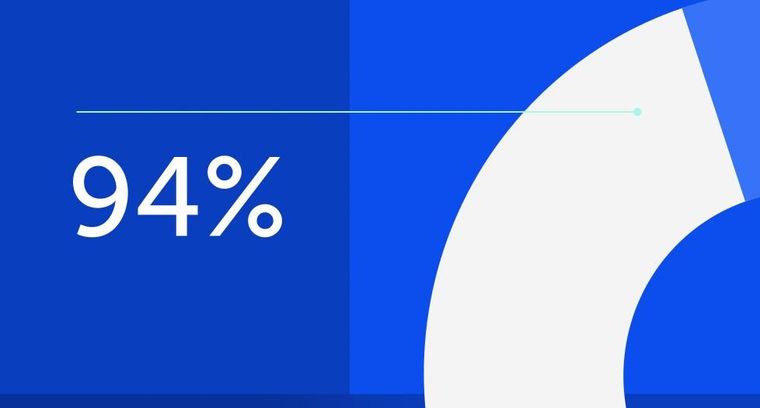
94% of researchers rate our articles as excellent or good
Learn more about the work of our research integrity team to safeguard the quality of each article we publish.
Find out more
ORIGINAL RESEARCH article
Front. Public Health, 24 March 2023
Sec. Digital Public Health
Volume 11 - 2023 | https://doi.org/10.3389/fpubh.2023.1036877
This article is part of the Research TopicDigital Health Quality, Acceptability, and Cost: Steps to Effective Continuity of Cancer CareView all 7 articles
Background: The progression into the Digital Age has brought an array of novel skill requirements. Unlike traditional literacy, there are currently few measures that can reliably measure eHealth literacy. The Transactional Model of eHealth Literacy and subsequent Transactional eHealth Literacy Instrument may provide a feasible option for measuring eHealth literacy.
Objective: This instrument has yet to be validated, which is the aim of this study. In particular, this article was conducted to validate the TeHLI to see which components of the tool (how many and which components included) would be the best fit statistically and whether the tool applies to groups of different characteristics.
Methods: We conducted an online cross-sectional study among 236 Vietnamese young people. A exploratory factor analysis was used to identify the best fit model of the Transactional eHealth Literacy Instrument. A confirmatory factor analysis tested measurement invariance at four levels: configural, metric, scalar, and strict invariance. Only metric invariance was partially invariant, while the rest tested fully invariant. Even with partial metric invariance, there is reason to assume that functional, communicative, critical, and translational eHealth literacy (the four levels according to the transactional model) are consistently measured when deploying the Transactional eHealth Literacy Instrument across groups.
Results: The study findings substantiate that the most optimal composition of the TeHLI consists of four factors: functional, communicative, critical, and translational eHealth literacy, with RMSEA = 0.116; CFI = 0.907, and the highest internal consistency (Cronbach's α = 0.91, 0.92, 0.88, and 0.92 for each factor respectively). After using measurement invariance, that gender, education, marital status, age, location, and household economy do not influence the way participants to respond to the TeHLI to the point that would introduce measurement bias. In other word, using TeHLI across population groups should not produce error margins that substantially differ from each other.
Conclusions: This study suggests the instrument can be used for comparisons across groups and has the potential to generate high-quality data usable for informing change agents as to whether a particular population is proficient enough to adopt novel eHealth innovations.
eHealth literacy is a skill set that is becoming increasingly relevant as the Digital Age progresses (1, 2). When healthcare and public health services slowly moved to the digital sphere (3), the skills required to search for, identify, and access these services started changing incrementally (4). However, much like most of the world, these services transitioned to digital environments at the beginning of the COVID-19 pandemic (5). While innovations are typically taken up gradually by a population (6), society adapted acutely to the pandemic—resulting in digital and technological advances neither equally nor equitably permeating all layers of society across the globe and potentially widening existing inequalities (7–9). In fact, there are some indications that the migration to the digital environment was used as a short-term solution and that—when traditional health services reemerged—these were again preferred by those seeking healthcare (10).
eHealth literacy is a specific branch in the field of digital literacy (4). Given the transition toward digitalization globally, there have been attempts to define eHealth literacy. For instance, UNESCO frames digital literacy as a set of foundational skills needed to work in the digital world and is considered a catalyst for individuals to achieve health and social outcomes (11). Current training programs tend to focus heavily on technical skills required to use digital technologies, while cognitive and ethical considerations are disregarded entirely (12, 13). It takes ~5 years for the average individual to become sufficiently proficient in using digital technologies (12). However, this 5-year gap is not equal across all societal domains. For example, the medical sector has been slower to adapt to the digital world (14).
