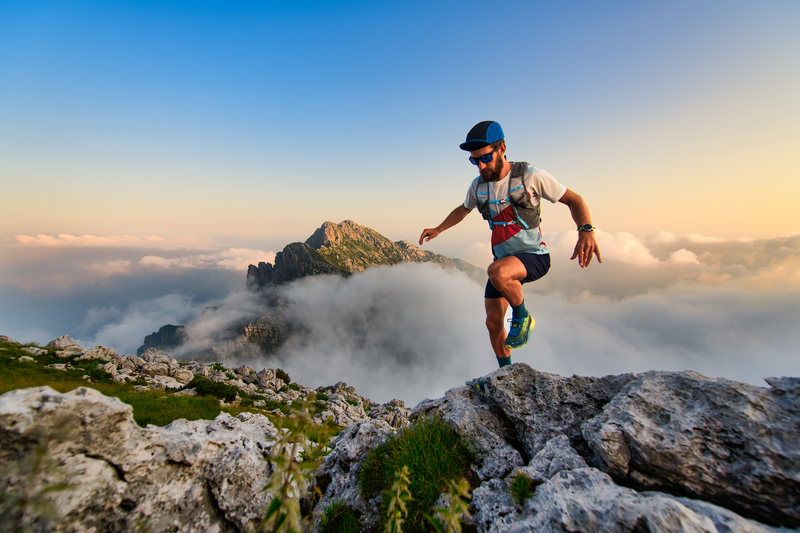
94% of researchers rate our articles as excellent or good
Learn more about the work of our research integrity team to safeguard the quality of each article we publish.
Find out more
SYSTEMATIC REVIEW article
Front. Public Health , 24 March 2023
Sec. Disaster and Emergency Medicine
Volume 11 - 2023 | https://doi.org/10.3389/fpubh.2023.1029558
This article is part of the Research Topic Evaluation of Global Health, Disaster Response, and Humanitarian Projects and Programs View all 11 articles
Background: Remote teaching and online learning have significantly changed the responsiveness and accessibility after the COVID-19 pandemic. Disaster medicine (DM) has recently gained prominence as a critical issue due to the high frequency of worldwide disasters, especially in 2021. The new artificial intelligence (AI)-enhanced technologies and concepts have recently progressed in DM education.
Objectives: The aim of this article is to familiarize the reader with the remote technologies that have been developed and used in DM education over the past 20 years.
Literature scoping reviews: Mobile edge computing (MEC), unmanned aerial vehicles (UAVs)/drones, deep learning (DL), and visual reality stimulation, e.g., head-mounted display (HMD), are selected as promising and inspiring designs in DM education.
Methods: We performed a comprehensive review of the literature on the remote technologies applied in DM pedagogy for medical, nursing, and social work, as well as other health discipline students, e.g., paramedics. Databases including PubMed (MEDLINE), ISI Web of Science (WOS), EBSCO (EBSCO Essentials), Embase (EMB), and Scopus were used. The sourced results were recorded in a Preferred Reporting Items for Systematic Reviews and Meta-Analyses (PRISMA) flowchart and followed in accordance with the PRISMA extension Scoping Review checklist. We included peer-reviewed articles, Epubs (electronic publications such as databases), and proceedings written in English. VOSviewer for related keywords extracted from review articles presented as a tabular summary to demonstrate their occurrence and connections among these DM education articles from 2000 to 2022.
Results: A total of 1,080 research articles on remote technologies in DM were initially reviewed. After exclusion, 64 articles were included in our review. Emergency remote teaching/learning education, remote learning, online learning/teaching, and blended learning are the most frequently used keywords. As new remote technologies used in emergencies become more advanced, DM pedagogy is facing more complex problems.
Discussions: Artificial intelligence-enhanced remote technologies promote learning incentives for medical undergraduate students or graduate professionals, but the efficacy of learning quality remains uncertain. More blended AI-modulating pedagogies in DM education could be increasingly important in the future. More sophisticated evaluation and assessment are needed to implement carefully considered designs for effective DM education.
The emerging evolution of remote technology in disasters has been developed in a multidisciplinary manner and has progressed tremendously in completely different settings, e.g., the COVID-19 pandemic and armed conflicts (1–4). The pandemic has changed the paradigm of traditional face-to-face classroom education and contributed to technology-driven pedagogies such as digital transformations and distance learning through hybrid telecommunications (5–7). The changes are not only affecting postsecondary student pedagogies in developed countries but also in developing countries such as the Southern African Customs Union (SACU) (8–10).
Over the last 20 years, the occurrence, impact magnitude, and economic loss from disasters have increased significantly and show an upward trend worldwide (11). According to the 2022 Disasters Year in Review (Emergency Event Database, EM-DAT), published by the Center for Research on the Epidemiology of Disasters (CRED), the category natural hazards, e.g., flood, storm, and earthquake, had dominated the major events of 2021, and the annual occurrence records are higher than those for the period covering 2001–2020. A total of 432 catastrophic events were recorded in 2021, which is significantly higher than the average of 357 annual catastrophic events for the 2001–2020 average. The catastrophic events of 2021 increased significantly compared to the previous two decades and resulted in extensive economic losses (Supplementary Data). In 2021, the disastrous events accounted for 10,492 deaths, 101.8 million people affected, and 252.1 billion USD in economic losses (12). Asia, especially China and India, remain the most severely impacted countries and has nearly half of the total number of mortalities and 66% of the total wounded. Northern America, especially the US, was severely affected by floods, storms, and the COVID-19 pandemic in 2021, which led to extensive economic losses of up to 112.5 billion USD. Furthermore, Europe was affected by unexpected cold and heat waves in 2021. The severe cold in France in April and wildfires in the Mediterranean regions (Algeria, Bulgaria, Cyprus, Greece, Italy, Macedonia, Tunisia, and Turkey) during the summer caused substantial agricultural damage with devastating consequences (13). The need for disaster medicine education to adequately prepare undergraduate students or graduate professionals for these kinds of disasters will require novel technologies, as well as traditional ones, to meet this challenge.
Due to the advent of artificial intelligence (AI) that relies on big data and the proliferation of the Internet of Things (IoT) for universal applications, e.g., smartphones, smart industries, and smart healthcare, wirelessly powered communication (WPC) devices and distant healthcare delivery systems are booming (14, 15). Mobile edge computing (MEC), a recently developed concept since 2014, is an emergent architecture where cloud computing services are extended to the edge of networks leveraging mobile base station (16). Edge computing refers to a broad set of techniques designed to move computing and storage out of the remote cloud (public or private) and closer to the source of data (17), i.e., the computing resources could be sent to the network's edge nearby the end-mobile devices (18). The MEC could not only efficiently perform emergency computational tasks but also provide advanced, realistic DM training. For example, unmanned aerial vehicles (UAVs) are deployed as flying IoT base stations (UAV-enabled sensors network) in order to enhance communication coverage and facilitate real-time data in the affected areas after disasters (19, 20). UAVs equipped with sensors have versatile uses from detecting toxic pollution to providing a 3-D realistic simulation to enabling an education scenario for first responders in disasters (21). Moreover, these UAVs equipped with miniaturized sensors could also support special missions such as sampling and identification of chemical, biological, radiological, and nuclear (CBRN) events for either civilian organizations or military forces (e.g., NATO Defense Alliance) (22). The significant technological development in mission-oriented UAVs could not only perform detection, identification, and monitoring of CBRN events in affected areas but could also be used as a useful tool for offering postgraduate courses in CBRN protection (22). Digital devices have transformed from “networked computers for collaborative learning” in the 1997–2006 period to “online digital learning” in the 2007–2016 period (23, 24). By using a high-fidelity immersive head-mounted device (HMD) designed by virtual/augmented reality (VR/AR) or newly developed mixed reality (MR)/HoloLens technology, along with disaster risk big data, we can easily build more comprehensive scenarios and learning models for trainees while facing disasters (25). The AI-enhanced training platform is a feasible alternative for undergraduate medical students, professionals, or ordinary people to be familiar with pre-emergency preparedness, disaster assessment, or emergency response/evacuation in simulated settings rather than dangerous environments, e.g., fire, pandemic, earthquake, or toxic situations (26, 27). The HMD-based teaching tools were found to be more effective and viable than other digital devices in medical education (28). Extended reality (XR)-based HMD utilizes the concepts of AR, VR, and MR and could provide more beneficial effects on medical skills and knowledge in medical training (29).
