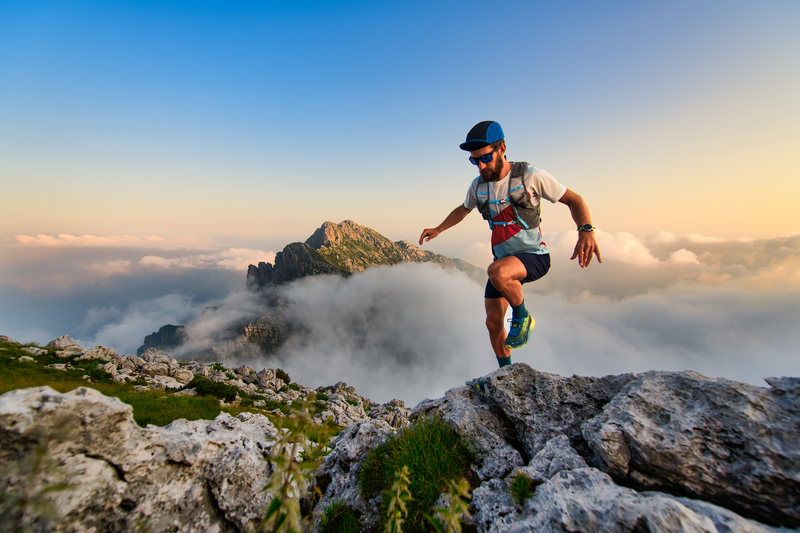
94% of researchers rate our articles as excellent or good
Learn more about the work of our research integrity team to safeguard the quality of each article we publish.
Find out more
ORIGINAL RESEARCH article
Front. Public Health , 21 February 2023
Sec. Public Health Education and Promotion
Volume 11 - 2023 | https://doi.org/10.3389/fpubh.2023.1043485
This article is part of the Research Topic COVID-19: Vaccine and Vaccination Communication View all 28 articles
Objective: To investigate the relationship among information processing, risk/benefit perception and the COVID-19 vaccination intention of OHCs users with the heuristic-systematic model (HSM).
Methods: This study conducted a cross-sectional questionnaire via an online survey among Chinese adults. A structural equation model (SEM) was used to examine the research hypotheses.
Results: Systematic information processing positively influenced benefit perception, and heuristic information processing positively influenced risk perception. Benefit perception had a significant positive effect on users' vaccination intention. Risk perception had a negative impact on vaccination intention. Findings revealed that differences in information processing methods affect users' perceptions of risk and benefit, which decide their vaccination intention.
Conclusion: Online health communities can provide more systematic cues and users should process information systematically to increase their perceived benefits, consequently increase their willingness to receive COVID-19 vaccine.
The COVID-19 pandemic has caused a major health crisis in humans (1). As of 13 Sep 2022, the World Health Organization has reported more than 600 million cumulative confirmed cases of COVID-19 and more than 6 million cumulative deaths (2). Ensuring that all people are vaccinated will help control the spread of COVID-19 (3) and thus protect the public from COVID-19 (4).
In China, the government legislated an emergency authorization of COVID-19 vaccine for people at high risk in June 2020 (5), and subsequently approved COVID-19 vaccines for public use in December 2020 (5, 6). As of March 2020, China has passed through its peak of the pandemic. However, China is still experiencing a small increase in cases due to the impact of COVID-19 mutations and the importation of cases from abroad. Vaccination and testing are the main vaccination policies in China. As of July 22, 2022, the first full-round vaccination rate reached 89.7% and the booster vaccination rate was 71.7% (7). Although overall the vaccination rate is relatively high. However, the booster vaccination rate is much lower compared to the first full vaccination rate. In order to strengthen the protective efficacy of the vaccine against COVID-19 mutations, ensuring the booster vaccination rate is an effective measure. In this context, continued attention to the factors influencing the intention to vaccinate against COVID-19 can inform the maintenance of the intention to vaccinate against COVID-19, the improvement of booster vaccination rates, and the development of vaccination policies.
Previous studies have explored the effect of perceived risk/benefit on intention to vaccinate for COVID-19 (8–10). For example, a study by Liora Shmueli showed that perceived benefit was the most important predictor of acceptance of the COVID-19 vaccine (11). Another study showed a strong correlation between risk perception and vaccine acceptance (12). And vaccine-related information affects users' perceptions of the risk and benefit of vaccines. Users' processing of vaccine-related information shapes their perception of vaccines (13, 14). Some studies have found that having the correct knowledge is directly related to a higher perception of risk in the older population (15). Knowledge about vaccines was associated with how individuals perceived the relevant risks and benefits of those aspects of the vaccine (16). Information related to the efficacy and safety of vaccines critically influences the acceptance of COVID-19 vaccines (4). Knowledge formation comes from information processing. However, there are no studies that have explored the effects of perceived risks/benefits on the willingness to vaccinate for COVID-19 from an information processing perspective. Processing of vaccine-related information is a key factor in the formation of people's perceived attitudes. As such, further research is necessary to determine how information processing affects risk/benefit perception associated with COVID-19 vaccination intention.
As access to information through the Internet has the advantage of being quick and convenient, online health communities (OHCs) have become one of the most important channels through which people obtain information during a pandemic (17–19). People used OHCs to learn about COVID-19 and seek information about available vaccines (20, 21). OHCs are online interactive platforms with health-related features, such as online consultation, health information exchange and experience sharing, which provide users with information and emotion support (19). Users can also benefit from OHCs by adopting healthier behaviors (22).