Recent work by Paige and colleagues created a tool with which to measure eHealth literacy (15). The Transactional eHealth Literacy Instrument (TeHLI) is a multi-dimensional instrument to measure increasing levels of eHealth literacy, based on the Transactional Model of eHealth Literacy (TMeHL) (16): functional (the ability to successfully read and write about health using technological devices), communicative (the ability to control, adapt, and collaborate communication about health with others in online social environments), critical (the ability to evaluate the relevance, trustworthiness, and risks of sharing and receiving health-related information on the Internet), and translational eHealth literacy (the ability to apply health-related information from the Internet in different contexts). However, given the novelty of this assessment tool, its application has only been focused on the general and middle age populations. However, as the driver population of eHealth utilization is the teenager and working population, more research is needed to test TeHLI on younger adults (15). This article aims to validate the TeHLI to see which components of the tool (how many components and which components included) would be the best fit statistically and whether the tool can be applied to groups of different characteristics (i.e., whether the different characteristics in terms of gender, education level, age group and other sociodemographic factors impact the effectiveness of the tool in any way) through exploratory and confirmatory factor analyses of survey data from Vietnamese young adults.
An online cross-sectional study was conducted among Vietnamese young people from April to June 2020 in Vietnam. The eligibility criteria for participating in this survey were: (1) ages between 16 and 35 years; (2) currently living in Vietnam; and (3) agreed to join this study by providing online informed consent.
In this study, the snowball sampling technique was used to recruit participants from all provinces of Vietnam. First, we developed a core group of participants with 20 people (including leaders of the Youth Union in different public institutions, companies, and organizations) and invited them to complete the survey. After completing the survey, these participants were requested to invite their peers in their respective networks to complete the online survey. At the end of the data collection period, 236 youths aged 16–35 living in Vietnam agreed to participate and completed the survey. This study was approved by the institutional review board of the Youth Research Institute, Vietnam.
In this study, we built an online survey on the Survey Monkey platform. This approach is low cost, consumes little time, is user-friendly for youth, and is highly accessible to reach the samples nationwide. To complete this survey, each participant spent about 5–10 min. A structured questionnaire was used to collect information, consisting of two components: (1) sociodemographic characteristics, and (2) the TeHLI. The survey was first piloted on five youths to ensure the cross-cultural validity of translated instruments in Vietnamese. After that, the revised questionnaire was uploaded into the online survey portal. The data collection began once the online survey system was tested to assure accurate question contents and no technical issues.
Participants were asked to report their sociodemographic information, including age, sex (male/female), educational attainment (below high school and high school/college/tertiary and higher), marital status (single/other), and living areas (urban/town/rural or mountain area).
The Transactional eHealth Literacy Instrument (TeHLI) was developed to reflect the theoretical assumptions of the TMeHL by Paige et al. (15). This scale consisted 18 items to reflect four aspects of the TMeHL such as functional (4 items), communicative (5 items), critical (5 items), and translational (4 items). A 5-point Likert scale from 1 (strongly disagree) to 5 (strongly agree) was used for each item. The total score of all items in each domain was calculated, with 4–16 points for both functional and translational domains and 5–25 points for both communicative and critical domains. The higher score of each domain indicated a higher level of eHealth skills. In this study, the Cronbach's α of four domains of TeHLI were 0.91; 0.92; 0.88; 0.92, respectively. Furthermore, by reviewing previous studies, the characteristics, as well as other versions of TeHLI were present in Table 1.
Statistical analysis was performed using STATA version 16 and R. Standard descriptive statistical analysis was conducted with mean and standard deviation (SD) for quantitative variables and frequency and percentage for qualitative variables. The value of Skewness and Kurtosis coefficients were reported. Floor and ceiling effects were identified if the percentage of participants answering the lowest or highest response option was above 15% (18). A p-value (p) < 0.05 was considered statistically significant. The Exploratory Factor Analysis (EFA) was performed to indicate the items belonging to the four models of the TMeHL: Model 1 (18 items divided into one factor); Model 2 (18 items into two factors); Model 3 (18 items into three factors); Model 3 (18 items into four factors). For the estimation of the number of questions of each component and properties of each item in each model, an orthogonal varimax rotation with Kaiser normalization was utilized. Furthermore, after using EFA, to determine the factor structure, we conducted a Confirmatory Factor Analysis (CFA) to test the original model (4 factors), then the new models of TeHLI. Multiple model fit indicators with respective cutoffs assessed the model fit of observed data (with Satorra-Bentler correction for non-normality data) (19), including:
- Relative Chi-square (χ2/df): a value ≤3.0 for good fit;
- Root Mean Square Error of Approximation (RMSEA): a value of ≤0.08 for good fit;
- Comparative Fit Index (CFI): a value of ≥0.9 for acceptable fit;
- Standardized Root Mean Square Residual (SRMR): a value of ≤0.08 for good fit.