Even though the immersive HMD could make it easier to come up with a new and interesting way for medical students to learn, the DM curriculum should be implemented using an evidence-based, multi-modal approach because training programs do not give students enough opportunities to do cooperative activities, group work, and social interaction at the same time (30). We will discuss HMD-based immersive VR (IVR) in DM education regarding new developments, training efficacies, and how participants value their experience with these technologies.
An extensive literature review was conducted in order to provide a cutting-edge evaluation of the development of medical school students' or health professionals' learning by using remote technologies in facing disasters or emergencies. This review checked different remote learning systems under development, the teaching effects of different applications, and the possible changes in the pedagogy of DM that could materialize in the future. This review focuses on the newly developed technologies applied in DM education and training programs for medical and other undergraduate students. The four specific queries are as follows:
1. What kinds of newly remote technologies have been developed in DM and DM education?
2. What are the related articles or keywords described in the field of DM education in recent years?
3. Could remote technologies adopted in learning pedagogies and training programs for medical staff improve their capabilities?
4. What future objectives will new DM learning attain or strive for?
Five major electronic databases, including PubMed database (MEDLINE), ISI Web of Science (WOS), EBSCO Essentials (EBSCO), EMBASE (EMB), and Scopus, from 1 January 2000 to 15 May 2022 were compiled in our review. All articles were from the Science Citation Index (SCI) and Social Science Citation Index (SSCI) databases. After the literature was reviewed, we obtained the related keywords with the high number of occurrences as queries with the following terms: remote technology (6, 31, 32); remote learning (6, 31, 33); online learning or teaching (7, 10); emergency or disaster response (34, 35); disaster medicine education (30, 36); blended learning (37–39); mobile edge computing (20, 40); virtual or augmented reality (23, 25, 41, 42); drone (14, 20, 43); machine learning (44, 45); deep learning (46, 47); and federated learning (48, 49). Similar articles included in references were also screened. The inclusion criteria were reports or peer-reviewed studies of education and training programs, which were focused on any kind of disaster or emergency such as medical, nursing, pharmacy, social work, or paramedical science, and also for other hospital staffs such as administrators; and peer-reviewed studies, accepted articles for publication, e.g., electronic publications (Epubs), and proceedings which were written in English. Two of our coauthors screened the abstracts for potential articles, and the full texts were checked in detail to decide whether they correlated with our eligibility criteria or not. The exclusion criteria were conference abstracts, unpublished manuscripts, and whitepapers available online; non-medical issues such as industrial or geoengineering planning or projects; articles not published in English; and articles on disaster medicine but do not mention specific education or training programs.
Information visualization (InfoVis) is a visual representation technique utilizing data from abstracts to allow researchers to quickly analyze and understand the huge amount of multidimensional data produced in emergencies (50). Bibliometrics is an InfoVis analysis that quantitatively and qualitatively evaluates citations to scientific publications by constructing and mapping citation graphs to a network representation (51–53). The VOSviewer is the most common tool specifically designed for constructing and visualizing research trends and the maps of co-occurrence keywords in health-related literature on natural disasters or epidemic outbreaks (54–56). The software generates network and cluster visualizations of the data, which can be useful in identifying patterns and relationships among the keywords, including the titles and abstracts of reviewed articles. Cluster Density Visualization is a vivid demonstration of the dataset, and the software will automatically be grouped in clusters according to those related keywords. High densities of connections among the keywords within a cluster will indicate that those keywords are highly related, while low densities of connections show that the keywords are less related. This representation provides a way to identify and explore the relationships between different groups of keywords. We used VOSviewer (v.1.6.18, 2022) to analyze the keywords from the semantic contents we retrieved from PubMed (reference). The keywords included “remote technology AND disaster education,” “remote learning AND disaster medicine education,” “remote learning experience AND disaster medicine education,” “remote system AND disaster medicine education,” “mobile edge computing AND disaster medicine education,” “virtual /augmented reality AND disaster medicine education,” “drone AND disaster medicine education,” and “machine learning AND disaster medicine education.” VOSviewer maps the keywords according to the frequencies and the co-occurrences. According to the circle size which is proportional to the frequencies of the keywords, we chose the remote technology-relevant keywords.
The Preferred Reporting Items for Systematic Reviews Analysis and Meta-Analyses (PRISMA) charting and PRISMA extension for Scoping Reviews (PRISMA-ScR) statement were adopted in the flow of the literature search process (57, 58). In the first, 1080 relevant articles were screened from the databases: 402 from MEDLINE, 250 from WOS, 150 from EBSCO, 134 from EMB, and 144 from Scopus (Figure 1).
Duplicated publications from different databases were checked by two of our authors manually. After duplicates were removed, 450 articles were assessed for eligibility. A further 410 articles were excluded due to failure to correlate with our stated criteria. We added the extended articles, which have been highly cited by other websites (e.g., Google) but were not shown in our previous search of databases (i.e., 24 articles extended). All of the 64 articles in the online electronic databases that were published in peer-reviewed journals are considered to be reliable.
The “hybrid/blended learning” (technology-enhanced learning, problem-based learning, table-top exercise, and computerized simulations) (59, 60) core curriculum focusing on different kinds of disaster scenarios, either in-hospital (e.g., contaminated victims) or out-hospital events (e.g., landslides, floods, and earthquakes), has been implemented in a variety of DM applications and delivered to undergraduate medical or paramedic students in order to improve their disaster preparedness knowledge and the capabilities of prehospital disaster management (36, 37, 61).
Many related state-of-the-art technologies have been integrated into disaster rescue, risk management, epidemic tackling, and remote learning and education (19, 31, 33, 43, 62–64). We reviewed three major categories of distance learning design in DM education and we discuss them in detail below.
The emergence of computation-intensive applications such as MEC devices could provide wide spatial coverage in affected areas during disasters and could perform emergency computational tasks in civil and military areas, which significantly improve post-disaster management (18, 19, 65). The MEC technologies such as webcam/photo-realistic 3-D model imagery capture (66, 67), intelligent wearable medical equipment (point-of-care sonography) (68), robotics (69), and social media (70–72) have all been shown to be beneficial in a variety of emergencies.
For instance, flying UAVs/drones integrated with a MEC server (UAV-enabled MEC), in conjunction with the Global Navigation Satellite System (GNSS), real-time computer vision, and advanced photogrammetric techniques (including deep learning algorithms), could enable first responders to obtain accurate 3-D imagery of affected areas via cloud computing (CC) and aid in the rapid identification of victims (73, 74). Not only could drones be used in disaster mapping for search and rescue operations but also could provide an excellent resource for training paramedic students in mass casualty incidents (MCIs) (75). In addition, 80% of nursing students in the Emergency Nursing Master program at the Catholic University of Murcia (UCAM) reported a significant improvement in their self-perception and performance in the MCI simulation with the assistance of drones (76).
Machine learning (ML) algorithms, such as the evidence-based medicine (EBM) movement, have become a powerful prediction tool ranging from clinical diagnosis to enhancing desired healthy behaviors (77). The medical school curriculum as well as the postgraduate medical education within academic hospitals around the world are facing the challenges of this AI-driven data science revolution (78). Among them, deep learning (DL) was developed from traditional ML techniques and provides more accurate classifications and predictions in disasters, e.g., as victims' detection, image segmentation, and medical information processing, due to powerful computing capacity and the high availability of large datasets (79–81). For example, DL trains a system to filter the digital images and to aid in the prediction of whether a corresponding patient is infected with COVID-19 or not (82). In addition, using convolutional neural network (CNN) technology, a radiologist in a remote area may determine whether a patient's lung is impacted by COVID-19 using chest X-ray images (83, 84). CNN is the most established algorithm among various DL models, which is an artificial neural network dominant in computer vision tasks by stacking mathematical layer operations, i.e., convolution (83). Innovative digital technologies were found to boost AI detection performance during the COVID-19 pandemic while also enhancing learning models for medical professionals in disease diagnosis (46, 47, 85). DL could also detect and identify large amounts of data from social media messages (urgent and not urgent tweets) during a disaster and confirmed a high efficacy in detecting urgent tweets during hurricanes, based on a combined CNN, trained on average word embedding, and support-vector machine (SVM), trained on full word embedding (71). DL not only provides a modern computational platform and automated medical practice but also refers to a comprehensive human learning approach and has been integrated into medical education (86). E-learning in teaching emergency disaster response (ELITE-DR) among undergraduate medical students could provide comprehensive levels of cognitive concepts and visual stimuli for disaster response medicine (87). However, surveys reveal few formal teaching programs incorporated into medical education about AI/ML, whether in Europe, the US, or even in Asian countries (88–91). For example, 66.5% of final year medical school students in Ireland reported zero hours of teaching on AI/ML during their degree, 43.4% had not heard of the term “machine learning,” and 80.6% had not read any academic journal articles on AI/ML (44).