Therefore, in the context of the rapid development of Internet medicine and the normalization of COVID-19 prevention and control. Our study explores the impact of users' vaccine-related information processing in OHCs and their risk/benefit perception of vaccines, consequent on COVID-19 vaccination intention. Our study findings may help relevant health authorities to take more effective measures to increase vaccination rates, maintain COVID-19 vaccination intentions among Chinese residents, and provide a reference for the development of Internet healthcare.
Wilson's information behavior theory suggests that users engage in information seeking through formal or informal means in order to satisfy their information needs, and then process and use the information (23). This process is influenced by activating mechanisms (e.g., stress/coping theory, risk/reward theory) and intervening variables (e.g., psychological, demographic, role-related or interpersonal). This model has been widely used in studies related to user information behavior (24, 25). Our study examines the factors associated with information behavior and the effect of perceived risk/benefit on willingness to vaccinate.
The heuristic-systematic model (HSM) of information processing includes two types of information processing: heuristic information processing and systematic information processing (26), where systematic information processing involves a more comprehensive analysis and understanding of information. On the other hand, heuristic information processing requires only simple decision rules such as intuition and experience to form judgments (27). The HSM has been widely used to explain people's attitude or behavior responses to information. The model considers information processing as a precursor to attitude formation or change, and therefore proposes two basic information processing patterns that people may adopt after acquiring information and assessing and judging risks or things.
Information needs are also known as information insufficiency, where people lack sufficient information to make informed decisions (28). Information needs arise when the information that people want to know is more than the knowledge they have. During the COVID-19 pandemic, people need sufficient information to make decisions about whether to receive the COVID-19 vaccine (29). Previous research found that users' demand for information on the prevention of COVID-19 accounted for 36.11% in OHCs (21), which shows their great concerns and information needs about COVID-19 prevention. Some studies have suggested that information needs predict information seeking (8, 30). We assume that information needs about the COVID-19 vaccine positively influence information seeking (H1a). People satisfy their information needs by seeking information (31). In addition, if people do not have enough information to cope with emergencies, the more intense their information needs are, and the more actively they will use systematic processing (8, 32). Conversely, the heuristic processing will become more active (13, 33). Therefore, it is assumed that information needs positively influence systematic information processing (H1b) and negatively influence heuristic information processing (H1c):
Hypothesis 1a (H1a). Information needs positively influence information seeking.
Hypothesis 1b (H1b). Information needs negatively influence heuristic information processing.
Hypothesis 1c (H1c). Information needs positively influence systematic information processing.
Information seeking is a dynamic process of acquiring information and knowledge (34). People seek information through various approaches to obtain reliable information (35–37). OHCs provide a platform for people to seek and obtain information. Kahlor thinks information seeking is the precondition for information processing (38). The research of Guo found that information seeking positively affects systematic information processing (26). Information seeking intention is positively correlated with systematic processing and heuristic processing (39). We proposed that when people actively seek information about COVID-19 vaccination, both heuristic information processing (H2a) and systematic information processing will improve (H2b):
Hypothesis 2a (H2a). Information seeking positively affects heuristic information processing.
Hypothesis 2b (H2b). Information seeking positively affects systematic information processing.
The heuristic-systematic information processing model states that people use one or two types of information processing to help them evaluate information to make decisions (13). Most people will only make decisions based on superficial information cues (40). Systematic information processing requires more comprehensive cognition and analysis by individuals (13, 41), and the process of systematic information processing consumes more time and effort on the part of the individual. Therefore, when people carry out systematic information processing, more reliable and effective information can be obtained (26). Trumbo demonstrated that heuristic information processing negatively affects risk perception, while systematic information processing positively affects risk perception in his study about cancer (13). Smerecnik et al. (42) used an adapted HSM scale to test the relationship between information processing and risk perception about hypertension. In a study about the risk associated with the companies of a petrochemical complex, systematic processing has a direct, positive, and significant influence on risk perception (43). For benefit perception, both heuristic and systematic information processing are linked to higher benefits of using of nanotechnology (44). However, few researchers have focused on the relationships between information processing and risk/benefit perception in the context of online health communities. Therefore, we established the following hypotheses:
Hypothesis 3a (H3a). Heuristic information processing has a negative effect on risk perception.
Hypothesis 3b (H3b). Heuristic information processing has a positively impact on benefit perception.
Hypothesis 4a (H4a). Systematic information processing positively affects risk perception.
Hypothesis 4b (H4b). Systematic information processing positively affects benefit perception.
With the experiment and implementation of the COVID-19 vaccine, the side effects and adverse effects of the vaccination began to appear (45), which increased people's risk perception of the COVID-19 vaccine. Kelly defined risk perception as potential adverse events or side effects from taking the drug (46). For example, myocarditis/pericarditis was a rare complication of COVID-19 mRNA vaccinations, especially in young and adolescent males (47). The lack of effectiveness of COVID-19 vaccines may also threaten people's life, thus people perceive the risk of vaccination, which will lead to vaccine hesitancy and anti-vaccination movements (48). Some scholars have found that risk perception negatively affects behavioral intentions (8, 49). This means that when people are aware of the potential risks of vaccination, they may refuse to receive it. Therefore, we proposed hypothesis H5.
Contrary to risk perception, benefit perception is considered as the perception of the benefits of vaccination, such as disease prevention and self-protection (10, 50). Wong et al. (9) and Yu et al. (10) proved the positive impact of benefit perception on vaccination intention with the health belief model. However, it has not been studied in the context of online health communities. We believed that the perceived benefit of COVID-19 vaccination information will positively affect users' willingness to vaccinate (H6):
Hypothesis 5 (H5). Risk perception will weaken OHCs users' intention to receive the COVID-19 vaccine.