Measurement invariance of 4-factor model of the TeHLI regarding gender, age group, education, marital status, location, and household economy was tested by using four models as recommended. four models with different levels of measurement invariance: (1) configural invariance; (2) metric invariance; (3) strong (scalar) invariance; and (4) strict invariance.
Firstly, we evaluated configural invariance to assess what model is least stringent. This step aimed to test whether the constructs have the same pattern of free and fixed loadings across groups. Secondly, when configural invariance was supported, we evaluated metric invariance (also called pattern or weak invariance) or equivalence of the item loadings on the factors. Metric invariance was established by constraining factor loadings to be equivalent across groups. Thirdly, we investigated scalar invariance by constraining equal intercepts and factor loadings across groups. This step assures that participants in different groups, on average, rate items similarly. Finally, we analyzed strict invariance, which is supported when equal error variances are constrained in addition to equal intercepts and factor loadings. Testing residual error establishes that the same amount of error, or variance not accounted by the factor, is consistent for each item across groups. Measurement invariance is tested by some goodness of fit indexes, such as ΔCFI, ΔRMSEA, Δχ2. When we used Δχ2 as a sole measure of exact fit, the results showed that it was associated with lower levels of scalar invariance. By contrast, using the ΔCFI (with or without other criteria like ΔRMSEA) was associated with higher levels metrics, scalar, and strict model. Therefore, in this study, change in the CFI (ΔCFI) was used as the primary criterion for comparing models, and ΔCFI < 0.01 between successively more restricted models provides evidence for measurement invariance (20–22).
Table 2 shows the sociodemographic of the 236 participants in this study. The mean age of respondents was 21.3 ± 4.8 years, with the most common age group about above 24 years old (78.8%). The majority of those were female (71.2%) and 56.8% of participants had the education tertiary and upper.
Table 3 presents the results of the descriptive analysis for 18 items of the TeHLI. All the 18 items had a range of scores from 1 to 5. Out of 18 items, the maximum and mimimum scores was recored at 3.3 (0.9), and 3.3 (0.9), respectively. Skewness and Kurtosis coefficients were reported with ranging from −1.0 to −0.2 and 2.8 to 4.3, respectively. Table 3 also presented the reliability of the modified TeHLI. Correlation coefficients with other items in respective factors ranged between 0.7 and 0.8. Cronbach's alpha value of factor 1 “Functional,” factor 2 “Communicative,” factor 3 “Critical,” and factor 4 “Translational” were good at 0.82, 0.92, 0.92, and 0.90, respectively.
Table 4 further shows the results of Confirmatory Factor Analysis on four different models. The model with 1 factor of the TeHLI showed an RMSEA and CFI score of 0.157 and 0.806. In contrast, the model with four factors showed an RMSEA and CFI score of 0.116 and 0.907, respectively. As such, this model was used to conduct measurement invariance.
Table 4. Exploratory factor analysis and confirmatory factor analysis models and fit indices evaluating factor structure of the transactional eHealth literacy scale.
The results obtained from the Confirmatory Factor Analysis for the TeHLI are estimated in Figure 1. The standardized coefficients range from 0.87 to 1.1. The highest coefficient was found in 4 items (Q4, Q11, Q13, and Q17), while the lowest was Q8.
Figure 1. CFA models through structural equation modeling for the transactional eHealth literacy instrument (RMSEA = 0.116; CFI: 0.907; SRMR: 0.047).
The values of ΔCFI were <0.01 when all models, with progressive restrictions, are compared across age groups, education, household economic (Table 5). These groups reported strict invariance. Besides, the configural model of gender, marital status, and location had fit statistics of RMSEA < 0.08, CFI > 0.9. However, in the metric model, the value of ΔCFI was higher than 0.01 at all three variables (gender, marital status, and location). Metric invariance was therefore not supported by the data.