The applications of AI, ML, and DL have been widely introduced and developed for disaster management, i.e., in the aspects of hazard prediction, vulnerability assessment, disaster early warning systems, disaster monitoring, damage assessment, and post-disaster response (92). The preparedness for disaster risk reduction (DRR) (93) and the training on AI for disaster management (94) are important teaching models and could improve first responders' capability while facing different catastrophes. Federated Learning (FL) approach, a de-centralized data collection technique, generates more robust models without sharing data in a central server and enables collaborative training of multiple MECs (e.g., UAVs) locally (48, 95). FL trains at distributed MEC devices to offload data in order to reduce the data crowdsensing, delay transmission, and also protect IoT application privacy (96). FL could prove a more effective training model in disaster scenarios due to massive irrelevant data and a high flow of scalable IoT networks while in disasters. The privacy-preserved federated transfer learning (FedTL) approach could also provide and verify a real disaster image dataset collected from distributed social computing nodes (97). The new-developed FL model will give both AI and humans (e.g., first responders) a sophisticated training course and achieve better performance for the medical image learning model (98).
Virtual reality simulation (VRS) technology is widely used and it seems to be a viable training alternative in different disciplines, e.g., a simulation realistically of different hospital disaster scenarios, which could provide positive effects of confidence and necessary knowledge acquisition for healthcare professionals in hospital (99). Due to the features of reproducibility and repeatability of VRS, the newly developed technology could also improve CBRN training courses for both military and civilian responders to CBRN events (VERTIgO project) (100). VR delivers virtual scenarios and combines with multi-source data, 360 degrees video, and intuitive interaction interfaces to facilitate quality interprofessional education (101, 102). The so-called “Just-in-time” training programs could offer effective personal preparation and resiliency by enhancing responders' situational awareness in critical overloading situations, e.g., in the COVID-19 pandemic (103). Furthermore, the serious game (SG), one of the popularly used game genres nowadays, combines practical aspects with original amusement but is implemented for health or medical education (non-entertain) purposes (104). SG has been applied in improving medical technical skills such as laparoscopic surgery or arthroscopic intervention for postgraduate residency training in medical settings (105, 106). Disaster risk management (DRM)-related SG/simulation also provides a good educational and engagement tool for affected communities, policy-makers, and other vulnerable population (107). The SGs/simulations, with the realistically simulating disaster reality, offer assistance especially in the realm of disaster risk awareness raising, identifying hazards, undertaking preventive actions, empathy triggering, and perspective-taking (107). Moreover, 3-D video capture HMDs provide a more interesting way to interact and contribute to positive feedback responses from children, which are also vulnerable groups in disaster and public health emergencies (108, 109). Although the VRS technology shows a higher learning atmosphere, comprehensibility, and overall recommendation of teaching courses than those with a non-immersive screen (110), some articles showed conflicting results on VR HMD benefits or even no benefits at all (111, 112). Among them, technology limitations (surgical training), usability challenges (less powerful hardware), cybersickness, and limited participants' induced questionable assessment were potential limitations (113).
By analyzing with VOSviewer, we obtained the co-occurrence MeSH keywords mapped as shown with Network and Cluster Density Visualization of the retrieved title and abstract (Figure 2). In the network visualization figure (Figure 2), the size of the circle is proportionate to the frequency of the keywords, and larger circles at keywords mean that they appear more frequently in the dataset. The keywords are categorized into six clusters according to the highly related connection. “COVID-19” classified in cluster 6, is correlated with the keywords “online learning,” “online teaching,” “remote learning,” and “remote teaching” in cluster 1. The keyword “medical education” classified in cluster 4 also has the same correlation with the keywords “online learning,” “online teaching,” “remote learning,” and “remote teaching” in cluster 1. The keywords were classified automatically into six clusters and are presented in Table 1.
Figure 3 presents the study trend as it varied from 2010 to the present. Early in 2020, the remote equipment applied to emergency management was studied. Then, the research target moved to telemedicine and remote sensing technology with the flourishing development of communication technology. While COVID-19 outbreaks had occurred by the end of 2019, the keywords “emergency remote teaching,” “remote learning,” “online learning,” “emergency remote learning,” “blended learning,” and “emergency remote education” mirror the global education remote technology methods that were necessitated to educate the students and to keep safe social distances. Based on the correlation, it seems that people did a lot of studies related to education while they were isolated during the COVID-19 pandemic.
Figure 4 shows the current application technology and the future trend of DM education. Using UAVs and photography equipment to obtain real disaster scenes and recreating them using VRS technologies, such as VR, AR, and MR technologies can provide students with a more authentic learning experience. These technologies can be used to simulate real-world scenarios and provide a more immersive experience, which can help students better understand and prepare for potential disasters.
Figure 4. The integration of real-world scenarios with artificial intelligence technologies initiates disaster medicine remote learning (Future Education Models).
Big data analysis and advanced AI technologies, such as deep learning and federated learning, can also be used in disaster management education. For example, these technologies can be used to analyze large amounts of data from past disasters and identify patterns and trends that can inform future disaster management strategies. They can also be used to develop more accurate simulations and prediction models, which can be used to train students and prepare them for real-world scenarios.
Integrating and applying these new technologies can also enable new educational models, such as remote learning and online teaching, which can increase access to disaster management education and make it more convenient and flexible for students. Overall, the use of technology in disaster management education can significantly enhance students' learning experiences and prepare them more effectively for real-world scenarios.
Blended learning in DM education has been implemented significantly by undergraduate medical or paramedic students and applied widely by clinical professionals. The occurrence of the COVID-19 global epidemic has significantly precipitated the progress and implementation of integrated hybrid (including station rotation, simulation, and distance models) learning in higher education and also in DM education (30, 39). Implementing special epidemic training for medical students, such as COVID-19 pandemic simulated programs, could significantly improve their preparedness, knowledge, and skills when dealing with emerging infectious diseases (114). The use of IoT, social media, UAVs, and advanced machine learning is not only engaging in rapid response, management, and risk reduction in disasters (DRR; e.g., Sendai Framework for Disaster Risk Reduction 2015–2030) but also stressing the important governance challenge of multidisciplinary, transdisciplinary, and interdisciplinary teaching courses on DRR implemented in many universities (115). The remote e-learning DM programs appear to be a crucial concern, and medical schools in vulnerable regions such as Asian countries or low- and middle-income countries, as we previously indicated, need to continue teaching emergency medicine (10, 33). The inability of these countries to afford state-of-the-art technologies (such as VRS-VR or AR) or to have a convenient AI network prevents medical students from using AI to further medical education. In addition to multidisciplinary approaches used in DRR, more inclusive curricula, a theoretical emphasis, a field orientation, and skill development appear to be essential for higher education. However, due to the popularity of AI-enhanced remote learning modalities among medical school students, it is important for faculty to be well-versed in online learning techniques, e-Learning tools, and cutting-edge technology (such as HoloLens) before classes. The new disruptive technologies based on integrated AI, big data, blockchain, and VR/AR could enrich teaching formats and provide broader guidance for faculty resource allocation in pedagogical methods (19, 115). Our review focuses on the application of remote technology in DM education rather than discussing the educational methods or content of different trainee levels. However, strict quality assurance methods (such as Quality Matters) (116) and continuous quality improvement (CQI) should be closely observed to enable faculty to quickly adjust to changes under the guidance of well-defined implementation plans (115).
We evaluated UAV-enabled MEC with 3-D imagery and found it useful for a variety of detection techniques in remote or disaster rescue operations. However, some restraints on the UAV during operations exist, e.g., the huge operational expenditure to maintain its infrastructural nodes or battery endurance for longer aerial communication. A “UAV-enabled wireless powering IoT” (Ue-WPIoT) with smart trajectory information of UAVs and an AI-deep learning model was developed to overcome the limitations and was designed for the consideration of more lightweight UAVs (14). The improvement of Ue-WPIoT could allow trainees more time to become familiar with the new device and fulfill the IoT wireless function in the air. In addition, a new system called the Aerial Remote Triage System has changed how MCI triage is usually done so that life-saving interventions and transportation can happen more quickly (117).