Hypothesis 6 (H6). Benefit perception will increase OHCs users' intention to receive the COVID-19 vaccine.
In accordance with our research hypotheses above, a new model was constructed by integrating HSM with risk/benefit perception to examine the mechanisms influencing users' willingness to vaccinate against COVID-19 in OHCs. As shown in Figure 1, in which information needs and information seeking are antecedents of information processing, information processing is assumed to predict risk/benefit perception, and risk/benefit perception directly influences vaccination intention.
Figure 1. The conceptual research model. Hypothesis: H1–H6. “+” means positive effect, “–” means negative effect. The arrows point to the affected variables.
This study aims to investigate the relationship between users' information needs, information seeking, heuristic-systematic information processing, risk perception, benefit perception, and vaccination intention against COVID-19 in OHCs. We conducted an online survey via Questionnaire Star (https://www.wjx.cn/ accessed on 30 June 2021). The questionnaire includes two parts: the first part is sociodemographic characteristics, namely, gender, age, education level, occupation, income, and health status; the second part is the scale measurement part, using a five-point Likert scale from “strongly disagree (1)” to “strongly agree (5),” which was adapted from previous studies. We revised it into Chinese scale and pre-tested. According to the advice of the pre-test participants and the experts group, we revised some sentences and words, for example, we changed “never” into “rarely” in the heuristic information processing items. The final scale settings are shown in Table 1.
Before the formal survey, this study obtained ethical approval from the Institutional Review Board of the College of Life Sciences at Central South University (Reference No. 2021-1-23). We explained the purpose and significance of our research, and provided privacy protection to all participants. All participants agreed to join this study. The investigation was administered from 1 May 2021 to 15 June 2021.
A total of 525 participants completed the questionnaire. Then we excluded the respondents who had never used OHCs before by using the option “Never used an OHC.” By removing duplicates and anomalies to ensure the validity of the data, we ended up with 410 valid questionnaires. According to the minimum sample size requirement, it must be at least 10–15 times the number of scale items (53), so our effective sample size was reasonable. Valid participants were older than 18. Among them, 64.1% are women, 89.7% of the participants have a bachelor's degree or above, and 78.5% of users are under 30 years old. Sociodemographic characteristics are presented in Table 2.
We used the structural equation model (SEM) to test our theoretical model. SEM is a multivariate statistical technique for testing hypotheses about the influences of sets of variables on other variables (54). It can measure the interrelation of latent variables that are not directly observable and is widely used in social sciences. Latent variables are measured by their corresponding observation variables, namely, scale items. In this research, latent variables include information needs (IN), information seeking (IS), heuristic information processing (HIP), systematic information processing (SIP), risk perception (RP), benefit perception (BP) and vaccination intention (VI). Because there are many latent variables and their relationship is complex, SEM is selected for verification. SEM incorporates two analytical procedures (55). Firstly, we conducted a confirmatory factor analysis (CFA), which evaluates the measurement component of a theoretical model. After, we carried out a path analysis, which evaluates the relationship between latent variables.
We used SPSS 26.0 and AMOS 23.0 of IBM company (Chicagao, America) to analyze the reliability and validity of the measurement model. The Cronbach Alpha coefficient (56) of the scale was 0.897, and the Cronbach Alpha coefficient of each latent variable was >0.7, indicating that the internal stability and consistent reliability of the questionnaire were good.
To further examine the convergent validity of the questionnaire, a confirmatory factor analysis was conducted by AMOS23.0 to obtain values of the average variance extracted (AVE) and standardized loadings of items. As shown in Table 3, all the standardized loadings of items were >0.6 (57). Values of composite reliability (CR) were between 0.796 and 0.870, which were higher than 0.70, indicating that the constructs have good convergent validity (54). The AVEs of all structures were greater than the benchmark value of 0.5, indicating that the overall model is valid (58).
Then we tested the model's fit indicators by AMOS23.0 (55), which are showed as Table 4: the ratio of Chi-square to the degree of freedom (χ2/df) was 2.879, which was smaller than the desired threshold of 3.0. The values of the comparative fit index (CFI), incremental fit index (IFI), and Tucker-Lewis index (TLI) were 0.927, 0.928, and 0.911, respectively. Moreover, the root mean square error of approximation (RMSEA) value was 0.068, which was lower than 0.08. These figures reveal a good fit between the measurement model and the dataset.
The results of CFA ensure the reliability of our following analysis. By using AMOS 23.0 to set up the structural model, a path analysis was performed to test the relationships among the constructs in the model framework. The standard path coefficients (β) and p-value can be seen in Table 5. All the hypothesized relationships were supported, except H3a, H3b, and H4a. Information needs had a positive effect on information seeking (H1a: β = 0.66, p < 0.001) and systematic information processing (H1c: β = 0.42, p < 0.001). Hence, H1a and H1c were supported. The relationship between information needs and heuristic information processing was the opposite (H1b: β = −0.31, p < 0.001), so H1b was supported. Information seeking had a positive effect on heuristic information processing (H2a: β = 0.72, p < 0.001) and systematic information processing (H2b: β = 0.41, p < 0.001). Thus, H2a and H2c were supported.