Table 5. Measurement invariance in the transactional eHealth literacy questionnaire for the different groups.
This article aimed to validate the TeHLI to see which components of the tool (how many and which components included) would be the best fit statistically and whether the tool applies to groups of different characteristics. Overall, we found that a TeHLI divided into four factors (functional, communicative, critical, and translational) had the best statistical fit (RMSEA = 0.116; CFI = 0.907) and the highest level of internal consistency (Cronbach's α = 0.96). The individual items in the TeHLI have medium-to-high levels of correlation with other items in respective factors (r > 0.6). Upon further analysis, we found partial invariance of the TeHLI—only lacking support for metric invariance.
The study findings substantiate that the most optimal composition of the TeHLI consists of four factors: functional, communicative, critical, and translational eHealth literacy. In this model, Q1–Q4 measure functional eHealth literacy, Q5–Q9 measure communicative eHealth literacy, Q10–Q14 measure critical eHealth literacy, and Q15–Q18 measure transactional eHealth literacy. This tool composition is in line with the original dimensions of the TMeHL (16). The initial measurements of high internal consistency of the four domains by Paige and colleagues (Cronbach's α = 0.91, 0.92, 0.88, and 0.92 respectively) was also supported by our findings (Cronbach's α = 0.88, 0.92, 0.92, and 0.90 respectively) (15).
In terms of measurement invariance, the support for configural invariance indicates that the same construct is measured in different population groups. In the context of this study, functional, communicative, critical, and translational eHealth literacy are consistently measured when the TeHLI is deployed in different population groups. The support for scalar invariance indicates the ability to justify the comparison of means across population groups. It indicates that gender, education, marital status, age, location, and household economy do not influence the way participants to respond to the TeHLI to the point that would introduce measurement bias. The support for strict invariance indicates that the residual errors are equivalent across participants. In other words, using the TeHLI across population groups should not produce error margins that substantially differ from each other. However, metric invariance was only found in two groups (education [ΔCFI = 0.002] and household economy [ΔCFI = 0.005]; age group being precisely on the threshold [ΔCFI = 0.010]) and, therefore, only partial metric invariance is supported by this data (23), which suggests that the constructs measured have different meanings across some of the participant groups.
Finding partial support for metric invariance is reported to have minimal effects on the mean differences of a latent factor (24). Since two factor loadings reported metric invariance, there are grounds to assume the TeHLI can be used for comparisons across groups (25, 26). Simultaneously, there is also the question of whether partial invariance is sufficient as significant bias can still be introduced into study findings if the partial invariance is ignored (27, 28). As such, deploying a partially (including invariant items only) and a fully invariant model (including invariant and non-invariant items) and compare the results of interest arises as a viable solution to use the TeHLI across different population groups (29). The risk of this solution is that—if the results differ substantially—there is no clear path forward.
Some limitations apply to this study. The survey findings had a mild-to-moderate negative skewness. However, as extrapolation to the population is impossible given the lack of participants, this limitation does not significantly affect the study findings. The survey was also distributed only among the Vietnamese population. Therefore, the influence of culture could not be determined and needs to be examined in a future study that includes multiple countries or regions. Lastly, the current study sample did not reflect a balanced distribution of some socio-demographic characteristics and could lead to limit representativeness with respect to the Vietnamese youth population. Hence, further studies are required to explore the effect of the data imbalance on measurement invariances of the scales.
Ultimately, the TeHLI can be considered a valuable tool to measure different competency levels of eHealth literacy. As such, the TeHLI has the potential (if deployed correctly) to generate high-quality data that can be used to inform governments and change agents whether the general or a target population is capable of understanding, appraising, distinguishing, and acting appropriately on high- and low-quality health information on the Internet. As a result, it can make vital contributions in informing and guiding policy and practice sustainably into the Digital Age.
The raw data supporting the conclusions of this article will be made available by the authors, without undue reservation.
The protocol of this study was approved by the Institutional Review Board of Youth Research Institute, Vietnam (No 404QÐ*/TWÐ*TN-VCNTN) on 08/01/2020. The patients/participants provided their written informed consent to participate in this study.
TPTN: conceptualization, data collection, project management, and writing—review and editing. AD and HD: conceptualization, data collection, and project management. TV and RK: formal analysis, validation, writing—original draft, and writing—review and editing. BLHW, LB, GF, and PA: formal analysis, writing—original draft, and writing—review and editing. TTN: formal analysis, data collection, data analysis, and writing—original draft. CL and CH: conceptualization and writing—review and editing. RH: conceptualization, validation, and writing—review and editing. All authors contributed to the article and approved the submitted version.