With the development of telecommunication technology, the fifth generation (5G) remote network significantly increased the band and the speed of data transmission, which was very helpful for long-distance medical diagnosis. In the field of DM, telemedicine benefited from remote devices related to research that were popular before the pandemic COVID-19. Remote learning-related research became the hottest study topic for conducting medical education corresponding to the COVID-19 isolation policy. Based on the well-established communication infrastructure and rapidly progressing telecommunication technology, we anticipate that future research will focus on the application of wearable devices in fields such as medical care and monitoring, educational instruments, and emergency rescue training programs.
The Internet of Things has been formulated to establish wireless sensor networks, AI-enhanced monitoring, and smart embedded devices (ex. in UAVs) to facilitate crowd-sourcing (CS) communication, IoT-based Early Warning System (IoTEWS), efficient victim localization (RFID tag and topology preserving maps), data analytics, and knowledge aggregation (effective data mining of collected big data) in disaster management (118, 119). Moreover, the concept of the Internet of Everything (IoE) or even the Internet of Nano Things (IoNT) was built to account for everything that could be connected to the Internet, i.e., it facilitated the connections among people, processes, data, and things (“four pillars”). The IoE provides the potential to analyze millions of connected sensors' data in order to aid automated- and people-based processes. The application of IoE could provide a remote platform by the expansion of CC to connect “everything” online with the implementation of machine-to-machine (M2M), machine-to-people (M2P), and people-to-people (P2P) (120). The concept of IoNT is being extended from IoE to achieve nanoscale networks by embedding nanosensors in diverse objects such as wearable medical devices and by connecting conventional microsensors to nanonetworks (121). Using nanodevices embedded in the environment and medical equipment, the IoNT supports computing for end users to improve in-time data monitoring and early disease diagnosis. Moreover, the IoT could provide positive implications for computer science education by reshaping web programming, tremendous excitement from online teaching, and real-world sensing applications, performed by UK Open University according to IoT principles in remote lessons for more than 2000 students in 2012 (120, 122).
Our review indicates that the newly emergent information technologies, including IoT, Big Data, social media, and machine learning, could facilitate DM tasks in visualizing, analyzing, and predicting disasters and improve the resilience of communities (123–125). Using Big Data Analytics tools and social media data mining (e.g., large-scale satellite imagery data mining) could empower all sectors, from citizens to community, government to non-government organizations, effective remote communication networks, and knowledge graphs. Remote technologies could also provide an integrated conceptual approach to higher education, such as in massive open online courses (MOOCs) or Flipped Classroom (126, 127). However, the AI innovation in DM education programs did not turn out as planned (44, 89, 128). To address the various ways in which medical students and instructors learn and receive feedback from customized lessons, more AI-guided learning pathways need to be developed. Furthermore, assessing the effectiveness of AI teaching modality and their progress in AI technologies, such as IVR, also require AI-mediated pathways (129).
As previously stated, immersive HMDs have many beneficial potentials in disaster scenarios, and there has been a lot of research interest in DM education program applications (23). VRS technologies, especially VR devices, are widely incorporated into education, teaching, and training in various application domains. VRs are useful for many aspects of “soft” skills acquisition, such as cognitive skills, spatial and visual information, visual scanning or observational skills, and affective skills related to controlling emotional responses to stressful or difficult situations (130). Moreover, VR, MR, or HoloLens, via visualizing 3D anatomies, could provide medical training programs, such as surgical technique, catheter placement, or cardiopulmonary resuscitation (131–133). The VRS technologies can also create disaster scenarios based on real-world events, and inexperienced students could learn the details of disaster response without being in dangerous situations. Although many authors treated VR or HoloLens as promising learning tools for higher education, only some design-oriented studies based on systematic target theories are constructed (23). Although the procedure- or practice-oriented content, i.e., realistic surrounding teaching environments, made HMDs more exciting and appealing, the actual usability and performance of students using HMDs remained primarily experimental. Only 2.6% of a scoping review study revealed that conceptual frameworks or theories were designed in medical students' VR education (131). Finally, when considering the use of HMDs, we should consider what goals are attainable with them, which technologies are predisposed to incorporate them, and what steps are required to implement them. Although most participants enjoyed and engaged in these novel techniques, realistic HMDs could not replace traditional methods for providing curriculum content in a short time (42). However, with the improvement of new HMD technologies, the wide-range web capacity, cybersickness-prevented design, and cordless HMDs, the new learning modalities will gradually reduce the technological limitations and make the new learning modalities more popular in the future.
We presented the visualized graph drawn by VOSviewer to stress the connection between various keywords, which mirrored the popular study fields and the progress changing with time. However, we have to point out that VOSviewer could not integrate the search results from different databases. The visualized graph was drawn from the literature searched on either PubMed or WES websites. Other limitations are the option methods of VOSviewer to draw the graph, which can affect the visualized result. For example, choosing the keywords defined by the author or MeSH keywords can result in a different view of the graph.
In this scoping review, by reviewing the state-of-the-art technologies in the last two decades, we found the potential implementation of MECs, such as UAVs combined with IoT, cloud sensor networks, and machine learning was well-suited for integration into emergency services for disaster management. In the future, DM education will be more likely to use blended AI-modulated remote learning models, such as well-developed HMD optical devices based on AI-enhanced real-world scenarios (Figure 4). Moreover, for students to learn well using remote technologies, there should be real-time individual feedback, interactive curriculum review, and AI-assessed algorithms.
The original contributions presented in the study are included in the article/Supplementary material, further inquiries can be directed to the corresponding authors.
C-LK contributed to the conception and design of the study. L-CC organized the database. C-LK and C-CC reviewed all related articles and decided to include extended articles in the study. M-CW and J-ST revised the references. P-CH analyzed and drafted the figures and table. C-LS supervised the study. All authors contributed to the article and approved the submitted version.
The authors declare that the research was conducted in the absence of any commercial or financial relationships that could be construed as a potential conflict of interest.
All claims expressed in this article are solely those of the authors and do not necessarily represent those of their affiliated organizations, or those of the publisher, the editors and the reviewers. Any product that may be evaluated in this article, or claim that may be made by its manufacturer, is not guaranteed or endorsed by the publisher.
The Supplementary Material for this article can be found online at: https://www.frontiersin.org/articles/10.3389/fpubh.2023.1029558/full#supplementary-material
COVID-19, coronavirus disease 2019; DM, disaster medicine; AI, artificial intelligence; MEC, mobile edge computing; UAVs, unmanned aerial vehicles; DL, deep learning; HMD, head-mounted display; EM-DAT, emergency event database; CRED, Center for Research on the Epidemiology of Disasters; IoT, Internet of Things; WPC, wirelessly powered communication; CBRN, chemical, biological, radiological, and nuclear; VR/AR/MR, virtual/augmented/mixed reality; XR, extended reality; GNSS, global navigation satellite system; CC, cloud computing; MCIs, mass casualty incidents; ML, machine learning; CNN, convolutional neural network; SVM, support-vector machine; ELITE-DR, E-learning in teaching emergency disaster response; DRR, Disaster risk reduction; FL, federated learning; FedTL, federated transfer learning; VRS, virtual reality simulation; SG, serious game; DRM, disaster risk management; InfoVis, information visualization; CQI, continuous quality improvement; Ue-WPIoT, UAV-enabled wireless powering IoT; WSNs, Wireless sensor networks; CS, crowd-sourcing; IoTEWS, IoT-based early warning system; IoE, Internet of Everything; IoNT, Internet of Nano Things; MOOCs, massive open online courses.