The results show that heuristic information processing had a positive relationship with risk perception (H3a: β = 0.61, p < 0.001), since this was contrary to our hypothesis, and it had an insignificant negtive effect on the benefit perception (H3b: β = −0.02, p = 0.644 > 0.05). Thus, H3a and H3b were not supported. Systematic information processing had a positive effect on benefit perception (H4b: β = 0.73, p < 0.001), so H4b was supported. While systematic information processing to risk perception was insignificant (H4a: β = −0.06, p = 0.217 > 0.05), so H4a was not supported. Finally, risk perception had a negative impact on the vaccination willingness of OHCs users (H5: β = −0.08, p = 0.046 < 0.05), and benefit perception had a significant positive impact on the vaccination willingness of OHCs users (H6: β = 0.84, p < 0.001), indicating that H5 and H6 were supported. The model of final research results was as seen in Figure 2.
Figure 2. The model of final research results, including path coefficients (β), and explained variances (R2). For example, R2 = 0.44, means that the predictors of information seeking explain 44% of its variance.
Our research found a strong positive correlation between vaccine information needs and information seeking among users of OHCs. The COVID-19 pandemic has severely affected the everyday life of people around the world. Even though COVID-19 sometimes mutates, vaccines are still an effective means of prevention (59), and there is often uncertainty about people's attitudes toward emerging technologies (30, 60). When it comes to the COVID-19 vaccine, this manifests itself as concerns about the safety and efficacy of the vaccine (61). To reduce uncertainty, people require accurate and effective information, which leads to further product information seeking and information processing behaviors. Savolainen (62) thinks that information needs are the fundamental factor that motivates people to identify and access information sources and the driver that stimulates them to continuously search for information. Our study adds to the empirical evidence, in which vaccine information seeking behavior was largely explained by information needs (R2 = 0.44). A study by Zhou (63) found that pandemic risk stimulates information needs and thus positively influences information seeking behavior. This point is consistent with the findings of our study.
The relationship between vaccine information needs and information processing styles was also explored in this study. The need for vaccine-related information positively influenced systematic information processing, and negatively influenced heuristic information processing. According to Griffin et al. (32), people are more likely to process information systematically when they have a greater desire for information. Hubner and Hovick (64) proved that information insufficiency is positively associated with systematic processing. Our study implies that Griffin's model also applies to users' vaccine-related information processing within the online health community. On the other hand, our work reflects that users prioritize risk information when they look for vaccine-related information. Users need comprehensive information to determine vaccination risks and to make vaccination decisions. In this process, information seeking positively correlated with both heuristic and systematic processing. This result suggests that information seeking behavior further facilitates information processing behavior, which is the same as Zhu et al. (39)'s research findings.
The focus of this study was to investigate the relationship between information processing, risk/benefit perception, and vaccination intention. Heuristic information processing negatively influences vaccination intention of OHCs users by positively affecting their risk perception, and systematic information processing positively influences vaccination intention of OHCs users by positively affecting their benefit perception. Fast, intuition-based information processing is more likely to elicit users' perception of risk. This feature may be influenced by a large amount of information on vaccine side effects available on the Internet. When people employ heuristic information processing, they verify information less from multiple sources. In addition, because heuristic information processing makes it easier to make quick decisions, it is more difficult for them to spend time searching for more comprehensive information. On online communication platforms, people tend to spread the side effects of vaccines more often than the positive effects of vaccines (65). Concerns about the side effects are a barrier to achieving high vaccination rates (66, 67), and the perceived risk of vaccines reduces the willingness of people to receive COVID-19 vaccines.
A comprehensive and systematic approach to information processing helps people to perceive the benefits of vaccines and thus promotes their intention to receive vaccinations, and Jing et al. found that parents were more likely to accept childhood vaccinations when they systematically described and processed information (68). Jing suggested that systematic information processing could lead parents to adopt an “objective” or “balanced” approach, which is compatible with their perceived benefits of childhood vaccination, thus promoting vaccination (68). Our findings further establish the relationship between systematic information processing, perceived benefits, and vaccination intentions. Another study concluded that active participation in information behavior helps reduce the public's uncertainty and mitigate risk about COVID-19 pandemic (69). In our study, users more actively involved in information dissemination were more likely to have comprehensive exposure to vaccine information, adopt systematic information processing, and enhance perceived benefits, thus increasing their vaccination intention.
Our study also found that systematic information processing failed to positively influence perceived risk. In contrast, a previous study showed that systematic processing positively influenced perceived risk and thus protective behavioral intentions (49), which is inconsistent with our findings. The possible reason for this is that the information processing in their study was for the vaccine scandal, whereas the participants in our study were exposed to comprehensive vaccine information. Participants who used more systematic processing may have been more likely to use authoritative, official information, whereas authoritative information in China showed more benefits of vaccination. The failure of heuristic processing to predict benefit perception may also be related to the rapidity of the information processing subject. The way decisions are made are based on surface information cues, and the complexity of internet information.
In the context of the global COVID-19 pandemic, we combined information behavior theory and the heuristic-systematic information processing model to study the COVID-19 vaccination intention of OHC users. Compared with previous studies, we innovatively explored the relationship between cognitive processing and risk/benefit perceptions from two pathways (heuristic or systematic processing). It also provides new theoretical guidance for the application of HSM. Also our study found that the online health community, an information platform, plays a significant role in disseminating information and improving users' vaccination confidence during the pandemic. It provides a basis in the literature for using the network platform to promote public health.