The article process charge of this paper is supported by the NUS Department of Psychological Medicine (R-177-000-100-001/R-177-000-003-001); and NUS iHeathtech Other Operating Expenses (R-722-000-004-731).
The authors would like to acknowledge supports by all respondents who participated in the study.
The authors declare that the research was conducted in the absence of any commercial or financial relationships that could be construed as a potential conflict of interest.
All claims expressed in this article are solely those of the authors and do not necessarily represent those of their affiliated organizations, or those of the publisher, the editors and the reviewers. Any product that may be evaluated in this article, or claim that may be made by its manufacturer, is not guaranteed or endorsed by the publisher.
1. Sonnier P. The Fourth Wave: Digital Health-A New Era of Human Progress. San Francisco, CA: The Story of Digital Health (2020).
2. Kickbusch I, Piselli D, Agrawal A, Balicer R, Banner O, Adelhardt M, et al. The Lancet and Financial Times Commission on governing health futures 2030: growing up in a digital world. Lancet. (2021) 398:1727–76. doi: 10.1016/S0140-6736(21)01824-9
3. Odone A, Buttigieg S, Ricciardi W, Azzopardi-Muscat N, Staines A. Public health digitalization in Europe: EUPHA vision, action and role in digital public health. Eur J Public Health. (2019) 29(Supplement_3):28–35. doi: 10.1093/eurpub/ckz161
4. van Kessel R, Wong BLH, Clemens T, Brand H. Digital health literacy as a super determinant of health: more than simply the sum of its parts. Internet Intervent. (2022) 27:148. doi: 10.1016/j.invent.2022.100500
5. Almeida F, Santos JD, Monteiro JA. The challenges and opportunities in the digitalization of companies in a post-COVID-19 world. IEEE Eng Manag Rev. (2020) 48:97–103. doi: 10.1109/EMR.2020.3013206
6. Rogers EM, Murcott S. Attributes of innovations and their rate of adoption. Diff Innovat. (1995) 12:204–51.
7. Honeyman M, Maguire D, Evans H, Davies A. Digital Technology and Health Inequalities: A Scoping Review. Cardiff: Public Health Wales NHS Trust (2020).
8. van Kessel R, Hrzic R, O'Nuallain E, Weir E, Wong BLH, Anderson M, et al. Digital health paradox: international policy perspectives to address the increased health inequalities for people living with disabilities. J Med Int Res. (2022) 24:e33819. doi: 10.2196/33819
9. van Kessel R, Wong BLH, Forman R, Gabrani J, Mossialos E. The European Health Data Space needs to be more inclusive to bridge digital divides. BMJ. (2022) 378:e071913. doi: 10.1136/bmj-2022-071913
10. van Kessel R, Kyriopoulos I, Wong BLH, Mossialos E. The effect of the COVID-19 pandemic on digital health-seeking behavior: Big data interrupted time-series analysis of Google Trends. J Med Internet Res. (2023) 25:e42401. doi: 10.2196/42401
11. UNESCO. Digital Literacy in Education. London: UNESCO (2011). Available online at: https://iite.unesco.org/pics/publications/en/files/3214688.pdf (accessed September 03, 2022).
12. Chetty K, Qigui L, Gcora N, Josie J, Wenwei L, Fang C. Bridging the digital divide: measuring digital literacy. Economics. (2018) 12:14598. doi: 10.5018/economics-ejournal.ja.2018-23
13. Wong BLH, Khurana MP, Smith RD. Harnessing the digital potential of the next generation of health professionals. Hum Resour Health. (2021) 19:50. doi: 10.1186/s12960-021-00591-2
14. Richard Bartlett AS, Christian Z. How the Medtech Industry can Capture Value from Digital Health. Ashburn: McKinsey&Company (2021).
15. Paige SR, Stellefson M, Krieger JL, Miller MD, Cheong J, Anderson-Lewis C. Transactional eHealth literacy: developing and testing a multi-dimensional instrument. J Health Commun. (2019) 24:737–48. doi: 10.1080/10810730.2019.1666940
16. Paige SR, Stellefson M, Krieger JL, Anderson-Lewis C, Cheong J, Stopka C. The transactional model of electronic health (eHealth) literacy. J Med Internet Res. (2018) 20:e10175. doi: 10.2196/10175
17. Vasquez TS, Bylund CL, Fisher CL, Paige SR. Validation of the transactional eHealth literacy instrument with cancer caregivers. PEC Innovat. (2022) 1:100075. doi: 10.1016/j.pecinn.2022.100075
18. McHorney CA, Tarlov AR. Individual-patient monitoring in clinical practice: are available health status surveys adequate? Qual Life Res. (1995) 4:293–307.
