dis. (1998) 21(Suppl 1):86–100. doi: 10.1023/a:1005313809037
31. Böger RH. Asymmetric dimethylarginine, an endogenous inhibitor of nitric oxide synthase, explains the “L-arginine paradox” and acts as a novel cardiovascular risk factor. J Nutr. (2004) 134(Suppl:28) 42S−287S. doi: 10.1093/jn/134.10.2842S
32. Ahmad T, Mabalirajan U, Ghosh B, Agrawal A. Altered asymmetric dimethyl arginine metabolism in allergically inflamed mouse lungs. Am J Respir Cell Mol Biol. (2010) 42:3–8. doi: 10.1165/rcmb.2009-0137RC
33. Holguin F. Arginine and nitric oxide pathways in obesity-associated asthma. J Allergy. (2013) 2013:714595. doi: 10.1155/2013/714595
34. Holguin F, Comhair SA, Hazen SL, Powers RW, Khatri SS, Bleecker ER, et al. An association between L-arginine/asymmetric dimethyl arginine balance, obesity, and the age of asthma onset phenotype. Am J Respir Crit Care Med. (2013) 187:153–9. doi: 10.1164/rccm.201207-1270OC
Keywords: air pollution, chronic obstructive pulmonary disease, metabolomics, PM2.5, arginine
Citation: Yu T, Wu H, Huang Q, Dong F, Li X, Zhang Y, Duan R, Niu H and Yang T (2023) Outdoor particulate matter exposure affects metabolome in chronic obstructive pulmonary disease: Preliminary study. Front. Public Health 11:1069906. doi: 10.3389/fpubh.2023.1069906
Received: 14 October 2022; Accepted: 03 March 2023;
Published: 21 March 2023.
Edited by:
Chih-Da Wu, National Cheng Kung University, TaiwanReviewed by:
Ting Zhou, Wuhan University of Science and Technology, ChinaSasho Stoleski, Saints Cyril and Methodius University of Skopje, North Macedonia
Vladimir Koblizek, University Hospital Hradec Kralove, Czechia
Maria Gabriella Verso, University of Palermo, Italy
Copyright © 2023 Yu, Wu, Huang, Dong, Li, Zhang, Duan, Niu and Yang. This is an open-access article distributed under the terms of the Creative Commons Attribution License (CC BY). The use, distribution or reproduction in other forums is permitted, provided the original author(s) and the copyright owner(s) are credited and that the original publication in this journal is cited, in accordance with accepted academic practice. No use, distribution or reproduction is permitted which does not comply with these terms.
*Correspondence: Ting Yang, enJ5eXlhbmd0aW5nQDE2My5jb20=; Hongtao Niu, b3hob25ndGFvQDE2My5jb20=
†These authors have contributed equally to this work