There are several practical applications of our research to increase people's intention to vaccinate against COVID-19. Firstly, the government administration should strengthen the regulation of information about the COVID-19 pandemic and propagandize knowledge of COVID-19 vaccine among the public. Secondly, managers of online health communities should provide users with systematic and positive clues, such as more informative and effective information (e.g., a list of vaccination places, time or price). They are also recommended to expand the retrieval systems of OHCs so that searching is as easy as possible for users. In addition, both government administration and OHCs managers should enhance the dissemination of benefit-related information. Appropriate risk information is needed but must be truthful, as it can stimulate informative behavior and thus promote vaccination intentions. Finally, users should be more proactive in adopting a systematic approach to information processing and making comprehensive judgments about information, for example, by comparing information from different sources (experts, other users or third-party organizations).
The study confirmed the adaptability of HSM in the background of online health communities and the COVID-19 pandemic, where information needs and information seeking remain the antecedents of information processing. Differences in how OHCs users process information cause differences in perception, with heuristic information processing leading to risk perception and systematic information processing leading to benefit perception. In contrast, risk perception and benefit perception directly influence OHCs users' willingness to vaccinate against COVID-19. Although the negative effect of risk perception on vaccination intention is small but present, the positive effect of benefit perception on vaccination intention is much more significant.
There are some limitations of this study. First, the study was only on Chinese online health community users. Therefore, our findings lack generalizability. Considering the differences between China and foreign countries in terms of COVID-19 pandemic prevention policies and internet management, the impact of online health communities in different countries can be further studied in the future. Second, our quantification of user information behavior through scales may be subjective. More objective measurement tools (i.e., eye-tracking) or methods (i.e., in-depth interview method) could be used further in future studies. Third, cross-sectional studies are limited to confirming the relationship between variables at a particular time period. As vaccination intentions of OHCs users may vary with the development of the COVID-19 pandemic and policies, longitudinal studies can be adopted in future studies to discover further changes in users' vaccination intentions against COVID-19. Finally, since our target population are people who frequently use online health communities and can agree to participate in our survey, some people will refuse to participate in the survey due to disease privacy concerns. Although our sample size meets the minimum requirements for SEM, it is still relatively small. Future studies can expand the sample size; additionally, studies might investigate populations not using online health communities to obtain more comprehensive conclusions.
The original contributions presented in the study are included in the article/supplementary material, further inquiries can be directed to the corresponding author.
The studies involving human participants were reviewed and approved by Institutional Review Board of College of Life Sciences, Central South University. The patients/participants provided their written informed consent to participate in this study. Written informed consent was obtained from the individual(s) for the publication of any potentially identifiable images or data included in this article.
HL and DH: conceptualization and methodology. HL, LG, CW, and YuG: software, validation, investigation, and formal analysis. YiG and MY: resources and data curation. HL and LG: writing—original draft preparation. HJ, XW, and DH: writing—review and editing. DH: visualization, supervision, project administration, and funding acquisition. All authors have read and agreed to the published version of the manuscript.
This research was funded by the National Social Science Fund of China (Grant No: 20BTQ081), Key International Cooperation Projects of Hunan Province of China (Grant No: 2021WK2003), and the Project of Theory, Practice and Popularization of Scientific and Technological Novelty Search by Shenzhen Health Development Research and Data Management Center (Grant No: H202111120250001).
The authors declare that the research was conducted in the absence of any commercial or financial relationships that could be construed as a potential conflict of interest.
All claims expressed in this article are solely those of the authors and do not necessarily represent those of their affiliated organizations, or those of the publisher, the editors and the reviewers. Any product that may be evaluated in this article, or claim that may be made by its manufacturer, is not guaranteed or endorsed by the publisher.
1. Abdulazeem HM, von Groote TC, Jayarajah U, Weerasekara I, Borges do Nascimento IJ, Cacic N, et al. Novel coronavirus infection (COVID-19) in humans: a scoping review and meta-analysis. J Clin Med. (2020) 9:941. doi: 10.3390/jcm9040941
2. World Health Organization. WHO Coronavirus (COVID-19) Dashboard. Geneva: WHO (2022). Available online at: https://covid19.who.int (accessed September 13, 2022).
3. Rahman IU, Ali N, Ijaz F, Afzal A, Abd-Allah EF. COVID-19: important considerations for developing and using a vaccine. Hum Vacc Immunotherap. (2021) 17:414–5. doi: 10.1080/21645515.2020.1781507
4. Laine C, Cotton D, Moyer DV. COVID-19 vaccine: promoting vaccine acceptance. Ann Intern Med. (2021) 174:252–3. doi: 10.7326/M20-8008
5. Joint COVID-19. Prevention and control mechanism of the State Council, China. In: Conditional Listing of New Coronavirus Vaccine. Wuhan: COVID-19 (2020). Available online at: http://www.scio.gov.cn/video/42600/42601/Document/1696292/1696292.htm (accessed October 21, 2022).
6. HCA Plan. The central people's government of the People's Republic of China. In: China's New Coronavirus Vaccine Approved for Marketing! 6 Important News!. Nashville: HCA Plan (2020). Available online at: http://www.gov.cn/fuwu/2020-12/31/content_5575768.htm (accessed October 21, 2022).
7. National Health Commission. National Health Commission of the People's Republic of China. Beijing: National Health Commission (2022). Available online at: http://www.nhc.gov.cn/xcs/s3574/202207/f920442a8a4845e7833ca8d7e7cd876e.shtml (accessed October 21, 2022).