1. Song X, Zhang HR, Akerkar R, Huang HW, Guo S, Zhong L, et al. Big data and emergency management: concepts, methodologies, and applications. IEEE Trans Big Data. (2022) 8:397–419. doi: 10.1109/tbdata.2020.2972871
2. Arabi YM, Azoulay E, Al-Dorzi HM, Phua J, Salluh J, Binnie A, et al. How the COVID-19 pandemic will change the future of critical care. Intensive Care Med. (2021) 47:282–91. doi: 10.1007/s00134-021-06352-y
3. Asadzadeh A, Pakkhoo S, Saeidabad MM, Khezri H, Ferdousi R. Information technology in emergency management of COVID-19 outbreak. Inform Med Unlocked. (2020) 21:100475. doi: 10.1016/j.imu.2020.100475
4. Davies S, Pettersson T, Oberg M. Organized violence 1989-2021 and drone warfare. J Peace Res. (2022) 59:593–610. doi: 10.1177/00223433221108428
5. Yuan YP, Tan GWH, Ooi KB, Lim WL. Can COVID-19 pandemic influence experience response in mobile learning? Telemat Inform. (2021) 64:101676. doi: 10.1016/j.tele.2021.101676
6. Camilleri MA, Camilleri AC. Remote learning via video conferencing technologies: implications for research and practice. Technol Soc. (2022) 68:101881. doi: 10.1016/j.techsoc.2022.101881
7. Adedoyin OB, Soykan E. Covid-19 pandemic and online learning: the challenges and opportunities. Interact Learn Environ. (2020) 41:1–13. doi: 10.1080/10494820.2020.1813180
8. Williams KM, Corwith A. Beyond bricks and mortar: the efficacy of online learning and community-building at College Park Academy during the COVID-19 pandemic. Educ Inform Technol. (2021) 26:5055–76. doi: 10.1007/s10639-021-10516-0
9. Ray JM, Wong AH, Yang TJ, Buck S, Joseph M, Bonz JW, et al. Virtual telesimulation for medical students during the COVID-19 pandemic. Acad Med. (2021) 96:1431–5. doi: 10.1097/ACM.0000000000004129
10. Ndzinisa N, Dlamini R. Responsiveness vs. accessibility: pandemic-driven shift to remote teaching and online learning. High Educ Res Dev. (2022) 41:1−16. doi: 10.1080/07294360.2021.2019199
11. UCLouvain C, USAID. 2021 Disasters in Numbers-Extreme Events Defining our Lives CRED. Brussels (2022).
12. CRED Crunch 66 - Disasters Year in Review 2021 [Internet]. USAID. (2022). Available online at: https://www.cred.be/publications (accessed April 2022).
13. CRED Crunch 64 - Extreme weather events in Europe [Internet]. USAID. (2021). Available online at: https://cred.be/sites/default/files/CredCrunch64.pdf (accessed Spetember 2021).
14. Liu YL Dai HN, Wang QB, Imran M, Guizani N. Wireless powering Internet of Things with UAVs: challenges and opportunities. IEEE Netw. (2022) 36:146–52. doi: 10.1109/MNET.013.2000385
15. Kelly JT, Campbell KL, Gong EY, Scuffham P. The Internet of Things: impact and implications for health care delivery. J Med Internet Res. (2020) 22:e20135. doi: 10.2196/20135
16. Abbas N, Zhang Y, Taherkordi A, Skeie T. Mobile edge computing: a survey. IEEE Internet Things J. (2018) 5:450–65. doi: 10.1109/JIOT.2017.2750180
17. Hu YC, Patel M, Sabella D, Sprecher N, Young V. Mobile Edge Computing: A Key Technology Towards 5G. France: ETSI (2015). Report No.: 1–16.
18. Abrar M. Ajmal Ua, Almohaimeed ZM, Gui X, Akram R, Masroor R. Energy efficient UAV-enabled mobile edge computing for IoT devices: a review. IEEE Access. (2021) 9:127779–98. doi: 10.1109/ACCESS.2021.3112104
19. Munawar HS, Mojtahedi M, Hammad AWA, Kouzani A, Mahmud MAP. Disruptive technologies as a solution for disaster risk management: a review. Sci Total Environ. (2022) 806:151351. doi: 10.1016/j.scitotenv.2021.151351
20. Xu J, Ota K, Dong M. Big data on the fly: UAV-mounted mobile edge computing for disaster management. IEEE Trans Netw Sci Eng. (2020) 7:2620–30. doi: 10.1109/TNSE.2020.3016569
21. Bruzzone A, Longo F, Massei M, Nicoletti L, Agresta M, Di Matteo R, et al. Disasters and emergency management in chemical and industrial plants: drones simulation for education and training. In: MESAS 2016: Proceedings of the Third International Workshop on Modelling and Simulation for Autonomous Systems - Volume 9991. Rome: Springer-Verlag (2016). p. 301–8. doi: 10.1007/978-3-319-47605-6_25
22. Di Giovanni D, Fumian F, Chierici A, Bianchelli M, Martellucci L, Carminati G, et al. Design of miniaturized sensors for a mission-oriented UAV application: a new pathway for early warning. Int J of Saf Secur Eng. (2021) 11:435–44. doi: 10.18280/ijsse.110417
23. Radianti J, Majchrzak TA, Fromm J, Wohlgenannt I. A systematic review of immersive virtual reality applications for higher education: design elements, lessons learned, and research agenda. Comput Educ. (2020) 147:103778. doi: 10.1016/j.compedu.2019.103778
24. Khanal S, Medasetti US, Mashal M, Savage B, Khadka R. Virtual and augmented reality in the disaster management technology: a literature review of the past 11 years. Front Virtu Real. (2022) 3:843195. doi: 10.3389/frvir.2022.843195
25. Feng Z, González VA, Mutch C, Amor R, Cabrera-Guerrero G. Instructional mechanisms in immersive virtual reality serious games: earthquake emergency training for children. J Comput Assist Learn. (2020) 37:542–56. doi: 10.1111/jcal.12507
26. Andreatta PB, Maslowski E, Petty S, Shim W, Marsh M, Hall T, et al. Virtual reality triage training provides a viable solution for disaster-preparedness. Acad Emerg Med. (2010) 17:870–6. doi: 10.1111/j.1553-2712.2010.00728.x
27. Feng Z, González VA, Trotter M, Spearpoint M, Thomas J, Ellis D, et al. How people make decisions during earthquakes and post-earthquake evacuation: using verbal protocol analysis in immersive virtual reality. Saf Sci. (2020) 129:104837. doi: 10.1016/j.ssci.2020.104837
28. Xu X, Mangina E, Campbell AG. HMD-based virtual and augmented reality in medical education: a systematic review. Front Virtual Real. (2021) 2:692103. doi: 10.3389/frvir.2021.692103
29. Barteit S, Lanfermann L, Bärnighausen T, Neuhann F, Beiersmann C. Augmented, mixed, and virtual reality-based head-mounted devices for medical education: systematic review. JMIR Serious Games. (2021) 9:e29080. doi: 10.2196/29080
30. Voicescu GT, Valente M, Della Corte F, Becerril M, Ragazzoni L, Caviglia M. Medical students' education in disaster medicine: a systematic literature review of existing curricula. Int J Disaster Risk Reduct. (2022) 77:103090. doi: 10.1016/j.ijdrr.2022.103090
31. Ewing LA, Cooper HB. Technology-enabled remote learning during COVID-19: perspectives of Australian teachers, students and parents. Technol Pedagogy Educ. (2021) 30:41–57. doi: 10.1080/1475939X.2020.1868562
32. Pandey VK, De S. Energy-efficient remote mobile device management in infrastructure-less environment for emergency assessment. Int J Commun Syst. (2021) 34:e4930. doi: 10.1002/dac.4930
33. Ciano JD, Acerra J, Tang A. Development of a remote learning educational model for international Emergency Medicine trainees in the era of COVID-19. Int J Emerg Med. (2022) 15:1–6. doi: 10.1186/s12245-021-00405-1
34. Potutan G, Arakida M. Evolving disaster response practices during COVID-19 pandemic. Int J Environ Res Public Health. (2021) 18:3137. doi: 10.3390/ijerph18063137
35. Feng Y, Cui S. A review of emergency response in disasters: present and future perspectives. Nat Hazards. (2020) 105:1109–38. doi: 10.1007/s11069-020-04297-x