19. Hooper D, Coughlan J, Mullen M. Structural equation modeling: guidelines for determining model fit. Elect J Bus Res Methods. (2008) 6:11426–36. doi: 10.21427/D7CF7R
20. Putnick DL, Bornstein MH. Measurement invariance conventions and reporting: the state of the art and future directions for psychological research. Dev Rev. (2016) 41:71–90. doi: 10.1016/j.dr.2016.06.004
21. Villarreal-Zegarra D, Copez-Lonzoy A, Bernabé-Ortiz A, Melendez-Torres GJ, Bazo-Alvarez JC. Valid group comparisons can be made with the patient health questionnaire (PHQ-9): a measurement invariance study across groups by demographic characteristics. PLoS ONE. (2019) 14:e0221717-e. doi: 10.1371/journal.pone.0221717
22. Hu Lt, Bentler PM. Cutoff criteria for fit indexes in covariance structure analysis: conventional criteria vs. new alternatives. Struct Equ Model Multidiscipl J. (1999) 6:1–55.
23. Pirralha A. Testing for measurement invariance with many groups. In: Workshop at the WAPOR 73rd Annual Conference, Virtual Conference. Lincoln, NE: World Association for Public Opinion Research (WAPOR) (2020). Available online at: https://www.andrepirralha.com/talk/invariance (accessed September 03, 2022).
24. Steinmetz H. Analyzing observed composite differences across groups: is partial measurement invariance enough? Methodol Eur J Res Methods Behav Soc Sci. (2013) 9:1. doi: 10.1027/1614-2241/a000049
25. Byrne BM, Shavelson RJ, Muthén B. Testing for the equivalence of factor covariance and mean structures: the issue of partial measurement invariance. Psychol Bull. (1989) 105:456.
26. Steenkamp JB, Baumgartner H. Assessing measurement invariance in cross-national consumer research. J Consum Res. (1998) 25:78–90.
27. Guenole N, Brown A. The consequences of ignoring measurement invariance for path coefficients in structural equation models. Front Psychol. (2014) 5:980. doi: 10.3389/fpsyg.2014.00980
28. Steinmetz H. Estimation and Comparison of Latent Means Across Cultures: Cross-Cultural Analysis. New York, NY: Routledge (2018). p. 95–126. doi: 10.4324/9781315537078-4
Keywords: eHealth, eHealth literacy, transactional model of eHealth literacy, digital health, validation
Citation: Nguyen TPT, Do AL, Do HN, Vu TMT, van Kessel R, Wong BLH, Boyer L, Fond G, Auquier P, Nguyen TT, Latkin CA, Ho CSH and Ho RCM (2023) Evidence of internal structure of the transactional eHealth literacy among Vietnamese youth: An instrument validation study. Front. Public Health 11:1036877. doi: 10.3389/fpubh.2023.1036877
Received: 05 September 2022; Accepted: 06 March 2023;
Published: 24 March 2023.
Edited by:
Anna Christina Singleton, The University of Sydney, AustraliaReviewed by:
Saeedeh Valizadeh, Shahid Beheshti University of Medical Sciences, IranCopyright © 2023 Nguyen, Do, Do, Vu, van Kessel, Wong, Boyer, Fond, Auquier, Nguyen, Latkin, Ho and Ho. This is an open-access article distributed under the terms of the Creative Commons Attribution License (CC BY). The use, distribution or reproduction in other forums is permitted, provided the original author(s) and the copyright owner(s) are credited and that the original publication in this journal is cited, in accordance with accepted academic practice. No use, distribution or reproduction is permitted which does not comply with these terms.
*Correspondence: Thao Phuong Thi Nguyen, bmd1eWVudHBodW9uZ3RoYW83M0BkdXl0YW4uZWR1LnZu
Disclaimer: All claims expressed in this article are solely those of the authors and do not necessarily represent those of their affiliated organizations, or those of the publisher, the editors and the reviewers. Any product that may be evaluated in this article or claim that may be made by its manufacturer is not guaranteed or endorsed by the publisher.
Research integrity at Frontiers
Learn more about the work of our research integrity team to safeguard the quality of each article we publish.