8. Guo QZ, Yao NZ, Zhu WW. How consumers' perception and information processing affect their acceptance of genetically modified foods in China: a risk communication perspective. Food Res Int. (2020) 137:109518. doi: 10.1016/j.foodres.2020.109518
9. Wong LP, Alias H, Wong PF, Lee HY, AbuBakar S. The use of the health belief model to assess predictors of intent to receive the COVID-19 vaccine and willingness to pay. Hum Vaccin Immunother. (2020) 16:2204–14. doi: 10.1080/21645515.2020.1790279
10. Yu Y, Lau JTF, She R, Chen X, Li L, Li L, et al. Prevalence and associated factors of intention of COVID-19 vaccination among healthcare workers in China: application of the health belief model. Hum Vaccin Immunother. (2021) 17:2894–902. doi: 10.1080/21645515.2021.1909327
11. Shmueli L. Predicting intention to receive COVID-19 vaccine among the general population using the health belief model and the theory of planned behavior model. BMC Public Health. (2021) 21:804. doi: 10.1186/s12889-021-10816-7
12. Nusair MB, Arabyat R, Khasawneh R, Al-Azzam S, Nusir AT, Alhayek MY. Assessment of the relationship between COVID-19 risk perception and vaccine acceptance: a cross-sectional study in Jordan. Hum Vaccin Immunother. (2022) 18:2017734. doi: 10.1080/21645515.2021.2017734
13. Trumbo CW. Information processing and risk perception: an adaptation of the heuristic-systematic model. J Commun. (2002) 52:367–82. doi: 10.1111/j.1460-2466.2002.tb02550.x
14. Kahneman D, Tversky A. Prospect theory: an analysis of decision under risk. Econometrica. (1979) 47:263–91.
15. Gallè F, Sabella EA, Roma P, Ferracuti S, Da Molin G, Diella G, et al. Knowledge and lifestyle behaviors related to COVID-19 pandemic in people over 65 years old from Southern Italy. Int J Environ Res Public Health. (2021) 18:10872. doi: 10.3390/ijerph182010872
16. Robertson DA, Mohr KS, Barjaková M, Lunn PD, A. lack of perceived benefits and a gap in knowledge distinguish the vaccine hesitant from vaccine accepting during the COVID-19 pandemic. Psychol Med. (2021) 31:1–4. doi: 10.1017/S0033291721003743
17. Liu S, Yang L, Zhang C, Xiang YT, Liu Z, Hu S, et al. Online mental health services in China during the COVID-19 outbreak. Lancet Psychiatry. (2020) 7:17–8. doi: 10.1016/S2215-0366(20)30077-8
18. Bento AI, Nguyen T, Wing C, Lozano-Rojas F, Ahn YY, Simon K. Evidence from internet search data shows information-seeking responses to news of local COVID-19 cases. Proc Natl Acad Sci U S A. (2020) 117:11220–2. doi: 10.1073/pnas.2005335117
19. Lu Y. Automatic topic identification of health-related messages in online health community using text classification. Springerplus. (2013) 2:309. doi: 10.1186/2193-1801-2-309
20. Jung G, Jang SH. Understanding the role of ethnic online communities during the COVID-19 pandemic: a case study of Korean immigrant women's information-seeking behaviors. Asian J Soc Sci. (2022) 50:292–300. doi: 10.1016/j.ajss.2022.04.001
21. Wang J, Wang L, Xu J, Peng Y. Information needs mining of COVID-19 in Chinese online health communities. Big Data Res. (2021) 24:100193. doi: 10.1016/j.bdr.2021.100193
22. Li Y, Yan X. How could peers in online health community help improve health behavior. Int J Environ Res Public Health. (2020) 17:2995. doi: 10.3390/ijerph17092995
23. Wilson TD. Information behavior: an interdisciplinary perspective. Inf Process Manag. (1997) 33:551–72. doi: 10.1016/S0306-4573(97)00028-9
24. Xiaoli Hu Y, Shaofeng Z. Information processing in the “not-in-my-backyard” strategy: an empirical study of anti-nuclear behavioral responses. Hum Ecol Risk Assess Int J. (2020) 26:2266–87. doi: 10.1080/10807039.2019.1672138
25. Diwanji V, Reed A, Ferchaud A, Seibert J, Weinbrecht V, Sellers, N. Don't just watch, join in: exploring information behavior and copresence on Twitch. Comput Hum Behav. (2020) 105:106221. doi: 10.1016/j.chb.2019.106221
26. Chaiken S. Heuristic vs. systematic information processing and the use of source vs message cues in persuasion. J Person Soc Psychol. (1980) 39:752–66. doi: 10.1037/0022-3514.39.5.752
27. Kim J, Paek HJ. Information processing of genetically modified food messages under different motives: an adaptation of the multiple-motive heuristic-systematic model. Risk Anal. (2009) 29:1793–806. doi: 10.1111/j.1539-6924.2009.01324.x
28. Moore N. A model of social information need. J Inf Sci. (2002) 28:297–303. doi: 10.1177/016555150202800404
29. Lin Y, Hu Z, Zhao Q, Alias H, Danaee M, Wong LP. Understanding COVID-19 vaccine demand and hesitancy: a nationwide online survey in China. PLoS Negl Trop Dis. (2020) 14:e0008961. doi: 10.1371/journal.pntd.0008961
30. Zeng J, Wei JC, Zhao DT, Zhu WW, Gu JB. Information-seeking intentions of residents regarding the risks of nuclear power plant: an empirical study in China. Nat Hazards. (2017) 87:739–55. doi: 10.1007/s11069-017-2790-x
31. Wilson TD. Human information behavior. Inform Sci Int J Emerg Transdiscip. (2000) 3:49–55. doi: 10.1002/sdr.4260070210
32. Griffin RJ, Dunwoody S, Neuwirth K. Proposed model of the relationship of risk information seeking and processing to the development of preventive behaviors. Environ Res. (1999) 80:230–45. doi: 10.1006/enrs.1998.3940
33. Johnson BB. Testing and expanding a model of cognitive processing of risk information. Risk Anal. (2005) 25:631–50. doi: 10.1111/j.1539-6924.2005.00609.x
34. Kuhlthau CC. Inside the search process: information seeking from the user's perspective. J Am Soc Inform Sci. (1991) 42:361–71. doi: 10.1002/(SICI)1097-4571(199106)42:5<361::AID-ASI6>3.0.CO;2-#
35. Ter Huurne E, Gutteling J. Information needs and risk perception as predictors of risk information seeking. J Risk Res. (2008) 11:847–62. doi: 10.1080/13669870701875750
36. Che D, Hu DH. Developing the information-seeking behavior scale for undergraduates. J Data Inform Sci. (2013) 6:78–96.