36. Bajow N, Djalali A, Ingrassia PL, Ragazzoni L, Ageely H, Bani I, et al. Evaluation of a new community-based curriculum in disaster medicine for undergraduates. BMC Med Educ. (2016) 16:225. doi: 10.1186/s12909-016-0746-6
37. Ingrassia PL, Ragazzoni L, Tengattini M, Carenzo L, Della Corte F. Nationwide program of education for undergraduates in the field of disaster medicine: development of a core curriculum centered on blended learning and simulation tools. Prehosp Disaster Med. (2014) 29:508–15. doi: 10.1017/S1049023X14000831
38. Liu Q, Peng WJ, Zhang F, Hu R, Li YG, Yan WR. The effectiveness of blended learning in health professions: systematic review and meta-analysis. J Med Internet Res. (2016) 18:e2. doi: 10.2196/jmir.4807
39. Singh J, Steele K, Singh L. Combining the best of online and face-to-face learning: hybrid and blended learning approach for COVID-19, post vaccine, & post-pandemic world. J Educ Technol Syst. (2021) 50:140–71. doi: 10.1177/00472395211047865
40. Shakarami A, Ghobaei-Arani M, Shahidinejad A. A survey on the computation offloading approaches in mobile edge computing: a machine learning-based perspective. Comput Netw. (2020) 182:107496. doi: 10.1016/j.comnet.2020.107496
41. Stromberga Z, Phelps C, Smith J, Moro C. Teaching with disruptive technology: the use of augmented, virtual, and mixed reality (HoloLens) for disease education. Adv Exp Med Biol. (2021) 1317:147–62. doi: 10.1007/978-3-030-61125-5_8
42. Moro C, Phelps C, Redmond P, Stromberga Z. HoloLens and mobile augmented reality in medical and health science education: a randomised controlled trial. Br J Educ Technol. (2020) 52:680–94. doi: 10.1111/bjet.13049
43. Yazid Y, Ez-Zazi I, Guerrero-González A, El Oualkadi A, Arioua M. UAV-enabled mobile edge-computing for IoT based on AI: a comprehensive review. Drones. (2021) 5:148. doi: 10.3390/drones5040148
44. Blease C, Kharko A, Bernstein M, Bradley C, Houston M, Walsh I, et al. Machine learning in medical education: a survey of the experiences and opinions of medical students in Ireland. BMJ Health Care Inform. (2022) 29:e100480. doi: 10.1136/bmjhci-2021-100480
45. Mendo IR, Marques G. de la Torre Diez I, Lopez-Coronado M, Martin-Rodriguez F. Machine learning in medical emergencies: a systematic review and analysis. J Med Syst. (2021) 45:88. doi: 10.1007/s10916-021-01762-3
46. Yadav SS, Jadhav SM. Deep convolutional neural network based medical image classification for disease diagnosis. J Big Data. (2019) 6:113. doi: 10.1186/s40537-019-0276-2
47. Sufian A, Ghosh A, Sadiq AS, Smarandache F. A survey on deep transfer learning to edge computing for mitigating the COVID-19 Pandemic. J Syst Archit. (2020) 108:101830. doi: 10.1016/j.sysarc.2020.101830
48. Aledhari M, Razzak R, Parizi RM, Saeed F. Federated learning: a survey on enabling technologies, protocols, and applications. IEEE Access. (2020) 8:140699–725. doi: 10.1109/ACCESS.2020.3013541
49. Tehseen R, Farooq MS, Abid A. A framework for the prediction of earthquake using federated learning. Peer J Comput Sci. (2021) 7:e540. doi: 10.7717/peerj-cs.540
50. Dusse F, Júnior PS, Alves AT, Novais R, Vieira V, Mendonça M. Information visualization for emergency management: a systematic mapping study. Expert Syst Appl. (2016) 45:424–37. doi: 10.1016/j.eswa.2015.10.007
51. Fan J, Gao Y, Zhao N, Dai R, Zhang H, Feng X, et al. Bibliometric analysis on COVID-19: a comparison of research between English and Chinese studies. Front Public Health. (2020) 8:477. doi: 10.3389/fpubh.2020.00477
52. Du HS, Ke X, Chu SKW, Chan LT. A bibliometric analysis of emergency management using information systems (2000-2016). Online Inf Rev. (2017) 41:454–70. doi: 10.1108/OIR-05-2017-0142
53. van Eck NJ, Waltman L. Software survey: VOSviewer, a computer program for bibliometric mapping. Scientometrics. (2010) 84:523–38. doi: 10.1007/s11192-009-0146-3
54. Sweileh WM. A bibliometric analysis of health-related literature on natural disasters from 1900 to 2017. Health Res Policy Syst. (2019) 17:18. doi: 10.1186/s12961-019-0418-1
55. Hamidah I, Sriyono S, Nur Hudha M. A bibliometric analysis of Covid 19 research using VOSviewer. Indonesian J Sci Technol. (2020) 5:18. doi: 10.17509/ijost.v5i2.24522
56. Cobo MJ, López-Herrera AG, Herrera-Viedma E, Herrera F. Science mapping software tools: review, analysis, and cooperative study among tools. J Am Soc Inf Sci Technol. (2011) 62:1382–402. doi: 10.1002/asi.21525
57. Moher D, Liberati A, Tetzlaff J, Altman DG. Preferred reporting items for systematic reviews and meta-analyses: the PRISMA statement. BMJ. (2009) 339:b2535. doi: 10.1136/bmj.b2535
58. Tricco AC, Lillie E, Zarin W, O'Brien KK, Colquhoun H, Levac D, et al. PRISMA extension for scoping reviews (PRISMA-ScR): checklist and explanation. Ann Intern Med. (2018) 169:467–73. doi: 10.7326/M18-0850
59. Buus L, Georgsen M. A learning design methodology for developing short learning programmes in further and continuing education. J Interact Media Educ. (2018) 2018:1–10. doi: 10.5334/jime.469
60. Means B, Toyama Y, Murray R, Baki M. The effectiveness of online and blended learning: meta-analysis of the empirical literature. Teach Coll Rec. (2013) 115:030303. doi: 10.1177/016146811311500307
61. Bhattacharya S, Singh A, Semwal J, Marzo RR, Sharma N, Goyal M, et al. Impact of a training program on disaster preparedness among paramedic students of a tertiary care hospital of North India: a single-group, before-after intervention study. J Educ Health Promot. (2020) 9:5. doi: 10.4103/jehp.jehp_704_19
62. Liu F, Guo Y, Cai Z, Xiao N, Zhao Z. Edge-enabled disaster rescue. ACM Trans Intell Syst Technol. (2019) 10:1–21. doi: 10.1145/3331146
63. Sakurai M, Murayama Y. Information technologies and disaster management – benefits and issues. Progress Disaster Sci. (2019) 2:100012. doi: 10.1016/j.pdisas.2019.100012
64. Li N, Sun N, Cao C, Hou S, Gong Y. Review on visualization technology in simulation training system for major natural disasters. Nat Hazards. (2022) 112:1851–82. doi: 10.1007/s11069-022-05277-z
65. Huda SMA, Moh S. Survey on computation offloading in UAV-enabled mobile edge computing. J Netw Comput Appl. (2022) 201:103341. doi: 10.1016/j.jnca.2022.103341
66. Abrahamsen HB. A remotely piloted aircraft system in major incident management: concept and pilot, feasibility study. BMC Emerg Med. (2015) 15:12. doi: 10.1186/s12873-015-0036-3
67. Chuang CC, Rau JY, Lai MK, Shih CL. Combining unmanned aerial vehicles, and internet protocol cameras to reconstruct 3-D disaster scenes during rescue operations. Prehosp Emerg Care. (2019) 23:479–84. doi: 10.1080/10903127.2018.1528323
68. Gharahbaghian L, Anderson KL, Lobo V, Huang RW, Poffenberger CM, Nguyen PD. Point-of-care ultrasound in austere environments: a complete review of its utilization, pitfalls, and technique for common applications in austere settings. Emerg Med Clin North Am. (2017) 35:409–41. doi: 10.1016/j.emc.2016.12.007
69. Robin R, Murphy ST, Alexander K. Disaster robotics. In: Handbook of Robotics, eds B. Siciliano and O. Khatib (Berlin Heidelberg: Springer Nature) (2016), p. 1577–604. doi: 10.1007/978-3-319-32552-1_60
70. Kryvasheyeu Y, Chen H, Obradovich N, Moro E, Van Hentenryck P, Fowler J, et al. Rapid assessment of disaster damage using social media activity. Sci Adv. (2016) 2:e1500779. doi: 10.1126/sciadv.1500779