37. Lu C, Xu W, Shen H, Zhu J, Wang KZ. MIMO channel information feedback using deep recurrent network. IEEE Commun Lett. (2019) 23:188–91. doi: 10.1109/LCOMM.2018.2882829
38. Kahlor LA, Yang ZJ, Liang MC. Risky politics: applying the planned risk information seeking model to the 2016 US presidential election. Mass Commun Soc. (2018) 21:697–719. doi: 10.1080/15205436.2018.1498900
39. Zhu WW, Wu TT, Liao CH. Impact of information processing on individuals' intentions toward reducing PM2.5: evidence from Hefei City, China. J Environ Plan Manag. (2022) 22:1–18. doi: 10.1080/09640568.2022.2036601
40. Smith SW, Hitt R, Russell J, Nazione S, Silk K, Atkin CK, et al. Risk belief and attitude formation from translated scientific messages about PFOA, an environmental risk associated with breast cancer. Health Commun. (2017) 32:279–87. doi: 10.1080/10410236.2016.1138350
41. Chaiken S, Liberman A, Eagly AH. Heuristic and systematic information processing within and beyond the persuasion context. In: Bargh JS, and Uleman JA, editors. Unintended Thought. New York, NY: The Guilford Press (1989). p. 212–52
42. Smerecnik CM, Mesters I, Candel MJ, De Vries H, De Vries NK. Risk perception and information processing: the development and validation of a questionnaire to assess self-reported information processing. Risk Anal. (2012) 32:54–66. doi: 10.1111/j.1539-6924.2011.01651.x
43. Tortosa-Edo V, Lopez-Navarro MA, Llorens-Monzonis J, Rodriguez-Artola RM. The antecedent role of personal environmental values in the relationships among trust in companies, information processing and risk perception. J Risk Res. (2014) 17:1019–35. doi: 10.1080/13669877.2013.841726
44. Kim J, Yeo SK, Brossard D, Scheufele DA, Xenos MA. Disentangling the influence of value predispositions and risk/benefit perceptions on support for nanotechnology among the American public. Risk Anal. (2014) 34:965–80. doi: 10.1111/risa.12141
45. Yigit M, Ozkaya-Parlakay A, Senel E. Evaluation of COVID-19 vaccine refusal in parents. Pediatr Infect Dis J. (2021) 40:134–6. doi: 10.1097/INF.0000000000003042
46. Kelly BJ, Rupert DJ, Aikin KJ, Sullivan HW, Johnson M, Bann CM, et al. Development and validation of prescription drug risk, efficacy, and benefit perception measures in the context of direct-to-consumer prescription drug advertising. Res Soc Adm Pharm. (2021) 17:942–55. doi: 10.1016/j.sapharm.2020.07.028
47. Lazarus JV, Ratzan SC, Palayew A, Gostin LO, Larson HJ, Rabin K, et al. A global survey of potential acceptance of a COVID-19 vaccine. Nat Med. (2021) 27:225–8. doi: 10.1038/s41591-020-1124-9
48. Luxi N, Giovanazzi A, Capuano A, Crisafulli S, Cutroneo PM, Fantini MP, et al. COVID-19 vaccination in pregnancy, paediatrics, immunocompromised patients, and persons with history of allergy or prior SARS-CoV-2 infection: overview of current recommendations and pre- and post-marketing evidence for vaccine efficacy and safety. Drug Saf. (2021) 44:1247–69. doi: 10.1007/s40264-021-01131-6
49. Yan J, Ouyang Z, Vinnikova A, Chen MX. Avoidance of the threats of defective vaccines: how a vaccine scandal influences parents' protective behavioral response. Health Commun. (2021) 36:962–71. doi: 10.1080/10410236.2020.1724638
50. Yan E, Lai DWL, Lee VWP. Predictors of intention to vaccinate against COVID-19 in the general public in Hong Kong: findings from a population-based, cross-sectional survey. Vaccines. (2021) 9:696. doi: 10.3390/vaccines9070696
51. Costa-Font M, Gil JM. Structural equation modelling of consumer acceptance of genetically modified (GM) food in the Mediterranean Europe: a cross country study. Food Qual Prefer. (2009) 20:399–409. doi: 10.1016/j.foodqual.2009.02.011
52. Cheng P, Ouyang Z, Liu Y. The effect of information overload on the intention of consumers to adopt electric vehicles. Transportation. (2020) 47:2067–86. doi: 10.1007/s11116-019-10001-1
53. Anthoine E, Moret L, Regnault A, Sébille V, Hardouin JB. Sample size used to validate a scale: a review of publications on newly-developed patient reported outcomes measures. Health Qual Life Outcomes. (2014) 12:176. doi: 10.1186/s12955-014-0176-2
54. Gefen DS DW, Boudreau MC. Structural equation modeling and regression: guidelines for research practice. Commun Assoc Inform Syst. (1978) 4:1–70. doi: 10.17705/1CAIS.00407
55. McVeigh J, MacLachlan M, Vallieres F, Hyland P, Stilz R, Cox H, et al. Identifying predictors of stress and job satisfaction in a sample of merchant seafarers using structural equation modeling. Front Psychol. (2019) 10:70. doi: 10.3389/fpsyg.2019.00070
56. Zhang YY, Liu CY, Luo SM, Xie YT, Liu F, Li X, et al. Factors influencing patients' intentions to use diabetes management apps based on an extended unified theory of acceptance and use of technology model: web-based survey. J Med Internet Res. (2019) 21:e15023. doi: 10.2196/15023
57. Amini A, Alimohammadlou M. Toward equation structural modeling: an integration of interpretive structural modeling and structural equation modeling. J Manag Anal. (2020) 8:693–714. doi: 10.1080/23270012.2021.1881927
58. Hoque R, Sorwar G. Understanding factors influencing the adoption of mHealth by the elderly: an extension of the UTAUT model. Int J Med Inform. (2017) 101:75–84. doi: 10.1016/j.ijmedinf.2017.02.002
59. Fernandes Q, Inchakalody VP, Merhi M, Mestiri S, Taib N, El-Ella DMA, et al. Emerging COVID-19 variants and their impact on SARS-CoV-2 diagnosis, therapeutics and vaccines. Ann Med. (2022) 54:524–40. doi: 10.1080/07853890.2022.2031274
60. Bearth A, Siegrist M. Are risk or benefit perceptions more important for public acceptance of innovative food technologies: a meta-analysis. Trends Food Sci Technol. (2016) 49:14–23. doi: 10.1016/j.tifs.2016.01.003
61. Kaplan RM, Milstein A. Influence of a COVID-19 vaccine's effectiveness and safety profile on vaccination acceptance. Proc Natl Acad Sci U S A. (2021) 118:e2021726118. doi: 10.1073/pnas.2021726118
62. Savolainen R. Information need as trigger and driver of information seeking: a conceptual analysis. Aslib J Inf Manag. (2017) 69:2–21. doi: 10.1108/AJIM-08-2016-0139
63. Zhou SH. Impact of perceived risk on epidemic information seeking during the outbreak of COVID-19 in China. J Risk Res. (2021) 24:477–91. doi: 10.1080/13669877.2021.1907609
64. Hubner AY, Hovick SR. Understanding risk information seeking and processing during an infectious disease outbreak: the case of Zika virus. Risk Anal. (2020) 40:1212–25. doi: 10.1111/risa.13456
65. Okuhara T, Ishikawa H, Okada M, Kato M, Kiuchi T. Contents of Japanese pro- and anti-HPV vaccination websites: a text mining analysis. Patient Educ Couns. (2018) 101:406–13. doi: 10.1016/j.pec.2017.09.014
66. Shoots-Reinhard B, Lawrence ER, Schulkin J, Peters E. Excluding numeric side-effect information produces lower vaccine intentions. Vaccine. (2022) 40:4262–9. doi: 10.1016/j.vaccine.2022.06.001
67. Holzmann-Littig C, Frank T, Schmaderer C, Braunisch MC, Renders L, Kranke P, et al. COVID-19 vaccines: fear of side effects among German health care workers. Vaccines. (2022) 10:689. doi: 10.3390/vaccines10050689
68. Jing Y, Wei JC, Zhe OY, Vinnikova A, Zhao DT, Zhang HB. The influence of parents' information processing on childhood vaccine acceptance after a vaccine crisis in China. Health Risk Soc. (2019) 21:284–303. doi: 10.1080/13698575.2019.1619672
Keywords: COVID-19 vaccine, online health community, information processing, risk perception, benefit perception
Citation: Liu H, Gong L, Wang C, Gao Y, Guo Y, Yi M, Jiang H, Wu X and Hu D (2023) How information processing and risk/benefit perception affect COVID-19 vaccination intention of users in online health communities. Front. Public Health 11:1043485. doi: 10.3389/fpubh.2023.1043485
Received: 13 September 2022; Accepted: 03 February 2023;
Published: 21 February 2023.
Edited by:
Victoria Team, School of Nursing and Midwifery, Faculty of Medicine, Nursing and Health Sciences, Monash University, AustraliaReviewed by:
Patrizia Laurenti, Catholic University of the Sacred Heart, Rome, ItalyCopyright © 2023 Liu, Gong, Wang, Gao, Guo, Yi, Jiang, Wu and Hu. This is an open-access article distributed under the terms of the Creative Commons Attribution License (CC BY). The use, distribution or reproduction in other forums is permitted, provided the original author(s) and the copyright owner(s) are credited and that the original publication in this journal is cited, in accordance with accepted academic practice. No use, distribution or reproduction is permitted which does not comply with these terms.
*Correspondence: Dehua Hu, aHVkZWh1YUBjc3UuZWR1LmNu
†These authors have contributed equally to this work and share first authorship
Disclaimer: All claims expressed in this article are solely those of the authors and do not necessarily represent those of their affiliated organizations, or those of the publisher, the editors and the reviewers. Any product that may be evaluated in this article or claim that may be made by its manufacturer is not guaranteed or endorsed by the publisher.
Research integrity at Frontiers
Learn more about the work of our research integrity team to safeguard the quality of each article we publish.