71. Devaraj A, Murthy D, Dontula A. Machine-learning methods for identifying social media-based requests for urgent help during hurricanes. Intern J Disaster Risk Reduct. (2020) 51:101757. doi: 10.1016/j.ijdrr.2020.101757
72. Huang CM, Chan E. Hyder AA. Web 20_internet social networking: a new tool for disaster management? - lessons from Taiwan. BMC Medical Inform Decis Mak. (2010) 10:5. doi: 10.1186/1472-6947-10-57
73. Daud S, Yusof M, Heo CC, Khoo LS, Singh MKC, Mahmood MS, et al. Applications of drone in disaster management: a scoping review. Sci Justice. (2022) 62:30–42. doi: 10.1016/j.scijus.2021.11.002
74. Pi Y, Nath ND, Behzadan AH. Convolutional neural networks for object detection in aerial imagery for disaster response and recovery. Adv Eng Inform. (2020) 43:101009. doi: 10.1016/j.aei.2019.101009
75. Jain T, Sibley A, Stryhn H, Hubloue I. Comparison of unmanned aerial vehicle technology-assisted triage versus standard practice in triaging casualties by paramedic students in a mass-casualty incident scenario. Prehosp Disaster Med. (2018) 33:375–80. doi: 10.1017/S1049023X18000559
76. Fernandez-Pacheco AN, Rodriguez LJ, Price MF, Perez ABG, Alonso NP, Rios MP. Drones at the service for training on mass casualty incident: a simulation study. Medicine. (2017) 96:e7159. doi: 10.1097/MD.0000000000007159
77. James CA, Wheelock KM, Woolliscroft JO. Machine learning: the next paradigm shift in medical education. Acad Med. (2021) 96:954–57. doi: 10.1097/ACM.0000000000003943
78. Kolachalama VB, Garg PS. Machine learning and medical education. NPJ Digit Med. (2018) 1:54. doi: 10.1038/s41746-018-0061-1
79. Hartawan DR, Purboyo TW, Setianingsih C. Disaster victims detection system using convolutional neural network method. In: 2019 IEEE International Conference on Industry 40 Artificial Intelligence and Commuication Technology (IAICT): IEEE Xplore (2019), p. 105–11. doi: 10.1109/ICIAICT.2019.8784782
80. Alzubaidi L, Zhang J, Humaidi AJ, Al-Dujaili A, Duan Y, Al-Shamma O, et al. Review of deep learning: concepts, CNN architectures, challenges, applications, future directions. J Big Data. (2021) 8:53. doi: 10.1186/s40537-021-00444-8
81. Valdez DB, Godmalin RAG. A deep learning approach of recognizing natural disasters on images using convolutional neural network and transfer learning. In: Proceedings of the International Conference on Artificial Intelligence and its Applications Jaipur (2021), p. 1–7. doi: 10.1145/3487923.3487927
82. Bhattacharya S, Reddy Maddikunta PK, Pham QV, Gadekallu TR, Krishnan SS, Chowdhary CL, et al. Deep learning and medical image processing for coronavirus (COVID-19) pandemic: a survey. Sustain Cities Soc. (2021) 65:102589. doi: 10.1016/j.scs.2020.102589
83. Yamashita R, Nishio M, Do RKG, Togashi K. Convolutional neural networks: an overview and application in radiology. Insights Imaging. (2018) 9:611–29. doi: 10.1007/s13244-018-0639-9
84. Sarki R, Ahmed K, Wang H, Zhang Y, Wang K. Automated detection of COVID-19 through convolutional neural network using chest X-ray images. PLoS ONE. (2022) 17:e0262052. doi: 10.1371/journal.pone.0262052
85. Daniel Shu Wei Ting, Lawrence Carin, Dzau V, Wong TY. Digital technology and COVID-19. Nat Med. (2020) 26:458. doi: 10.1038/s41591-020-0824-5
86. Carin L. On artificial intelligence and deep learning within medical education. Acad Med. (2020) 95:S10–1. doi: 10.1097/ACM.0000000000003630
87. Saiboon IM, Zahari F, Isa HM, Sabardin DM, Robertson CE. E-learning in teaching emergency disaster response among undergraduate medical students in Malaysia. Front Public Health. (2021) 9:628178. doi: 10.3389/fpubh.2021.628178
88. Sit C, Srinivasan R, Amlani A, Muthuswamy K, Azam A, Monzon L, et al. Attitudes and perceptions of UK medical students towards artificial intelligence and radiology: a multicentre survey. Insights Imaging. (2020) 11:14. doi: 10.1186/s13244-019-0830-7
89. Wood EA, Ange BL, Miller DD. Are we ready to integrate artificial intelligence literacy into medical school curriculum: students and faculty survey. J Med Educ Curric Dev. (2021) 8:23821205211024078. doi: 10.1177/23821205211024078
90. Ejaz H, McGrath H, Wong BL, Guise A, Vercauteren T, Shapey J. Artificial intelligence and medical education: a global mixed-methods study of medical students' perspectives. Digit Health. (2022) 8:1–11. doi: 10.1177/20552076221089099
91. Ishii E, Ebner DK, Kimura S, Agha-Mir-Salim L, Uchimido R, Celi LA. The advent of medical artificial intelligence: lessons from the Japanese approach. J Intensive Care. (2020) 8:35. doi: 10.1186/s40560-020-00452-5
92. Linardos V, Drakaki M, Tzionas P, Karnavas YL. Machine learning in disaster management: recent developments in methods and applications. Mach Learn Knowl Extra. (2022) 4:446–73. doi: 10.3390/make4020020
93. Harnessing Earth System Science Technology and Services to Reduce Disaster Risk-WMO Contributions [Internet]. Geneva: WMO (2022).
94. Nunavath V, Goodwin M, editors. The Use of AI in Disaster Management- A Systematic Literature Review. (2019) Paris, France: IEEE. doi: 10.1109/ICT-DM47966.2019.9032935
95. Ahmed L, Ahmad K, Said N, Qolomany B, Qadir J, Al-Fuqaha A. Active learning based federated learning for waste and natural disaster image classification. IEEE Access. (2020) 8:208518–31. doi: 10.1109/ACCESS.2020.3038676
96. Nguyen DC, Ding M, Pathirana PN, Seneviratne A, Li J, Poor HV. Federated learning for Internet of Things: a comprehensive survey. IEEE Commun Surv Tutor. (2021) 23:1622–58. doi: 10.1109/COMST.2021.3075439
97. Zhang Z, He N, Li D, Gao H, Gao T, Zhou C. Federated transfer learning for disaster classification in social computing networks. J Safety Sci Resilience. (2022) 3:15–23. doi: 10.1016/j.jnlssr.2021.10.007
98. Xia Q, Ye W, Tao Z, Wu J, Li Q. A survey of federated learning for edge computing: research problems and solutions. High Confidence Comput. (2021) 1:100008. doi: 10.1016/j.hcc.2021.100008
99. Jung YH. Virtual reality simulation for disaster preparedness training in hospitals: integrated review. J Med Internet Res. (2022) 24:e30600. doi: 10.2196/30600
100. Regal G, Schrom-Feiertag H, Migliorini M, Guarneri M, Di Giovanni D, D'Angelo A, et al. editors. Challenges in virtusl reality training for CRBN events. In: Extended Reality XR Salento 2022 Lecture Notes in Computer Science, Part II; 2022 July 6–8; Lecce, Italy: Springer (2022). doi: 10.1007/978-3-031-15553-6_6
101. Pottle J. Virtual reality and the transformation of medical education. Future Healthc J. (2019) 181–5. doi: 10.7861/fhj.2019-0036
102. Weiner DL, Rosman SL. Just-in-time training for disaster response in the austere environment. Clin Ped Emerg Med. (2019) 20:95–110. doi: 10.1016/j.cpem.2019.07.001
103. Ragazzoni L, Barco A, Echeverri L, Conti A, Linty M, Caviglia M, et al. Just-in-time training in a tertiary referral hospital during the COVID-19 Pandemic in Italy. Acad Med. (2021) 96:336–9. doi: 10.1097/ACM.0000000000003575
104. Wahyudin D, Hasegawa S, editors. The role of serious games in disaster and safety education: an integrative review. In: 25th International Conference on Computers in Education. New Zealand (2017).
105. IJgosse W, van Goor H, Rosman C, Luursema JM. Construct validity of a serious game for laparoscopic skills training: validation study. JMIR Serious Games. (2020) 8:e17222. doi: 10.2196/17222
106. Olgers TJ. bij de Weg AA, ter Maaten JC. Serious games for improving technical skills in medicine: scoping review. JMIR Serious Games. (2021) 9:e24093. doi: 10.2196/24093
107. Solinska-Nowak A, Magnuszewski P, Curl M, French A, Keating A, Mochizuki J, et al. An overview of serious games for disaster risk management – prospects and limitations for informing actions to arrest increasing risk. Int J Disaster Risk Reduct. (2018) 31:1013–29. doi: 10.1016/j.ijdrr.2018.09.001
108. Peek LA. Children and disasters: understanding vulnerability, developing capacities, and promoting resilience- an introduction. Child Youth Environ. (2008) 18:1–29. Available online at: https://www.jstor.org/stable/10.7721/chilyoutenvi.18.1.0001
109. Mutch C, Carol M, Dr Jay Marlowe D. “Sailing through a river of emotions”: capturing children's earthquake stories. Disaster Prev Manag. (2013) 22:445–55. doi: 10.1108/DPM-10-2013-0174
110. Omlor AJ, Schwarzel LS, Bewarder M, Casper M, Damm E, Danziger G, et al. Comparison of immersive and non-immersive virtual reality videos as substitute for in-hospital teaching during coronavirus lockdown: a survey with graduate medical students in Germany. Med Educ Online. (2022) 27:2101417. doi: 10.1080/10872981.2022.2101417
111. Bhatti I, Jones K, Richardson L, Foreman D, Lund J, Tierney G. E-learning vs lecture: which is the best approach to surgical teaching? Colorectal Dis. (2011) 13:459–62. doi: 10.1111/j.1463-1318.2009.02173.x
112. Huber T, Paschold M, Hansen C, Wunderling T, Lang H, Kneist W. New dimensions in surgical training: immersive virtual reality laparoscopic simulation exhilarates surgical staff. Surg Endosc. (2017) 31:4472–7. doi: 10.1007/s00464-017-5500-6
113. Renganayagalu Sk, Mallam SC, Nazir S. Effectiveness of VR head mounted displays in professional training: a systematic review. Technol Knowl Learn. (2021) 26:999–1041. doi: 10.1007/s10758-020-09489-9
114. Ashcroft J, Byrne MHV, Brennan PA, Davies RJ. Preparing medical students for a pandemic: a systematic review of student disaster training programmes. Postgrad Med J. (2021) 97:368–79. doi: 10.1136/postgradmedj-2020-137906
115. Shaw R. Thirty years of science, technology, and academia in disaster risk reduction and emerging responsibilities. Int J Disaster Risk Sci. (2020) 11:414–25. doi: 10.1007/s13753-020-00264-z
116. Martin F, Ndoye A, Wilkins P. Using learning analytics to enhance student learning in online courses based on quality matters standards. J Educ Technol Syst. (2016) 45:165–87. doi: 10.1177/0047239516656369
117. Álvarez-García C, Cámara-Anguita S, López-Hens JM, Granero-Moya N, López-Franco MD, María-Comino-Sanz I, et al. Development of the aerial remote triage system using drones in mass casualty scenarios: a survey of international experts. PLoS ONE. (2021) 16:e0242947. doi: 10.1371/journal.pone.0242947
118. Ray PP, Mukherjee M, Shu L. Internet of things for disaster management: state-of-the-art and prospects. IEEE Access. (2017) 5:18818–35. doi: 10.1109/ACCESS.2017.2752174
119. Javed F, Afzal MK, Sharif M, Kim BS. Internet of things (IoT) operating systems support, networking technologies, applications, and challenges: a comparative review. IEEE Commun Surv Tutor. (2018) 20:2062–100. doi: 10.1109/COMST.2018.2817685
120. Miraz MH, Ali M, Excell PS, Picking R, editors. A review on Internet of Things (IoT), Internet of Everything (IoE) and Internet of Nano Things (IoNT). In: Internet Technol Appl (ITA). Wrexham, UK: IEEE (2015). doi: 10.1109/ITechA.2015.7317398
121. Balasubramaniam S, Kangasharju J. Realizing the internet of nano things challenges solutions and applications. Computer. (2013) 46:62–8. doi: 10.1109/MC.2012.389
122. Kortuem G, Bandara AK, Smith N, Richards M, Petre M. Educating the Internet-of-Things generation. Computer. (2013) 46:53–61. doi: 10.1109/MC.2012.390
123. Arinta RR, Andi WRE. Natural disaster application on big data and machine learning: a review. In: 2019 4th International Conference on Information Technology, Information Systems and Electrical Engineering (ICITISEE). (2019), p. 249–54. doi: 10.1109/ICITISEE48480.2019.9003984
124. Sarker MNI, Peng Y, Yiran C, Shouse RC. Disaster resilience through big data: way to environmental sustainability. Int J Disaster Risk Reduct. (2020) 51:101769. doi: 10.1016/j.ijdrr.2020.101769
125. Joseph JK, Dev KA, Pradeepkkumar AP, Mohan M. Big data analytics and social media in disaster management. In:Samui P, Kim D, Ghosh C, , editors. Integrating Disaster Science and Management: Global Case Studies in Mitigation and Recovery. Netherlands: Candice Janco. (2018), p. 287–94.
126. Moreira F, Ferreira MJ, Cardoso A, editors. Higher education disruption through IoT and Big Data: a conceptual approach. In: Learning and Collaboration Techologies: Novel Learning Ecosystems; 2017 08 June. Vancouver, BC: Springer, 389–405. doi: 10.1007/978-3-319-58509-3_31
127. Klemke R, Eradze M, Antonaci A. The flipped MOOC: using gamification and learning analytics in MOOC design- a conceptual approach. Educ Sci. (2018) 8:25–38. doi: 10.3390/educsci8010025
128. Al-Balas M, Al-Balas HI, Jaber HM, Obeidat K, Al-Balas H, Aborajooh EA, et al. Distance learning in clinical medical education amid COVID-19 pandemic in Jordan: current situation, challenges, and perspectives. BMC Med Educ. (2020) 20:341. doi: 10.1186/s12909-020-02257-4
129. Chan KS, Zary N. Applications and challenges of implementing artificial intelligence in medical education: integrative review. JMIR Med Educ. (2019) 5:e13930. doi: 10.2196/13930
130. Jensen L, Konradsen F. A review of the use of virtual reality head-mounted displays in education and training. Educ Inform Technol. (2017) 23:1515–29. doi: 10.1007/s10639-017-9676-0
131. Jiang H, Vimalesvaran S, Wang JK, Lim KB, Mogali SR, Car LT. Virtual reality in medical students' education: scoping review. JMIR Med Educ. (2022) 8:e34860. doi: 10.2196/34860
132. Schoeb DS, Schwarz J, Hein S, Schlager D, Pohlmann PF, Frankenschmidt A, et al. Mixed reality for teaching catheter placement to medical students: a randomized single-blinded, prospective trial. BMC Med Educ. (2020) 20:510. doi: 10.1186/s12909-020-02450-5
Keywords: remote learning, remote technology, AI pedagogical approach, disaster medicine (DM), mobile edge computing (MEC), head-mounted display (HMD)
Citation: Kao C-L, Chien L-C, Wang M-C, Tang J-S, Huang P-C, Chuang C-C and Shih C-L (2023) The development of new remote technologies in disaster medicine education: A scoping review. Front. Public Health 11:1029558. doi: 10.3389/fpubh.2023.1029558
Received: 27 August 2022; Accepted: 27 February 2023;
Published: 24 March 2023.
Edited by:
Ozra Tabatabaei-Malazy, Tehran University of Medical Sciences, IranReviewed by:
Victoria Ramos Gonzalez, Carlos III Health Institute (ISCIII), SpainCopyright © 2023 Kao, Chien, Wang, Tang, Huang, Chuang and Shih. This is an open-access article distributed under the terms of the Creative Commons Attribution License (CC BY). The use, distribution or reproduction in other forums is permitted, provided the original author(s) and the copyright owner(s) are credited and that the original publication in this journal is cited, in accordance with accepted academic practice. No use, distribution or reproduction is permitted which does not comply with these terms.
*Correspondence: Chia-Chang Chuang, Y2h1YW5nZXJAbWFpbC5uY2t1LmVkdS50dw==; Chung-Liang Shih, c2hpaDExOTAwMUBuaGkuZ292LnR3
†These authors have contributed equally to this work and share last authorship
Disclaimer: All claims expressed in this article are solely those of the authors and do not necessarily represent those of their affiliated organizations, or those of the publisher, the editors and the reviewers. Any product that may be evaluated in this article or claim that may be made by its manufacturer is not guaranteed or endorsed by the publisher.
Research integrity at Frontiers
Learn more about the work of our research integrity team to safeguard the quality of each article we publish.